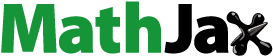
Abstract
A data assimilation method capable of constraining the sea ice of an Earth system model in a dynamically consistent manner has the potential to enhance the accuracy of climate reconstructions and predictions. Finding such a method is challenging because the sea ice dynamics is highly non-linear, and sea ice variables are strongly non-Gaussian distributed and tightly coupled to the rest of the Earth system – particularly thermodynamically with the ocean. We investigate key practical implementations for assimilating sea ice concentration – the predominant source of observations in polar regions – with the Norwegian Climate Prediction Model that combines the Norwegian Earth System Model with the Ensemble Kalman Filter. The performances of the different configurations are investigated by conducting 10-year reanalyses in a perfect model framework. First, we find that with a flow-dependent assimilation method, strongly coupled ocean–sea ice assimilation outperforms weakly coupled (sea ice only) assimilation. An attempt to prescribe the covariance between the ocean temperature and the sea ice concentration performed poorly. Extending the ocean updates below the mixed layer is slightly beneficial for the Arctic hydrography. Second, we find that solving the analysis for the multicategory instead of the aggregated ice state variables greatly reduces the errors in the ice state. Updating the ice volumes induces a weak drift in the bias for the thick ice category that relates to the postprocessing of unphysical thicknesses. Preserving the ice thicknesses for each category during the assimilation mitigates the drift without degrading the performance. The robustness and reliability of the optimal setting is demonstrated for a 20-year reanalysis. The error of sea ice concentration reduces by 50% (65%), sea ice thickness by 25% (35%), sea surface temperature by 33% (23%) and sea surface salinity by 11% (25%) in the Arctic (Antarctic) compared to a reference run without assimilation.
1. Introduction
Sea ice is a key element of the climate system as it affects the radiative surface balance and strongly restricts exchanges of momentum, heat and moisture between the ocean and the atmosphere. In addition, the salt and freshwater exchanges of the sea ice with the ocean through freezing and melting influences the thermohaline circulation (Mauritzen and Häkkinen, Citation1997; Serreze et al., Citation2007). Reliable predictions of sea ice from several months to several years would have many implications: They would help the Arctic local communities to prepare for the substantial impacts of anomalous sea ice conditions on fishing and extreme events (McGoodwin, Citation2007; Einarsson et al., Citation2014). Likewise, they would help to limit accidents associated with commercial activities in the polar regions, such as the exploration of natural resources and maritime transportation (Liu and Kronbak, Citation2010), which are rapidly expanding because of the anthropogenically driven sea ice decline. The anomalous Arctic sea ice conditions are also expected to have a large influence on the climate beyond the polar regions (Deser et al., Citation2015). Besides, numerous studies indicate links between the Arctic sea ice state and remote spring weather events (see Gao et al., Citation2015 for a review of that topic). The mechanisms involved are not fully understood, in particular, as the sparse (both temporal and spatial) observational network severely hampers their interpretation. In the Antarctic, though the overall sea ice extent is slightly increasing, regional changes in the spatio-temporal characteristics of the sea ice have a significant impact on the Antarctic ecosystem, as for instance, in the region of the West Antarctic Peninsula (Massom and Stammerjohn, Citation2010). As in the Arctic, the mechanisms involved are poorly understood (Holland et al., Citation2014; Yuan et al., Citation2017). A deeper comprehension can be inferred from continuous and reliable reconstructions of the past climate to reduce its uncertainties.
Historical reanalyses serve this purpose by fusing scarce observations into dynamically consistent models. While reanalyses of the atmosphere (e.g. Kalnay et al., Citation1996; Dee et al., Citation2011) have been available for over a decade, the paucity of observation in the ocean and sea ice have made these reconstructions more challenging. Atmospherically forced reconstructions of the ocean and the sea ice have been attempted with a predominant focus on the Arctic (e.g. Nguyen et al., Citation2011; Sakov et al., Citation2012; Chevallier et al., Citation2016) compared to the Antarctic (e.g. Massonnet et al., Citation2013; Barth et al., Citation2015). Running coupled ocean and sea ice models with atmospheric forcing has allowed reaching high levels of accuracy, but it does not optimally account for the coupled dynamics of the climate system and affects the realism of the variability mechanisms. There is a need for reconstructions that can assimilate observations directly into Earth system models.
Another asset of reanalyses is that they can provide initial conditions for climate predictions that aim to initialise the internal climate variability of the system. While weather forecasting is limited to several days due to the chaotic nature of the atmosphere, there is a potential for skilful predictions up to a decade or more by constraining the slowly varying modes of the climate system, which mainly reside in the ocean, the land and in the sea ice (Meehl et al., Citation2009). In the Arctic, a significant part of the predictability can be explained by persistence of sea ice anomaly, mostly involving sea ice thickness and its coupling to the upper ocean (Guemas et al., Citation2016). For the Antarctic, only very few studies on the topic are available, indicating significant potential predictability (Holland et al., Citation2013). However, except for the most recent few decades when a more exhaustive observational network is available, sea ice concentration has been the predominant source of observations available to monitor the variability of the polar region during the past nearly 40 years. Hence, a method that can optimally and reliably make use of ice concentration observations to constrain the climate variability of the polar regions – including unobserved variables such as ice thickness and sub-surface hydrography – would have the potential to enhance the skill of seasonal to decadal predictions.
Data assimilation (DA) methods make use of observations, a dynamical model and their respective error statistics to estimate a new improved model state in a consistent manner. Previous assimilation systems have mainly demonstrated skill for initialising a single dominant component of the Earth system (atmosphere, ocean, land surface or cryosphere) where the adjustments across model components occur purely dynamically during the model integration. This approach is often categorised as weakly coupled DA (Laloyaux et al., Citation2016). A joint update of several components of an Earth system is referred to as strongly coupled DA (Laloyaux et al., Citation2016; Penny and Hamill, Citation2017), and aims at instantly propagating information from the observations across all model components. Strongly coupled DA (see Penny and Hamill, Citation2017 for a review of that topic) is expected to improve the dynamical consistency of the initial conditions because all model components are jointly updated using a given set of observations. Furthermore, it is also expected to improve the optimality of the assimilation because observations can influence the variability across the model components where they have been observed. This becomes particularly important when one model component crucially lacks observations as for example, the ocean component in the Arctic Ocean.
Assimilation of ice concentration is clearly a coupled problem and serves as a good test case for assessing the benefits of strongly coupled DA over weakly coupled DA. For instance, the ocean and the sea ice are thermodynamically coupled. Guemas et al. (Citation2016) envisioned that a system capable of initialising consistently the ocean and the sea ice components will lead to further improved model states over the weakly coupled approach when only the sea ice is updated by the assimilation. Although one expects that a system capable of jointly assimilating data in the ocean, in the sea ice and in the atmosphere has great potential, it raises new challenges as it involves complex, coupled phenomena over widely separated spatial and temporal scales. Considering only the ocean and the sea ice – the main objective of our study – is somewhat simpler because the time and spatial scales and the amplitude of the velocities between the upper ocean and the sea ice are more comparable, but strongly coupled ocean–sea ice DA is still a challenging task. While the relation between the sea ice and the ocean surface temperature always has the same sign, the cross-covariance between sea ice and the sea surface salinity is strongly non-stationary and anisotropic (Lisæter et al., Citation2003; Sakov et al., Citation2012). Besides, updating the ocean surface temperature without salinity may destratify the ocean, which can have severe implications on the overturning circulation.
Flow-dependent assimilation methods, such as the Ensemble Kalman Filter (EnKF; Evensen, Citation2003), have shown some assets for providing a joint update of the ocean and sea ice states as such methods can handle well the non-stationary and anisotropic covariances. The EnKF approach has been extensively tested with atmospherically forced, coupled ocean and sea ice models for reanalyses and short-time forecasts up to 10 days (Lisæter et al., Citation2003; Mathiot et al., Citation2012; Sakov et al., Citation2012; Massonnet et al., Citation2015; Barth et al., Citation2015). However, Mathiot et al. (Citation2012) reported that sea ice DA led to a net increase in the oceanic salt content in their simulations, a degradation that can be overcome by salinity restoring to climatological values (see also Massonnet et al., Citation2014). Salinity restoring is commonly used in forced ocean and sea ice simulations to counteract the drift caused by the de-coupled estimation of the precipitation, evaporation and other unresolved coupled feedbacks. Yet, the use of salinity restoring in coupled climate simulations is problematic because it dampens the internal variability of the system and defining a climatological reference in a changing climate with very sparse observations is challenging. There is a need to clearly identify the influence of the assimilation of ice concentration on the ocean hydrography in a controlled assimilation experiment, before one can assimilate real observations in an Earth System model without restoring to climatology. Flow-dependent assimilation methods like the EnKF have also been found to be advantageous due to their multivariate properties. For instance, several studies using such methods found that the assimilation of ice concentration reduces the bias of the sea ice thickness in the Arctic (Sakov et al., Citation2012; Yang et al., Citation2015; Xie et al., Citation2016) and in the Antarctic (Massonnet et al., Citation2013). However, the presented results are somewhat debatable due to the scarce number of ice thickness observations and the short-time span over which the validations are carried out. Finally, assimilation of sea ice has become even more challenging with the emergence of multicategory sea ice models such as CICE4.0 (Hunke and Lipscomb, Citation2008) or LIM3 (Vancoppenolle et al., Citation2009). A method for adapting the assimilation of sea ice to such a model was proposed in Massonnet et al. (Citation2015). A typical challenge of assimilating sea ice is that the linear analysis update – as used in the EnKF – applied to a non-Gaussian distributed variable (such as sea ice concentration or thickness), returns unphysical values that introduce a bias when these variables are postprocessed (e.g. when forced to maintain a physical range). In multicategory models, the number of variables that are non-Gaussian distributed is increased compared to a single category model, which may amplify the emergence of the bias. Projecting the state variables into a Gaussian distributed space (Barth et al., Citation2015) may diminish those biases. This approach, however, also entails issues as the cumulative density function of sea ice concentration is not differentiable (close to 0 and 1), generating atoms of the PDF. Thus, a properly defined bijective function which projects the PDF to a Gaussian function is lacking.
It is therefore timely to investigate the feasibility, advantages and inconveniencies of different possible implementations of sea ice concentration assimilation in a controlled environment before applying the method in a fully-coupled system with real observations. By only assimilating ice concentration in a fully-coupled system that uses a multicategory sea ice model, we aim at identifying the optimal assimilation strategy that can maximise the efficiency of the assimilation while minimising the drift in the performance. All tests are carried out in idealised twin experiments (observing system simulation experiments), meaning that we extract synthetic observations from the same model on the same grid at a different period; all data presented in this manuscript are derived under pre-industrial conditions. As such the true solution is known, the model is perfect (unbiased) and we can thoroughly study the impact of each assimilation implementation on the full state vector. Note that, we assimilate observations in both hemispheres. The performance of these schemes is tested in reanalysis mode with a monthly assimilation cycle for a period of 10 years. We use the fully-coupled Norwegian Climate Prediction Model (NorCPM, Counillon et al., Citation2014) that is based on a CMIP5 system, the Norwegian Earth System Model (NorESM, Bentsen et al., Citation2013) and the EnKF DA method. NorESM uses an isopycnal ocean model and the multicategory sea ice model CICE4.0 (Hunke and Lipscomb, Citation2008).
This paper is organised as follows. In Section 2, we present NorCPM which combines the NorESM (Section 2.1) and the EnKF (Section 2.2). Section 3 describes the experimental set-up. In Section 4, we first present the results that identify the optimal approach to constrain a multicategory sea ice model (Section 4.1) and latter discuss the optimal formulation for cross model component updates between the ocean and the sea ice (Section 4.2). In Section 5, we verify the optimal setting for a 20-year reanalysis. We close the article with a section, where we discuss our findings and give an outlook for future developments.
2. The Norwegian Climate Prediction Model
The Norwegian Climate Prediction Model (NorCPM, Counillon et al., Citation2014; Counillon et al., Citation2016; Wang et al., Citation2016; Wang et al., Citation2017) comprises the Norwegian Earth System Model (NorESM, see Section Bentsen et al., Citation2013) and an implementation of the Ensemble Kalman filter (EnKF, see Section 2.2). Former versions of NorCPM carried out DA in the ocean component of NorESM only (weakly coupled DA), letting the adjustment to the other components occur dynamically during the integration of the system. Here, we aim at constraining the sea ice component of NorESM and we evaluate the possibility of carrying out a joint update (strongly coupled DA) of the ocean and the sea ice
2.1. The Norwegian Earth System Model
NorESM (Bentsen et al., Citation2013) is a global, fully-coupled system developed for climate simulations. It is based on the Community Earth System Model version 1.0.3 (CESM1, Vertenstein et al., Citation2012), which is a successor to the Community System Model version 4 (CCSM4, Gent et al., Citation2011). As in the CESM1, the NorESM combines the Community Atmosphere Model (CAM4,Neale et al., Citation2010), the Community Land Model (CLM4, Oleson et al., Citation2010; Lawrence et al., Citation2011), the Los Alamos sea ice model (CICE4, Gent et al., Citation2011; Holland et al., Citation2012) and an updated version (Bentsen et al., Citation2013) of the isopycnal coordinate ocean model MICOM (Bleck et al., Citation1992) with the version 7 coupler (CPL7, Craig et al., Citation2011).
This study uses a computationally light variant of NorESM (Schwinger et al., Citation2016; Wang et al., Citation2017) with the original CAM4 atmosphere component configured on a regular 1.9 by 2.5
latitude–longitude grid and the ocean component MICOM and the sea ice component CICE4 both configured on a tripolar grid with a nominal resolution of 2
that is enhanced towards the equator.
MICOM uses 2 density varying super layers representing the mixed layer and 51 isopycnal layers respecting the chosen reference potential densities in the range with reference pressure set to 2000 dbar. In contrast to the remaining isopycnal layers, the first isopycnal layer (below the mixed layer) is not required to stay close to its prescribed reference potential density. Applying potential density as vertical coordinate in MICOM minimises spurious mixing across the neutral surfaces and ensures excellent conservation of water masses. Salt released during freezing of sea ice is distributed evenly below the mixed layer – below the two super layers – down to the depth with a density contrast of
compared to the surface and a cut-off at 500 m (Bentsen et al., Citation2013).
The sea ice component CICE4 is equipped with a number of ice thickness categories (we use the predefined value of ) to account for the different thermodynamic and dynamic properties of ice with different thicknesses. Volume of snow and ice, energy content, as well as ice concentration, surface temperature and the volume-weighted mean ice age are determined for each of the ice thickness categories. As in CESM1, we use the delta–Eddington short-wave radiation transfer (Briegleb and Light, Citation2007), as well as melt pond and aerosol parameterisations, all detailed in Holland et al. (Citation2012).
NorESM was initialised with data from the Polar Hydrographic Climatology version 3.0 (PHC, Steele et al., Citation2001) and then spun up for more than 1500 years using constant preindustrial forcing. All runs presented in this study are using pre-industrial forcing. The initial ensemble and the reference ‘truth’, from which the observations have been constructed, were generated by sampling from that run as explained in Section 3.
2.2. The ensemble Kalman filter
Data assimilation (DA) schemes use observations, a dynamical model and their error statistics in order to derive improved model states. Newtonian relaxation (nudging, see e.g. Tietsche et al., Citation2013) assumes error statistics to be constant in space and time and yields monovariate updates, which are suboptimal and introduce dynamical inconsistencies. DA methods based on static and multivariate covariances have been tested with some degree of success for a regional system of the Canadian East coast region (Caya et al., Citation2010) and the Baltic Sea (Axell and Liu, Citation2016). However, the cross-covariance between sea ice and the sea surface salinity in the Arctic is strongly non-stationary and anisotropic (Lisæter et al., Citation2003; Sakov et al., Citation2012), which requires the use of flow-dependent assimilation methods such as the EnKF DA method. Multiple systems based on the EnKF have been recently emerging (Sakov et al., Citation2012; Massonnet et al., Citation2014; Yang et al., Citation2015; Barth et al., Citation2015).
The EnKF (Evensen, Citation2003) is a sequential DA that consists of a Monte Carlo model integration and a linear variance minimising update. The method is well designed for non-autonomous and non-stationary dynamical systems such as our Earth system model. The method is multivariate – meaning it propagates the information from an observed variable to non-observed variables. The covariances are constructed from the ensemble and as such are flow-dependent. Finally, assimilation shocks are limited as the covariances are model-based and the resulting updates are in equilibrium – presuming the model error correlations are linear (Evensen, Citation2003).
We use a deterministic variant of the EnKF (DEnKF) as described in Sakov and Oke (Citation2008). The DEnKF updates the ensemble perturbations around the updated mean using a Taylor expansion of the expected corrections towards the forecast. This yields an approximate but deterministic form of the traditional stochastic EnKF.
Given an ensemble of m (forecast) model states , the ensemble average
and
. The ensemble anomalies are defined as
. The observation vector is given by
and has a prescribed error covariance matrix
that is diagonal, meaning the observation errors are assumed uncorrelated. The quantity
is the linear observation operator (here a matrix) that is mapping the state vector to the observational space. The DEnKF update of the forecast model states can be written as follows:
(1)
(1)
(2)
(2)
(3)
(3)
where is referred to as the Kalman gain and
is the forecast error covariance matrix. As we do not expect that using the DEnKF would change any of the conclusions of that paper (Sakov and Oke, Citation2008), we do not distinguish the DEnKF from the traditional EnKF in the following.
In our applications, we use 30 ensemble members. To limit spurious long-range correlations resulting from sampling errors, we use a local analysis framework (Evensen, Citation2003). We found that a fixed localisation radius of 800 km performs well for the assimilation of sea ice. This value is in a good agreement with the value used by Massonnet et al. (Citation2015) and by Wang et al. (Citation2017) at high latitudes. Beside, this value is within the spatial decorrelation range (500 km to 1000 km) of sea ice thickness as reported in Blanchard-Wrigglesworth and Bitz (Citation2014). The rest of the practical implementation follows that of Counillon et al. (Citation2014), Wang et al. (Citation2016).
The observations are sequentially assimilated in the middle of each month. The innovation (i.e. the difference between the observation and the model) compares the monthly averaged observations with a snapshot of the model (ensemble mean of the restart files). As a consequence, the variability of the model and of the observations are not fully comparable, but it allows for carrying out a centred analysis. This approach was found successful in Counillon et al. (Citation2014). For the ocean variables, the analysis is only applied to the first time level (of the leap frog scheme) and copied to the second time level. The observational errors are weighted by a distance-dependent localisation function (Gaspari and Cohn, Citation1999; Hamill et al., Citation2001; Sakov and Bertino, Citation2010) to avoid discontinuities at the edge of the local domain. In order to avoid an abrupt start of the DA spin up, the observation error variance is inflated by a factor of 8 and gradually decreased over five assimilation cycles (5 months), as proposed in Sakov et al. (Citation2012). Note also that for practical reasons, we skip the very first assimilation of each assimilation experiment. The ensemble spread is sustained using a moderation technique and a pre-screening method (Sakov et al., Citation2012). The moderation technique consists of increasing the observational error variance (here by a factor of 2) for the update of the ensemble anomalies while the original observation error variance is used for the update of the ensemble mean. In the pre-screening method, the observation error is inflated so that the posterior () remains within two standard deviations of the prior (
). It should also be noted that the DEnKF overestimates the analysed error covariance by adding a positive-semidefinite term to the theoretical error covariance given by the Kalman filter, which reduces the amount of inflation needed.
3. Experimental set-up
In a fully-coupled Earth system model, single error contributions can evolve in a very complex and non-linear way across all model components. Due to the lack of independent data for monitoring the performance of the entire system, it is common practice to test the system in an idealised perfect twin experiment assimilation framework – also referred to as an Observing System Simulation Experiment (OSSE).
In our experimental set-up, the ‘true’ solution (henceforth denoted as TRUTH) stems from January 2021 to December 2040 of our preindustrial run of NorESM. All other experiments are started from a 30-member ensemble, which is sampled from the January month of our preindustrially forced simulation, taken every 20 years between 1101 and 1681. These choices ensure that the initial ensemble spans sufficient spread and that the initial condition can be considered as independent from TRUTH. The model has very little drift so that the framework can be considered to be bias-free. Every month, the assimilation experiments assimilate synthetic observations (monthly averaged ice concentration extracted from TRUTH) for a period of 10 years. Their skill will be assessed by comparing the accuracy of the whole state variables against that of TRUTH. Their performance will be compared to the experiment FREE, for which the same initial ensemble is freely evolving without assimilation throughout the entire considered period. FREE serves as a benchmark to quantify when an assimilation experiment has improved or degraded the error.
The sea ice concentration field ranges between 0% and 100%, and its Probability Density Function (PDF) has peak modes around 0% and 100%. Adding white noise would produce unphysical values beyond that range and truncating the unphysical values would change the mean. This is exemplified in Fig. , where we added white noise with 10% standard deviation to the observation; cut-off the values out of the bound (0% and 100%) and recalculated the mean. Adding a bias when generating synthetic observations is problematic as the bias is one of the criteria that we assess in the different assimilation schemes. We have therefore decided not to perturb the observations derived from the monthly sea ice concentrations of TRUTH, but we assume an observation error of 10% during the assimilation as in the standard observational product of ice concentration, which is the order of magnitude of uncertainties seen in the observations (see e.g. Mathiot et al., Citation2012). This choice implies that we assimilate a data-set that is more accurate than assumed in the DA framework. Hence, our assimilation is suboptimal, which will have implications in the reliability budget analysis carried out in Section 6 – the ensemble spread is expected to overestimate the root mean square error (rmse), see Equations (Equation4(4)
(4) )–(Equation7
(7)
(7) ).
Figure 1. Histogram of the synthetic observations of sea ice concentration (aice [%]) in December 1 without added white noise (blue) and with added white noise (red) with 10% standard deviation and cut-off of unphysical values. The evaluation is restricted to grid cells in which TRUTH contained sea ice at least once. The dashed lines indicate the mean values of the resulting ice concentrations, which differ between the unperturbed and the perturbed case.
![Figure 1. Histogram of the synthetic observations of sea ice concentration (aice [%]) in December 1 without added white noise (blue) and with added white noise (red) with 10% standard deviation and cut-off of unphysical values. The evaluation is restricted to grid cells in which TRUTH contained sea ice at least once. The dashed lines indicate the mean values of the resulting ice concentrations, which differ between the unperturbed and the perturbed case.](/cms/asset/1d8c97f7-3bf3-4be4-a072-4d0f0cc1f431/zela_a_1435945_f0001_oc.gif)
We explore different assimilation strategies of ice concentration with a suite of experiments that are run for a period of 10 years. The experiments differ in the composition of the assimilation state vector () and the determination of the diagnosed variables (see Table ).
Table 1. List of experiments analysed. The second and the third column detail the variables assimilated in the sea ice and in the ocean. The right column indicates the variables that are diagnosed a posteriori, see also Appendix, Table . The index ‘1 : 5’ refers to the five thickness categories, while the lack of an index in the ice state variables refers to the aggregate values. The abbreviation ‘ML’ refers to the elements in the mixed layer of the ocean, i.e. layers 1 and 2; ‘:’ indicates that we include the entire ocean column into the state vector.
Table 2. List of variables. We use the five thickness categories of CICE4.0 with one being the thinnest and five the thickest.
A postprocessing step is applied after each assimilation to ensure that the prognostic variables are in agreement with the variables assimilated and that the initial condition is sufficiently consistent with the model physics (see Table of the Appendix 1). While the assimilation only updates a small subset of the model state variables (those that contain most of the ’climate memory’) in our experiments, the remaining state variables are thus adjusted in the postprocessing step to minimise the assimilation shock and to allow a (smooth) model restart after the assimilation.
The first set of experiments in Table is used in Section 4 to investigate the optimal choice of the sea ice state vector for the assimilation and the second set of experiments is used in Section 5 to investigate the optimal choice for the ocean component.
To assess the performances of the experiments, we will use the following metrics. They are all calculated from monthly averages and determined for different state variables that are presented in Table . While consists of the ensemble of the monthly averaged analysis, the quantity
refers to its ensemble mean
. The overline indexed with
denotes ensemble averaging.
is the monthly average of TRUTH.
(4)
(4)
(5)
(5)
(6)
(6)
(7)
(7)
where is the area weighted spatial average. Unless otherwise specified, this average includes both hemispheres together. For the evaluation of the rmse and the bias of a sea ice variables, the spatial mean is restricted to grid cells that contained sea ice at least once in any of the simulations. For the evaluation of the error statistics of ocean variables we consider only regions with latitudes poleward of
North and South in order to focus on the local impact of the assimilation on the hydrography of the polar regions and not to include the remote oscillations (e.g. ENSO), that may shadow the local variability.
denotes the temporal average of the monthly quantity. The time-dependent spatial and the space-dependent temporal average of the ensemble spread (i.e. the variance),
and
, are calculated in a similar way as for bias and rmse.Footnote1
4. Optimised sea ice vector with multicategory ice states
CICE 4.0 allows the use of different ice thickness categories, meaning that the sea ice in each grid cell is partitioned into N ice thickness categories. Prognostic variables, such as ice concentration (aice) or volume (vice
), are defined for each ice category. In contrast, satellite ice concentrations only estimate the total ice concentration (aice). In this section we evaluate, which sea ice variables should be updated by the assimilation in order to make best use of these observations. For instance, we discuss the question, whether it is preferable to update all category-dependent variables in the analysis step or whether it is beneficial to update only the accumulated variables and then to adjust the individual category proportionally after the assimilation via postprocessing. Note, that this comparison does not discuss the quality of multicategory and single category sea ice models (as done e.g. in Uotila et al., Citation2017), but how to best assimilate data with a multicategory one. In all experiments of this section, we update the ocean temperature and salinity of the mixed layer in addition to the sea ice variables. This choice is found to be reasonable in Section 5.
4.1. Assimilation of aggregated ice states
We focus first on the assimilation run referred to as SINGLE (see Table ). In SINGLE, only the aggregate ice concentration (aice) and the aggregate ice volume (vice) are corrected. aice and vice
are then uniformly scaled with the changes of aice and vice. An interesting property of uniform scaling is that variables cannot change sign (i.e. aice
and vice
cannot become negative) and that a variable in a category being null remains so. Unphysical values returned by the assimilation are postprocessed and variables not updated by the assimilation (i.e. Tsfc
, vsno
, eice
) are diagnosed from the assimilated variables (see Appendix 1).
Figure 2. (bold lines) and
(thin lines) of aice (a,b), hi (c,d) and vice
(e,f) in the Arctic (left column) and in the Antarctic (right column) for FREE (red), SINGLE (orange), MULTI (green) and HI_PRESERVE (blue).
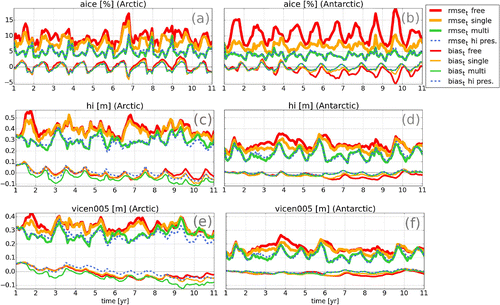
In FREE, the of aice fluctuates around 10% in the Arctic (bold red line in Fig. a) and shows a comparatively weaker seasonal variability in the performance, unlike in the Antarctic (Fig. b) where the
strongly varies interseasonally (up to 10%) reaching values up to 19% during the Antarctic winter. The slow variability in the performance of the bias relates to the slow decadal variability of TRUTH in the Antarctic that is removed by the ensemble averaging in FREE.
Figure 3. Differences in of aice between SINGLE and FREE in the Arctic (a) and in the Antarctic (b) with maximum
of 29%, and between MULTI and SINGLE in the Arctic (c) and in the Antarctic (d) with maximum
of 21%.
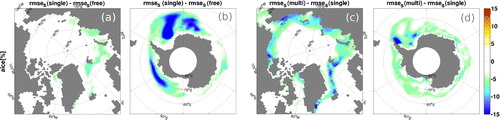
Figure 4. rmse of all considered variables for FREE (red), SINGLE (orange), MULTI (green) and HI_PRESERVE (blue) restricted to the Arctic region (a) and to the Antarctic region (b), respectively.
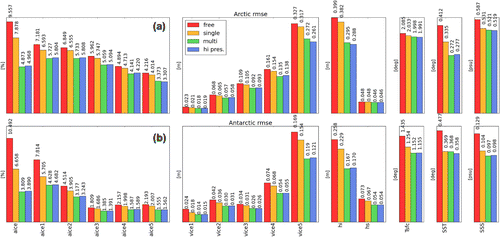
SINGLE (bold orange lines) reduces the rmse of aice compared to FREE, which is expected since this variable is assimilated. DA imposes that the rmse of the analysis is below 10% during each assimilation cycle. We therefore anticipate that the error of the monthly average (shown here) might only slightly exceed that level unless the error increases rapidly within the assimilation cycle. The of SINGLE fluctuates around 8% in the Arctic and around 7% in the Antarctic but repeatedly crosses the observation error level of 10% in the Arctic during summer time. The slow variability mode of the bias in the Antarctic seen in FREE is well constrained by the assimilation. In both hemispheres and alike FREE, SINGLE produces slightly too high aice values in summer and slightly too low values in winter compared to TRUTH. Looking at the spatial distribution of the time-averaged rmse reduction for aice (Fig. a,b), the main benefit of SINGLE is found along the ice edge, in places where the error in FREE is largest. The strongest (overall) improvements occur in the Antarctic – e.g. in the Weddell Sea and in the Amundsen Sea with maximal enhancements of nearly 30%. There, the interplay of Antarctic Circumpolar Current with the topography causes large variability in the advection of sea ice (see e.g. Timmermann et al., Citation2002). In the Arctic, the improvements are smaller and mostly distributed in regions influenced by Atlantic water inflow, e.g. the Barents Sea, the Kara Sea and the Laptev Sea. In both hemispheres, the improvements are more pronounced for regions dominated by thin and young ice. It should be noted that the sea ice in the Antarctic is predominantly thin and young compared to the Arctic, where sea ice is spread over more thickness categories and may survive a year. The fact that the improvement of concentrations are mostly found for thin ice category is also obvious from Fig. that shows the averaged reduction of error of concentration organised per category. The error reduction is also rather minor for the total ice thickness (Fig. c,d) and for the ice volume of the thickest ice (Fig. e,f). The improvement of total thickness of SINGLE over FREE is small (1.7 cm; a reduction of less than 5% in the Arctic and 2.9 cm (11%) in the Antarctic).
There is a slight error reduction in the remaining variables (e.g. snow fraction and temperature of ice) that are not updated during assimilation; it occurs dynamically during the model integration as a downstream consequence of the improved sea ice state.
4.2. Multicategory assimilation
Instead of updating the aggregated ice concentration and volume by the EnKF as done in SINGLE, we now update the ice concentration and the ice volume of each category (aice and vice
) by the EnKF (MULTI). As in SINGLE, unphysical values returned by the assimilation are postprocessed as described in Appendix 1. In contrast to SINGLE, the
of aice of MULTI (bold green lines in Fig. a,b) constantly remains below the observation error level; fluctuating around 5% in the Arctic and 4% in the Antarctic; the
is 40% lower than in SINGLE. Comparing the spatial distribution of the
reduction for MULTI over SINGLE (Fig. c,d) with those of SINGLE over FREE (Fig. a,b) we recognise in both hemispheres that in contrast to Fig. a,b the improvements in Fig. c,d are of similar order of magnitude and found in regions beyond the first-year ice covering also multi-year ice areas. Accordingly, MULTI overall noticeably reduces the rmses in the ice concentrations of all thickness categories (Fig. ). Note, that except for the concentrations of the thinnest ice in the Antarctic (and thus for the overall Antarctic aice), the error reduction of the ice concentrations in MULTI over SINGLE is larger than that of SINGLE over FREE.
The improved performance of MULTI over SINGLE is also very pronounced for hi, the aggregate ice thickness, in both hemispheres (Figs. c,d and ). MULTI shows a substantial rmse reduction over FREE (10.4 cm (26%) in the Arctic and 9.1 cm (35%) in the Antarctic). The improvements in each category of vice seem to be tightly related to the improvements of aice
(not shown). The errors in the remaining variables, such as snow thickness hs, temperature of the ice/snow at the top surface Tsfc
and the ocean surface variables SST and SSS are also smaller in MULTI than in SINGLE (and FREE) in both hemispheres (Fig. ).
We now aim to provide an explanation on why MULTI is more efficient than SINGLE at reducing the error in the sea ice concentration, in particular, over regions of thick ice. Massonnet et al. (Citation2015) showed that in such places usually one or a few categories covary most with the aggregate sea ice concentration while all other categories exhibit no relationship. This feature is also seen in our ensemble (not shown). In SINGLE, the increments of aggregated concentration are redistributed proportionally to the mean concentration of the individual category. This may lead to a wrong distribution of the increments because the error is not necessarily largest for the category with large ice concentration. As an example, if the ice concentration of a category is very large and similar through all members, the covariance between this category and the aggregated concentration would be null, but SINGLE will still give it a larger share of the increments. In MULTI, the updates would occur only for the categories that covary with the aggregated ice concentration. As a consequence, we expect SINGLE to perform poorly in locations where ice is distributed over several categories, which typically corresponds to regions of thicker ice.
Another explanation for why MULTI outperforms SINGLE, is the increased number of degrees of freedom in the minimisation process. As a consequence, the impact of the assimilation is suboptimal in SINGLE if two categories are anti-correlated. However, increasing the degrees of freedom while keeping the ensemble size constant will proportionally increase the sampling error. This combined with the fact that the relation between the observed variable and the state vector is often non-linearly related can lead to a degradation of the performance of the system over time. We recognise such a degradation in the bias of the ice thickness and in the ice volume in the thickest category in the Arctic (see Fig. c,e), drifting away from the bias of FREE in the last two years of model integration. While these biases are not necessarily problematic for systems that operate for a short (subseasonal to annual) period of time or for regions of first-year ice, where the ice fully melts during the summer, it is a serious concern for long reanalyses because the performance of the system will gradually get poorer with time. The Arctic drift is found to be introduced during the postprocessing of the unphysical values returned by the EnKF.
Ice concentration and volume in each category have a bimodal distribution with largest density near the lower and the upper bounds. When assimilating such a variable with the EnKF, the linear analysis update will make the distribution more Gaussian and return unphysical values (similar to Fig. ). In order to be able to restart the model, these values must be postprocessed (e.g. truncated), which will introduce a bias (Wang et al., Citation2016). The truncation of ice concentration – so that it falls within [0–100]% – and of the ice volume in a given category – so that the corresponding thickness falls within the bounds of the thickness category (see Table ) – are the two main candidates to explain this drift in the Arctic. The drift is mostly visible in the bias of the thickest ice category, because in that category the distribution is more exponential with a high density near the lower bound that leads to an asymmetrical postprocessing. The drift is stronger for MULTI than for SINGLE because the number of assimilated variables are increased and so is the risk of returning unphysical values. In the Antarctic, we do not recognise this drift in the bias as most of the ice is distributed rather in the thinner ice (as indicated also in Fig. b) and thus, the distribution is less strongly non-Gaussian. Other approaches for constraining the unphysical values of thickness have been proposed (see for example, Massonnet et al., Citation2015, or anamorphosis Barth et al., Citation2015) but they have not been tested here as they also have drawbacks and do not preserve the mean either.
4.3. Preserving ice thicknesses
In order to identify the origin of the (Arctic) drift and to counteract it, we have run an additional experiment (hereafter referred to as HI_PRESERVE, see also Table ), which is comparable to MULTI but for which the category ice volume vice is no longer added to the state vector. In each category, vice
is diagnosed from the update of the category ice concentration (aice
), so that the forecast ice thicknesses in all categories are preserved. HI_PRESERVE mitigates the drift in the bias and is now indiscernible from FREE during the last two years (Fig. c,e). The reduction of the bias leads to a reduction of the rmse of the same amplitude. This suggests that the drift observed in MULTI is mainly the consequence of the ice volume postprocessing and not of the ice concentration postprocessing (which are the same in MULTI and in HI_PRESERVE). More surprisingly, it is found that HI_PRESERVE achieves similar performance as MULTI for all variables (see Fig. ) and even for the overall ice thickness hi, which is a mean of the category thicknesses (which remain unchanged during the assimilation steps) weighted by concentration area of the different category. Thus the benefits found in hi and in the ice volumes in MULTI are a direct consequence of the improved category ice concentrations aice
and not from the updates of the vice
. This merely implies that adding the category volume does not add skill but causes a drift in the performance of the system. It would be interesting to study whether adding vice
to the assimilation state vector could add skill if a higher frequency of assimilation or a larger ensemble size would be used. It would also be interesting to assess whether we could further improve the impact of assimilation by increasing the number of categories, but this is beyond the scope of our paper.
It should be mentioned that another experiment has been attempted where the temperature of the ice/snow at the top surface was added to the state vector. However, it is not presented as the results were merely insensitive. We have not tested the sensitivity of adding vsno to the state vector. In Section 6, we will consider HI_PRESERVE as our optimal choice for the sea ice state vector.
5. Importance of ocean – sea ice cross-covariances
The climate system includes complex, coupled phenomena over wide, separated, spatial and temporal scales (atmosphere, ocean, land, cryosphere). On the contrary, DA procedures are mostly designed to deal with a single dominant scale of motion and have thus been yet mostly applied separately to the component of the Earth system (weakly coupled DA). It is expected that DA would allow for more optimal and dynamically more consistent updates if observations were assimilated across model components (strongly coupled DA) (Laloyaux et al., Citation2016; Penny and Hamill, Citation2017). The coupling between the ocean and the sea ice is predominantly thermodynamic; exemplified by the strong negative correlation between aice and SST. The cross-covariances between aice and SSS are more complex and change sign depending on the mechanisms that drive the variability of sea ice in the Arctic (Lisæter et al., Citation2003). Updating the ocean temperature without adapting the salinity may destratify the ocean and can have severe implications on the overturning circulation. In the Southern Ocean, sea ice strongly impacts the stratification of the upper Southern Ocean in the melting season and as well contributes to deep convection processes (Wong and Riser, Citation2011; Abernathey et al., Citation2016; Haumann et al., Citation2016). Here, we focus our analysis on the importance of adding the variables relevant for the thermodynamic – i.e. temperature and salinity – to the assimilation state vector and do not explore the role of the dynamical variables such as the ocean surface currents.
The experiments considered in this section are summarised in part 2 of Table . Note that, we do not use the ‘optimal’ choice (i.e. HI_PRESERVE) for the ice state, but still one that performs well. The reason is that the conclusions of Section 4 were unknown at the time these experiments were carried out. Also, the differences between HI_PRESERVE and MULTI are small, and we argue that applying HI_PRESERVE instead would not change the conclusions of this section. Some key experiments were repeated with HI_PRESERVE and yield very similar results, but we decided to use MULTI (or here STRONG) for the sake of consistency with the remaining experiments in this section.
We start with the comparison of the first three cases: In the first experiment (WEAK), only sea ice is assimilated by adding aice and vice
of each category to the assimilation state vector and a postprocessing, as described in Table , is applied after the assimilation. In the second experiment (referred to as STRONG), the assimilation updates not only the sea ice (as in WEAK) but also, globally, the ocean state – here, temperature and salinity of the mixed layer (ML). Note that the latter case is identical to MULTI examined in Section 4. In the third experiment (PRESCRIBED), we diagnose the ocean temperature of the mixed layer based on the (assimilated) sea ice state – see Table (Section 4) and Table (Appendix 1) for a detailed description. This run is performed in order to highlight the importance of the flow-dependent covariance in formulating the covariance. The temperature in the ML is set to the freezing temperature (i.e.
C in NorESM) when there is non-zero ice concentration; elsewhere the temperature is ensured to be larger than freezing temperature. The salinity is kept unchanged in this experiment during the assimilation (similar to WEAK) as there is no clear (simple) climatological relation between SSS and ice concentration.
Figure 5. Differences in of aice between WEAK and FREE in the Arctic (a) and in the Antarctic (b) with maximum
of 35%, and between STRONG and WEAK in the Arctic (c) and in the Antarctic (d) with maximum
of 14%.
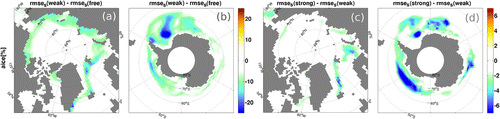
Figure 6. Differences in between WEAK and FREE for SST in the Arctic (a) and in the Antarctic (b), for SSS in the Arctic (c) and in the Antarctic (d). Cool colours indicate an improvement, warm colours a degradation in WEAK compared to FREE. Panels (e,f) and (g,h) depict the differences in
in temperature and salinity in the Arctic and in the Antarctic for different depths between WEAK and FREE with latitudes
.
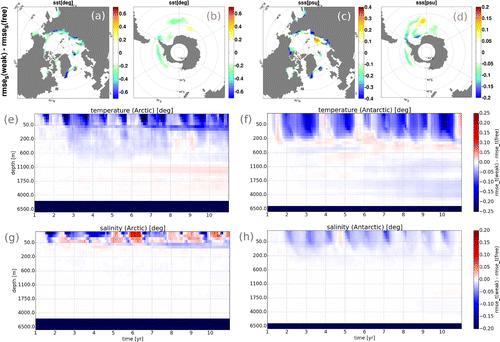
Figure 7. Time and space averaged rmses of the ice component and of the 2D sea surface salinity and temperature for FREE (red), WEAK (yellow) and STRONG (green) in the Arctic (a) and the Antarctic (b).
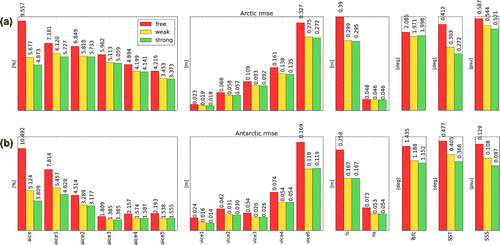
5.1. Weakly coupled assimilation
We start with an examination of the experiment WEAK. The (time averaged) benefits of this run over FREE for of aice and the sea surface variables SST and SSS are presented in Figs. and a–d, respectively. The overall improvements in the (time- and space-averaged) rmses are given in Fig. a,b. For the observed variable aice, WEAK reduces the rmse of FREE by 40% in the Arctic and by 50% in the Southern Ocean. There is no location where WEAK degrades the performance for aice, and the rmse reductions are mostly pronounced in the first-year ice regions (Fig. a,b). Improvements are not only noticeable in the first-year ice regions, but are also found for the ice concentrations and volume of the thicker categories in both hemispheres (Fig.). Thus, the upper ocean adapts to the modified ice state during the assimilation cycle, such that the oceanic feedback to the ice state is preserving the benefits of the assimilation to a large extent. The rmses of the other sea ice variables such as ice thickness, snow thickness and the temperature of ice/snow at the top surface are reduced as well.
The ocean surface hydrography is also mostly improved, see Fig. a,c for the northern hemisphere. These improvements are the consequence of the improved sea ice state that is propagated dynamically during the model integration. In the southern hemisphere (Fig. b,d), the impact for SST and SSS is of weaker amplitude with patches of improvement around 0.2C for SST and around 0.07 psu for SSS in the Weddell Sea and in the Amundsen Sea. However, the reduction of rmse is proportionally of the same order than in the Arctic.
The Hovmöller diagrams in Fig. e–h allow to investigate whether the improvements seen at the surface propagate into the deeper ocean, or whether a bias builds up over time. For temperature, WEAK predominantly reduces the error in the entire ocean interior (see Fig. e,f) with larger improvements in the upper mixed layer (in spatial average about 0.15 C in the Arctic and about 0.1
C in the Southern Ocean with maximal
reductions of 0.4
C and 0.2
C, resp.). Note, that the mixed layer is shallower in the Arctic (about 50 m) than in the Southern Ocean (upper 200 m).
For salinity, the improvements are also mostly confined to the mixed layer. In the Arctic, there is a clear improvement during the melting season while there is a tendency to a degradation during the freezing season that builds up over time (see Fig. g). In the Southern Ocean, the impact is predominantly positive and weaker than in the Arctic (Fig. h); the maximum improvement in the in the Arctic is 0.25 psu (maximum degradation is 0.1 psu). The maximum improvement in the Southern Ocean is 0.08 psu. There is no sign of degradation there. It should be noted that the 10-years analysis is relatively short and the variation in the bias may also relate to the slow internal variability of the system. Thus, we can conclude that constraining only the ice component yields good improvements in the ice and is found beneficial for the ocean temperature in both hemispheres as well as for the salinity budget in the Southern Ocean. There seems to be a (slight) degradation of Arctic salinity – and thus of the system – with time. It was somewhat unexpected that WEAK already leads to such a strong reduction of the errors. It should be noted, that we are focusing here on monthly averaged variability at a relatively coarse resolution of 100 km. It remains to be investigated whether WEAK could perform well for operational oceanography needs that are targeting rapid high-resolution changes of the sea ice front.
Figure 8. Differences in between STRONG and WEAK for SST in the Arctic (a) and in the Antarctic (b), for SSS in the Arctic (c) and in the Antarctic (d). Cold colours indicate an improvement and warm colours a degradation of STRONG compared to WEAK. Panels (e,f) and (g,h) depict the differences in
in temperature and salinity for different depths between STRONG and WEAK for latitudes
in the Arctic and in the Antarctic, respectively.
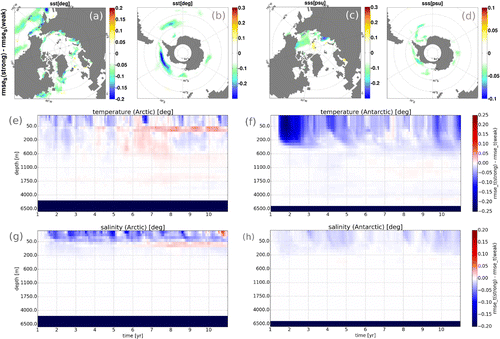
5.2. Strongly coupled flow-dependent assimilation
Updating not only the ice but also the ocean component (in the mixed layer) in STRONG further improves the performance of aice. The improvements are mainly present in regions with predominant first-year ice (see Fig. c,d). In the Arctic, the improvements are mostly noticeable in the Barents Sea, the Greenland Sea and the Bering Sea, in places where the variability of sea ice is largely influenced by the oceanic heat flux (Bitz et al., Citation2005; Woodgate et al., Citation2010; Onarheim et al., Citation2015; Årthun et al., Citation2017). Consistently, the improvements in Arctic SST of STRONG over WEAK mirror those of Arctic aice (compare Figs. c and a). In the Southern Ocean (Fig. b), the improvements are stronger and reach up to 0.35C (temporal average) in the Amundsen Sea.
For the SSS, regions of improvements in the Arctic are found near the river delta in the Kara Sea and in the Beauford Sea where the Pacific water inflow influences the ice front (Fig. c). Though we notice the presence of smaller patches with degradation (up to 0.1 psu), the regions with improvements (reaching 0.1–0.25 psu) clearly dominate (see also the uppermost layer in the Hovmöller plot in Fig. g). Generally, the drift in the SSS bias is reduced in STRONG compared to WEAK, particularly in the Arctic (not shown). In the Southern Ocean, WEAK already performed well and the improvements in SSS are much smaller with patches reaching values up to 0.05 psu (Fig. d).
Accordingly, the benefits over time for temperature and salinity of STRONG in the interior of the ocean are larger than that of WEAK (see Fig. e–h). The benefit is for instance twice as large for the temperature of the Southern Ocean during the first years (compare Figs. f and f). Similarly, the error for salinity is greatly reduced through the entire period (compare Figs. g,h and g,h). The Arctic ocean temperature in STRONG (Fig. e) improves compared to WEAK but shows a slight degradation in 50 m–80 m depth from year four (and to lesser impact for salinity). It will be discussed later in this section. Generally, we can conclude that it is preferable to update both the ice and the ocean state (in the ML) during the assimilation. It does not only lead to an improved temperature and salinity state, but also positively feedbacks to the ice state, particularly in the thin ice. The improvement in the Southern Ocean is deeper than in the Arctic Ocean as the mixed layer is deeper there. We suspect that the benefit of STRONG over WEAK is a combination of an improved thermodynamical consistency of the initial state and a more efficient use of the observation. An explanation for the results obtained is attempted:
During the assimilation in WEAK, when ice is added on top of water that is warmer than the freezing temperature, the model will work against it until enough sea ice is melted to create a capping halocline. Thus, the upper ocean temperatures are reduced towards the freezing point. In STRONG, the cooling and salinity adjustment will be handled by the assimilation and would allow for a faster synchronisation of the ocean with the sea ice state. When ice is removed in WEAK, SST is still at the freezing temperature in these places. The model will respond with more sea ice production. During the sea ice production, the equivalent in latent energy is released to the atmosphere; so, effectively it introduces a heat source which should help to push the model state closer to TRUTH. The sea ice production will lead to an increase of the SSS.
The Southern Ocean is mostly temperature stratified and the variability of salinity is mostly driven by the freezing and melting processes. While STRONG is still expected to be more efficient than WEAK, the dynamical adjustment after the assimilation for salinity will have the expected sign. On the contrary in the Arctic both, temperature and salinity, influence the stratification and the thermohaline circulation can lead to a reversal of the correlation between sea ice and salinity. It was therefore expected that STRONG greatly improves the performance for salinity there.
5.3. Strongly coupled assimilation using prescribed covariances
In STRONG, we adjusted the ocean states in a flow-dependent manner by taking advantage of the ensemble cross-covariances. Though this approach provides satisfactory results, running a large ensemble can be prohibitively expensive with a high-resolution system. If one could succeed in prescribing the covariance between the ocean and sea ice, it would imply that a computationally cheaper DA method could be used for assimilating sea ice concentration. In our experiment PRESCRIBED, we attempt to prescribe the ocean mixed layer based on the sea ice state by setting the temperature of the mixed layer to freezing temperature (i.e. ) where there is ice (aice
). Note that we still rely on flow-dependent DA for the sea ice variables. The performance of the system is very poor. The error in aice is much larger than the observation error (not shown), at times also being larger than FREE. We also see strong degradations in the ocean surface fields SST and SSS, mainly in first-year ice regions (see Fig. a,b for SST).
The error in the ocean interior is even more disastrous. The spurious behaviour at the surface is rapidly propagating downwards. The very strong criterion used (setting SST C if aice
) delays the melting and provokes spurious growth of thin ice, particularly in warmer seasons. We have tested different thresholds for aice from which the temperature should be set to the freezing temperature. The larger the criterion, the milder the degradation got, but the performance remained poorer than WEAK (and STRONG) and that even with a threshold for aice of 80%. Hence, changing temperature without salinity induces a density change in the mixed layer. It is likely that the isopycnal ocean model is particularly sensitive to density changes. Improvements could be achieved by additionally changing the salinity in a static way to balance the buoyancy flux associated with the prescribed cooling. However, such an approach would underestimate the fresh water contribution of the sea ice decline, which is problematic for climate applications. Volpi et al. (Citation2017) also supports the challenges and highlights the difficulty to derive a balanced physical state when two variables (temperature and salinity) are non-linearly related to each other (through density). We do not further elaborate on that in this work. The results are also in good agreement with Lisæter et al. (Citation2003), Sakov et al. (Citation2012), who demonstrated the importance of flow-dependent coupled DA between the ocean and the sea ice for sustaining the ocean stratification.
Figure 11. Panel (a) and (b) depict the time and space averaged rmses of the ice component and of the 2D SST and SSS for FREE (red), the optimal run in the first decade (green) and the optimal run in the second decade (light green) in the Arctic and in the Antarctic. Panels (c) and (d) show differences in for aice between the optimal run and the free run averaged over the first 10 years; panel (e) shows the differences in
for SSS and panel (f) in
for the first 10 years.
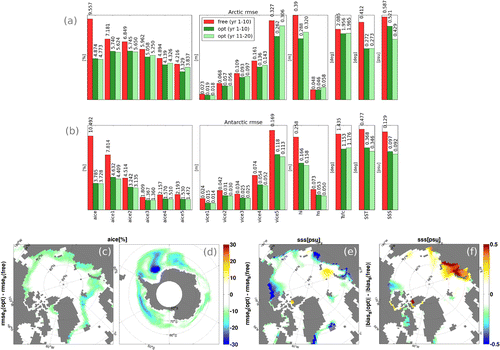
5.4. Strongly coupled assimilation beyond the mixed layer
Most of the co-variability between the ocean and the sea ice is driven by the heat and salt exchange and is confined to the mixed layer. One may expect a deeper signature near the ice front where the isopycnals can plunge below the surface. For this purpose and in order to avoid introducing a vertical discontinuity in the updates, Lisæter et al. (Citation2003), Sakov et al. (Citation2012), Massonnet et al. (Citation2015) recommend an update of the full ocean depths. However, our ensemble size (30 members) is small compared to the 100 members used in Sakov et al. (Citation2012), Lisæter et al. (Citation2003). It is unclear whether the degradation caused by the sampling error would not overcome the benefits for such ensemble size. Massonnet et al. (Citation2015) successfully applied the technique with only 25 members, which is encouraging. We have therefore repeated the experiment STRONG with an update of the entire water column. We denote the latter run as DEPTH. For all variables, the time and space averaged rmses of the two experiments (STRONG and DEPTH) show no distinct differences and are thus not presented. Regarding the evolution of the in the ocean interior (see Fig. ), neither DEPTH nor STRONG is clearly superior for the temperature near the surface with alternating phase where one performs better than the other.
For the salinity, DEPTH is initially performing poorer than STRONG in the Arctic, but rapidly becomes better. For both, Arctic temperature and Arctic salinity, there is a notable improvement for DEPTH at about 50–80 m. In MICOM, salt released during freezing of sea ice is distributed evenly below the mixed layer, down to the depth with a density contrast of 0.4 kg m compared to the surface and limited to 500 m. This is done in order to reduce SSS and the biases in the stratification at high latitudes (Nguyen et al., Citation2009). As a consequence, STRONG considers the correlation related to the melting (present in the ML) but not the correlation related to freezing (below the ML) leading to a weak degradation of the salinity over time. We suspect that this impact is not as clear in the Antarctic, because the depth of the ML is not as uniform there as in the Arctic ocean. In the Southern Ocean, for both, temperature and salinity, DEPTH seems to introduce a weak degradation at intermediate depth that is counterbalanced by a weak improvement in the deep. We can conclude that there is no big difference between updating only the ML and updating the entire depth, but the performance in the Arctic for the salinity field is slightly improved in DEPTH (cool colors in Fig. c). We will therefore consider this option as the optimal one for Section 6. It should be noted that in a realistic framework the model may have large biases (e.g. due to the effect of atmospheric circulation, cloud biases and unresolved melt ponds); and model biases in the observed variables would be propagated to the ocean interior by the DA when updating the full water column. Therefore, considering the little benefit DEPTH brings compared to STRONG but knowing the potential risk of using a full depth update with a biased model, we would recommend updating only the hydrography of the ML adding a few layers below in order to include the correlations related to brine rejection.
Figure 12. Panels (a,b) and (c,d): (bold lines) and
(dotted lines) of vice
and SSS for FREE (red, only first 10 years) and for the optimal setting (green, 20 years) in the Arctic and in the Antarctic, respectively. Panel (e) shows the summary of the global time and space averaged reliabilities of all considered variables for FREE (red), and the optimal setting (green), where the evaluation is splitted into decades.
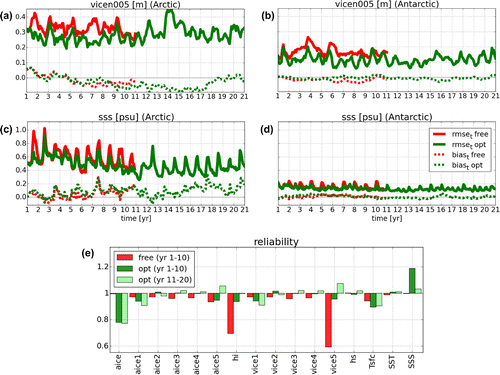
6. Verification of the optimised settings
We combine now the most successful assimilation settings from Sections 4 and 5 into one, denoted as DEPTH HI_PRESERVE (see also the Table in Section 3 and Table in Appendix 1), that consists of the following: First, updating the multicategory sea ice state with preservation of the forecast thickness (as in HI_PRESERVE); second, updating the ocean using the ensemble flow-dependent covariance (as in STRONG) and third, extending the ocean covariance to the entire depth (as in DEPTH). We carried out the reanalysis for 20 years (twice as long as in the experiments detailed in the previous sections) to ensure that no slow degradations are developing. To limit the computational costs, FREE is only run for 10 years as its performance is not expected to vary with preindustrial forcing. In Fig. a,b we compare the Arctic and Antarctic rmses of the optimised run restricted to the first 10 years (green bars) with that of FREE (red bars). Beginning with an analysis of the first 10 years, we notice that in an idealised perfect model framework the assimilation of ice concentration allows for a substantial reduction of the error in our system: the rmse in the observed variable (aice) is reduced by in the Arctic and by
in the Southern Ocean, the ice concentrations in each thickness category have been improved as well with a (in the Arctic slightly) larger reduction in the thinner ice categories. Likewise, the errors in all the remaining variables decreased, e.g. hi by 28
% (resp. 36%), SST by 34
% (resp. 23%) and SSS by 13
% in the Arctic (resp. 25
% in the Southern Ocean). For the observed variable aice, the spatial distribution of the
reduction compared to FREE (calculated over the first 10 years) is shown in Fig. c,d. In the regions of first-year ice, the mean improvement is about 15% and we reach gains up to 40
% in the Weddell Sea. The rmse reduction of the hi mirrors that of aice with mean improvements in the first-year ice areas of 0.2–0.4
m and values of up to 0.7 m on the south east coast of Greenland and in the Weddell Sea (not shown). The largest error reductions of the ocean surface hydrography (SST and SSS) is found at the ice edge. While the improvements for SST are about 0.7
C along the entire Siberian coast (not shown), the rmse in SSS mainly improves in the Beaufort Sea, in the Laptev Sea and along the southern coast of Greenland with improvements up to 0.87 psu South of Greenland (see Fig. e). We notice that there is a degradation in the bias of salinity in the Kara Sea near the exit of the Ob and Yenisei rivers. It should be mentioned that the increased bias is still smaller than the reduction of the rmse and that the peak of the increasing trend is in year four for the Kara Sea (not shown). The decreasing trend can also be seen in Fig. a (comparing the dark and light green bars for SSS). This region is quite particular: The Kara Sea is a semi-enclosed basin that is shielded from the strong winds by the high mountains of Novaya Zemlya and the warm oceanic inflow is comparatively small – the inflow through the Kara Gate is rather weak. The sea ice extent is primarily driven by the thermodynamic exchange of the atmosphere (Polyakov et al., Citation2003). As a consequence, we do not expect the coupling between the ocean and the sea ice to play an important role in that region. Additionally, the salinity variability is largely influenced by the very strong river plumes and range from a few psu to 34.5 psu. Spurious correlations from the sampling error can as such lead to large salinity corrections there. The river run-off is also connected to the large-scale atmospheric circulation. We expect that adding assimilation to the atmosphere component would further improve the performance in that region.
In order to assess whether the performance of the system is degrading over time, we plotted the rsmes of the second decade along with those of the first decade (Fig. a,b). We notice that the rmses in the second decade (year 11–20) show very similar performance to those of the first decade (year 1–10), except for the variables vice and concomitantly for hi, for which the performance is slightly poorer in the Arctic, and SSS, for which it is slightly improved (particularly in the Arctic). The time series of the performance for these two variables are presented in Fig. a–d. Despite the fact that the
of the Arctic SSS is lower in the second decade than in the first one, the bias of the Arctic SSS is increasingly positive over time. In contrast, the performance for the SSS in the Southern Ocean is stable (Fig. d). The
of the Arctic vice
slightly increases in the beginning of the second decade, but seems to recover to a stable, lower value. Similarly, the
of vice
shows a decreasing trend towards the end of the similation. In the Southern Ocean, we again observe a stable performance with smaller errrors.
In order to assess the trustworthiness of our results, we look at the reliability of our system – a measure for the property of an ensemble to estimate its accuracy (Rodwell et al., Citation2016) – and its evolution over time (Fig. e). For a definition of the term ‘reliability’, recall the definition in Equation (Equation7(7)
(7) ). We notice that, for the optimal run, the reliability of aice is smaller than 1 and smaller than FREE, indicating that our model spread is larger than our rmse. This is consistent with the fact that we have by construction overestimated the observation error during the assimilation (we consider perfect observations while having a 10% error). However, the ratio is relatively close to 1 and it is stable over time. The reliability of the other variables is very good, usually very close to 1 or in similar agreement with FREE (with the exception of SSS in the first decade, which we addressed further above). The reliability suggests that the ensemble can be used to quantify the uncertainty of the system (at least in an OSSE framework) and that there is no collapse of the ensemble spread with time.
To summarise, much of the variability of the sea ice and the ocean surface variables in the polar regions can be constrained by assimilating aggregated ice concentrations with an appropriate implementation. The performance of the system seems robust over time, with a hint of a degradation for the bias of SSS and in the thick ice variables. The system is also found to be reliable.
7. Discussions and conclusions
In this study, different implementations for the assimilation of sea ice concentration observations in a fully-coupled Earth prediction system (NorCPM) have been tested with idealised perfect twin experiments (OSSE experiments with preindustrial forcing). We aimed at identifying the implementation that can maximise the error reduction and minimise the drift in the performance of the system. In our assessment of the assimilation techniques we focussed on the treatment of ocean and multicategory sea ice state updates, leaving the atmosphere component unchanged. Monthly accumulated sea ice concentration has been assimilated with the EnKF into the NorESM and the performance has been tested for a 10-year reanalysis. We found: (1) it is best to update the multicategory ice concentration and to diagnose the volume so that it preserves the forecast ice thickness of each category; (2) it is best to carry out a joint assimilation of the ocean (full depth) and a sea ice component with flow-dependent covariance. The performance of the system is relatively stable and reliable when tested for a 20-year reanalysis.
Towards the development of seamless prediction of the Earth system – aiming at prediction from weather up to a decade – (Brunet et al., Citation2015; Hoskins, Citation2013; Palmer et al., Citation2008) there is a need to revisit the DA formalism and the way cross-component covariances are formulated. An approach referred to as strongly coupled DA aims at solving the analysis of the Earth system as the whole in order to enhance the optimality of the assimilation and the dynamical consistency of the system (Laloyaux et al., Citation2016; Penny and Hamill, Citation2017). The proposed study paves the way to such systems by testing extensively coupled ocean–sea ice DA in a fully-coupled Earth system model. We demonstrated the applicability of methods proposed in operational oceanography (Lisæter et al., Citation2003; Sakov et al., Citation2012; Mathiot et al., Citation2012; Barth et al., Citation2015; Massonnet et al., Citation2015) to Earth system models for which the atmosphere can freely evolve and no salinity restoring to climatology is used. In good agreement with Lisæter et al. (Citation2003), Sakov et al. (Citation2012), flow-dependent DA methods are found to skilfully span the cross-covariance between the ocean and the sea ice, which does not seem achievable with prescribed covariances. A weakly coupled DA approach (only the ice state is updated) shows already reasonable improvements, also for the ocean (predominantly in the upper 200 m for temperature and salinity in the Southern Ocean and in the upper 50 m for temperature in the Arctic). However, strongly coupled DA further enhances the efficiency of the assimilation and improves the performance for salinity in the Arctic.
Assimilating the full multicategory sea ice state as proposed in Massonnet et al. (Citation2015) is found to greatly improve the performance for the sea ice state and also of the ocean surface, but it also introduces a bias in the volume of the thick ice categories. Ensuring that the assimilation preserves the sea ice thickness of the forecasts in each category can mitigate this drift without degrading the performance of the system. Our results suggest that the skill in total ice thickness relates to the improvement in the multicategory ice concentration. We did not investigate how changing the number of categories would impact the efficiency of the assimilation. Adding ice volumes to the state vector did not have a beneficial effect. We also found that the drift in thickness was caused by the postprocessing of the ice volume rather than by the postprocessing of the ice concentration. This emphasises the need to wisely choose the assimilation state vector in order to prevent possible drifts in the bias caused by the postprocessing of unphysical values. Note, that in our experiments regarding the ice states, we used the strongly coupled approach in order to avoid suboptimal feedback from the ocean state.
The current study is solely based on the assimilation of ice concentration for the purpose of reanalysis and is carried out in an idealised perfect twin experiment framework (or OSSE). While the results indicate that the method proposed is capable of dynamically constraining parts of the ocean and the sea ice variability of the climate system in the poles, there is need for further investigation before the result can be directly used in production runs:
While perfect twin experiments allow a careful analysis of the impact of assimilation, it assumes erroneously that the model is unbiased. The optimal setting must be tested in a non-perfect framework, e.g. using fraternal twin experiments where the observations are constructed from an independent model, or with real data relying on independent observations for validation.
The performance of the different assimilation schemes are evaluated based on their performance during the reanalysis and it remains to be investigated how long the benefit from the assimilation persists in prediction runs. However, our assimilation cycle of one month is relatively long. Our results thus indicate that the performance of the assimilation is not rapidly declining after the assimilation. In addition, the assimilation demonstrated skill for ice thickness that previous studies found to provide the strongest predictive skill.
We have quantified the reduction of the error that can potentially be achieved with ice concentration only. However, there are many other variables observed. For instance, sea ice variability has been successfully constrained by only making use of ocean and atmospheric observations (Bushuk et al., Citation2017). There is a need to quantify the additional value of assimilating ice concentration when a more complete observation network is assimilated.
Recently other sources of observations have become available in the polar region: ice tethered profiles (Toole et al., Citation2011), acoustic tomography (Sagen et al., Citation2016), ice drift from buoys or derived from SAR images (Sumata et al., Citation2014), and ice thickness available from ICESat CryoSat–2 or SMOS product (Kwok and Rothrock, Citation2009; Laxon et al., Citation2013; Kaleschke et al., Citation2012). While they would, in a first stage, serve as independent source of validation for our realistic framework, we aim at developing capability to ingest them in our system. The same procedure as the one used here will be repeated.
In our study, we solely focussed on the ice and the ocean compartment, however, other components of the Earth system model are influenced by changes in the ice and in the ocean. This should be examined for finer resolutions in order to account for the different scales in the atmosphere compared to the ocean and the sea ice.
Acknowledgements
The authors thank the anonymous reviewers for their helpful remarks on this manuscript.
Additional information
Funding
Notes
No potential conflict of interest was reported by the authors.
1 For relatively large sample size it is acceptable to use the biased estimator of the variance (divide by instead of
). Here this may have caused discrepancies of about 2% for spread
used in the calculation of the reliability.
References
- Abernathey, R. P., Cerovecki, I., Holland, P. R., Newsom, E., Mazloff, M. and co-authors. 2016. Water-mass transformation by sea ice in the upper branch of the Southern Ocean overturning. Nat. Geosci. 9, 596–601.
- Årthun, M., Eldevik, T., Viste, E., Drange, H., Furevik, T. and co-authors. 2017. Skillful prediction of northern climate provided by the ocean. Nat. Commun. 8, 15875. DOI: 10.1038/ncomms15875.
- Axell, L. and Liu, Y. 2016. Application of 3-D ensemble variational data assimilation to a Baltic Sea reanalysis 1989--2013. Tellus A: Dyn. Meteorol. Oceanogr. 68, 24220.
- Barth, A., Canter, M., Van Schaeybroeck, B., Vannitsem, S., Massonnet, F. and co-authors. 2015. Assimilation of sea surface temperature, sea ice concentration and sea ice drift in a model of the Southern Ocean. Ocean Model. 93, 22–39.
- Bentsen, M., Bethke, I., Debernard, J. B., Iversen, T., Kirkevåg, A. and co-authors. 2013. The Norwegian Earth System Model, NorESM1-M - Part 1: Description and basic evaluation of the physical climate. Geosci. Model Dev. 6, 687–720.
- Bitz, C. M., Holland, M. M., Hunke, E. C. and Moritz, R. E. 2005. Maintenance of the sea-ice edge. J. Climate 18, 2903–2921.
- Blanchard-Wrigglesworth, E. and Bitz, C. M. 2014. Characteristics of Arctic Sea-Ice Thickness Variability in GCMs. J. Climate 27, 8244–8258.
- Bleck, R., Rooth, C., Hu, D. and Smith, L. T. 1992. Salinity-driven thermocline transients in a wind- and thermohaline-forced isopycnic coordinate model of the North Atlantic. J. Phys. Oceanogr. 22, 1486–1505.
- Briegleb, B. and Light, B. 2007. delta-Eddington Multiple Scattering Parameterization for Solar Radiation in the Sea Ice Component of the Community Climate System Model. NCAR Tech. Note NCAR/TN-472+STR.
- Brunet, G., Jones, S. and Ruti, P. 2015. Seamless Prediction of the Earth System: From Minutes to Months. WMO.
- Bushuk, M., Msadek, R., Winton, M., Vecchi, G. A., Gudgel, R. and co-authors. 2017. Skillful regional prediction of Arctic sea ice on seasonal timescales. Geophys. Res. Lett. 44, 4953–4964.
- Caya, A., Buehner, M. and Carrieres, T. 2010. Analysis and forecasting of sea ice conditions with three-dimensional variational data assimilation and a coupled ice-ocean model. J. Atmos. Ocean. Technol. 27, 353–369.
- Chevallier, M., Smith, G. C., Dupont, F., Lemieux, J.-F., Forget, G. and co-authors. 2016. Intercomparison of the Arctic sea ice cover in global ocean-sea ice reanalyses from the ORA-IP project. Climate Dyn. 49(3), 1107–1136.
- Counillon, F., Bethke, I., Keenlyside, N., Bentsen, M., Bertino, L. and co-authors. 2014. Seasonal-to-decadal predictions with the ensemble Kalman filter and the Norwegian Earth System Model: A twin experiment. Tellus A: Dyn. Meteorol. Oceanogr. 66(1), 21074.
- Counillon, F., Keenlyside, N., Bethke, I., Wang, Y., Billeau, S. and co-authors. 2016. Flow-dependent assimilation of sea surface temperature in isopycnal coordinates with the Norwegian Climate Prediction Model. Tellus A: Dyn. Meteorol. Oceanogr. 68(1), 32437.
- Craig, A. P., Vertenstein, M. and Jacob, R. L. 2011. A New flexible coupler for earth system modeling developed for CCSM4 and CESM1. Int. J. High Perform. Comput. Appl. 26(1), 31–42.
- Dee, D. P., Uppala, S. M., Simmons, A. J., Berrisford, P., Poli, P. and co-authors. 2011. The ERA-Interim reanalysis: Configuration and performance of the data assimilation system. Q. J. R. Meteorol. Soc. 137, 553–597.
- Deser, C., Toma, R. A. and Sun, L. 2015. The role of Ocean-Atmosphere Coupling in the Zonal-Mean Atmospheric Response to Arctic Sea Ice Loss. J. Climate 28, 2168–2186.
- Einarsson, N., Nilsson, A., Nymand Larsen, J. & Young, O. (2014). Arctic human development report. AHDR (Arctic Human Development Report) 2004. Akureyri: Stefansson Arctic Institute, Springer, Netherlands, pp. 213–214.
- Evensen, G. 2003. The ensemble Kalman filter: Theoretical formulation and practical implementation. Ocean Dyn. 53, 343–367.
- Gao, Y., Sun, J., Li, F., He, S., Sandven, S. and co-authors. 2015. Arctic sea ice and Eurasian climate: A review. Adv. Atmos. Sci. 32, 92–114.
- Gaspari, G. and Cohn, S. E. 1999. Construction of correlation functions in two and three dimensions. Q. J. R. Meteorol. Soc. 125, 723–757.
- Gent, P. R., Danabasoglu, G., Donner, L. J., Holland, M. M., Hunke, E. C. and co-authors. 2011. The community climate system model version 4. J. Climate 24, 4973–4991.
- Guemas, V., Blanchard-Wrigglesworth, E., Chevallier, M., Day, J. J., Déqué, M. and co-authors. 2016. A review on Arctic sea-ice predictability and prediction on seasonal to decadal time-scales. Q. J. R. Meteorol. Soc. 142, 546–561.
- Hamill, T., Whitaker, J. and Snyder, C. 2001. Distance-dependent filtering of background error covariance estimates in an ensemble Kalman filter. Mon. Weather Rev. 129, 2776–2790.
- Haumann, F. A., Gruber, N., Münnich, M., Frenger, I. and Kern, S. 2016. Sea-ice transport driving Southern Ocean salinity and its recent trends. Nature 537, 89–92.
- Holland, M. M., Bailey, D. A., Briegleb, B. P., Light, B. and Hunke, E. 2012. Improved sea ice shortwave radiation physics in CCSM4: The impact of melt ponds and aerosols on Arctic sea ice. J. Climate 25, 1413–1430.
- Holland, M. M., Blanchard-Wrigglesworth, E., Kay, J. and Vavrus, S. 2013. Initial-value predictability of Antarctic sea ice in the community climate system model 3. Geophys. Res. Lett. 40, 2121–2124.
- Holland, P. R., Bruneau, N., Enright, C., Losch, M., Kurtz, N. T. and co-authors. 2014. Modeled trends in Antarctic sea ice thickness. J. Climate 27, 3784–3801.
- Hoskins, B. 2013. The potential for skill across the range of the seamless weather-climate prediction problem: a stimulus for our science. Q. J. R. Meteorol. Soc. 139, 573–584.
- Hunke, E. and Lipscomb, W. 2008. CICE: The Los Alamos Sea Ice Model Documentation and Software User’s Manual. Technical Report, T-3 Fluid Dynamics Group, Los Alamos National Laboratory, Los Alamos, NM 87545.
- Kaleschke, L., Tian-Kunze, X., Maaß, N., Mäkynen, M. and Drusch, M. 2012. Sea ice thickness retrieval from SMOS brightness temperatures during the Arctic freeze-up period. Geophys. Res. Lett. 39(5), L05501.
- Kalnay, E., Kanamitsu, M., Kistler, R., Collins, W., Deaven, D. and co-authors. 1996. The NCEP/NCAR 40-year reanalysis project. Bull. Am. Meteorol. Soc. 77, 437–471.
- Kwok, R. and Rothrock, D. A. 2009. Decline in Arctic sea ice thickness from submarine and ICESat records: 1958–2008. Geophys. Res. Lett. 36(15), L15501.
- Laloyaux, P., Balmaseda, M., Dee, D., Mogensen, K. and Janssen, P. 2016. A coupled data assimilation system for climate reanalysis. Q. J. R. Meteorol. Soc. 142, 65–78.
- Lawrence, D. M., Oleson, K. W., Flanner, M. G., Thornton, P. E., Swenson, S. C. and co-authors. 2011. Parameterization improvements and functional and structural advances in Version 4 of the community land model. J. Adv. Model. Earth Syst. 3(1), M03001.
- Laxon, S. W., Giles, K. A., Ridout, A. L., Wingham, D. J., Willatt, R. and co-authors. 2013. CryoSat-2 estimates of Arctic sea ice thickness and volume. Geophys. Res. Lett. 40, 732–737.
- Lisæter, K. A., Rosanova, J. and Evensen, G. 2003. Assimilation of ice concentration in a coupled ice-ocean model, using the Ensemble Kalman filter. Ocean Dyn. 53, 368–388.
- Liu, M. and Kronbak, J. 2010. The potential economic viability of using the Northern Sea Route (NSR) as an alternative route between Asia and Europe. J. Transport Geogr. 18, 434–444.
- Massom, R. A. and Stammerjohn, S. E. 2010. Antarctic sea ice change and variability - Physical and ecological implications. Polar Sci. 4(2), 149–186.
- Massonnet, F., Fichefet, T. and Goosse, H. 2015. Prospects for improved seasonal Arctic sea ice predictions from multivariate data assimilation. Ocean Model. 88, 16–25.
- Massonnet, F., Goosse, H., Fichefet, T. and Counillon, F. 2014. Calibration of sea ice dynamic parameters in an ocean-sea ice model using an ensemble Kalman filter. J. Geophys. Res. Oceans 119, 4168–4184.
- Massonnet, F., Mathiot, P., Fichefet, T., Goosse, H., König, C. and co-authors. 2013. A model reconstruction of the Antarctic sea ice thickness and volume changes over 1980--2008 using data assimilation. Ocean Model. 64, 67–75.
- Mathiot, P., König Beatty, C., Fichefet, T., Goosse, H., Massonnet, F. and co-authors. 2012. Better constraints on the sea-ice state using global sea-ice data assimilation. Geosci. Model Dev. 5, 1501–1515.
- Mauritzen, C. and Häkkinen, S. 1997. Influence of sea ice on the thermohaline circulation in the Arctic-North Atlantic ocean. Geophys. Res. Lett. 24, 3257–3260.
- McGoodwin, J. R. 2007. Effects of climatic variability on three fishing economies in high-latitude regions: Implications for fisheries policies. Mar. Policy 31(1), 40–55.
- Meehl, G. A., Goddard, L., Murphy, J., Stouffer, R. J., Boer, G. and co-authors. 2009. Decadal prediction. Bull. Am. Meteorol. Soc. 90, 1467–1485.
- Neale, R. B., Richter, J. H., Conley, A. J., Park, S., Lauritzen, P. H. and co-authors. 2010. Description of the NCAR Community Atmosphere Model (CAM 4.0). NCAR Tech. Note NCAR/TN-464+STR.
- Nguyen, A. T., Menemenlis, D. and Kwok, R. 2009. Improved modeling of the Arctic halocline with a subgrid-scale brine rejection parameterization. J. Geophys. Res. Oceans 114(C13),C11014.
- Nguyen, A. T., Menemenlis, D. and Kwok, R. 2011. Arctic ice-ocean simulation with optimized model parameters: approach and assessment. J. Geophys. Res. Oceans 116(C4), C04025.
- Oleson, K. W., Lawrence, D. M., Bonan, G. B., Flanner, M. G., Kluzek, E. and co-authors. 2010. Technical Description of Version 4.0 of the Community Land Model (CLM). Technical Report NCAR/TN-478+STR, National Center for Atmospheric Research, Boulder, Colorado, 622 USA.
- Onarheim, I. H., Eldevik, T., Årthun, M., Ingvaldsen, R. B. and Smedsrud, L. H. 2015. Skillful prediction of Barents Sea ice cover. Geophys. Res. Lett. 42, 5364–5371.
- Palmer, T. N., Doblas-Reyes, F. J., Weisheimer, A. and Rodwell, M. J. 2008. Toward seamless prediction: Calibration of climate change projections using seasonal forecasts. Bull. Am. Meteorol. Soc. 89, 459–470.
- Penny, S. G. and Hamill, T. M. 2017. Coupled data assimilation for Integrated Earth System Analysis and Prediction. Bull. Am. Meteorol. Soc. 98(7), 169–172.
- Polyakov, I. V., Genrikh, V. A., Bekryaev, R. V., Bhatt, U. S., Colony, R. and co-authors. 2003. Long-term ice variability in Arctic marginal seas. J. Climate 16, 2078–2085.
- Rodwell, M. J., Lang, S. T. K., Ingleby, N. B., Bormann, N., Hólm, E. and co-authors. 2016. Reliability in ensemble data assimilation. Q. J. R. Meteorol. Soc. 142, 443–454.
- Sagen, H., Dushaw, B. D., Skarsoulis, E. K., Dumont, D., Dzieciuch, M. A. and co-authors. 2016. Time series of temperature in Fram Strait determined from the 2008--2009 DAMOCLES acoustic tomography measurements and an ocean model. J. Geophys. Res. Oceans 121, 4601–4617.
- Sakov, P. & Bertino, L. (2010). Dependence of the minimal ensemble size in the EnKF on the ensemble spread. In EGU General Assembly Conference Abstracts. Vol. 12, EGU General Assembly Conference Abstracts, Vienna, 828 pp.
- Sakov, P., Counillon, F., Bertino, L., Lisæter, K. A., Oke, P. R. and co-authors. 2012. TOPAZ4: An ocean-sea ice data assimilation system for the North Atlantic and Arctic. Ocean Sci. 8, 633–656.
- Sakov, P. and Oke, P. R. 2008. A deterministic formulation of the ensemble Kalman filter: An alternative to ensemble square root filters. Tellus A: Dyn. Meteorol. Oceanogr. 60, 361–371.
- Schwinger, J., Goris, N., Tjiputra, J., Kriest, I., Bentsen, M. and co-authors. 2016. Evaluation of NorESM-OC (versions 1 and 1.2), the ocean carboncycle stand-alone configuration of the Norwegian Earth System Model (NorESM1). Geosci. Model Dev. 9, 2589–2622.
- Serreze, M. C., Holland, M. M. and Stroeve, J. 2007. Perspectives on the Arctic’s shrinking sea-ice cover. Science 315, 1533–1536.
- Steele, M., Morley, R. and Ermold, W. 2001. PHC: A global Ocean hydrography with a high-quality Arctic ocean. J. Climate 14, 2079–2087.
- Sumata, H., Lavergne, T., Girard-Ardhuin, F., Kimura, N., Tschudi, M. A. and co-authors. 2014. An intercomparison of Arctic ice drift products to deduce uncertainty estimates. J. Geophys. Res. Oceans 119, 4887–4921.
- Tietsche, S., Notz, D., Jungclaus, J. H. and Marotzke, J. 2013. Assimilation of sea-ice concentration in a global climate model - physical and statistical aspects. Ocean Sci. 9, 19–36.
- Timmermann, R., Beckmann, A. and Hellmer, H. H. 2002. Simulation of ice-ocean dynamics in the Weddell sea Part I: Model configuration and validation. J. Geophys. Res. 107(C3), 10-1–10-11, DOI: 10.1029/2000JC000741.
- Toole, J. M., Krishfield, R. A., Timmermans, M.-L. and Proshutinsky, A. 2011. The ice-tethered profiler: Argo of the Arctic. Oceanography 24, 126–135.
- Uotila, P., Iovino, D., Vancoppenolle, M., Lensu, M. and Rousset, C. 2017. Comparing sea ice, hydrography and circulation between NEMO3.6 LIM3 and LIM2. Geosci. Model Dev. 10, 1009–1031.
- Vancoppenolle, M., Fichefet, T., Goosse, H., Bouillon, S., Madec, G. and co-authors. 2009. Simulating the mass balance and salinity of Arctic and Antarctic sea ice. 1. Model description and validation. Ocean Model. 27, 33–53.
- Vertenstein, M., Craig, T., Middleton, A., Feddema, D. and Fischer, C. 2012. CESM. 1.0.3 {U}ser {G}uide. Online at: http://www.cesm.ucar.edu/models/cesm1.0/cesm/cesm\_doc\_1\_0\_4/ug.pdf
- Volpi, D., Guemas, V., Doblas-Reyes, F. J., Hawkins, E. and Nichols, N. K. 2017. Decadal climate prediction with a refined anomaly initialisation approach. Climate Dyn. 48, 1841–1853.
- Wang, Y., Counillon, F. and Bertino, L. 2016. Alleviating the bias induced by the linear analysis update with an isopycnal ocean model. Q. J. R. Meteorol. Soc. 142, 1064–1074.
- Wang, Y., Counillon, F., Bethke, I., Keenlyside, N., Bocquet, M. and co-authors. 2017. Optimising assimilation of hydrographic profiles into isopycnal ocean models with ensemble data assimilation. Ocean Model. 114, 33–44.
- Wong, A. P. S. and Riser, S. C. 2011. Profiling float observations of the upper ocean under sea ice off the wilkes land coast of Antarctica. J. Phys. Oceanogr. 41, 1102–1115.
- Woodgate, R. A., Weingartner, T. and Lindsay, R. 2010. The 2007 Bering Strait oceanic heat flux and anomalous Arctic sea-ice retreat. Geophys. Res. Lett. 37, L01602.
- Xie, J., Counillon, F., Bertino, L., Tian-Kunze, X. and Kaleschke, L. 2016. Benefits of assimilating thin sea ice thickness from SMOS into the TOPAZ system. The Cryosphere 10, 2745–2761.
- Yang, Q., Loza, S. N., Losch, M., Liu, J., Zhang, Z. and co-authors. 2015. Assimilating summer sea-ice concentration into a coupled ice-ocean model using a LSEIK filter. Ann. Glaciol. 56, 38–44.
- Yuan, N., Ding, M., Ludescher, J. and Bunde, A. 2017. Increase of the Antarctic sea ice extent is highly significant only in the Ross sea. Sci. Rep. 7, 41096.
Appendix 1
Postprocessing of sea ice analysis state with CICE 4.0
After each assimilation step, one needs to ensure the physical consistency of the initial condition of the ice and the ocean components. Inconsistency can be introduced during assimilation by for example the linear analysis update of non-Gaussian distributed variables that can return unphysical values or because some variables have not been updated by assimilation and need to be diagnosed from the updated variables. The postprocessing proposed is specially tailored to our particular system (MICOM and CICE 4.0) and a different postprocessing maybe needed with a different ocean or sea ice model. Each of the conditions are verified sequentially from top to bottom as described in Table . Model crashes were found to be very sensitive to the energy budgets available for melting of ice and snow. Neglecting the energy or using an improper treatment easily leads to a crash of the system. The energy profiles of snow and ice in each thickness category are estimated proportionally to the changes of sea ice volume or are estimated as proposed in ice_init.F from CICE 4.0 if new ice is created.
Table A1. List of postprocessings (upper part) and diagnosed variables (lower part, see Table for an explanation of the variables). Variables with index f are forecast values, those with index a are the values after the analysis and variables with index p denote values after the postprocessing. The index pp denotes a second postprocessing of the already postprocessed variable. and
denote the lower and the upper bound of the n-th thickness category (predefined CICE4.0 values).
is the threshold, set to
. ML refers to the ocean mixed layer. SINGLE, HI_PRESERVE, DEPTH HI_PRESERVE and PRESCRIBED are particular experiments differing in the assimilation state vector and the diagnosed variables. The general case applies for all of the considered experiments, see also Table for an overview.