Abstract
The prosperity of urban life is dependent on its infrastructure. Urban underground infrastructure components (assets) are aging and need regular monitoring, maintenance and rehabilitation. These assets are often placed under pavements and in close vicinity to each other. Managing them in a coordinated way is rational considering costs and disruption of services and communities caused by each intervention on the different assets. Recently, interest in practice as well as in research has grown to manage urban infrastructures in a coordinated way. This article reviews journal articles and grey literature to evaluate managing these infrastructures in an integrated way (i.e. the highest level of coordination) and describe possible obstacles for doing so. This article identifies seven main challenges of integrated multi-infrastructure asset management (IMAM) that need to be addressed by practitioners and researchers. These challenges are related to: (i) dependencies and interdependencies, (ii) data quality, availability and interoperability, (iii) uncertainties in modelling and decision-making, (iv) comparability, (v) problems of scale, (vi) problems of fit and (vii) problems of interplay. This article provides details on these challenges and discusses future research and practical directions.
1. Introduction
The rehabilitation of our ageing municipal infrastructure will require substantial investments to maintain or improve its level of service (LOS). Due to the dense nature of urban fabrics, underground urban infrastructure assets are often placed under pavements and in close vicinity of each other, creating geographical dependencies and interdependencies. Those interdependencies can be a source of mutual vulnerabilities (Johansson & Hassel, Citation2010; Rinaldi, Peerenboom, & Kelly, Citation2001), e.g. the failure of one may cause cascading failures of the other interdependent infrastructures. The interdependent infrastructures can be vulnerable structurally (i.e. physically) and/or functionally (i.e. operationally) (see Ouyang, Hong, Mao, Yu, & Qi, Citation2009). Interdependencies can also cause extra costs if individual infrastructure asset management (IIAM) is applied, e.g. to replace a pipe under a road, one should incur the cost of opening and reinstating the road in addition to the cost of pipe replacement. On the other hand, the interdependencies can turn into opportunities to reduce intervention costs, community and service disruption if repeated works are avoided by coordinating and combining individual infrastructure interventions into common projects wherever possible. The total project costs are expected to rise when there is a lack of communication and coordination with other adjacent utilities. This can be observed directly by evaluating costs of repeating setup, excavation and backfilling, as well as indirectly by looking at social costs e.g. caused by disturbances, traffic disruptions and delays due to rehabilitation works. A utility can, in theory, avoid task repetition and share expenses by coordinating its work with other utilities. Exploiting these synergies is the focus of integrated multi-infrastructure asset management (IMAM). While this is, in theory, the goal of every rehabilitation and construction project, it is seldom realised in a well-structured way in practice.
Multiple studies in the two most recent decades have been conducted to integrate the interventions on multi-infrastructure assets. A large variety of terms is used, e.g. municipal infrastructure (Elsawah, Guerrero, & Moselhi, Citation2014), multi-utility (Tscheikner-Gratl, Egger, Rauch, & Kleidorfer, Citation2017), integrated infrastructure (Abu-Samra, Ahmed, & Amador, Citation2020), mixed assets (Saad & Hegazy, Citation2017), mixed infrastructures (Marzouk & Osama, Citation2015), municipal right-of-way (Carey & Lueke, Citation2013), and more, making literature search challenging. In this paper, the term IMAM is used to condense the meaning of these different terms without differentiating by infrastructure ownership (e.g. municipality or privately owned). Existing studies describe the process of holistically planning the rehabilitation of physical municipal assets, located near each other along with surface infrastructure including pavements or rails (i.e. co-located assets). They often focused on optimizing certain benefits from integrated interventions e.g. finding the optimum time of intervention while balancing cost, risk and performance (e.g. Abu Samra, Ahmed, Hammad, & Zayed, Citation2018; Carey & Lueke, Citation2013; Kleiner, Nafi, & Rajani, Citation2010), or prioritizing interventions among many different options (e.g. Elsawah, Bakry, & Moselhi, Citation2016; Shahata & Zayed, Citation2016; Tscheikner-Gratl, Sitzenfrei, Rauch, & Kleidorfer, Citation2016b).
A typical concept of integrated interventions for a pair of infrastructure assets is depicted in , showcasing different viewpoints on performance representation. As per ISO 55000:2014, performance is a ‘measurable result’, either qualitatively or quantitatively, that ‘can relate to assets in their ability to fulfil requirements or objectives’ (p. 12). Following this definition, condition, which is often used as a proxy of performance in literature, can be described in terms of structural performance for this figure. The (structural) performance of two co-located assets, in this example, pavement and sewer pipe (in ), deteriorates over time. When they reach their minimum acceptable performance thresholds individually, their times are often seen as the necessary and optimum times for their individual rehabilitation. Sometimes these rehabilitation times are close enough to each other to allow integrated rehabilitation by advancing or delaying the time of individual rehabilitation. The size of this window of opportunity is often decided by estimating or assuming the cost savings of the integrated rehabilitation in comparison to the individual ones. assumes a gradual performance deterioration of assets. In reality, assets’ performance deterioration is characterised by hybrid behaviour, combining gradual and sudden changes (). For instance, a pipe encrustation that reduces the pipe’s effective diameter is a gradual phenomenon, while pipe crack can be a sudden phenomenon (e.g. when caused by external factors). Both may affect the (functional or structural) performance of pipes. Although convey the concept of integrated rehabilitation, they may not be representative of practical cases.
Figure 1. Illustration of pavement and sewer performance deterioration and their integrated rehabilitation: (a) gradual deterioration (Ugarelli, Citation2008); (b) hybrid deterioration; (c) gradual deterioration with uncertainties and threshold areas; (d) discrete deterioration with uncertainties and threshold areas; (e) IMAM from risk point of view. (1) The figures are made using synthetic data to illustrate the concepts. (2) Effects of minor repairs on deterioration curves are not considered. (3) Different uncertainties for performance predictions of the two assets are considered to illustrate that there can be difference in uncertainties. Still, we are not yet able to say that uncertainty in one asset is higher than in the other, as no studies were found to provide this information.
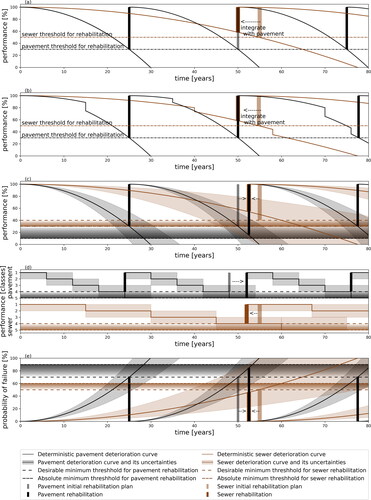
A more useful representation of asset deterioration and integrated rehabilitation may look more like the ones depicted in e). They depict uncertainties in performance predictions and these uncertainties may vary from asset type to asset type. Moreover, the minimum acceptable threshold for the assets’ performance may not be one line but rather an area, limited by one desirable, and one absolute threshold (Haas, Hudson, & Falls, Citation2015). shows a common simplification of deterioration that classifies the performance into discrete classes with connected needs for actions. It is the most common approach in the literature, as condition assessment of various infrastructures uses discrete condition classes which in turn are used as indicators of structural performance. This way the decision process is simplified by limiting the options. is a depiction from a risk point of view. The probability part of the risk is represented by the deterioration curves and the consequence part can be represented by the horizontal threshold lines. The probability of failure increases as the asset’s structural performance (i.e. condition) decreases. Rehabilitation of the asset (represented by the bold vertical lines) then decreases the probability of failure. To assess the probability, there is the need to select the (structural) performance-based deterioration curves per infrastructure (Marlow et al., Citation2009). The consequences of failure, on the other hand, can be represented by the horizontal lines which in fact are the maximum acceptable probability of failure or simply the intervention thresholds (here, we are talking only about the absolute threshold lines for simplicity). The threshold values vary for different assets depending on their consequences of failure. Based on the assessment of those consequences, utility managers set intervention threshold values for each group of assets. Comparatively, an asset with higher consequence of failure has a lower threshold value for the probability of failure (or higher threshold value for performance) than an asset with lower consequences of failure. Therefore, the intervention threshold lines can be used as proxies for the consequences of failure. Hence, can represent both parts of the risk (probability and consequences).
From , few challenges of IMAM are already identifiable: (1) Available data might not represent the real deterioration mechanism and might be of different qualities and quantities for different infrastructures. (2) Uncertainties in performance prediction obviously exist in all asset types. On top of that, the level or extent of uncertainties can be different for different asset types. (3) Flexibility in interventions may vary from asset type to asset type. Other challenges of IMAM are scattered in literature and addressed in a quite limited way. Consequently, this article reviews and classifies the existing approaches and highlights the challenges of IMAM in a structured way. It further outlines future research opportunities and knowledge gaps. The focus of this study is mainly on pavements, sewer pipes and drinking water distribution pipes (water pipes). One reason for this is that available IMAM literature is mostly focusing on these three asset types. Second, they can represent the other asset types in the urban fabric (e.g. gas pipes, electric and ICT cables) due to their similarities in layout and behaviour from an asset management point of view (Kielhauser, Adey, & Lethanh, Citation2017).
2. Review of existing approaches
Literature on IMAM is limited and mainly conducted in the last two decades. IMAM studies are listed in with attributes of domain, scale of application, objective and approach. Their domains have mostly been infrastructures with similar layouts, mainly pavements, sewer and water networks (the linear components). A few studies included other networks (e.g. electricity, gas and bridges) that share the same public space (e.g. Kielhauser, Citation2018; Marzouk & Osama, Citation2015) included electricity and gas networks). Saad and Hegazy (Citation2017) addressed co-located pavements, bridges and culverts. The studies did not address other structures such as manholes, blue-green infrastructure, treatment facilities, pump stations, reservoirs, valves, street furniture, etc., as individual assets but if at all, in combination with or as ‘parts’ of the pipes and pavements.
Table 1. Categorical literature review on IMAM.
The scale of application of the studies is categorised as project, network and organisational level. The distinction between project and network applicability is on the level of details: project-level studies are applied on asset level or group of assets that can be tendered as one project, whereas network-level studies are applied on the entire or part of a network without delivering asset-specific information. Studies applicable to organisations address the infrastructure managing authorities. Most of the studies are on the project level (see ) and are applicable to tactical and operational level decisions. However, few studies found ways to conduct IMAM studies also on a network level, which are useful for long-term planning and strategic decisions. For instance, Abu Samra et al. (Citation2018) and Abu-Samra et al. (Citation2020) assessed the benefits of IMAM compared to IIAM on a network level. The studies can provide good motives for strategic decision-makers to encourage and enforce coordinated works. Considering the objectives and approaches of the studies, the studies are categorised into three types: (1) guidelines and qualitative studies, (2) decision support studies and (3) others.
Guidelines and qualitative studies, being mostly grey literature, discussed the needs for IMAM, recommended or regulated AM practices, and identified best practices of coordination for utilities. The studies mostly addressed departments and organisations dealing with infrastructure AM. For instance, InfraGuide (Citation2003a) provided a five-step guideline for assessing, evaluating, and renewal of infrastructure networks in an integrated way. In the first step, an inventory of the networks was proposed that can be stored in a geographic information system (GIS) platform and thereby be accessible to stakeholders. The second step was an investigation (inspection) of the networks. The third and fourth steps proposed are condition assessment and performance evaluation of each component of the networks. The last step was making a renewal plan that identifies the best method and the best time of intervention based on cost, risk and environmental conditions. InfraGuide (Citation2003b) identified best practices of coordinated works by surveying the municipalities across Canada, reviewing publications, and use of experts’ knowledge. The best practices were categorised into five types: (1) Coordination practices, where the utilities are to make and implement their plans in coordination with each other. (2) Corridor upgrades, using a street section as a container and upgrading all its assets at once. (3) Restrictive practices, where some form of restrictions is put in place to not disturb the newly rehabilitated pavement. (4) Approval processes/communicating needs, in which funding approval relates to how effective the expenditure is planned. (5) Technical considerations, where the use of new technologies, models and other solutions are analysed to see the efficiency of infrastructure works.
Other literature in this category shows that other municipalities and states around the world are also guiding/regulating the utilities to coordinate their works in order to reduce service and community disruptions (e.g. see Dubbeldam et al. (Citation2020) for Amsterdam, Netherlands; Hafskjold (Citation2010) for municipalities of Norway; NSW SOCC (Citation2018) for New South Wales, Australia; NYC DOT (Citation2019) for New York City, USA). Moreover, a study by Yang, Ng, Xu, and Skitmore (Citation2018) describes the development of an IMAM framework by considering the integration of infrastructure AM data, processes, interdependencies and decisions. The study reviewed best practices in the United States, Canada, United Kingdom and Australasia and conducted interviews with experts in Hong Kong to identify the criteria for best practices of IMAM, and to set the basis for developing the framework. In general, this literature category provides the basic guidelines, concepts, and frameworks for researchers and utilities to start with and to dive deep into the complexities of IMAM.
Decision support studies are mainly aimed at comparing and prioritizing projects for interventions. Those studies can be grouped into the ones that provide a mathematical optimisation solution and the ones that abstained from this due to the high uncertainties in data and stakeholder weighting. They focused more on the ranking process and the involved assumptions to reflect real-world decision-making. Mathematical optimisation highlights one of the challenges of IMAM studies – the data quality and quantity are often not sufficient to allow any definitive statement about the optimality of a solution or to develop a meaningful objective function and set the system boundaries. Nevertheless, the studies are useful in a variety of practical problems, e.g. efficient budget expenditure, managing risk and improving LOS, and better intervention decisions, as long as its limitations are considered.
Mathematical optimisation has been the focus of most IMAM journal articles. Among the optimisation studies, most utilised multi-objective optimisation techniques to describe the trade-offs between risk, cost and LOS, and to find the optimum intervention time or optimum group of components for intervention (Abu-Samra et al., Citation2020; Abu Samra et al., Citation2018; Osman, Citation2016). Alinizzi, Chen, Labi, and Kandil (Citation2018) went one step further by adjusting the optimum time of interventions that are subject to certain interdependencies. The study argued that operations and maintenance phase interdependencies can affect the scheduled optimum time of interventions and hence, the optimum times need adjustments accordingly. Other studies utilised single-objective optimisation techniques to optimise one objective, for instance, costs (e.g. Carey & Lueke, Citation2013; Metayer, Torres-Machi, & Bastias, Citation2020; Saad & Hegazy, Citation2017). No IMAM study was found to account for the uncertainties of the future predictions and its effects on the optimisation results.
The methods of the studies that focused less on optimisation than on the decision variables and the mimicking of “real world” decision-making by providing tools for decision-makers rather than an optimum solution are mainly based on risk assessment approaches. The most challenging part of this type of study is the weighting of assets/projects’ importance among the different asset/project types. These weightings are prone to subjectivity since they are to be delivered by different stakeholders. Therefore, decision-making process is often made in terms of consequences that are often translated into a single measure (costs, therefore monetary units) (Abu-Samra et al., Citation2020; Abu Samra et al., Citation2018). Elsawah et al. (Citation2016) considered three dimensions (corresponding to 13 economic, social, and environmental factors) for the consequences of failure of sewer and water pipes to prioritise projects. The study then consulted practitioners and consultants to weigh the factors and used analytic hierarchy process (AHP) to compare the relative importance of the factors. Shahata and Zayed (Citation2016) considered four dimensions (corresponding to 18 economic, social, environmental and operational factors). To minimise the disagreements on the relative importance of the factors among the experts, they combined the AHP model with an interactive Delphi approach, which discusses the given weights with the experts in a workshop until a consensus among the experts and an acceptable consistency in the AHP model is achieved.
Other studies were miscellaneous that could not be grouped any other way due to their diverse objectives and approaches and limited in numbers. These studies, for instance, include modeling infrastructure interdependencies for AM purposes (e.g. Islam and Moselhi (Citation2012)). The study investigated the geographical co-location of adjacent assets. Torbaghan et al. (Citation2020) conducted on-site experiments to investigate how trenching affects pavements and pipes. The study concluded that even a high-quality backfilled trench accelerates pavement deterioration. Other than interdependency studies, this group also includes literature on evaluating the effect of data quality on IMAM decisions (van Riel, Langeveld, Herder, & Clemens, Citation2017), developing tools to enable a coordination environment (Halfawy, Citation2008, Citation2010), supporting tools for IMAM decisions (Wei et al., Citation2020), and identifying the causes of poor coordination among the different infrastructure organisations (Yilema & Gianoli, Citation2018).
3. Challenges in practicing IMAM
The authors have, based on the literature in , identified seven main challenges that can hinder the application and acceptance of IMAM if not addressed. These challenges are:
Dependencies and interdependencies: interactions exist among the infrastructures, but they are often not addressed from an AM perspective.
Data quality, availability and interoperability: the problem of data insufficiency and data silos often challenge IMAM practices.
Uncertainties in modelling and decision-making: the AM decisions are coupled with uncertainties, and this is usually not addressed in IMAM studies.
Comparability: the problem of comparing corridors containing different asset types.
Problems of scale: size and lifetime of asset types differ largely.
Problems of fit: the problem of horizontal and vertical location discrepancies that exist among the asset types.
Problems of interplay: the interaction problems that exist among the different stakeholders.
The following sections elaborate on these challenges and provide insights on the possibilities of addressing them. The use of the terms ‘problems of scale, fit and interplay’ are inspired by Young, Agrawal, King, and Sand (Citation1999) which used the terms for the challenges of environmental management by different institutions. Here, the definitions of the terms are adapted to comply with the context of IMAM.
3.1. Challenge I. Dependencies and interdependencies
Urban infrastructures are not standalone systems but are dependent on each other in many ways to improve their performance (Ouyang, Citation2014). At the same time, those dependencies can also be sources of risk and may decline each other’s performance (Alinizzi et al., Citation2018; Rogers et al., 2012), and can even cause cascading failures (Ouyang, Citation2014). The dependency of infrastructures can be unidirectional (dependency) or bidirectional (interdependency), and different types of infrastructure dependencies exist (see e.g. Gillette, Fisher, Peerenboom, & Whitfield, Citation2002 and Rinaldi et al., Citation2001). In general, dependencies among the infrastructures are studied extensively, see Ouyang (Citation2014) for a review of interdependent infrastructure modelling. On the very specific case, that is the analysis of infrastructure dependency and interdependency for the use of AM purposes, little information can be gleaned from the literature, especially when it comes to quantifying their impacts. These dependencies are seldom described or evaluated, let alone implemented into IMAM practices. If the dependencies of infrastructures are considered in IMAM, the relative weights of the infrastructures in MCDA and risk analysis approaches will differ from current approaches. This is because the dependencies impact the relative importance of infrastructure components.
Certain dependencies and interdependencies in the different phases of the infrastructure system’s life cycle exist (from the design and construction phase to the end of service and replacement). The dependencies of any two mutual infrastructure systems for AM purposes should be at least described from five dimensions: (1) types of dependencies (Rinaldi et al., Citation2001), (2) direction of dependencies (Alinizzi et al., Citation2018), (3) development phases of the interdependent systems (Alinizzi et al., Citation2018), (4) impact size and (5) impact frequency. The types of dependencies as explained in Rinaldi et al. (Citation2001) are geographical, logical, cyber and physical dependencies. The direction of dependency explains whether the relation is unidirectional or bidirectional. The different phases of the interdependent systems, considered in this study, are planning, design, construction, operations, monitoring, maintenance, end of service and replacement. Impact size refers to the severity or the extent of impact an asset imposes on the other asset during its certain phases. Impact frequency refers to a binary state defining whether the impact is a single event or a continuous one.
provides two examples of how the dependencies of two infrastructure systems for AM purposes can be described. In , types of dependencies are shown by a vertical line, phases of two systems (A and B) by two horizontal lines on both sides of the vertical line. The arrow direction represents the directionality of dependency, its size represents relative impact size, and the number of arrows represents whether it’s a one-time impact or a continuous impact. In example 1, system A is a water pipe and system B is the pavement above it. The leaking water pipe is washing away the clay particles around it. Multiple, small arrows directing from left to right indicate minor but continuous impact of the leaking pipe on the pavement’s structural performance. Example 1 is thus showing a geographical interdependency with small but continuous impacts of two systems in the operations phase. In example 2, system A is electric cables that need to be renewed, and system B is a water supply pump that conveys water powered by electricity. The renewal of electric cables temporarily impacts the supply of water. Example 2 is showing a unidirectional, physical dependency, with big impact but not frequent, of a water supply pump on electric cables. Asset managers deal mostly with operations and interventions (maintenance, monitoring, and replacement) phases of an infrastructure’s life cycle. This study’s focus is therefore on these two phases of infrastructures with geographically dependent infrastructures (co-located infrastructures). However, broadening of research is considered useful for further studies.
Figure 2. Dependencies of two (A and B) infrastructure systems seen from five perspectives for AM purposes.
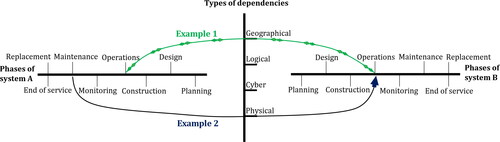
(Inter-) dependencies in the operations phase of co-located infrastructures can be further categorised into functioning-state (operating) and non-functioning-state (failure) dependencies. The former refers to how an asset is impacted by the functional performance of another co-located asset. It exists, for example, between pavements and water/sewer pipes (example 1). This type, if addressed and quantified, helps utilities understand the interactions among the infrastructure and can become good incentives for utilities to coordinate. Non-functioning-state dependencies refer to how an asset is impacted by the failure of another co-located asset. The failure impact may differ from asset type to asset type. For example, the impact of a pavement failure on a water pipe under the pavement may not be as severe as the impact of the water pipe’s failure on the pavement. The water pipe failure (bursting) may lead to part of the pavement’s failure (Alinizzi et al., Citation2018), but the opposite may not be necessarily true. So, delaying a water pipe’s intervention is coupled with the risk of failure of both the pipe and the pavement above it. In risk and multi-criteria decision-making studies, this dependency type can add a higher weight for water pipes compared to pavements.
(Inter-) dependencies in the intervention phase of co-located infrastructure systems refer to how an asset is impacted by the interventions taken on the other co-located asset. The impact frequency in the intervention phase can be continuous and/or temporary. For instance, interventions on underground assets often need excavation of pavement that will cause pavement service disruption (except only special cases of no-dig methods are applied). The disruption ends after the intervention is completed (which includes pavement resurfacing). Thus, a temporal impact is imposed by the interventions of underground assets on the pavement. Continuous impacts are then the effect of those interventions on the pavement in the long run. For instance, digging and backfilling part of a pavement to renew a water pipe may weaken the whole pavement section afterwards and lead to accelerated deterioration of the pavement. Alinizzi et al. (Citation2018) argued that irregularity in the reconstructed part of pavement is the reason for the accelerated deterioration of the whole pavement section. In an investigation by Eskandari Torbaghan et al. (Citation2020), two trenches in pavements were opened to install pipes, and then the pavements were restored, one by the lower quality and the other by the highest quality of the country’s standards. Their results showed that trenching, even after high-quality restoration, reduced the whole pavement section’s structural performance. From the study, we can learn that there is a factor in favour of integrated rehabilitation of whole pavement sections with co-located underground assets.
Although almost every IMAM study points out the existence of interdependencies in infrastructures, studies can be rarely found to quantify the interdependencies for AM purposes and utilise the benefits or minimise the risks hidden in the interactions. (Inter-) dependency of infrastructures is indeed a key motivator for IMAM when looking at the reduction of interruptions or costs. The reason for the lack of studies quantifying the interdependencies can be found in the challenging nature of such a quantification as well as in the lack of established methods. The studies’ focus is mainly on benefiting from the economy of scale as a result of the proximity of infrastructures (e.g. Kielhauser et al., Citation2017; Marzouk & Osama, Citation2015; Nafi & Kleiner, Citation2010). With the help of emerging technologies and digitalisation of infrastructures, there is a high possibility of understanding and measuring the degree of interconnectedness between different infrastructures, for instance, the use of digital twins (DT coupled with real-time monitoring has the capacity of observing some of the relations between the infrastructures, see Callcut, Agliozzo, Varga, & Mcmillan, Citation2021; Conejos Fuertes, Martínez Alzamora, Hervás Carot, & Alonso Campos, Citation2020). In this section, we proposed a way how to consider the interdependencies for AM purposes. More studies need to be conducted to estimate to what extent those interdependencies exist and how they affect our decisions. If interdependencies are represented in risk and multi-criteria decision-making problems, the importance of the assets may look different.
3.2. Challenge II. Data quality, availability, and interoperability
IMAM, like every other form of AM, relies on data to allow informed decisions. Due to the broad scope of IMAM, a multitude of data is required to do so. Tscheikner-Gratl (Citation2016) has defined four kinds of necessary data: (1) infrastructure network data, including the physical properties, (2) assets’ condition (structural performance) data, (3) sensors and models’ output data, providing information about functional performance, and allow for scenario analysis and control and (4) data on external influences, including all necessary information on influences from outside the set system boundaries on assets’ performance. All these data may not be of sufficient quality, availability or interoperable between different AM decision levels of the same infrastructure (Okwori, Pericault, Ugarelli, Viklander, & Hedström, Citation2021) or adjacent infrastructures, to actively support data-driven IIAM and IMAM.
Every kind of data has its own inherent challenges and depends strongly on the utility and operator, but one of the main factors affecting the quality and availability of any of the above data type is that data collection is costly. Infrastructure network data are often by no means complete, especially for smaller utilities, with missing historical data on infrastructure development and aging. Comparing the condition data of pavements, sewer and water pipes in urban areas; it can be found that data can be collected with lower, moderate and higher costs and efforts, respectively, due to assets’ accessibility reasons. Therefore, the condition data of sewer and water pipes of any network is usually far from complete. For instance, in the work of Caradot et al. (Citation2020), the network in their case study initially consisted of around 235,000 segments but only almost half of the data was complete and useable for modelling.
Sensor and model output data availability is increasing due to the trend towards digitalisation and hydroinformatics (Makropoulos & Savic, Citation2019). As the amount of sensor data is increasing, the challenge of data handling arises, encompassing data selection, storage and processing. Also, the management of the sensors themselves must be considered, either on their own or preferably included in the operation and maintenance scheme of IMAM. Finally, data on external influences are often difficult to include in IMAM decisions since they may be unknown, or not shared due to security reasons, and or unavailability of information. Among the four types of data, data on external influences are very diverse and varies from case to case. Due to its nebulous definition and lack of study to pinpoint its importance, this type of data is not actively and systematically collected by utilities. Of the four data types, data on structural (and functional) performance is the core of the challenge in this section, as their impact on the decisions is high while their availability is low compared to the other kinds of data (e.g. network data).
For pavements, data on structural and functional performance are relatively easy to collect and of higher availability. This reason is founded on its easy accessibility to be inspected, in contrast to underground infrastructures. Furthermore, semi and fully automated data collection methods, in addition to manual methods, have evolved (Pierce, McGovern, & Zimmerman, Citation2013). These methods have helped the pavement management sector to efficiently collect abundant data (Peraka & Biligiri, Citation2020). The quality and type of data depend on whether the data is collected for decisions on the network level (less detail) or on the project level (more detail) (Flintsch & McGhee, Citation2009). Studies (e.g. Ong, Noureldin, & Sinha, Citation2010; Peraka & Biligiri, Citation2020) imply that with good quality control and assurance, even higher quality data can be achieved that can better support pavement AM.
In sewer pipes, condition data collection is normally conducted visually by using closed-circuit television (CCTV) cameras (Tscheikner-Gratl et al., Citation2019). Due to lower accessibility of the buried pipes for inspections, as well as the need for manual interpretation and coding of defects from the CCTV footage, data collection is costly and time-consuming, and hence data is often limited. Furthermore, predicting the future conditions or the other pipes’ conditions using available condition data results in poor-quality outcomes. One of the reasons is the aggregation of the condition scores (Rokstad & Ugarelli, Citation2015, Citation2016). Another problem with the condition data is the involvement of subjectivity in CCTV-based condition assessment (Dirksen et al., Citation2013). Hence, sewer condition data, due to its quality and availability problems, contribute poorly to predictive models (Hawari, Alkadour, Elmasry, & Zayed, Citation2020), but the outcomes of those predictive models are needed for IMAM plans. There are some techniques that handle some of the problems to some extent. For missing data, evidential reasoning and Bayesian Belief Network techniques can be used (Hawari et al., Citation2020). For the subjectivity inherent to CCTV inspections, Kumar, Abraham, Jahanshahi, Iseley, and Starr (Citation2018), Meijer, Scholten, Clemens, and Knobbe (Citation2019) and Myrans, Everson, and Kapelan (Citation2018) proposed effective automated image processing techniques using machine learning algorithms.
Condition data for water distribution pipes often do not exist but proxies are used for assessing the intervention need. The reason is that accessibility to water pipes is very limited because of lack of manholes, high-pressure operating pipes, and water quality concerns among other reasons. Nevertheless, techniques are available to inspect and collect data from water pipes, provided that high costs are incurred. These costs can be argued for major transmission pipelines where the consequences of failure are very high (Kleiner & Rajani, Citation2001), but for the distribution pipes, the high costs of inspection are usually not justified by the benefits achieved from the inspection data. Also, as opposed to condition assessment of sewers, there is no ‘classification standard’ for water distribution pipes. Researchers and practitioners assess the need for intervention of water pipes differently – they model the reliability of pipe breaks based on statistical models (see Kleiner & Rajani, Citation2001), or AI-based and fuzzy logic-based models (see Dawood, Elwakil, Novoa, & Gárate Delgado, Citation2020), or sometimes physical models (see Rajani & Kleiner, Citation2001). To feed those models, input data are often missing, or are not reliable about the structural characteristics, surrounding environment, or short periods of data are registered (Tscheikner-Gratl, Sitzenfrei, Rauch, & Kleidorfer, Citation2016a). In a review, Barton, Farewell, Hallett, and Acland (Citation2019) listed 13 dominant factors affecting pipe breaks which are categorised into three groups: intrinsic, operational and environmental. The practice of collecting data about these factors differs from utility to utility, but generally, it is common that data on only a few factors can be available. The data problems in water pipes lead to less reliability in the pipes’ predictions and hence, affect AM decisions.
From the interoperability perspective, current tools do not provide enough support for data interoperability (Yang et al., Citation2018). Interoperability of data is also a challenging topic in the digitalisation of infrastructures as the collected data is huge and extremely heterogeneous (Silva, Khan, & Han, Citation2018). Modern techniques of infrastructure AM such as the use of Building Information Modelling (BIM), BIM integration with GIS (BIM-GIS) and DT have reported interoperability as the major challenge (Costin, Adibfar, Hu, & Chen, Citation2018; Garramone et al., Citation2020; Lu, Xie, Heaton, Parlikad, & Schooling, Citation2020). Although the use of these techniques is not yet studied for IMAM practices, an exacerbated interoperability problem should be expected. In IMAM, the problem of data interoperability is twofold: interoperability between different decision levels of the same infrastructure (vertical interoperability) and adjacent infrastructures (horizontal interoperability). Okwori et al. (Citation2021) discussed the problems of data silos that exist between different decision levels of water/sewer utilities in IIAM due to a lack of data interoperability and quality issues. Halfawy (Citation2008, Citation2010) discussed the problem of data heterogeneity among different infrastructures and developed a prototype tool that can deal with the heterogeneity of a few data sources. The framework developed by Okwori et al. (Citation2021) for data-driven AM of single infrastructure, and the framework proposed by Yang et al. (Citation2018) for IMAM may be used as a guide for developing a framework for data-driven AM of multi-infrastructure.
These data problems may affect IMAM practices. For short-term IMAM planning, data quality and availability of individual infrastructures has little effect on IMAM decisions as the decision is often made based either on their current performance, intuition or triggered by other infrastructures (van Riel, Langeveld, et al., Citation2017). But, on a tactical and strategic level, one needs to predict the future performance of the assets under consideration with an acceptable level of uncertainty. To do so, the data is often not enough of high quality and availability. Moreover, one can easily notice that there are inequalities in data quality and availability among the different infrastructures. These inequalities may affect IMAM decisions. Research is missing to investigate how inequalities among the infrastructures in terms of data quality and availability affect IMAM for medium- and long-term decisions. It would also be interesting to know if the data inequalities are quantifiable/comparable, and how low-/high-quality data of one infrastructure affect other infrastructures to practice IMAM. In terms of data quality, maintenance effectiveness is another aspect that would affect the quality of decision-making. Most IMAM publications do not address these matters. The focus is often on the application of models based on available data rather than data quality and management considerations. Recently, the adoption of emerging technologies such as internet of things (IoT) in civil infrastructures has become a hot topic for researchers and practitioners as it is proposing enormous opportunities for quality data collection. However, the use of such technologies requires rethinking and reconfiguration of data collection systems from their roots (Brous, Janssen, & Herder, Citation2019; Li, Yang, & Sitzenfrei, Citation2020).
3.3. Challenge III. Uncertainties in modelling and decision-making
The process of assessing and predicting performance, and deciding on interventions involves uncertainties (Caradot et al., Citation2020; Dirksen et al., Citation2013; Okuda, Suzuki, & Kohtake, Citation2018; Xu, Qiang, Chen, Liu, & Cao, Citation2018). Not accounting for the uncertainties in predicting integrated interventions may lead to sub-optimal decisions (Yuan, Citation2017). Different types and different dimensions of uncertainties exist that require consideration. In this text, we will follow the taxonomy from Walker et al. (Citation2003), which identified dimensions and types of uncertainties for model-based decision-making, to describe how uncertainties are addressed in the context of IIAM and IMAM. Furthermore, as mentioned earlier, data about the assets’ performance is of high importance for the assets’ future performance predictions, and so are for their intervention decisions. Hence, special focus is put on, but not only on, the uncertainties in performance predictions.
In pavement AM plans, despite many probabilistic studies in literature, a comprehensive understanding of uncertainties (and risk) is still not achieved (Menendez & Gharaibeh, Citation2017). Deterministic models for performance predictions, which do not consider uncertainties, are still often used, especially for low-volume roads (Rose et al., Citation2018). However, uncertainties due to environmental conditions, traffic load (Kırbaş & Karaşahin, Citation2016; Rose et al., Citation2018), construction quality and soil characteristics (Okuda et al., Citation2018) affect pavement behaviour predictions, and hence decision-making. There exist studies (e.g. Han & Lee, Citation2016; Okuda et al., Citation2018), that point out those uncertainties, their impact on pavement AM plans, and methods to deal with them. Okuda et al. (Citation2018) proposed a bootstrapping method to quantify the uncertainties of pavement condition prediction for the use of decision-makers. Han and Lee (Citation2016) applied a Bayesian Markov hazard model to assess the life expectancy of pavements while quantifying their uncertainty ranges. Menendez and Gharaibeh (Citation2017) provided a methodology to assess the risks by considering uncertainties in pavement current condition, performance prediction, available funds and unit cost of maintenance and rehabilitation. The study found that uncertainties in performance (or condition) prediction have the highest impact on the network’s AM plan among the others. Other studies proposed methods to deal with uncertainties. For instance, Fani, Golroo, Ali Mirhassani, and Gandomi (Citation2022) proposed an optimisation approach to find the optimal maintenance and rehabilitation plans on a network level while considering the uncertainties in condition deterioration rate and budget allocations. García-Segura et al. (Citation2022) introduced an optimisation approach to find the optimum maintenance programme and minimise the uncertainties of condition prediction.
The limitations of sewer condition data quality and availability clearly have an influence on its current condition assessment as well as on the prediction of its future conditions. Two review articles (Hawari et al., Citation2020; Mohammadi et al., Citation2019) concluded that condition prediction models are adequately developed to predict the future condition with enough precision, but data quality and availability remains the main barrier. Caradot et al. (Citation2020) quantified uncertainties in assessing the current and future conditions using a dataset of a sewer network in Berlin, Germany. Their findings show that the probability of correctly assessing the current condition class of a pipe in bad condition is 81% and their deterioration model results show that condition prediction at 100 years has ±12% uncertainty for the pipes in poor condition. Rokstad and Ugarelli (Citation2015) and Caradot et al. (Citation2018) showed that condition prediction on a pipe level is connected with high uncertainties that can hardly support rehabilitation decisions but can support inspection prioritisations. Their results for network-level condition prediction contradicted each other. The former study concluded that network-level prediction has low accuracy while the latter concluded that it has high accuracy. Both studies used the same models i.e. GompitZ (see Le Gat, Citation2008) and Random Forest, but different case studies (Oslo and Berlin, respectively). The main difference between the two is that different condition classification protocols are applied. The condition data used by Rokstad and Ugarelli (Citation2015) are an aggregation of different defect types (representing both functional and structural performance). Furthermore, the conditions are classified into five classes. On the other hand, the condition data used in Caradot et al. (Citation2018) represents more the structural performance and not an aggregation of different defect types. The conditions in Caradot et al. (Citation2018) study are classified into three classes. The contradicting results suggest that input data uncertainties can be different from case to case. Research is needed to see how and to which degree the variations in uncertainties can affect sewer AM and IMAM decisions.
In water supply AM, although countless studies exist that estimate future pipe break rates, less attention has been paid to quantifying the uncertainties of these predictions. Khameneh, Lavasani, Nodehi, and Arjmandi (Citation2020) combined fuzzy set theory with fault tree analysis as an approach to deal with uncertainties under data unavailability. Xu et al. (Citation2018) utilised a statistical approach to model pipe failure rate considering only pipe age relation with pipe breaks and used a Poisson process to assess the uncertainties of their model. Other studies compared different models’ performance with regard to their uncertainties by quantifying each model’s uncertainties (e.g. Kabir, Tesfamariam, Loeppky, & Sadiq, Citation2015). Similar to sewer AM, there is a lack of studies to evaluate how the uncertainties in water pipes failure prediction influence water AM and IMAM decisions.
Addressing uncertainties in the context of IMAM is found to be very rare in literature. van Riel, Langeveld, et al. (Citation2017) used a serious gaming research tool to evaluate the influence of information quality, which is related to data and model uncertainties, on IMAM decisions. They concluded that short-term IMAM decisions are mostly based on current conditions, intuition, or as a result of interactions with other utilities. For longer-term decisions, the uncertainties of individual infrastructure influencing IMAM decisions remain unaddressed. Moreover, unexpected sudden changes in the structural or functional performance of an asset can occur due to extreme environmental conditions, imperfect knowledge about the state of the assets, or the interventions on other assets. These changes are theoretically addressed in some studies (e.g. Abu-Samra et al., Citation2020; Kielhauser, Citation2018) to some extent but a real-world case study application is missing. Abu-Samra et al. (Citation2020) assumed that 10% of failures can occur unexpectedly in their network under the study due to extreme conditions. The failures were randomly distributed over the network and their effects were included in their optimisation model. These assumptions would also merit further research.
Comparing the uncertainties addressed in each IIAM and IMAM, it can be seen that only model, input, and parameter uncertainties are addressed in IIAM to some extent, and even less considered in IMAM. In sewer and water asset management most of the existing practices either partly consider uncertainty (the so called aleatory or inherent variability) or do not take it into account at all (Yuan, Citation2017). A holistic view of uncertainties in IMAM is missing and how the combination or linkage of different infrastructures and models affect uncertainties is still unanswered. Based on the inequalities in data, we expect inequalities in uncertainties among the different infrastructures. Research is needed to evaluate the effect of uncertainty inequalities on IMAM decisions. The same goes for the question of what limitations in terms of prediction timeframe within an acceptable level of uncertainty and what this acceptable level should be. Moreover, as the future performance is uncertain, so is the optimum time of intervention, rendering optimisation efforts difficult. Additionally, the urban infrastructure is also heavily influenced by its surroundings, and therefore uncertainties about future developments (e.g. urbanisation, climate change and policy change) will have an influence on the long-term planning and need to be considered, for example by exploratory modelling using what-if scenarios or scenario pathways (Urich & Rauch, Citation2014), or by adopting maintenance flexibility not only in IIAM (van den Boomen, Spaan, Shang, & Wolfert, Citation2020; Zhang & Yuan, Citation2021; Zuluaga & Sánchez-silva, Citation2020) but also in IMAM practices.
3.4. Challenge IV. Comparability
Comparing assets is at the heart of any asset management approach used for rehabilitation planning on a tactical level. In essence, the prioritisation of projects boils down to a comparison of assets. In IIAM, the challenge is to compare assets of the same infrastructure which is mainly done by using risk-based approaches (CitationAnbari, Tabesh, & Roozbahani, 2017; Michaud & Apostolakis, Citation2006; Saha & Ksaibati, Citation2016). In IMAM, it is about the comparison of corridors (groups of assets of different infrastructures). In comparing the corridors, the challenge is exacerbated as the comparison is not only among the assets of the same infrastructure but also among the assets of different infrastructures. The assets within the corridor diverge in terms of performance indicators, measurement scales and weighting. The corridors can still be compared using risk-based approaches given the differences are addressed carefully.
On the probability part of the risk, there lies the probability of failure of a performance indicator of each asset within the corridor. Performance indicators vary from infrastructure to infrastructure, in terms of both type and measurement scale. In water pipes, pipe break rate (which is a measure of functional performance and a proxy for structural performance) is the usual type of performance indicator for intervention decisions. The measurement scale of the performance of the water pipes is determined by a binary state, functioning or not functioning (Kielhauser et al., Citation2017), although the water pipes show a large range of states from sweating to full-blown breaks. In sewer pipes, usually condition (which is a measure of structural performance), is used as an indicator for intervention decisions which is mostly independent of or only partially linked to functional performance. The scale of the condition is determined in common practice by using a few discrete condition classes (Elsawah et al., Citation2014, Citation2016; Haugen, Citation2018), for example ranging from class 1 (best) to class 5 (worst).
Sewers with the worst condition class may still be able to fulfil their functions for years, even decades. The condition class is just an attribute based on CCTV inspection and does not necessarily indicate pipe failure. In other words, the bandwidth of an acceptable level of degradation differs per type of defect. Although this increases uncertainty, it also gives a much larger window of opportunity for AM actions. For pavements, intervention decisions are based on functional performance, but condition (the structural performance) is mostly used as a proxy for functional performance due to a more direct link between the two, especially In network-level decisions (Papageorgiou, 2019; Shah, Jain, Tiwari, & Jain, Citation2013). International Roughness Index (IRI) is a well-known indicator of pavements for intervention decisions, its scale ranges from 0 to 16 mm/m in continuous form. Pavements are ‘visible’ infrastructure systems, and their deteriorated condition can affect end users spontaneously, while underground infrastructure assets are ‘hidden’, and their deteriorated condition does not usually affect end users unless the assets fail to deliver their functional requirements.
This leads to the question of how those differences affect assets’ comparability and on which indicator the probability side of risk should be based. One approach, often used in literature, unifies the scales across the different assets by conversion. For example, Saad and Hegazy (Citation2017) converted the IRI range from 0–16 to 0–100, so it can be the same scale as bridges and culverts’ condition index. However, converting all the indicators into a common indicator may lead to increased uncertainties e.g. due to upscaling of categorical values (e.g. condition classes). In contrast, Osman (Citation2016) used LOS as the indicator for all infrastructure assets and then used condition grades of different assets as a proxy for LOS. Having the fact in mind that the structural performance of a water pipe is characterised by a binary state, sewer pipe by its discrete condition classes, and pavement by its continuous condition state (Kielhauser et al., Citation2017), combining these different measurement scales for integrated interventions cannot be straightforward. Moreover, some indicators, e.g. condition classes of sewer pipes, may not be proper representatives for LOS.
Another way is defining and applying a common intervention indicator in contrast to combining different existing ones for each asset type. Kielhauser et al. (Citation2017) used a common indicator ‘the probability of failure to provide an adequate level of service’ (p. 364) for all infrastructure assets in their study (electricity, gas, sewer, water and roads), thus using one functional performance indicator for all. This indicator seems reasonable in theory but in practice might be challenging for some asset types. For example, data on sewer pipes are currently being collected in a way that does not provide information on LOS but about the condition state. Probably a practical indicator would be ‘the probability of failure to be beyond an intervention threshold’. Intervention threshold is a more comprehensive term. It can contain LOS and condition states in it. The intervention threshold for each asset type is usually defined by their utilities. After all, Kielhauser et al. (Citation2017) implicitly used intervention thresholds rather than LOS.
On the consequences part of the risk, the consequences of failure of assets are not widely addressed compared to the probability of failure in IMAM studies, due to difficulties, uncertainties and subjectivities in their assessment (Elsawah et al., Citation2016; Salman & Salem, Citation2012; Shahata & Zayed, Citation2016). Furthermore, one often has to consider multiple consequence dimensions for each asset type (e.g. health, environmental and economic consequences). Some studies of IMAM consider risk-based approaches and hence consequences of failure (e.g. Carey & Lueke, Citation2013; Elsawah et al., Citation2016; Shahata & Zayed, Citation2010, Citation2016; Tscheikner-Gratl, Citation2016). Shahata and Zayed (Citation2016) used a combination of Delphi-AHP approaches to deal with the subjectivities in assessing the consequences and Elsawah et al. (Citation2016) developed a novel dynamic weighting system to integrate the risk of individual assets into integrated corridor segments. Kielhauser (Citation2018) used LOS as a criterion for intervention decisions but did not consider the relative importance of each component. If one incorporates the relevant importance of assets in , there can be different threshold areas for different groups of assets having different severities of consequences.
Moreover, the consequences of failure are usually used to weigh the importance of assets and eventually the corridors. Weighting a corridor’s importance among the other corridors is a more complex task than just using a weight per asset and combining them in a corridor as often done in the literature (for instance, Tscheikner-Gratl et al. (Citation2016b) prioritised some corridors over the others using weights per asset). In these cases, at least two more factors may be considered in the importance of corridors. One (inter-) dependencies of assets within the corridors, especially the failure impact of assets on the other interdependent assets, and two, the flexibility of the intervention time of an asset within the corridor. These two points are explained in the following paragraph.
First, the assets within a corridor are geographically interdependent and failure of some asset types has a higher impact on its interdependent assets than vice versa. For example, a water pipe burst will lead to the failure of the pavement above it, but the opposite is not necessarily true. This impact advocates for higher weights for water pipes than pavements. In comparing two corridors each containing a water pipe and a pavement section, the corridor in which its water pipe has a higher probability of failure than the pavement of the other corridor may be prioritised. The reason is that the failure impact of a water pipe is higher than a pavement; it fails itself and fails the pavement too. Second, as IMAM is a practice of delaying or advancing the interventions (or performing so-called flexible maintenance) to be able to integrate with other asset’s interventions, such flexibility varies from asset type to asset type.
Some asset types may wait longer for intervention after reaching their threshold point (e.g. pavements can operate with reduced LOS), and others may need immediate intervention (e.g. a water pipe burst needs immediate intervention). Intervention flexibility for pavements is discussed in Haas et al. (Citation2015) in which the authors argued that there is an intervention time range from desirable to absolute (see the horizontal lines in ). The same idea can be applied to pipe systems but a method of quantifying the time from a desirable to an absolute intervention threshold and comparing it with other networks is missing and needs further investigation. Once the intervention time range from desirable to absolute for each asset type is known, we can estimate how much delaying/advancing a corridor’s intervention is possible. Corridors with low flexibility in delaying/advancing interventions may get prioritised over the corridors with higher flexibility. The intervention flexibility in conjunction with the failure impact discussed above are two additional factors affecting the weights of corridors – an interesting research gap that needs to be filled.
To summarise, the following points are identified as research gaps of this section: is it feasible to integrate such diverse infrastructures with their different practices of management under one umbrella? In IMAM literature, individual management approaches are often linked together using risk-based approaches and/or multi-criteria decision support systems (e.g. Shahata & Zayed, Citation2016; Tscheikner-Gratl et al., Citation2016b). The challenge lies, therefore, mainly in the selection of the right and adequate performance indicators for the infrastructures, in the measurement and combining of the indicators, and in weighing the importance of asset types in a corridor containing different infrastructure assets, for which each guidance is missing. Also, the varying degrees of maintenance and intervention flexibility of different infrastructures and its effects on IMAM decisions need further research.
3.5. Challenge V. Problems of scale
When combining the interventions on different infrastructures, problems of spatial and temporal scale arise. The different sizes and different life expectancies of our infrastructure assets make it challenging to coordinate intervention efforts without incurring losses (either of useful lifespan or costs) to one of the infrastructures involved. Also, the scale differences play an important role in sharing the cost of common works of an integrated intervention among the involved utilities. Here the focus is on applying IMAM to project levels. However, there can be instances where high-level (e.g. network or sub-network) restructuring of urban networks takes place for transition and adaptation purposes (Guy, Marvin, & Moss, Citation2001). In these circumstances, none of the tools, data and decisions on the project level remain relevant. These circumstances are beyond the scope of this paper but merit further research.
The problem of spatial scale difference refers to the different sizes of co-located infrastructure assets that may not be simply grouped as one corridor in a cost-effective way. Pavement segments are usually separated by intersections, sewer pipes by manholes and water pipes by valves, bends, tees, reducers or similar. Often the length of one segment of an infrastructure is not equal to the length of the other co-located segment of another infrastructure. A pavement segment usually contains many segments of sewer/water pipes. Some IMAM studies raised the problem of differences but did not provide answers to the question of what segmentation could be cost-effective. For example, Elsawah et al. (Citation2016) recognised the scale differences but in the end, after consultation with municipalities, decided that the corridor segment is based on the pavement segment. Tscheikner-Gratl (Citation2016) also used the pavement segment as the governing corridor segment for intervention prioritisations. Neither of the studies considered if it was the best possible way because there is the concern of grouping assets of good and bad conditions into a corridor rehabilitation project in which the assets with good conditions do not need to be rehabilitated. As a result, this segmentation approach may not be the optimum approach in terms of overall cost savings.
Other studies did address the scale differences in their works and approached the problem differently. For instance, Shahata and Zayed (Citation2010) proposed that corridor segments should be variable and be based on the asset which triggers the intervention. Doing so brings us back to the interdependencies (Section 3.1): trenching part of a pavement accelerates the pavement segment’s deterioration. So, the pavement must incur a cost due to accelerated deterioration. The distribution of these costs is still a matter of research, see e.g. van Riel, Langeveld, et al. (Citation2017). As a result, the cost-effectiveness of this segmentation approach also needs to be evaluated. A possible solution could be an evaluation of the different segmentation approaches and comparing them with each other in terms of benefits. Moreover, punctual-type assets (e.g. manholes, valves, pumps and sensors), areal-type assets (e.g. infiltration facilities) and road furniture (e.g. signs and poles) have their own challenges to be included in IMAM practices. They are rarely treated as individual assets but if at all, in combination as ‘parts’ of the pipes and pavements.
Temporal scale difference refers to the different lifetimes and construction years of infrastructure assets. For instance, the service life of a typical asphalt road is between 15 and 30 years, while of a sewer pipe is 50–100 years. Once the two components are renewed at the same time, the next time it may be economically not justifiable to integrate the renewal of these two assets (sewer pipe may be in very good condition and does not need renewal at the time of pavement renewal, see ). Probably, the pavement section would be rehabilitated alone. Hence, the temporal scale difference implies that even for the same assets that once were integrated for intervention, the next time an integrated intervention may not be a reasonable choice.
Spatial and temporal scale differences are also important factors in sharing the cost of common works among the utilities. For works in common (e.g. excavation), each utility’s share to pay should be based on its share size of excavation and backfilling (which is partly related to the degree of the spatial scale differences) and its willingness to delay/advance the intervention time. There is also a third factor affecting cost-sharing models: the degree of co-location. This is discussed in the following section.
3.6. Challenge VI. Problems of fit
Problems of fit mean that interventions on different infrastructure assets may not fit into one project, completely or partially due to location discrepancies. The adjacent assets sometimes may have significant distances (horizontally and vertically) from each other that geographical interdependencies between them are disconnected and integrated intervention is not triggered. The proximity of infrastructures is dependent on local conditions, such as the depth of the infrastructure assets, urban density and design standards and norms.
For horizontal location discrepancy, consider the case of a road and a sewer pipe that lies under a pedestrian path, and the intervention on the sewer pipe does not interfere with the road. In this case, the assets’ co-location intervention interdependency is disconnected, and intervention on both assets does not fit into one project with assumed cost savings. However, one may still utilise some benefits of economies of scale due to the assets’ proximity in which sharing the machinery, site office, manpower, etc., would reduce some costs. There are many cases where intervention on one asset does not disturb the other asset’s service or condition at all. Most of the studies assumed that the assets are ‘ideally’ and completely co-located, and they grouped them in a common corridor segment. However, Mair, Zischg, Rauch, and Sitzenfrei (Citation2017) found that around 15–20% of water/sewer pipes cannot be found under the roads. Islam and Moselhi (Citation2012), in a more detailed study, modelled horizontally co-located assets by using a GIS environment. The study described co-located assets if the open-cut area (i.e. the area necessary to be opened to be able to conduct an intervention) of an asset overlaps with the open-cut area of another asset. In their case study, 72 out of 700 (∼10%) water main segments did not overlap with the road segments and hence were independent for interventions.
For vertical location discrepancy, consider the typical case of a sewer pipe that is located well below the top layer of a pavement, and the pavement only needs the top layer reconstruction. In this case, integrating the intervention of sewer pipes with the pavement may not be triggered, and if it does, cost-sharing for the reconstruction of the other layers of pavement among the utilities needs to be defined. This problem is unaddressed in IMAM studies.
The points discussed above implies that as a prerequisite for an integrated intervention decision, an investigation should be conducted to see if there are intervention interdependencies among the assets, and most importantly, how ‘co-location’ can be defined in order to objectively frame a cost-sharing model for the utilities. In contrast to current studies (e.g. Abu Samra et al., Citation2018; Mair et al., Citation2017) which defined co-location as a binary state (co-located or not co-located), there is a need to assess how much the assets are co-located i.e. degree of co-location (Islam & Moselhi, Citation2012). The degree of co-location, along with the degree of spatial and temporal scale differences, enables us to quantify the actual savings of common works and specify utility-specific cost savings. However, defining the degree of co-location could be challenging as infrastructure network data on underground assets may not be of high enough resolution.
3.7. Challenge VII. Problems of interplay
The holistic planning of interventions requires interaction between different stakeholders. These interactions are required within the AM levels of the same infrastructure (vertical coordination) as well as across the different infrastructures (horizontal coordination) (Hafskjold, Citation2010; Halfawy, Citation2008). Furthermore, motivation to coordinate among the infrastructures varies depending on incentives (often in terms of financial benefits) and personal motivation. Those incentives are still not fully understood and evaluated, which makes it difficult to advocate for IMAM. The lack of motivation to coordinate originates from the various challenges discussed in this paper. However, the main reason is the diversity of the infrastructures in terms of data (3.2), uncertainties (3.3), behaviour and approach (3.4), as well as unclear incentives for each utility, and the lack of a common coordination tool to systematically streamline the coordination process.
Vertical coordination has its own challenges and is discussed in IIAM-related studies (see e.g. Parlikad and Jafari (Citation2016)). Horizontal coordination is the added challenge while practicing IMAM. Horizontal coordination can be further categorised into coordination between different infrastructures of the same organisation (e.g. sewer and water systems managed by a municipality) and coordination between infrastructures of different organisations if the infrastructures are managed by different organisations. Horizontal coordination and especially coordination among different organisations is very difficult as the managers of different infrastructures have different priorities and budgets (Hafskjold, Citation2010) as well as different institutional arrangements and regulatory frameworks (see Yilema & Gianoli, Citation2018). Cross-organisation coordination problems have received little attention in IMAM studies and therefore addressing them is a research gap. InfraGuide (Citation2003b) summarised practical guidelines for coordination among sewer, water, and pavements. The study expects increased administrative costs due to changes in the organisational arrangements (increased staff and establishment of committees). Nakamura and Sakai (Citation2020) studied coordination problems between train operation and train infrastructure management organisations. The study concluded that to better facilitate coordination, informal communication mechanisms at individual levels are important. This is also expected to hold true for other coordination efforts.
Incentives may significantly trigger coordinated interventions, but they are not explored as much as needed. Utilities usually ask the question: ‘what is my benefit if I coordinate?’ A clear answer by IMAM studies is not provided (Yang et al., Citation2018). IMAM deals with decisions of delaying or advancing interventions to be able to integrate with other asset’s interventions. It is hard to argue to a manager that his/her intervention plan needs to be advanced/delayed in order to adapt to the other infrastructures’ plan. The studies usually assume an overall saving for all utilities, but utility-specific benefits are yet to be explored. A study by Carey and Lueke (Citation2013) quantified utility-specific savings in their framework. They quantified average savings of pavement, sewer, and water utilities, and showed that road utility can benefit the maximum among the utilities. However, the framework was applied to a synthetic case study. Real-world applications still need to be demonstrated. Tscheikner-Gratl (Citation2016) in one of his case studies estimated an overall average saving of 15.6% but did not specify utility-specific savings and pointed out that the savings may not be equally distributed.
From an economic and financial but also environmental perspective, both direct and indirect effects are to be considered, including the imposition of opportunity costs and externalities (Marlow, Pearson, MacDonald, Whitten, & Burn, Citation2011). It is to be mentioned that not only tangible incentives (i.e. cost savings) should be used to motivate IMAM practices as there are many other reasons that justify IMAM practices. For instance, intangible incentives (e.g. reduced service and community disruptions, impact on the utility image) usually play a significant role in choosing IMAM practices, thanks to interdependencies. Exceptions are interventions on pavements with minor repairs or interventions in very lightly populated areas. Nonetheless, quantification of intangible incentives is a challenge and few studies looked into this. Abu Samra et al. (Citation2018) developed a framework for IMAM and compared savings (cost savings and indirect savings that account for reduced traffic delay) gained from IMAM practices compared to IIAM practices. The framework was applied to a small network of pavement and water in Kelowna, Canada. The results show that up to 33% of direct and 50% of indirect costs can be saved if IMAM is practiced. In big and densely populated cities, the benefits from intangible incentives may by far be bigger and more important than tangible incentives. However, the benefits of intangible incentives including reductions in service and community disruptions due to interventions have received very little attention in analytical research. In populated cities in developed countries such as Sydney (NSW SOCC, Citation2018), New York (NYC DOT, Citation2019) and Amsterdam (Dubbeldam et al., Citation2020) coordinated activity of some sort is a necessity rather than an option because the reduction of intervention disruptions is crucial.
IMAM can also influence the project and tender management. For instance, Osman (Citation2016) argued that packing multi-infrastructure works can lead to better tender management of civil works. Amador and Magnuson (Citation2011) indicated that multi-infrastructure works in small municipalities can attract more contractors (and hence higher competitiveness) due to their bigger budget size. However, the limitations of those synergies are not clearly defined and need further clarification. Sometimes the competitiveness may be deterred by large tenders in markets where there are few large contractors. Subdividing tenders into multiple contracts to attract more contractors is a common practice in project management with a limited pool of large contractors. These (potential) synergies are thus dependent on the conditions in the local market, which may also change over time.
Asset managers also require tools to streamline the coordination by sharing data and information among the stakeholders (Yang et al., Citation2018). A shared platform that can integrate the data, processes and models (e.g. integrates different databases, objectively finds the optimum intervention time, evaluates the uncertainties, identifies and analyses scenarios, can be accessible among the stakeholders, etc.) is missing to streamline the coordination among the stakeholders. Rogers et al. (2012) argued for the need for an integrated database consisting of the condition data of pavement and underground infrastructures. Halfawy (Citation2008) discussed the challenges and the different possibilities of a tool that can enable the utilities to coordinate and streamline their works. The study discussed the pros and cons of centralised and non-centralised database architectures and came up with preferring the centralised database architecture. The study suggests that the tool should consist of an integrated set of independent but interoperable sub-modules, and lessons should be learned from the Architecture, Engineering and Construction (AEC) industry in the development of the tool, given the similarities and differences that exist between municipal infrastructure management and AEC. It was concluded that a multitier architecture tool could be the best option. The study also developed a prototype tool. However, a full-scale application of the tool needs to be examined.
Other studies focused on integrating a 3-dimension model of assets with GIS for various applications including infrastructure AM. See e.g. Liu et al. (Citation2017) for a review on the integration of BIM and GIS. Digitalisation of infrastructures under the umbrella of ‘Smart Cities’ for better infrastructure management is an ongoing discussion and implementation. A number of cities across the globe are embracing digitalisation at different speeds and to varying degrees. It is expected that smart cities will ease and automatise decision-making processes (Silva et al., Citation2018). This would be an ideal solution for the problem of interplay, but the barriers to digitalisation are still high for some infrastructures and require extensive research and experience to become a mature solution.
4. Conclusions
To benefit from the synergies of co-located assets, coordinated AM works are promising, although there can be different levels of coordination among the stakeholders. This article investigated the feasibility of the highest level (i.e. ideal way) of coordination in which infrastructures’ AM differences (in terms of data, methods, and predictabilities) and dependencies are dealt with in an optimised way, costs are shared objectively, and tools are available to streamline the coordination. One does not have to address all the challenges to be able to proceed with IMAM and reap benefits from it. IMAM can still be possible with a lower level of coordination. On the other hand, integrated interventions in case of minor repairs of an asset may not be justifiable. Minor repairs might be less disruptive to the users and therefore would be more cost-effective than considering the combination of interventions with other infrastructures.
This study identified seven main challenges in practicing IMAM: (I) dependencies and interdependencies, (II) Data quality, availability and interoperability, (III) Uncertainties in modelling and decision-making, (IV) Comparability, (V) problems of scale, (VI) Problems of fit and (VII) Problems of interplay. These challenges are not always mutually exclusive; there can be cases where an issue is transversal to more than a challenge. For instance, the intervention on an underground asset affecting the pavement in a localised point is a scale problem but also a dependency problem; the data interoperability problem can be often overlapped with the problem of interplay. Also, the increasing digitalisation of infrastructures is opening a wide range of opportunities for IMAM. Informed use of the emerging technologies can turn out as game changers regarding some of the challenges (I, II and VII). However, civil infrastructures and their management, by their nature, have high inertia against transformative changes. Nevertheless, many utilities around the world are in the process of transforming to digital management of their infrastructures, although with varying speeds and to different degrees. A thorough understanding of the benefits, risks and organisations’ reconfiguration need for embracing digitalisation is yet to unfold.
In the light of the identified challenges, endeavours should be directed towards addressing the highlighted knowledge gaps:
Dependencies and interdependencies – focus should be directed towards quantifying their impact and frequency in the different stages of the asset life cycle. In this study, we proposed a way how to consider the interdependencies for AM purposes. Detail studies on how the interdependencies influence our decision on IMAM should be further explored. If interdependencies are represented in risk and multi-criteria decision-making problems, the importance of the assets may look different.
Data quality, availability and interoperability – data are often not enough of high quality and availability to support IMAM decisions. Interoperability problems of data add more challenges to this issue. Moreover, inequalities exist in data quality and availability among the different infrastructures. Research is missing to investigate how these issues are affecting IMAM decisions. Utilities are entering into the era of digitalisation. Digitalisation is promising vast opportunities including high quality and quantities of data. Research should be focused on guiding the utilities about what types of data to be collected and how those data should be stored, managed, and utilised efficiently among the stakeholders.
Uncertainties in modelling and decision-making – this is the most unaddressed issue in IMAM. Based on the inequalities in data, inequalities in uncertainties among the different infrastructures should be expected. Studies are missing to evaluate the effect of unequal uncertainties among the infrastructures on IMAM decisions. The same goes for answering what level of uncertainties is acceptable for IMAM decisions. The uncertainties should be minimised whenever possible but also awareness about the issue should be raised. We need to ‘live’ with uncertainties but knowingly and cautiously, and we need to understand the consequences of the uncertainties involved in the decisions we make. One way is to conduct what-if scenarios and make separate intervention plans for each scenario to find robust solutions.
Comparability – assets of the same infrastructure are compared using risk-based approaches that use consequences of failure of each asset group as the criteria. The same approach may be used in comparing the corridors, but the differences in terms of performance indicators and the complexities in weighting the assets of different infrastructures need to be not ignored. These complexities arise due to the interdependencies between infrastructures and the different flexibilities in the intervention time of assets.
Problems of scale and fit– unlike most of the IMAM studies which ignore or assume binary states of the scale and fit differences, there is a need in defining degrees of scale and fit differences among the assets. It can then be used to develop applicable and more objective cost-sharing schemes among the utilities. Current work is in progress to develop a cost-sharing model for works in common in an IMAM context. The cost-sharing model can specify each utility’s savings per integrated intervention, greatly helping in the understanding of the utilities about cost savings of integrated interventions.
Problems of interplay – there are horizontal and vertical coordination problems among the infrastructures. The problems’ roots are not only in the vast diversity of the infrastructures but also in the lack of motivation among the stakeholders and tools to streamline the coordination. Different studies are needed to address the problem from a variety of aspects e.g. engineering, social and technological aspects.
Abbreviations | ||
LOS | = | level of service |
AM | = | asset management |
IIAM | = | individual infrastructure asset management |
IMAM | = | integrated multi-infrastructure asset management |
ISO | = | International Organization for Standardization |
ICT | = | information and communications technology |
AHP | = | analytic hierarchy process |
MCDA | = | multi-criteria decision analysis |
GIS | = | geographic information system |
DT | = | digital twins |
CCT V | = | closed-circuit television |
BIM | = | building information modelling |
IoT | = | internet of things |
IRI | = | international roughness index |
AEC | = | architecture, engineering and construction |
Disclosure statement
No potential conflict of interest was reported by the authors.
References
- Abu-Samra, S., Ahmed, M., & Amador, L. (2020). Asset management framework for integrated municipal infrastructure. Journal of Infrastructure Systems, 26(4), 04020039. doi:10.1061/(ASCE)IS.1943-555X.0000580
- Abu Samra, S., Ahmed, M., Hammad, A., & Zayed, T. (2018). Multiobjective framework for managing municipal integrated infrastructure. Journal of Construction Engineering and Management, 144(1), 04017091. doi:10.1061/(ASCE)CO.1943-7862.0001402
- Alinizzi, M., Chen, S., Labi, S., & Kandil, A. (2018). A methodology to account for one-way infrastructure interdependency in preservation activity scheduling. Computer-Aided Civil and Infrastructure Engineering, 33(11), 905–925. doi:10.1111/mice.12380
- Amador, L. E., & Magnuson, S. (2011). Adjacency modeling for coordination of investments in infrastructure asset management: Case study of Kindersley, Saskatchewan, Canada. Transportation Research Record: Journal of the Transportation Research Board, 2246(1), 8–15. doi:10.3141/2246-02
- Anbari, M. J., Tabesh, M., & Roozbahani, A. (2017). Risk assessment model to prioritize sewer pipes inspection in wastewater collection networks. Journal of Environmental Management, 190, 91–101. doi:10.1016/j.jenvman.2016.12.052.
- Barton, N. A., Farewell, T. S., Hallett, S. H., & Acland, T. F. (2019). Improving pipe failure predictions: Factors effecting pipe failure in drinking water networks. Water Research, 164, 114926. doi:10.1016/j.watres.2019.114926.
- Brous, P., Janssen, M., & Herder, P. (2019). Internet of Things adoption for reconfiguring decision-making processes in asset management. Business Process Management Journal, 25(3), 495–511. doi:10.1108/BPMJ-11-2017-0328
- Callcut, M., Agliozzo, J.-P. C., Varga, L., & Mcmillan, L. (2021). Digital twins in civil infrastructure systems. Sustainability, 13(20), 11549. doi:10.3390/su132011549
- Caradot, N., Riechel, M., Fesneau, M., Hernandez, N., Torres, A., Sonnenberg, H., … Rouault, P. (2018). Practical benchmarking of statistical and machine learning models for predicting the condition of sewer pipes in Berlin, Germany. Journal of Hydroinformatics, 20(5), 1131–1147. doi:10.2166/hydro.2018.217
- Caradot, N., Riechel, M., Rouault, P., Caradot, A., Lengemann, N., Eckert, E., … Cherqui, F. (2020). The influence of condition assessment uncertainties on sewer deterioration modelling. Structure and Infrastructure Engineering, 16(2), 287–296. doi:10.1080/15732479.2019.1653938
- Carey, B. D., & Lueke, J. S. (2013). Optimized holistic municipal right-of-way capital improvement planning. Canadian Journal of Civil Engineering, 40(12), 1244–1251. doi:10.1139/cjce-2012-0183
- Conejos Fuertes, P., Martínez Alzamora, F., Hervás Carot, M., & Alonso Campos, J. (2020). Building and exploiting a digital twin for the management of drinking water distribution networks. Urban Water Journal, 17, 704–713. doi:10.1080/1573062X.2020.1771382
- Costin, A., Adibfar, A., Hu, H., & Chen, S. S. (2018). Building Information Modeling (BIM) for transportation infrastructure – Literature review, applications, challenges, and recommendations. Automation in Construction, 94, 257–281. doi:10.1016/j.autcon.2018.07.001
- Dawood, T., Elwakil, E., Novoa, H. M., & Gárate Delgado, J. F. (2020). Water pipe failure prediction and risk models: State-of-the-art review. Canadian Journal of Civil Engineering, 47(10), 1117–1127. doi:10.1139/cjce-2019-0481
- Dirksen, J., Clemens, F. H. L. R., Korving, H., Cherqui, F., Le Gauffre, P., Ertl, T., … Snaterse, C. T. M. (2013). The consistency of visual sewer inspection data. Structure and Infrastructure Engineering, 9(3), 214–228. doi:10.1080/15732479.2010.541265
- Dubbeldam, F., Begeer, R., Smiers, R., van Hoek, H., de Jong, J., Vlaar, T., … Veerkamp, J. (2020). Werkplan Ondergrond 2020: Integrale benadering Amsterdamse Ondergrond [Underground work plan 2020: Integrated approach to the Amsterdam’s underground]. Amsterdam, the Netherlands: Gemeente Amsterdam.
- Elsawah, H., Bakry, I., & Moselhi, O. (2016). Decision support model for integrated risk assessment and prioritization of intervention plans of municipal infrastructure. Journal of Pipeline Systems Engineering and Practice, 7(4), 04016010. doi:10.1061/(ASCE)PS.1949-1204.0000245
- Elsawah, H., Guerrero, M., & Moselhi, O. (2014). Decision support model for integrated intervention plans of municipal infrastructure. ICSI 2014: Creating Infrastructure for a Sustainable World - Proceedings of the 2014 International Conference on Sustainable Infrastructure (pp. 1039–1050). doi:10.1061/9780784478745.098
- Fani, A., Golroo, A., Ali Mirhassani, S., & Gandomi, A. H. (2022). Pavement maintenance and rehabilitation planning optimisation under budget and pavement deterioration uncertainty. International Journal of Pavement Engineering, 23(2), 414–424. doi:10.1080/10298436.2020.1748628
- Flintsch, G. W., & McGhee, K. K. (2009). NCHRP Synthesis 401: Quality management of pavement condition data collection. Washington, DC: Transportation Research Board of the National Academies.
- García-Segura, T., Montalbán-Domingo, L., Llopis-Castelló, D., Lepech, M. D., Sanz, M. A., & Pellicer, E. (2022). Incorporating pavement deterioration uncertainty into pavement management optimization. International Journal of Pavement Engineering, 23(6), 2062–2073. doi:10.1080/10298436.2020.1837827
- Garramone, M., Moretti, N., Scaioni, M., Ellul, C., Re Cecconi, F., & Dejaco, M. C. (2020). BIM and GIS integration for infrastructure asset management: A bibliometric analysis. ISPRS Annals of the Photogrammetry, Remote Sensing and Spatial Information Sciences, VI-4/W1-2020(4/W1), 77–84. doi:10.5194/isprs-annals-VI-4-W1-2020-77-2020
- Gillette, J., Fisher, R., Peerenboom, J., & Whitfield, R. (2002). Analyzing water/wastewater infrastructure interdependencies. Analyzing water/wastewater infrastructure interdependencies (No. ANL/DIS/CP-107254). Lemont, IL: Argonne National Lab. www.ipd.anl.gov/anlpubs/2002/03/42598.pdf.
- Guy, S., Marvin, S., & Moss, T. (2001). Urban infrastructure in transition: Networks, buildings plans. London: Earthscan.
- Haas, R., Hudson, W. R., & Falls, L. C. (2015). Pavement asset management. Beverly, MA: Scrivener Publishing LLC.
- Hafskjold, L. S. (2010). SIP - Future rehabilitation strategies for physical infrastructure: Coordination of rehabilitation planning and measures - co-infrastructure interactions. SINTEF (Report No. SBF IN A10316). doi:10.1080/02508068008685878
- Halfawy, M. R. (2008). Integration of municipal infrastructure asset management processes: Challenges and solutions. Journal of Computing in Civil Engineering, 22(3), 216–229. doi:10.1061/(ASCE)0887-3801(2008)22:3(216)
- Halfawy, M. R. (2010). Municipal information models and federated software architecture for implementing integrated infrastructure management environments. Automation in Construction, 19(4), 433–446. doi:10.1016/j.autcon.2009.11.013
- Han, D., & Lee, S. H. (2016). Stochastic fo recasting of life expectancies considering multi-maintenance criteria and localized uncertainties in the pavement-deterioration process. Journal of Testing and Evaluation, 44(1), 20140246–20140140. doi:10.1520/JTE20140246
- Haugen, H. J. (2018). Rørinspeksjon av hovedledninger for vann og avløp 234/2018 [Interior inspection of main pipes for water and wastewater]. Hamar, Norway: Norsk Vann BA.
- Hawari, A., Alkadour, F., Elmasry, M., & Zayed, T. (2020). A state of the art review on condition assessment models developed for sewer pipelines. Engineering Applications of Artificial Intelligence, 93, 103721. doi:10.1016/j.engappai.2020.103721
- InfraGuide. (2003a). An Integrated approach to assessment and evaluation of municipal road, sewer and water networks. Federation of Canadian Municipalities and National Research Council, National Guide to Sustainable Municipal Infrastructure, Ottawa.
- InfraGuide. (2003b). Coordinating infrastructure works: A best practice by the national guide to sustainable municipal infrastructure. In Federation of Canadian Municipalities and National Research Council, National Guide to Sustainable Municipal Infrastructure, Ottawa.
- Islam, T., & Moselhi, O. (2012). Modeling geospatial interdependence for integrated municipal infrastructure. Journal of Infrastructure Systems, 18(2), 68–74. doi:10.1061/(ASCE)IS.1943-555X.0000065
- ISO 55000:2014 (2014). Asset management - overview, principles and terminology. Geneva, Switzerland: International Organization for Standardization.
- Johansson, J., & Hassel, H. (2010). An approach for modelling interdependent infrastructures in the context of vulnerability analysis. Reliability Engineering & System Safety, 95(12), 1335–1344. doi:10.1016/j.ress.2010.06.010
- Kabir, G., Tesfamariam, S., Loeppky, J., & Sadiq, R. (2015). Integrating Bayesian linear regression with ordered weighted averaging: Uncertainty analysis for predicting water main failures. ASCE-ASME Journal of Risk and Uncertainty in Engineering Systems, Part A: Civil Engineering, 1(3), 04015007. doi:10.1061/AJRUA6.0000820
- Kammouh, O., Nogal, M., Binnekamp, R., & Wolfert, A. R. M. R. (2021). Multi-system intervention optimization for interdependent infrastructure. Automation in Construction, 127, 103698. doi:10.1016/j.autcon.2021.103698
- Khameneh, A. P., Lavasani, M. S. M., Nodehi, N. R., & Arjmandi, R. (2020). Water distribution network failure analysis under uncertainty. International Journal of Environmental Science and Technology, 17(1), 421–432. doi:10.1007/s13762-019-02362-y
- Kielhauser, C. (2018). A method for determining optimal intervention programs for interrelated infrastructure networks (Doctoral dissertation). Zürich, Switzerland: ETH Zurich. doi:10.3929/ethz-b-000269313
- Kielhauser, C., Adey, B. T., & Lethanh, N. (2017). Investigation of a static and a dynamic neighbourhood methodology to develop work programs for multiple close municipal infrastructure networks. Structure and Infrastructure Engineering, 13(3), 361–389. doi:10.1080/15732479.2016.1162818
- Kırbaş, U., & Karaşahin, M. (2016). Performance models for hot mix asphalt pavements in urban roads. Construction and Building Materials, 116, 281–288. doi:10.1016/j.conbuildmat.2016.04.118
- Kleiner, Y., Nafi, A., & Rajani, B. (2010). Planning renewal of water mains while considering deterioration, economies of scale and adjacent infrastructure. Water Supply, 10(6), 897–906. doi:10.2166/ws.2010.571
- Kleiner, Y., & Rajani, B. (2001). Comprehensive review of structural deterioration of water mains: Statistical models Rajani, B.B.; Kleiner, Y. NRCC-43722. Urban Water, 3(3), 131–150. www.elsevier.com/locate/urbwat. doi:10.1016/S1462-0758(01)00032-2
- Kumar, S. S., Abraham, D. M., Jahanshahi, M. R., Iseley, T., & Starr, J. (2018). Automated defect classification in sewer closed circuit television inspections using deep convolutional neural networks. Automation in Construction, 91, 273–283. doi:10.1016/j.autcon.2018.03.028
- Le Gat, Y. (2008). Modelling the deterioration process of drainage pipelines. Urban Water Journal, 5(2), 97–106. doi:10.1080/15730620801939398
- Li, J., Yang, X., & Sitzenfrei, R. (2020). Rethinking the Framework of Smart Water System. Review Water, 12(2), 412. doi:10.3390/w12020412
- Liu, X., Wang, X., Wright, G., Cheng, J. C. P., Li, X., & Liu, R. (2017). A state-of-the-art review on the integration of Building Information Modeling (BIM) and Geographic Information System (GIS). ISPRS International Journal of Geo-Information, 6(2), 53–21. doi:10.3390/ijgi6020053
- Lu, Q., Xie, X., Heaton, J., Parlikad, A. K., & Schooling, J. (2020). From BIM towards digital twin: Strategy and future development for smart asset management. Studies in Computational Intelligence, 853, 392–404. doi:10.1007/978-3-030-27477-1_30
- Mair, M., Zischg, J., Rauch, W., & Sitzenfrei, R. (2017). Where to find water pipes and sewers?-On the correlation of infrastructure networks in the urban environment. Water (Switzerland), 9(2), 146. doi:10.3390/w9020146
- Makropoulos, C., & Savic, D. A. (2019). Urban hydroinformatics: Past, present and future. Water, 11(10), 1959. doi:10.3390/w11101959
- Marlow, D., Davis, P., Trans, D., Beale, D., Burn, S., & Urquhart, A. (2009). Remaining asset life: A state of the art review. Water environment research foundation. London: IWA Publishing. doi:10.2166/9781780403427
- Marlow, D., Pearson, L., MacDonald, D. H., Whitten, S., & Burn, S. (2011). A framework for considering externalities in urban water asset management. Water Science and Technology, 64(11), 2199–2206. doi:10.2166/wst.2011.789
- Marzouk, M., & Osama, A. (2015). Fuzzy approach for optimum replacement time of mixed infrastructures. Civil Engineering and Environmental Systems, 32(3), 269–280. doi:10.1080/10286608.2014.1002715
- Meijer, D., Scholten, L., Clemens, F., & Knobbe, A. (2019). A defect classification methodology for sewer image sets with convolutional neural networks. Automation in Construction, 104, 281–298. doi:10.1016/j.autcon.2019.04.013
- Menendez, J. R., & Gharaibeh, N. G. (2017). Incorporating risk and uncertainty into infrastructure asset management plans for pavement networks. Journal of Infrastructure Systems, 23(4), 04017019. doi:10.1061/(ASCE)IS.1943-555X.0000379
- Metayer, G., Torres-Machi, C., & Bastias, A. (2020). A proposed framework for the integrated management of municipal infrastructure. Construction Research Congress, 2020, 846–855. 10.1061/9780784482865%0Ahttps://trid.trb.org/view/1760497.
- Michaud, D., & Apostolakis, G. E. (2006). Methodology for ranking the elements of water-supply networks. Journal of Infrastructure Systems, 12(4), 230–242. doi:10.1061/(ASCE)1076-0342(2006)12:4(230)
- Mohammadi, M. M., Najafi, M., Kaushal, V., Serajiantehrani, R., Salehabadi, N., & Ashoori, T. (2019). Sewer pipes condition prediction models: A state-of-the-art review. Infrastructures, 4(4), 64–16. doi:10.3390/infrastructures4040064
- Myrans, J., Everson, R., & Kapelan, Z. (2018). Automated detection of faults in sewers using CCTV image sequences. Automation in Construction, 95, 64–71. doi:10.1016/j.autcon.2018.08.005
- Nafi, A., & Kleiner, Y. (2010). Scheduling renewal of water pipes while considering adjacency of infrastructure works and economies of scale. Journal of Water Resources Planning and Management, 136(5), 519–530. doi:10.1061/(ASCE)WR.1943-5452.0000062
- Nakamura, E., & Sakai, H. (2020). Does vertical integration facilitate coordination between infrastructure management and train operating units in the rail sector? Implications for Japanese railways. Utilities Policy, 66, 101099. doi:10.1016/j.jup.2020.101099
- NSW SOCC. (2018). Guide to codes and practices for streets opening. NSW, Australia: NSW SOCC.
- NYC DOT. (2019). Highway rules, title 34, Chapter 2: Rules of the city of New York. New York, NY: Department of Transportation.
- Okuda, T., Suzuki, K., & Kohtake, N. (2018). Non-parametric prediction interval estimate for uncertainty quantification of the prediction of road pavement deterioration. IEEE Conference on Intelligent Transportation Systems, Proceedings, ITSC, 2018-Novem, 824–830. doi:10.1109/ITSC.2018.8569337
- Okwori, E., Pericault, Y., Ugarelli, R., Viklander, M., & Hedström, A. (2021). Data-driven asset management in urban water pipe networks: A proposed conceptual framework. Journal of Hydroinformatics, 23(5), 1014–1029. doi:10.2166/hydro.2021.068
- Ong, G. P., Noureldin, S., & Sinha, K. C. (2010). Automated pavement condition data collection quality control, quality assurance and reliability. Report FHWA/IN/JTRP-2009/17. West Lafayette, IN: Purdue University.
- Osman, H. (2016). Coordination of urban infrastructure reconstruction projects. Structure and Infrastructure Engineering, 12(1), 108–121. doi:10.1080/15732479.2014.995677
- Ouyang, M. (2014). Review on modeling and simulation of interdependent critical infrastructure systems. Reliability Engineering & System Safety, 121, 43–60. doi:10.1016/j.ress.2013.06.040
- Ouyang, M., Hong, L., Mao, Z. J., Yu, M. H., & Qi, F. (2009). A methodological approach to analyze vulnerability of interdependent infrastructures. Simulation Modelling Practice and Theory, 17(5), 817–828. doi:10.1016/j.simpat.2009.02.001
- Papageorgiou, G. (2019). Appraisal of road pavement evaluation methods. Journal of Engineering Science and Technology Review, 12(6), 158–166. doi:10.25103/jestr.126.20
- Parlikad, K., & Jafari, M. (2016). Challenges in infrastructure asset management. IFAC-Papers Online, 49(28), 185–190. doi:10.1016/j.ifacol.2016.11.032
- Peraka, N. S. P., & Biligiri, K. P. (2020). Pavement asset management systems and technologies: A review. Automation in Construction, 119, 103336. doi:10.1016/j.autcon.2020.103336
- Pierce, L. M., McGovern, G., & Zimmerman, K. A. (2013). Practical guide for quality management of pavement condition data collection. Practical guide for quality management of pavement condition data collection. https://www.fhwa.dot.gov/pavement/management/qm/data_qm_guide.pdf.
- Rajani, B., & Kleiner, Y. (2001). Comprehensive review of structural deterioration of water mains: Physically based models. Urban Water, 3(3), 151–164. doi:10.1016/S1462-0758(01)00032-2
- Ramos, C., Muñuzuri, J., Aparicio-Ruiz, P., & Onieva, L. (2021). A decision support system to design water supply and sewer pipes replacement intervention programs. Reliability Engineering & System Safety, 216, 107967. doi:10.1016/j.ress.2021.107967
- Rinaldi, S. M., Peerenboom, J. P., & Kelly, T. K. (2001). Identifying, understanding, and analyzing critical infrastructure interdependencies. IEEE Control Systems Magazine, 21(6), 11–25. doi:10.1109/37.969131
- Rogers, C. D. F., Hao, T., Costello, S. B., Burrow, M. P. N., Metje, N., Chapman, D. N., … Saul, A. J. (2012). Condition assessment of the surface and buried infrastructure - A proposal for integration. Tunnelling and Underground Space Technology, 28(1), 202–211. doi:10.1016/j.tust.2011.10.012
- Rokstad, M. M., & Ugarelli, R. M. (2015). Evaluating the role of deterioration models for condition assessment of sewers. Journal of Hydroinformatics, 17(5), 789–804. doi:10.2166/hydro.2015.122
- Rokstad, M. M., & Ugarelli, R. M. (2016). Improving the benefits of sewer condition deterioration modelling through information content analysis. Water Science and Technology: A Journal of the International Association on Water Pollution Research, 74(10), 2270–2279. 10.2166/wst.2016.419.
- Rose, S., Mathew, B. S., Isaac, K. P., Abhaya, A. S., Rose, S., Mathew, B. S., … Abhaya, A. S. (2018). Risk based probabilistic pavement deterioration prediction models for low volume roads. International Journal of Pavement Engineering, 19(1), 88–97. doi:10.1080/10298436.2016.1162308
- Saad, D. A., & Hegazy, T. (2017). Economic optimization for the rehabilitation of co-located mixed assets. Canadian Journal of Civil Engineering, 44(10), 820–828. doi:10.1139/cjce-2016-0509
- Saha, P., & Ksaibati, K. (2016). A risk-based optimisation methodology for pavement management system of county roads. International Journal of Pavement Engineering, 17(10), 913–923. doi:10.1080/10298436.2015.1065992
- Salman, B., & Salem, O. (2012). Risk assessment of wastewater collection lines using failure models and criticality ratings. Journal of Pipeline Systems Engineering and Practice, 3(3), 68–76. doi:10.1061/(ASCE)PS.1949-1204.0000100
- Shah, Y. U., Jain, S. S., Tiwari, D., & Jain, M. K. (2013). Development of overall pavement condition index for urban road network. Procedia - Social and Behavioral Sciences, 104, 332–341. doi:10.1016/j.sbspro.2013.11.126
- Shahata, K., & Zayed, T. (2010). Integrated decision-support framework for municipal infrastructure asset. Pipelines 2010: Climbing New Peaks to Infrastructure Reliability - Renew, Rehab, and Reinvest - Proceedings of the Pipelines 2010 Conference (Vol. 386, pp. 1492–1502). January 2018. doi:10.1061/41138(386)145
- Shahata, K., & Zayed, T. (2016). Integrated risk-assessment framework for municipal infrastructure. Journal of Construction Engineering and Management, 142(1), 04015052. doi:10.1061/(ASCE)CO.1943-7862.0001028
- Silva, B. N., Khan, M., & Han, K. (2018). Towards sustainable smart cities: A review of trends, architectures, components, and open challenges in smart cities. Sustainable Cities and Society, 38, 697–713. doi:10.1016/j.scs.2018.01.053
- Torbaghan, E. M., Curioni, G., Hayati, F., Royal, A. C., Chapman, D. N., Atkins, P. R., & Rogers, C. D. (2020). Investigating the relationship between trenching practice and road deterioration. Infrastructure Asset Management, 7(4), 282–296. doi:10.1680/jinam.19.00052
- Tscheikner-Gratl, F. (2016). (Integrated approach for multi-utility rehabilitation planning of urban water infrastructure: Focus on small and medium sized municipalities). (Doctoral Dissertation, University of Innsbruck). https://www.uibk.ac.at/umwelttechnik/teaching/phd/dissertation_ftg_ohne_papers.pdf.
- Tscheikner-Gratl, F., Caradot, N., Cherqui, F., Leitão, J. P., Ahmadi, M., Langeveld, J. G., … Clemens, F. (2019). Sewer asset management–state of the art and research needs. Urban Water Journal, 16(9), 662–675. doi:10.1080/1573062X.2020.1713382
- Tscheikner-Gratl, F., Egger, P., Rauch, W., & Kleidorfer, M. (2017). Comparison of multi-criteria decision support methods for integrated rehabilitation prioritization. Water, 9(2), 68. doi:10.3390/w9020068
- Tscheikner-Gratl, F., Hammerer, M., Rauch, W., Mikovits, C., & Kleidorfer, M. (2013). Integrated Rehabilitation management for different infrastructure sectors, gwf-Wasser-Abwasser, Special (1/2013), 50–56. https://www.gwf-wasser.de/produkte/2013-integrated-rehabilitation-management-for-different-infrastructure-sectors-1/.
- Tscheikner-Gratl, F., Sitzenfrei, R., Rauch, W., & Kleidorfer, M. (2016a). Enhancement of limited water supply network data for deterioration modelling and determination of rehabilitation rate. Structure and Infrastructure Engineering, 12(3), 366–380. doi:10.1080/15732479.2015.1017730
- Tscheikner-Gratl, F., Sitzenfrei, R., Rauch, W., & Kleidorfer, M. (2016b). Integrated rehabilitation planning of urban infrastructure systems using a street section priority model. Urban Water Journal, 13(1), 28–40. doi:10.1080/1573062X.2015.1057174
- Tscheikner-Gratl, F., Sitzenfrei, R., Stibernitz, C., Rauch, W., & Kleidorfer, M. (2015). Integrated rehabilitation management by prioritization of rehabilitation areas for small and medium sized municipalities. World Environmental and Water Resources Congress 2015, 2045–2057. doi:10.1061/9780784479162.201
- Ugarelli, R. (2008). Asset management of wastewater networks (Doctoral dissertation). Bologna, Italy: Alma Mater Studiorum - Università di Bologna. http://amsdottorato.unibo.it/878/.
- Urich, C., & Rauch, W. (2014). Exploring critical pathways for urban water management to identify robust strategies under deep uncertainties. Water Research, 66, 374–389. doi:10.1016/j.watres.2014.08.020.
- van den Boomen, M., Spaan, M. T. J., Shang, Y., & Wolfert, A. R. M. (2020). Infrastructure maintenance and replacement optimization under multiple uncertainties and managerial flexibility. Construction Management and Economics, 38(1), 91–107. doi:10.1080/01446193.2019.1674450
- Van Dijk, D., & Hendrix, E. M. T. (2016). Pipe replacement in a water supply network: Coordinated versus uncoordinated replacement and budget effects. Informatica, 27(2), 387–403. doi:10.15388/Informatica.2016.91
- van Riel, W., Langeveld, J., Herder, P., & Clemens, F. (2017). The influence of information quality on decision-making for networked infrastructure management. Structure and Infrastructure Engineering, 13(6), 696–708. doi:10.1080/15732479.2016.1187633
- van Riel, W., Post, J., Langeveld, J., Herder, P., & Clemens, F. (2017). A gaming approach to networked infrastructure management. Structure and Infrastructure Engineering, 13(7), 855–868. doi:10.1080/15732479.2016.1212902
- Walker, W. E., Harremoes, P., Rotmans, J., Van Der Sluijs, J. P., Van Asselt, M. B. A., Janssen, P., & Krayer Von Krauss, M. P. (2003). Defining uncertainty. Integrated Assessment, 4(1), 5–17. https://www.narcis.nl/publication/RecordID/oai:tudelft.nl:uuid:fdc0105c-e601-402a-8f16-ca97e9963592. doi:10.1076/iaij.4.1.5.16466
- Wei, L., Du, H., Mahesar, Q. a., Al Ammari, K., Magee, D. R., Clarke, B., … Cohn, A. G. (2020). A decision support system for urban infrastructure inter-asset management employing domain ontologies and qualitative uncertainty-based reasoning. Expert Systems with Applications, 158, 113461. doi:10.1016/j.eswa.2020.113461
- Xu, Q., Qiang, Z., Chen, Q., Liu, K., & Cao, N. (2018). A superposed model for the pipe failure assessment of water distribution networks and uncertainty analysis: A case study. Water Resources Management, 32(5), 1713–1723. doi:10.1007/s11269-017-1899-8
- Yang, Y., Ng, S. T., Xu, F. J., & Skitmore, M. (2018). Towards sustainable and resilient high density cities through better integration of infrastructure networks. Sustainable Cities and Society, 42, 407–422. doi:10.1016/j.scs.2018.07.013
- Yilema, M. G., & Gianoli, A. (2018). Infrastructure governance: Causes for the poor sectoral coordination among infrastructure sectors of Addis Ababa. Cities, 83, 165–172. doi:10.1016/j.cities.2018.06.019
- Young, O. R., Agrawal, A., King, L. A., & Sand, P. H. (1999). Institutional dimensions of global environmental change. Public Administration and Public Policy, 2(1999), 100.
- Yuan, X. (2017). Principles and guidelines of deterioration modelling for water and waste water assets. Infrastructure Asset Management, 4(1), 19–35. doi:10.1680/jinam.16.00017
- Zhang, J., & Yuan, X. (2021). Stochastic modelling of maintenance flexibility in Value for Money assessment of PPP road projects stochastic modelling of maintenance flexibility in value for money. Construction Management and Economics, 39(2), 173–191. doi:10.1080/01446193.2020.1855666
- Zuluaga, S., & Sánchez-silva, M. (2020). The value of flexibility and sequential decision-making in maintenance strategies of infrastructure systems. Structural Safety, 84, 101916. doi:10.1016/j.strusafe.2019.101916