Abstract
Over the past decades, different drought indices have been suggested in the literature. This study tackles the problem of how to characterize droughts by defining a framework and proposing a generalized family of drought indices that is flexible regarding the use of different hydrological fluxes in the water balance. The sensitivity of various indices and its skill to represent drought conditions is evaluated using a regional model simulation for Europe spanning the last two millennia as test bed. The framework combines an exponentially damped memory with a normalization method based on quantile mapping. Both approaches are more robust and physically meaningful compared to the existing methods used to define drought indices. Still, the framework is flexible with respect to hydrological fluxes used for the water balance, enabling users to adapt the index formulation to the data availability of different locations. Based on it, indices using different hydrological fluxes in the water balance are compared with each other showing that a drought index considering only precipitation in the water balance is sufficient for western to central Europe. In the Mediterranean, temperature effects via evapotranspiration rather than potential evapotranspiration, need to be considered to produce meaningful indices representative of water deficit. In addition, our results indicate that in north-eastern Europe and Scandinavia, snow and run-off effects need to be considered simultaneously in the index definition to obtain accurate results.
1. Introduction
Droughts are natural hazards with devastating effects on nature and human activities, as the latest drought in California has demonstrated (Arndt et al., Citation2015). Indeed, droughts have played an important role in the rise or downfall of civilizations in the past (Haug et al., Citation2003; Cook et al., Citation2015), as availability of water is essential for human development. In the light of current and future climate change, the increasing population demands an enhanced intensity in the use of agricultural resources, which then leads to a higher demand in water and makes the socio-economic system in general more vulnerable to droughts (Mishra and Singh, Citation2010). Consequently, a better understanding of the processes generating and driving droughts, as well as their predictability, are of high relevance and have attracted a growing attendance in the scientific community (Mariotti et al., Citation2013; Heim, Citation2002; Keyantash and Dracup, Citation2002; Cook et al., Citation2015).
Despite the importance of droughts for mankind, there are several caveats that limit our current understanding of this phenomenon. Droughts are rare phenomena that require long observational data records to identify and characterize them. Unfortunately, this information is not available for many regions of interest. There is no objective quantitative and universal definition of what a drought is, as a multitude of factors may play a role in it, challenging the characterization in a quantitative way (Wilhite, Citation2000; Keyantash and Dracup, Citation2002). Moreover, droughts are classified in four different types, namely meteorological, agricultural, hydrological and socio-economic (e.g. Svoboda et al., Citation2002; Andreadis and Lettenmaier, Citation2006). This study focuses on meteorological droughts defined as a reduction of available water during an extended time period.
The latter caveat is illustrated by the fact that several drought index definitions exist in literature: one of the first attempts to characterize droughts is the so-called Palmer Drought Severity Index [PDSI; ][]Palmer1965. Palmer’s definition of a drought is ‘an interval of time, generally of the order of months or years in duration, during which the actual moisture supply at a given place rather consistently falls short of the climatically expected or climatically appropriate moisture supply’ (Palmer, Citation1965). With this definition, it is possible to quantitatively represent drought severity and to make different locations comparable. Still, this index uses a number of subjective criteria, e.g. a fixed timescale, and empirical relations for specific locations, which may reduce the universality and applicability of this index. In this context, Alley (Citation1984) argued that having one inherent timescale renders the PDSI not suitable for different kinds of droughts. Additionally, the index does not consider snow and the normalization procedure used in the PDSI produces different distributions for different locations. Hence, spatial comparability is not straightforward (Mishra and Singh, Citation2010). Some of the caveats with the PDSI led to the self-calibrated Palmer Drought Severity Index (Wells et al., Citation2004), where some of the subjectively determined empirical constants are replaced with values calibrated with the local climatic conditions and ensuring a spatial comparability. Further, Mo and Chelliah (Citation2006) suggested to use the Noah Land Surface Model (Ek, Citation2003; Chen and Dudhia, Citation2001) instead of Palmer’s simple soil model. Still, these improvements do not solve the arbitrary nature of the calculation and normalization procedure of the index.
Another index commonly used is the standardized precipitation index [SPI; ][]Mckee1993,Edwards1997. This rather simple approach is only based on monthly precipitation. As for the PDSI, the SPI depends on various assumptions, in particular the fit of the precipitation data to a given probability density distribution (PDF) used within the procedure (Lloyd-Hughes and Saunders, Citation2002; Mishra and Singh, Citation2010; Wu et al., Citation2007). Recently, Farahmand and AghaKouchak (Citation2015) suggested non-parametric normalization procedure for the SPI, which avoids this conceptual drawback. Another caveat of the SPI is that it is based only on precipitation and thus ignores other processes like evaporation, temperature, run-off or soil–water content, which are known to affect the water balance but are difficult to measure (Vicente-Serrano et al., Citation2010). Therefore, Vicente-Serrano et al. (Citation2010) developed the Standardized Precipitation Evapotranspiration Index (SPEI) taking temperature effects in the SPI into account by introducing potential evapotranspiration (Thornthwaite, Citation1948; Penman, Citation1948). Still, the SPEI suffers from the same conceptual drawbacks as the SPI, as the normalization procedure requires fitting a matching model to the PDF. Still, Ma et al. (Citation2014) argued that the influence of temperature is strongly overestimated in the SPEI because potential evapotranspiration is generally much higher than evapotranspiration, especially in dry areas where soil water is limited (Bouchet, Citation1963; Hobbins et al., Citation2004). This is in contrast to Beguería et al. (Citation2014) who found the choice of potential evapotranspiration to be sufficient, as it represents the water demand of the atmosphere, while the evapotranspiration is influenced by the availability of precipitation.
One possibility to tackle the variety of drought indices, their shortcomings and their underlying ad hoc assumptions is to assess a number of indices and combine them in a monitoring system as, e.g., suggested by the Drought Monitor for the United States (Svoboda et al., Citation2002). Another possibility, which is the purpose of this study, is to integrate the various drought indices in a stepwise framework which helps to identify shortcomings. Based on this framework, we introduce a new drought index, which combines advantages of different existing indices in a new way, and which is flexible enough to be applied to different regions and climate conditions. The flexibility of the framework, and thus the new index formulation, also allows adjusting the hydrological fluxes included in the water balance. Thus another purpose of this study is to investigate the complexity of the water balance which is necessary to sufficiently describe drought conditions on the regional scales. Both, the evaluation of the framework as well as the issue of complexity of the water balance, are performed in an idealized test bed that consists of a regional climate model simulation for Europe for the last two millennia (Gómez-Navarro et al., Citation2013; Gómez-Navarro et al., Citation2015).
Recent advances in climate modelling make it possible to realistically simulate climate processes of the water balance at regional scales being able to reproduce the major precipitation regimes in Europe (Gómez-Navarro et al., Citation2013; Gómez-Navarro et al., Citation2015). An advantage of such simulations is that they guarantee physical consistency and thus provides an ideal test bed for assessing drought indices over long timescales. Note that the focus of the study is on the methods introduced rather than on the variability during the last two millennia, so the latter will be therefore not shown.
The structure of the study is as follows. Section 2 provides an overview of the model data used as test bed. Then, the framework of drought indices is presented discussing how existing drought indices fit in this framework (Section 3). Based on it, a new index is presented, which remains flexible in the selected fluxes included in the water balance (Section 3). In Section 4, the framework is applied to a regional climate model simulation for the last two millennia to test the newly introduced memory and normalization procedure and to demonstrate the differences and similarities of the drought indices which include different fluxes in the water balance (Section 5). Finally, the results are summarized and conclusions are presented in Section 6.
2. Regional climate simulation and analysis methods
To evaluate the framework for drought indices including the new index, a simulation of the last two millennia for Europe is considered as test bed. It is based on a model chain consisting of a regional climate model (RCM) driven by a coupled atmosphere – ocean general circulation model (AO-GCM). Both models use identical reconstructions of greenhouse gas concentrations and solar irradiance for the last two millennia as external forcing factors. Volcanic activity is not included as there were no reliable estimates available for first millennium when the simulations were performed.
The AO-GCM is the so-called ECHO-G model, which consists of the spectral atmospheric European Centre Hamburg model [ECHAM4; version 4]Roeckner1996 and the ocean model Hamburg Ocean Model in Primitive Equations [HOPE-G]Legutke1999. The atmospheric model has a horizontal resolution of T31 (approximately ) and 19
-pressure levels in the vertical. The ocean model has a horizontal resolution of
, which is refined to
in tropics. In the vertical, HOPE-G is discretized in 20 unevenly distributed layers. Additionally, the two components are coupled with a flux adjustment constant in time. A description of the model set of ECHO-G is given in Legutke and Voss (Citation1999), a control simulation for 1990 conditions is described in Raible et al. (Citation2001, (Citation2004) and Luksch et al. (Citation2005), and the set-up of the transient two millennia simulation is presented in Zorita et al. (Citation2004).
The RCM is a climate version of a mesoscale model for atmospheric research (MM5, version 5; Skamarock et al., Citation2008) coupled to the Noah land surface model (Chen and Dudhia, Citation2001). Horizontally, two two-way nested domains with spatial resolutions of 135 km and 45 km are employed, respectively. The RCM is driven by the AO-GCM at its outer boundary by blending over five grid points. Nudging of the large-scale circulation is not used. Further, 24 levels are used in the vertical, with the highest level at 100 hPa. This setting, in particular the land model, is selected because of its skill in dry areas compared to simpler soil models (Jerez et al., Citation2010). Topography, land use, vegetation and soil types are given as input, but remain fixed at the present-day value throughout the entire simulation, as there is no reliable reconstruction of these parameters for the last two millennia. For this study, only the data of the inner domain with a resolution of 45 km was used. A detailed analysis of this model set-up focusing on the added value of the RCM in comparison to the AO-GCM shows that the RCM simulation is superior in particular for precipitation (Gómez-Navarro et al., Citation2013) and resembles hydroclimatic field reconstructions (Gómez-Navarro et al., Citation2015).
The overview of the variety of drought indices in Section 1 showed that there is no reference drought index which makes it difficult to show the skill of new drought indices. To overcome this problem, the different indices are evaluated by the Pearson Correlation analysis between each pair of indices for each grid point using the years 1 to 1800 AD. The choice of this period, which excludes the anthropogenic forcing, guarantees that the correlation coefficients are not influenced by any trends in the period 1850-2000 AD – a prerequisite for meaningful correlation analysis.
3. Framework of new drought indices
Droughts can be characterized by indices, which incorporate the drought intensity, duration and severity at a given location and time. An appropriate index shall be applicable for different timescales and ideally is normalized to ensure comparability between different locations. As a number of meteorological and hydrological processes are involved in generating droughts, a universal definition of an index remains a challenge. Several indices are suggested in the literature (Mishra and Singh, Citation2010; Vicente-Serrano et al., Citation2010), briefly presented in Section 1. In the following, we introduce a framework for drought indices, in which most commonly used indices can be integrated. Finally, a new index is proposed, which overcomes some of the shortcomings of existing drought indices.
3.1. The framework
The framework of the design of a drought index considers four steps:
(i) | The user defines the hydrological fluxes (e.g. precipitation, run-off, evaporation, etc.) considered relevant for the water balance. Ideally, these variables have a monthly (or even sub-monthly) resolution and high horizontal resolution (either gridded or weather station network). Moreover, long-time series of these fluxes are required, as all approaches require some type of data fitting, which may become problematic for rare events such as droughts. | ||||
(ii) | The hydrological fluxes are combined in a water balance model, which defines the surplus or deficit of water | ||||
(iii) | The drought variable | ||||
(iv) | Finally, the drought variable D is normalized, leading to the drought index I. This procedure guarantees spatial comparability, as the same value of D may have a different meaning at different locations: |
The SPI relies only on the monthly precipitation . Thus, the water balance model is trivial and states that only precipitation is sufficient to establish drought conditions. Hence, Equation (Equation1
(1) ) becomes
The SPI takes the memory of the system into account by summing over the previous m months (where m is usually set between 3 and 48) so that Equation (Equation2(2) ) becomes
Note that each month included in the memory has the same weight. The fourth step of the framework implies the normalization of D. To obtain the SPI, the drought variable D is normalized at each location and for each month of the year separately. The latter is necessary to remove the annual cycle if the memory length m is not a multitude of 12. The normalization is done by mapping the PDF of D onto a Gaussian distribution. Thereby, a user-defined distribution function (typically the gamma distribution) is fitted to the histogram of D (McKee et al., Citation1993; Edwards and McKee, Citation1997).
A variant of this index was introduced as an attempt to include temperature effects into the SPI through evaporation effects (SPEI; Vicente-Serrano et al., Citation2010). This effect is mainly due to evapotranspiration (Appendix 1). However, evapotranspiration is a variable difficult to measure directly, so the SPEI uses the potential evapotranspiration, i.e. the maximum possible evapotranspiration if the soil contained an unlimited water reservoir. Although less informative than evapotranspiration, the advantage of potential evapotranspiration is that it can be easily estimated by the Thornthwaite method (Thornthwaite, Citation1948) or the Penman method ((Penman, Citation1948, Appendix A for further details)). Using the potential evapotranspiration PET in the water balance model by simply subtracting it from P-pagination leads to
where T is the temperature and the variables used to calculate PET depending on the selected approach (see Appendix 1). The memory is then introduced in the same way as for the SPI. Beguería et al. (Citation2014) showed that a three parameter log–logistic distribution provides meaningful results within the normalization step for most locations, as d can become negative when PET exceeds P.
A more elaborate method is the PDSI Palmer (Citation1965). In contrast to the relatively simple SPI and the SPEI, the PDSI takes a complex function of the water demand into account. Thus, the water balance model described by Equation (Equation1
(1) ) becomes
The water demand is obtained from the water balance of a soil model based on evapotranspiration ET, potential evapotranspiration PET, recharge R to the soil, potential recharge PR, run-off RO, potential run-off PRO, loss L from the soil and potential loss PL.
where ,
,
, and
are ratios and j is the month in the annual cycle and the bar above the symbols denotes the average over all time steps t of month j. The calculation is done for each month of the year separately in order to account for the annual cycle. Thus, to obtain the water demand
, a set of additional variables needs to be known on a monthly basis: PET, PR, PRO and PL. Note that in the approach of Palmer, step (iii) and (iv) of the framework are exchanged, i.e. first a normalization is applied, and then the memory effect is taken into account. The normalization is obtained by multiplying
with a climate characteristic
, which was originally estimated for nine different locations in the United States, and which renders this index not very universal. Finally, the memory is introduced using the recursive formula:
(4)
The duration factors 0.897 and were estimated by Palmer for two locations (central Iowa and western Kansas, US), to obtain a ‘nice? distribution with few extreme values and the maximum and mean value close to zero. This ad hoc selection questions the applicability of this index for other areas than those studied by Palmer.
3.2. A new standardized drought index
As outlined above, the existing approaches to characterize drought partly suffer from shortcomings – be it arbitrary selected functions fitted to the histogram of D as used for the SPI, the equally weighted memory effect of the SPI or the SPEI, or the arbitrary determined factors to normalize the PDSI. This calls for a new approach taking advantage of different existing index properties and being flexible to include various combinations of hydrological fluxes in the water balance. The latter remark is important especially in climate variability studies due to the scarcity of the data availability in the past. To guarantee flexibility, the new approach concentrates on the way memory is introduced and how the index is normalized, leaving the hydrological fluxes entering in the water balance model free to be selected by the user. This flexibility enables the users to adjust the drought index definition to the data availability and the requirements of the climatic features of the area of interest (see the recommendations for the European climate in Section 6).
The introduction of memory in the new approach is inspired by the fact that the SPI and SPEI consider each of the m months to have the same influence (or weight) in the drought condition at time t, while all other months have no influence at all. However, the water deficit of the current month is expected to have the strongest influence, with a continuously decreasing influence of previous months. Thus, we propose an approach inspired by the recursive procedure of the PDSI, that once developed, can be reformulated as:(5)
where is an arbitrary constant (not important due to the normalization in the last step) and l the number of previous time steps having an influence on a drought in the current time step. The recursive formula is interpreted as an average over all previous time steps, weighted with the exponential damping constant
. Hence, p is a characteristic timescale of the phenomenon drought, rather than a fitting constant (as in the PDSI formulation). Consequently, the recursive formula from the PDSI can be used to implement a more realistic memory. For instance, the p value of 0.897 suggested in the PDSI approach corresponds to a characteristic time (or e-folding time) of 6.6 time steps (in the case of PDSI, 6.6 months). Note, however, that we do not propose a given damping constant p, but we leave it open to be defined by the user depending on its particular necessities.
Finally, the normalization procedure of the new index is based on the approach of the SPI, i.e. mapping the D values on the Gaussian distribution. However, fitting a particular distribution to the histogram of D is avoided (which is in contrast to the SPI and SPEI approach). Instead, we propose using a non-parametric method, the so-called quantile mapping approach: calculating percentiles of all D values at one location from the histogram and mapping them to the corresponding percentile of the Gaussian distribution, which finally results in the index I. The mapping has to be performed for each month of the year separately to account for the annual cycle. Quantile mapping is regularly used in downscaling and bias correction of model output (Raible et al., Citation2012; Themessl et al., Citation2011; Gudmundsson et al., Citation2012; Rajczak et al., Citation2016) and is similar to non-parametric approaches used in drought index definitions (Farahmand and AghaKouchak, Citation2015).
Table 1. Mean Pearson correlation over all grid points between block memory and exponentially damped memory using different e-folding times for the SPI and only the e-folding time 6.6 months for PDSI.
4. Testing the new framework during the last two millennia over Europe
To illustrate the strengths and flexibility of this framework, the newly proposed memory and normalization procedures are compared to the methods used in the definitions of the commonly used indices. As discussed above, 1800 years of the regional simulation over Europe, explicitly excluding the period dominated by anthropogenic forcing, are used as test bed of the analysis.
4.1. Impact of memory
The new index uses an exponential function with a damping coefficient p (inspired by the recursive formula of the PDSI) and is compared to the block mean approach of the SPI, i.e. a running mean of the preceding months and the current month. For the sake of focusing on the role of the memory, we use the index which is based on the water balance model of the SPI and explore several damping coefficients around the one which is used in the classical PDSI definition, i.e.
. In following, we show results for
, which corresponds to e-folding times of 4.6, 6.6 and 11.2 months, respectively. Then, the results are compared with the ones obtained from the block mean approach for
and 48. The calculation is repeated for each grid point over Europe and for the 1800 years of the model simulation. Pearson correlation coefficients between all combinations are estimated at each grid point to illustrate agreement or disagreement between different indices (Section 2).
The highest correlations of above 0.9 are found when thee-folding time correspond to the length of the block mean. The results show that an e-folding time of 4.6/6.6/11.2 months correspond to approximately 9/12/18-month window in the block mean (Table ). Low correlations of around 0.4 to 0.6 are found when the e-folding time and the block mean deviate strongly, e.g. 1-month or 48-month block mean compared to the selected e-folding time. The correspondence is expected, as drought indices depend on the length of the period on which they are based (Steinemann and Cavalcanti, Citation2006), so other indices show the same behaviour (PDSI only shown for in Table , SPEI not shown but is similar to PDSI).
As an additional test, drought indices are used to classify the severity of simulated droughts. To illustrate the effect of the memory implementation on the severity of droughts, we evaluate how often the indices fall below a certain threshold simultaneously in the same location. This is done for the pair with the highest correlation, so an e-folding time 6.6 months and 12-month block mean. For severe droughts with an index below –2, both agree in of the time. For extreme droughts with an index below -3, the agreement between the two memory implementation drops to
. These results indicate that extremes in drought indices are highly dependent on the memory method selected.
Table 2. Median, 90th and 95th percentiles of the distribution of maximum D-values of the Kolmogorov–Smirnov test for all locations and three block memories. The percentiles are presented for the SPI and the PDSI.
Figure 1. Maximum distance between the empirical cumulative distribution of drought indices and the cumulative gamma distribution for January: (a), (b) SPI, (c), (d) PDSI. In panels (a), (c) a block memory of 1 month is used, in (b), (d) a block memory of 6 months. Stippling denotes the 5% significance level.
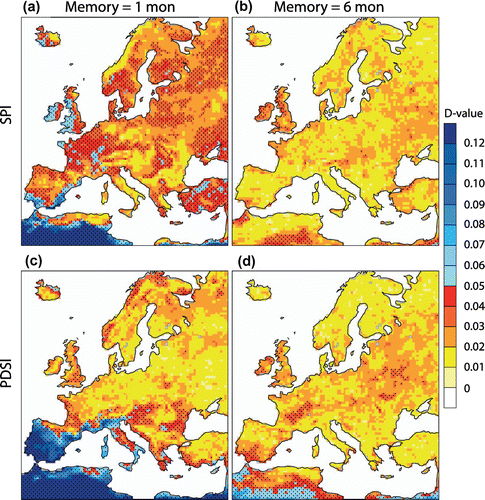
In summary, the memory implementation and the length of the memory has an effect on the drought index and the classification of severity. In particular, it is important to ensure that the length of memory is consistent when comparing results from different drought indices (Steinemann and Cavalcanti, Citation2006). In this sense, the PDSI is not flexible since it implicitly fixes a given memory length. Moreover, the implementation of exponential damping memory is justified, as it is physically more realistic, having a continuously decreasing influence of a given month over time until it becomes negligible, instead of having a constant influence that instantaneously drops to zero. Thus, the memory implementation proposed harmonizes the advantages from various existing indices.
4.2. Impact of the normalization procedure
Besides the implementation of the memory, a non-parametric procedure for normalizing the drought index is introduced, i.e. the so-called quantile mapping. This approach is compared to the standard SPI procedure, based on fitting a gamma distribution. For simplicity, we concentrate on the drought indices SPI and PDSI and three memory lengths (1, 6 and 12 months), the latter with the block mean method. Quantile mapping and fitting of a gamma distribution is applied to each location and month of the year separately. The quality of the fit is assessed with the Kolmogorov–Smirnov test using the D-value, i.e. the maximum distance between the empirical cumulative distribution function of the data (representing the quantile mapping) and the cumulative gamma distribution.
The D-values show that there are strong deviations from the empirical distribution and the gamma distribution in some regions, here shown for January (Fig. ). A similar range of D-values is found for other months, like July (not shown). These deviations exceed the error of the quantile mapping, which has maximal error of 0.024 for a record length of 1800 years. High values are found around the Mediterranean using a block memory of 1 month using the SPI (Fig. (a)). This is independent from the number of fluxes considered in the water balance used in the index definition as the comparison to the PDSI shows (Fig. (c)). The reason is that a substantial number of months have zero precipitation in these regions, like North Africa, which hampers a reasonable fit to a gamma distribution. For the SPI, the D-values decrease, i.e. the fit improves, when applying a block memory of 6 months (Fig. (b)) as 6-month periods without precipitation are rarely found. Also other regions like France and the United Kingdom show higher D-values when using the SPI and a block memory of 1 month, suggesting that fitting a gamma distribution is problematic in this case. The drought index PDSI shows partly a different behaviour, e.g. a block memory of 1 month leads to low D-values over France, which increase when using block memory of 6 months (Fig. (b), (d)). A similar behaviour is also found in eastern Europe (Fig. (b), (d)).
To further assess the deviations between the empirical and gamma distribution, we show the median 90th and 95th percentile of the distribution of all D-values for block memories of 1, 6 and 12 months (Table ). In agreement to Fig. , the median D-value distribution is reduced with increasing memory regardless of the water balance used in the index definition. Still, the tails of the D-value distribution (as illustrated by the 90th and 95th percentiles) show that in particular for the PDSI high D-values (of more than 0.17 in the case of a memory of 1 month) occur in 5% of the locations (Table ). This illustrates that the water balance used in an index definition needs to be tested for each location a priori in order to assess whether a gamma distribution can be reasonably fitted.
Table 3. Error in percentile of the estimating 90th percentile of a drought index using the quantile mapping based on N values (time steps). Note that the error (95% confidence interval) is calculated based on, in which percentile a certain values falls.
As stated above, there is also uncertainty associated with the quantile mapping, i.e. the uncertainty, in which percentile a certain values falls. This uncertainty increases with decreasing sample size. To illustrate this, we show the error of estimating an extreme index (90th percentile; Table ). The result suggests that the quantile mapping is superior to the method fitting a gamma distribution at least up to 250 years and delivers compatible results also for shorter sample sizes like 100 years (comparing the values of Table to ones of Table ).
Results above indicate that the ability of a gamma distribution to fit the empirical values critically depends on the region, the memory length applied, and the hydrological fluxes incorporated in the water balance. This implies that the applicability of a gamma distribution fit has to be critically tested a priori, as it depends on the location and hydrological fluxes entering in the water balance, which renders the introduction of a drought index using fits to a gamma distribution controversial, and favours the application of the quantile mapping in the normalization procedure. The latter is independent on assumptions about the distribution of the index and shows superior or similar results also for shorter time series (up to 100 years). These findings agree with Farahmand and AghaKouchak (Citation2015) who used also paramedic approaches in their drought indices.
5. Impact of the different hydrological fluxes included in the water balance
Based on the experience of the previous sections, we now assess the impact of different hydrological fluxes included in the water balance within the framework of drought indices. As no absolute reference exists, we select an approach consisting of the comparison of different indices with each other through correlation analysis. Later, deviations from high correlations are addressed under the light of the different physical mechanisms introduced by the variable complexity of such water balances (Section 2). The assumption is that the more components of the water balance are incorporated in a water balance model, the more realistic and representative of real situations a drought index is. However, this is at the expense of requiring more input variables, which are often not available on long timescales or are sparsely available in space, in particular when considering observations. Thus, in some cases it can be desirable to use a simple water balance model, such as the one of the SPI, that may explain the major part of the phenomenon while requiring only few variables easily recorded. The simulation enables us to analyse and identify, as a function of the season and the region of interest within Europe, which drought indices based on a simple water balance model are sufficient, compared to situations where more complex water balance models become necessary.
Before we start with the analysis, we recall and extend the water balance models introduced in Section 3. Additionally, different parametrizations for evapotranspiration and potential evapotranspiration are tested (Appendix 1).
The simplest water balance model only considers precipitation denoted in the following as SPI index. An increase in complexity is obtained when effects of evapotranspiration or potential evapotranspiration are additionally considered. Two parametrizations of potential evapotranspiration are used (Appendix 1): the Penman–Monteith method (Penman, Citation1948), and Thornthwaite method (Thornthwaite, Citation1948). As a third approach, evapotranspiration is deduced from the latent heat transfer (Appendix 1). The corresponding drought indices are called Standardized Precipitation Penman Evapotranspiration Index (SPPEI), the second Standardized Precipitation Thornthwaite Evapotranspiration Index (SPTEI) and the third Standardized Precipitation Latent Heat Evapotranspiration Index (SPLEI), respectively. Then, the water balance model is extended to include snow effects (named SPPEIs), which acts as a water storage. The water balance model becomes
where is the water equivalent of snow and PET the potential evapotranspiration after Penman (Citation1948) or Thornthwaite (Citation1948). Finally, the most complex water balance model additionally includes also the run-off effects (similar to the PDSI):
where is taken from the PDSI definition. This index is referred hereafter as Normalized Snow PDSI (PDSIs).
To concentrate on the effect of the number of fluxes included in the water balance (complexity of the water balance model), the memory is set to an e-folding time of 4.6 months () and quantile mapping is used as normalizing procedure in all tests hereinafter. Further, all correlations are estimated for each month of the year separately and then averaged to seasonal means (December, January, February, DJF; March, April, May, MAM; June, July, August, JJA; September, October, November, SON).
Figure 2. Pearson correlation pattern between SPI and (a) the SPPEI, (b) the SPTEI and (c) the SPLEI for each season separately. Note that all correlations are significant at the 1% level.
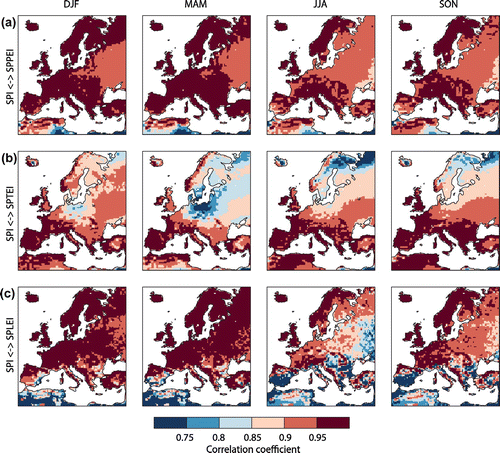
In a first step, the simplest drought index, SPI, is compared to SPEI in order to study the effect of evapotranspiration parameterized by three different methods. The correlation pattern between these drought indices shows that the agreement between the SPI and the SPPEI is high, with correlations above 0.9 in most of the regions and all seasons except North Africa (Fig. (a)). The lower correlation in North Africa can be attributed to an artefact in the potential evapotranspiration, which is much larger than the actual evapotranspiration due to long sunshine hours and low air humidity, thus having a stronger influence on the final index. The strong agreement between SPI and SPEI is not found when using the Thornthwaite method to estimate potential evapotranspiration (SPTEI; Fig. (b)). The reason is that the Thornthwaite method depends only on average temperature and the maximum possible radiation depending on the time of the year. However, it does not include other effects such as actual incoming radiation and cloud cover. Using evapotranspiration estimated from the latent heat transfer, we find strongest deviations in summer around the Mediterranean and north to the Black Sea (SPLEI; Fig. (b)). The correlation pattern is also not similar to the correlation of SPI and SPPEI, and thus there seems to be an important discrepancy between the indices, depending on if potential evapotranspiration or evapotranspiration is used.
To gain additional insight on why indices based on different parametrizations of evapotranspiration or potential evapotranspiration are found, the seasonal means of these variables are shown in Fig. for winter and summer. The comparison of the Penman-based and the Thornthwaite-based potential evapotranspiration shows that the latter overestimates the potential evapotranspiration in summer in high latitudes due to the long daytime duration (Fig. (a), (b)). The Penman method reduces potential evapotranspiration due to cloud cover, and thus adds more regional details to potential evapotranspiration. Comparing the evapotranspiration, as estimated by latent heat release, (Fig. (c)) with potential evapotranspiration (Fig. (b)) shows higher values for the latter, which is expected as potential evapotranspiration is defined as the maximum of evapotranspiration. Still, the high values in summer around the Mediterranean seem unrealistic. Beguería et al. (Citation2014) argued to compare the water supply to the water demand stating that the subsequent normalization of the drought index can compensate such overestimation. However, the latter seems to be not justified, as the index is dominated by potential evapotranspiration in the dry areas of the Mediterranean and precipitation plays a minor role.
Figure 3. Potential evapotranspiration for winter (DJF) and summer (JJA) using (a) the method of Thornthwaite, (b) the method of Penman and (c) the evapotranspiration for both seasons, which is approximated by the latent heat flux.
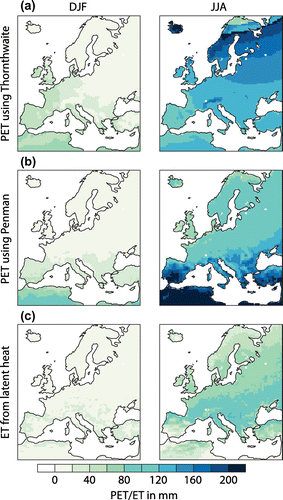
To account for this, the potential evapotranspiration can be renormalized to have the same time mean as evapotranspiration. Comparing the renormalized potential evapotranspiration based on the Penman method with evapotranspiration, estimated by latent heat release, (Section 2) shows positive and negative correlations (Fig. ). Positive correlations indicate areas where the evapotranspiration is not limited by water supply and, thus, follows the potential evapotranspiration as found mainly in autumn and winter for most of Europe. In summer, only Great Britain, France and parts of Scandinavia show positive correlations being the only regions where there is enough water available on most of the days. Negative correlations are caused by evapotranspiration not being limited by potential evapotranspiration, but by water supply. When water supply is low, evapotranspiration will be low too, but potential evapotranspiration increases simultaneously because of reduced cloud cover, eventually inducing these negative correlations. This is the case for the surrounding of the Mediterranean and in summer also for eastern Europe. The different temporal behaviour of the potential evapotranspiration and evapotranspiration, resembling findings from Bouchet (Citation1963); Hobbins et al. (Citation2004), also explains the differences in the correlation between the different drought indices in Fig. . Hence, we argue that potential evapotranspiration shall not be considered as a reasonable parametrizations in dry regions, because potential evapotranspiration is never reached in those regions and it is anti-correlated to actual evapotranspiration.
Figure 4. Pearson correlation pattern between the potential evapotranspiration based on Penman and the evapotranspiration approximated by the latent heat flux: (a) DJF and (b) JJA. Note that the potential evapotranspiration based on Penman is renormalized (see details in the text). Stippling denotes the 1% significance level.
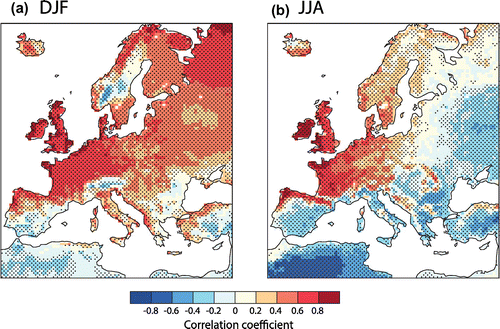
Figure 5. Pearson correlation pattern between drought indices SPPEI and the SPPEIs, the latter includes snow effects. Note that all correlations are significant at the 1% level.
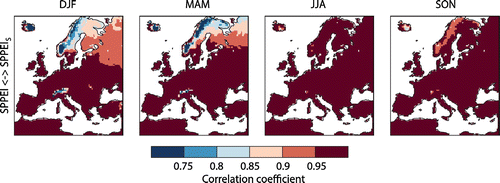
Figure 6. As Fig. , but showing the correlation between SPPEIs including snow effects and an index which additionally includes Run-off in the water balance (PDSIs). Note that all correlations are significant at the 1% level.
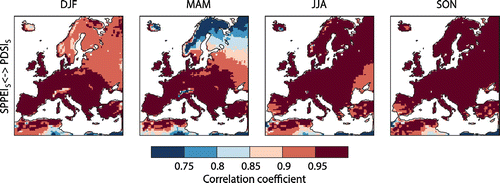
Figure 7. Pearson correlation pattern between PDSIs and soil moisture. Note that the soil moisture is averaged over 3 months, which shows the highest correlation to PDSIs. Stippling denotes the 1% significance level.
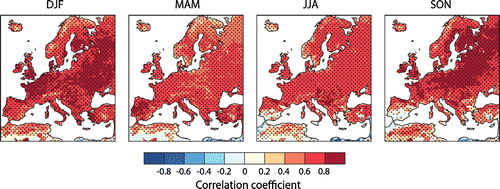
To assess the impact of snow effects on drought indices, the correlation between SPPEI and the same drought index including snow effects is shown in Fig. . As expected, the effects are limited to winter, when snow accumulates, and in the melting season spring. The influence of snow, highlighted by lower correlations, is mainly found in mountain areas like the Alps, the Carpathian Mountains, in Scandinavia, and in Iceland. Additionally, there is widespread reduced correlation in north-eastern Europe during winter and spring. This demonstrates how the influence of snow accumulation needs to be considered in these regions, which is in agreement to earlier findings (e.g. van der Schrier et al., Citation2007).
Including run-off effects seems to play a minor role as the correlation between the SPPEIs with snow effects and the PDSIs shows (Fig. ). An exception is again north-eastern Europe and Scandinavia during spring, where snow accumulation and subsequent melting leads to a substantial contribution to run-off. For north-eastern Europe and Scandinavia, the correlation is high in winter as both indices include snow accumulation, but lower in spring because the SPPEIs does not include run-off. The reduced correlation in North Africa in all seasons is due to the renormalized potential evapotranspiration in the PDSIs. Thus, the comparisons of snow and run-off effects suggest that they need to be considered simultaneously in certain areas in order to improve the drought index, in particular during winter and spring.
Another important variable used in drought definitions is the soil moisture (e.g. Mishra and Singh, Citation2010). To illustrate the relation between the (using a e-folding time of 4.6 months as above and evapotranspiration in the water balance model) and soil moisture content, we estimate the Pearson correlation at each grid point and for each season separately. Soil moisture content is a complex process coupled to the atmosphere that is consistently simulated by the model and naturally introduces memory whose length is dependent on the depth considered, as well as the physical properties of the soil and climate feedbacks in each location. However, as shown in Section 4.1, matching memory lengths is important to obtain meaningful correlations. Therefore, we tested various average lengths between 1 and 12 months. The tests show that 3-month averages of soil moisture lead to patterns with overall highest correlation between the chosen e–folding time for PDSIs and soil moisture, integrated over all level of the land model. Focusing on these correlation patterns, Fig. shows good agreement in most parts of the simulated region and in all seasons. The skill is quite uniform, further indicating that PDSIs is able to capture reasonably well actual soil moisture content, regardless of regional features. Still, lower correlations are found in spring and summer, as well as in mountain regions and known dry regions like North Africa and southern Spain. Some of the differences may be due to differences in how soil moisture is integrating water fluxes in the land model used (Chen and Dudhia, Citation2001). In particular, over dry regions, precipitation is restricted to one season but may have a stronger influence throughout the year in the PDSIs index due to the memory used than soil moisture and its memory. Also the processes, which define the memory of the soil moisture, can change with region, e.g. in regions with snow cover. This is another source for deviations as the memory in the PDSIs definition is fixed.
6. Discussion and conclusions
In this study, a flexible framework to define drought indices is presented and evaluated using a two millennia-long regional model simulation for Europe. It consists of four steps: (i) selection of the relevant hydrological fluxes used in the water balance, which depends on the data availability and requirements of the area of interest, (ii) combination of these fluxes in a water balance model, (iii) implementation of a memory using a physically meaningful exponential damping and (iv) normalization using quantile mapping.
The comparison to former definitions of drought indices (e.g. Palmer, Citation1965; McKee et al., Citation1993; Edwards and McKee, Citation1997; Vicente-Serrano et al., Citation2010) shows that the exponentially damped memory procedure shall be compared with the block mean memory approach (McKee et al., Citation1993) on similar timescales, resembling findings of e.g. Steinemann and Cavalcanti (Citation2006). Further, it can be argued that the exponentially damped memory procedure, inspired by the PDSI, is physically more meaningful than the block mean method and in contrast to the PDSI it is flexible with respect to the time window (i.e. users can define the e-folding time, whereas PDSI has a fixed e-folding time). The quantile mapping used in the normalization step of the framework additionally leads to further improvements, as it omits fitting to a predefined distribution functions. The latter is shown to be deficient in some regions, in particular in arid regions where the index values can be hardly fit to a gamma distribution and, thus, such fitting shall be carefully evaluated at each location. The quantile mapping is similar to the non-parametric approach used by Farahmand and AghaKouchak (Citation2015). Overall, the framework combines and extends existing approaches for the memory and normalization step in a new way.
A prominent feature of the framework is its flexibility regarding the hydrological fluxes used in the water balance. This is important as this step within the framework depends on the availability of data, i.e. if only precipitation data is available the water balance can only be formulated in a very simplistic way.
The framework is tested using different water balance models (differing in the number of hydrological fluxes included) to define various drought indices in variable complexity. The comparison of these different drought indices provides insight about regions where indices with simpler water balance models (i.e. reduced number of hydrological fluxes included) are sufficient to characterize a drought. The sketch of Fig. summarized this for Europe, showing that the simplest index, the SPI, shall be restricted to western Europe, whereas for southern Mediterranean and eastern Europe, the inclusion of temperature effects utilizing evapotranspiration becomes mandatory. Note that the areas in Fig. are based on our results, which rely on a single model simulation with known shortcomings, like the fixed land use, which might affect the extent of the regions where indices with simple water balances are actually suitable. Therefore, this figure shall be regarded as a qualitative sketch to be refined in future studies. The analysis further shows that the results are sensitive to the parametrizations of potential evapotranspiration. Our findings resemble observational evidence of the different behaviour of evapotranspiration and potential evapotranspiration (Bouchet, Citation1963; Hobbins et al., Citation2004). Thus, we suggest to avoid its use in favour of actual evapotranspiration whenever possible by data accessibility. For northern Europe, both, snow and run-off effects, need to be included for accurate representation of drought conditions.
The comparison with soil moisture shows that in most parts of Europe, the index with the most complex water balance model () agrees with the soil moisture, which is also used in drought definitions (e.g. Mishra and Singh, Citation2010). Still, in areas of highly reduced precipitation like North Africa and southern Spain the indices deviate, potentially due to the different handling of the memory in the land surface model which determines soil moisture compared to the user-defined, but fixed memory used in the framework presented in this study.
In summary, the analysis suggests that drought indices based on simple water balance models are only appropriate in specific regions. Somehow expected the complexity of the water balance models reflects the climatic conditions, i.e. in rain-dominated regions precipitation is sufficient, whereas in arid regions evapotranspiration needs to be considered and in regions with seasonal snow cover the inclusion of snow and run-off in the water balance model is mandatory. If the aim is to compare droughts in different regions, we recommend to use a complex water balance model for all regions to keep consistency. The study also shows that one has to be cautious when using and interpreting results based on simple index definitions, as fitting the distribution of the index to a gamma function is in some regions problematic.
Further studies shall be carried out to extend these conclusions to other regions and to analyse how different drought indices are related to external forcing variations and to internal variability including modes of variability (Gómez-Navarro et al., Citation2013; Raible et al., Citation2014; Ortega et al., Citation2015). Finally, the study shows that the current parametrizations of potential evapotranspiration are inaccurate in dry regions, calling for new approaches to either measure evapotranspiration directly (Nouri et al., Citation2015) or derive new parametrizations for evapotranspiration.
Additional information
Funding
Notes
No potential conflict of interest was reported by the authors.
References
- Alley, W. M. 1984. The palmer drought severity index: limitations and assumptions. J. Clim. Appl. Meteorol. 23, 1100–1109.
- Andreadis, K. M. and Lettenmaier, D. P. 2006. Trends in 20th century drought over the continental United States. J Clim. 22, 1962–1981.
- Arndt, D. S., Blunden, J. and Willett, K. W. 2015. State of the climate in 2014. Bull. Am. Meteorol. Soc. 96, 1–238.
- Beguería, S., Vicente-Serrano, S. M., Reig, F. and Latorre, B. 2014. Standardized precipitation evapotranspiration index (SPEI) revisited: parameter fitting, evapotranspiration models, tools, datasets and drought monitoring. Int. J. Climatol. 34, 3001–3023.
- Bouchet, R. J. 1963. Evapotranspiration reelle et potentielle, signification climatique. In: Proceedings of the International Association Scientific Hydrology Symposium on Hydrological Sciences Bulletin, Berkeley, CA, pp. 134–142.
- Chen, F. and Dudhia, J. 2001. Coupling an advanced land surface-hydrology model with the Penn State-NCAR MM5 modeling system. Part I: Model implementation and sensitivity. Mon. Weather Rev. 129, 569–585.
- Cook, E. R., Seager, R., Kushnir, Y., Briffa, K. R., Buentgen, U. and co-authors. 2015. Old World megadroughts and pluvials during the Common Era. Sci. Adv. 1, 1–9.
- Edwards, D. C. and McKee, T. B. 1997. Characteristics of 20th Century Drought in the United States at Multiple Time Scales. Technical report, Climatology Report Number 97-2. Colorado State University.
- Ek, M. B. 2003. Implementation of Noah land surface model advances in the national centers for environmental prediction operational mesoscale Eta model. J Geophys. Res. 108, 8851.
- Farahmand, A. and AghaKouchak, A. 2015. A generalized framework for deriving nonparametric standardized drought indicators. Adv. Water Res. 76, 140–145.
- Gómez-Navarro, J. J., Boethe, O., Wagner, S., Zorita, E., Werner, J. P. and co-authors. 2015. A regional climate palaeosimulation for Europe in the period 1501–1990. Part II: Comparison with gridded reconstructions. Clim. Past 11, 1077–1095.
- Gómez-Navarro, J. J., Montavez, J. P., Wagner, S. and Zorita, E. 2013. A regional climate palaeosimulation for Europe in the period 1500–1990. Part 1: Model validation. Clim. Past 9, 1667–1682.
- Gudmundsson, L., Bremnesa, J. B., Haugen, J. E. and Engen-Skaugen, T. 2012. Downscaling RCM precipitation to the station scale using statistical transformations -- a comparison of methods. Hydrol. Earth Syst. Sci. 16, 3383–3390.
- Haug, G. H., Gunther, D., Peterson, L. C., Sigman, D. M., Hughen, K. A. and co-authors. 2003. Climate and the collapse of Maya civilization. Science, 299, 1731–1735.
- Heim, R. R. 2002. A review of twentieth-century drought indices used in the United States. Bull. Am. Meteorol. Soc. 83, 1149–1165.
- Hobbins, M. T., Ramirez, J. A. and Brown, T. C. 2004. Trends in pan evaporation and actual evapotranspiration across the conterminous US: Paradoxical or complementary? Geophys. Res. Lett. 31, L13503.
- Jerez, S., Montavez, J. P., Gomez-Navarro, J. J., Jimenez-Guerrero, P., Jimenez, J. M. and co-authors. 2010. Temperature sensitivity to the land-surface model in MM5 climate simulations over the Iberian Peninsula. Meteorol. Z. 19, 363–374.
- Keyantash, J. and Dracup, J. A. 2002. The quantification of drought: an evaluation of drought indices. Bull. Am. Meteorol. Soci. 83, 1167–1180.
- Legutke, S. and Maier-Reimer, E. 1999. Climatology of the HOPE-G Global Ocean - Sea Ice General Circulation Model. Technical Report 21, Deutsches Klimarechenzentrum, Hamburg.
- Legutke, S. and Voss, R. 1999. The Hamburg Atmosphere-Ocean Coupled Circulation Model. Technical Report 18, Deutsches Klimarechenzentrum (DKRZ).
- Lloyd-Hughes, B. and Saunders, M. A. 2002. A drought climatology for Europe. Int. J. Climatol. 22, 1571–1592.
- Luksch, U., Raible, C. C., Blender, R. and Fraedrich, K. 2005. Decadal cyclone track variability in the North Atlantic. Metorol. Z. 14, 747–753.
- Ma, M., Ren, L., Yuan, F., Jiang, S., Liu, Y. and co-authors. 2014. A new standardized Palmer Drought Index for hydro-meteorological use. Hydrol. Processes 28, 5645–5661.
- Mariotti, A., Schubert, S., Mo, K., Peters-Lidard, C., Wood, A. and co-authors. 2013. Advancing drought understanding, monitoring, and prediction. Bull. Am. Meteorol. Soc. 94, 186–188.
- McKee, T. B., Doesken, N. J. and Kleist, J. 1993. The relationship of drought frequency and duration to time scales. Conference on Applied Climatology, Anaheim, CA, pp. 179–184.
- Mishra, A. K. and Singh, V. P. 2010. A review of drought concepts. J. Hydrol. 391, 202–216.
- Mo, K. C. and Chelliah, M. 2006. The modified Palmer Drought Severity Index based on the NCEP North American regional reanalysis. J. Appl. Meteorol. Climatol. 45, 1362–1375.
- Monteith, J. L. 1965. Evaporation and environment. Symp. Soc. Exp. Biol. 19, 205–234.
- Nouri, H., Beecham, S., Anderson, S., Hassanli, A. M. and Kazemi, F. 2015. Remote sensing techniques for predicting evapotranspiration from mixed vegetated surfaces. Urban Water J. 12, 380–393.
- Ortega, P., Lehner, F., Swingedouw, D., Masson-Delmotte, V., Raible, C. C. and co-authors. 2015. A multi-proxy model-tested NAO reconstruction for the last millennium. Nature 523, 71–75.
- Palmer, W. C. 1965. Meteorological Drought. U.S. Department of Commerce. Reserach Paper No. 45, 65 pp.
- Penman, H. L. 1948. Natural evaporation from open water, bare soil and grass. In: Proceedings of the Royal Society of London. Series A: Mathematical and Physical Sciences, Vol. 193. The Royal Society, London, pp. 120–145.
- Raible, C. C., Kleppek, S., Wueest, M., Kitoh, A., Murakami, H. and co-authors. 2012. Atlantic hurricanes and associated insurance loss potentials in future climate scenarios: limitations of high-resolution AGCM simulations. Tellus A 64, 15672.
- Raible, C. C., Lehner, F., Gonzalez-Rouco, J. F. and Fernandez-Donado, L. 2014. Changing correlation structures of the Northern Hemisphere atmospheric circulation from 1000 to 2100 AD. Clim. Past 10, 537–550.
- Raible, C. C., Luksch, U. and Fraedrich, K. 2004. Precipitation and northern hemisphere regimes. Atmos. Sci. Lett. 5, 43–55.
- Raible, C. C., Luksch, U., Fraedrich, K. and Voss, R. 2001. North Atlantic decadal regimes in a coupled GCM simulation. Clim. Dyn. 18, 321–330.
- Rajczak, J., Kotlarski, S. and Schär, C. 2016. Does quantile mapping of simulated precipitation correct for biases in transition probabilities and spell-lengths? J. Clim. 29, 1605–1615.
- Roeckner, E., Arpe, K., Bengtsson, L., Christoph, M., Claussen, M. and co-authors. 1996. The Atmospheric General Circulation Model ECHAM-4: Model Description and Simulation of Present-Day Climate. Technical Report 218, Max-Planck-Institut fuer Meteorologie.
- Skamarock, W. C., Klemp, J. B., Dudhia, J., Gill, D. O., Barker, D. M. and co-authors. 2008. A Description of the Advanced Research WRF Version 3. Technical Report tn-475+str, National Center for Atmospheric Research Boulder, Colorado, USA.
- Steinemann, A. C. and Cavalcanti, L. F. N. 2006. Developing multiple indicators and triggers for drought plans. J. Water Res. Planning Manage. 132, 164–174.
- Svoboda, M., LeComte, D., Hayes, M., Heim, R., Gleason, K. and co-authors. 2002. The drought monitor. Bull. Am. Meteorol. Soc. 83, 1181–1190.
- Themessl, M., Gobiet, A. and Leuprecht, A. 2011. Empiricalstatistical downscaling and error correction of daily precipitation from regional climate models. Int. J. Climatol. 31, 1530–1544.
- Thornthwaite, C. W. 1948. An approach toward a rational classification of climate. Am. Geograph. Soc. 38, 55–94.
- van der Schrier, G., Efthymiadis, D., Briffa, K. R. and Jones, P. D. 2007. European Alpine moisture variability for 1800–2003. Int. J. Climatol. 27, 415–427.
- Vicente-Serrano, S. M., Beguería, S. and López-Moreno, J. I. 2010. A multiscalar drought index sensitive to global warming: The standardized precipitation evapotranspiration index. J. Clim. 23, 1696–1718.
- Wells, N., Goddard, S. and Hayes, M. J. 2004. A self-calibrating Palmer Drought Severity Index. J. Clim. 17, 2335–2351.
- Wilhite, D. A. 2000. Drought as a Natural Hazard: Concepts and Definitions, Vol. 1. London: Routledge.
- Wu, H., Svoboda, M. D., Hayes, M. J., Wilhite, A. and Wen, F. 2007. Appropriate application of the standardized precipitation index in arid locations and dry seasons. Int. J. Climatol. 79, 65–79.
- Zorita, E., von Storch, H., Gonzalez-Rouco, F. J., Cubasch, U., Luterbacher, J. and co-authors. 2004. Climate evolution in the last five centuries simulated by an atmosphere-ocean model: global temperatures, the North Atlantic Oscillation and the Late Maunder Minimum. Meteorol. Z. 13, 271–289.
- Zotarelli, L., Dukes, M. D., Romero, C. C., Migliaccio, K. W. and Kelly, T. 2013. Step by Step Calculation of the Penman--Monteith Evapotranspiration (FAO-56 Method). University of Florida, IFAS Extension, pp. 1–10.
Appendix 1
Estimation of evapotranspiration and potential evapotranspiration
For the water balance, also temperature effects needs to be considered. Thereby, the concept of evapotranspiration ET is used for describing processes of water loss from the land surface to the atmosphere. The two major processes considered are evaporation and transpiration. However, direct measurements of ET are often not available due to technical difficulties. Therefore, several methods have been developed to estimate the effects of ET through more readily available data (Zotarelli et al., Citation2013).
In general, ET depends on solar radiation, air temperature, relative humidity, wind speed, crop characteristics and land use. In particular, the latter two variables make the estimation of ET difficult as they strongly vary on small spatial scales. However, when the latent heat flux from the Earth’s surface to the atmosphere is known, the monthly ET can be estimated directly:
where the latent heat flux,
is the total latent heat of a month, which is
integrated over one month,
the latent heat of water vaporization, and
is the number of seconds in a month. The advantage of this method is that the latent heat flux is calculated by the RCM, whereas ET is not.
Another approach is to use the so-called potential evapotranspiration PET. PET is defined by Zotarelli et al. (Citation2013) as the rate of evapotranspiration from a given uniform reference soil with readily available liquid water, overgrown with a dense, actively growing vegetation. It represents the evapotranspiration demand of the atmosphere, without taking into account the crop and soil type. Thus, PET is easier to estimate than ET, as only climatic variables are required.
Two methods are commonly used to estimate PET. The first one models PET using empirical relationships from observations (e.g. Thornthwaite, Citation1948). This method is often used because of its simplicity as it only depends on temperature and location:
where T is the monthly mean temperature in C.
is a heat index given by
where is the average temperature for month i. k is calculated by
(A1)
and K is a coefficient that accounts for latitude and month. For a year with 360 days, which is the calendar of the RCM used, it is given by(A2)
where is the latitude in radian and i is the month. The obvious advantage is that monthly temperatures are used to estimate PET. However, it is a crude approximation and is not defined for negative temperatures making it not suitable for many European regions in winter (Vicente-Serrano et al., Citation2010).
Another approach to estimate PET directly models the physical processes, e.g. the method of Penman–Monteith (Vicente-Serrano et al., Citation2010). It is based on an energy balance and aerodynamic formula, which requires temperature, wind speed, air humidity, elevation, and latitude (Penman, Citation1948; Monteith, Citation1965):(A3)
where is the slope of the saturation vapour pressure curve,
is the net radiation at the crop surface (MJ
), G is the soil heat flux density (MJ
),
is the psychrometric constant (
), T the daily mean temperature (
),
the wind speed at 2 m above ground (m
),
the mean saturated vapour pressure (kPa) and
the mean daily ambient vapour pressure (kPa). Details about how to calculate those variables from input data can be found in Zotarelli et al. (Citation2013). It can be calculated daily or hourly and is the recommended method when sufficient data are available.