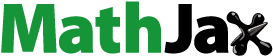
Abstract
The lake model FLake is currently widely used in numerical weather prediction and in climate models to parameterize the effect of freshwater lakes on the state of the boundary atmospheric layer. The model is based on a two-layer parametric representation of the evolving temperature profile (ETP) and on the integral budget of energy for the layers in question. The structure of the stratified layer between the upper mixed layer and the basin bottom is described using the concept of self-similarity of the temperature-depth curve. Capacity of a function of such type to simulate ETP accurately defines the model quality, that is, the extent of correspondence between numerical results and observational data. Several self-similar temperature-depth curves either obtained analytically or resulted from observational data handling, have been used in earlier FLake modifications with different extent of advance. The main shortcoming of parameterizations used previously was their inability to reproduce all types of the ETP known from observations. In the present study, a new parameterization of ETP in frames of FLake, also based on self-similarity of the temperature-depth curve, is proposed. It is demonstrated that a new parameterization is capable to reproduce most of the ETP types observed, whereas the self-similar functions proposed earlier are found to be its particular cases.
1. Introduction
Lakes and reservoirs with spatial extent up to several kilometres, belongs to the underlying surface, which interacts with the atmospheric boundary layer (ABL). Until recently, the effect of this type of reservoirs on the characteristics and structure of the ABL was taken into account in the numerical weather prediction and climate models either in a very simplified way, or ignored at all. The reason is that due to the small size of such water bodies in models with low spatial resolution are the subgrid, i.e. objects whose spatial extent is less than or comparable with the step of the computational grid of the model. However, at present, the use of computational grids with a resolution of 3 or even less kilometres in numerical weather prediction (NWP) has become a common practice, making impossible to ignore the relevant water objects due to their influence on the flow of heat, moisture and momentum in the ABL (Mironov et al., Citation2010). In such a situation, climate and NWP models are to be complemented by appropriate models/parameterizations of thermohydrodynamics of lakes to account for their effect on characteristics and structure of ABL. For this kind of parametrization schemes must meet certain requirements. They must be physically reasonable, adequately calculating the surface temperature and do not require large computational resources.
One of the main features of the mentioned water bodies is a significant horizontal homogeneity of the temperature field inside them and the predominance of the processes of vertical heat transfer over advective. In modelling the thermal regime of water bodies of this class, it is often sufficient to use a simple one-dimensional model based on integrating equations of vertical heat conductivity in one of two types:(1)
(1)
or(2)
(2)
Here, z and t are depth and time, respectively, T – temperature, K – the coefficient of vertical thermal diffusivity, Q is the kinematic (normalized by the density of water and its heat capacity) heat flux.
The solution of each of the equations has its own specifics. The solution of Equation (Equation1(1)
(1) ) requires the parameterization for the calculation of the coefficient of vertical turbulent diffusion, Equation (Equation2
(2)
(2) ) requires a pre-set of vertical distribution of temperature. However, Equation (Equation2
(2)
(2) ) in the numerical realization, in contrast to Equation (Equation1
(1)
(1) ), has a significant advantage. At adequate setting of the temperature profile it can be reduced to ordinary differential equations, making the model built on the basis of this approach, highly efficient in the computational sense, which is an important factor while implementing it into the NWP systems. One of such models was developed to account for the effect of lakes in numerical weather prediction and based on the second approach is a model FLake aimed at simulating the seasonal evolution of the vertical profile of temperature and mixing conditions in a lake (Mironov, Citation2008; www.lakemodel.net).
Currently, the model FLake is widely used by the international meteorological community as a parameterization of the impact of lakes on atmospheric boundary layer (information is available on the official website of the model www.lakemodel.net in the section Applications). In particular, it is used by consortium on mesoscale modelling of atmospheric processes COSMO (COnsortium for Small-scale MOdelling), which includes Germany, Greece, Italy, Poland, Russia, Romania and Switzerland. The model is also embedded into the model HIRLAM of the Finnish Meteorological Institute (FMI), is used at the European Centre for Medium-Range Weather Forecasts (ECMWF) including 22 member states within the Integrated Forecasting System (IFS). FLake also implemented into the UK Met Office Unified Model, into the NWP model suite of Meteo France, into the Weather Research and Forecasting model (WRF), and into the climate models CLM (the CLM Community), RCA (SMHI – Swedish Meteorological and Hydrological Institute), and the Canadian Regional Climate Model (Environment Canada).
FLake is an integral (bulk) fresh water lake model. It is based on a two-layer parametric representation of the evolving temperature profile (ETP) and on the integral budget of energy for the layers in question. The structure of the stratified layer between the upper mixed layer and the basin bottom is described by the concept of self-similarity (assumed shape) of the temperature-depth curve. The same concept is used to describe the temperature structure of the thermally active upper layer of bottom sediments and of the ice and snow cover. An entrainment equation is used to compute the depth of a convectively-mixed layer. A relaxation-type equation is used to compute the wind-mixed layer depth in stable and neutral stratification, where a multi-limit formulation for the equilibrium mixed-layer depth accounts for the effects of the earth’s rotation, of the surface buoyancy flux, and of the static stability in the thermocline. Both mixing regimes are treated with due regard to the volumetric character of solar radiation heating. Simple thermodynamic arguments are invoked to develop the evolution equations for the ice and snow depths. In this way, the problem of solving partial differential equations (in depth and time) for the temperature and turbulence quantities is reduced to solving ordinary differential equations for the time-dependent parameters that specify the ETP thus making the model efficient from a computational point of view and easy for implementation into atmospheric models. In Fig. , a scheme of the vertical distribution of temperature in the system ‘snow – ice – water – bottom sediment’ realized in the model FLake is presented.
Fig. 1. The scheme of the vertical temperature distribution in the system ‘snow – ice – water – bottom sediments’ realized in the model FLake: (a) open water case; (b) ice-covered period.
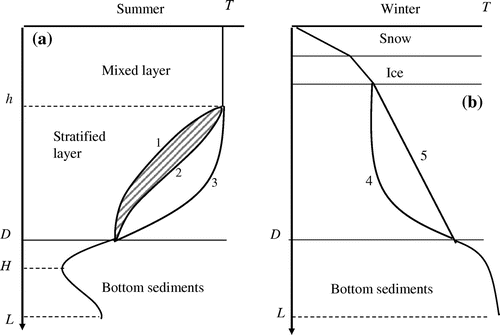
Meaning of the symbols used in Fig. , Equation (Equation3(3)
(3) ) and Table , is as follows.
Table 1. Parameterizations suggested by different authors to describe the vertical distribution of temperature in water basins.
Water column: t is time, z is depth, h(t) is depth of the upper mixed layer; D is the lake depth, TS(t) is water temperature within the upper mixed layer h; TD(t) is the temperature at z = D, is the ‘universal’ function of dimensionless depth ζ = (z – h(t))/(D – h(t)). T*(ζ) satisfies the boundary conditions
.
Bottom sediments: H(t) is the depth, reached by the temperature wave at every instant of time, L = const is the lower boundary of the thermal active layer (TAL) which thickness is (L–D)= const; TH and TL = const are the temperatures at depths z = H and z = L, respectively; and
are dimensionless ‘universal’ functions of dimensionless depths ξ1 = (z–D)/(H–D), ξ2 = (z–H)/(L–H) and satisfy the boundary conditions
; B is time dependent dimensionless parameter (detailed explanation of the meaning of B is given in the section Main results and Discussion).
As noted above, the model is based on the solution of the equation of thermal conductivity in the form (2), assuming a priori the vertical distribution of temperature in the system ‘snow – ice – water – bottom sediment’. The function describing the temperature profile should be considered as ‘universal’, i.e. it should describe the maximum number (preferably all) of the types of profiles observed in natural waters. In this case, such a function is called ‘self-similar’.
Kitaigorodskii and Miropolsky (Citation1970) were the first who suggested to describe the vertical temperature structure of the oceanic active layer by means of self-similar function that can be expressed as a functional dependence between the dimensionless temperature and depth as follows,(3)
(3)
Here, an explanation is necessary – what is the physical meaning of the self-similarity function in formula (Equation3(3)
(3) ). The easiest way to do this would be to use the linear vertical temperature distribution in the water column (Fig. ).
Fig. 2. The scheme of linear temperature profile (Table of symbols in Fig. are the same as those in Fig. ), T(z) is temperature at depth z, A, B, C, D, E are the vertices of a right triangles.
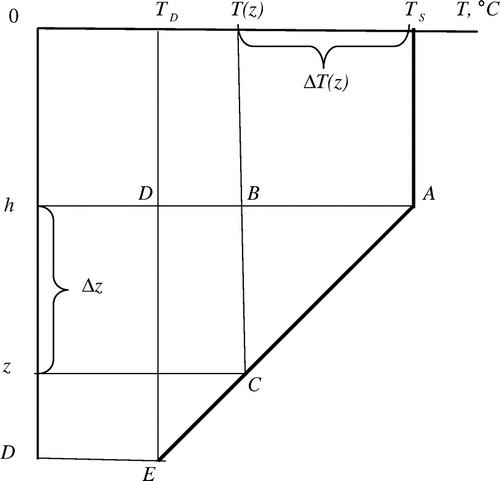
Let us consider two geometrically similar triangles, namely ∆ABC and ∆ADE: in accordance with the properties of similar triangles the following relation between the sides is true:(4)
(4)
In terms of Fig. and Equation (Equation3(3)
(3) ), the expression (Equation4
(4)
(4) ) can be rewritten in the form
(5)
(5)
In accordance with the notation adopted in Equation (Equation3(3)
(3) )
(6)
(6)
Now, taking the limit of the ratio when ∆ζ tends to zero, we obtain
(7)
(7)
Thus, self-similar representation of the vertical temperature profile in the form (3) indicates the constancy in time of the vertical gradient of temperature at any point of the considered profile. If the condition (Equation7(7)
(7) ) is true, the form of the temperature profile does not depend on time and remains constant.
The main parametric representations of the temperature profile suggested by different authors in the form (3) are presented in the Table .
Currently, to calculate the temperature profile in the water column of the lake in consideration within the period of open water in the FLake model the parameterization (10) is used. During the ice covered period for water column the linear parameterization (12) is used. The temperature profile in bottom sediments throughout the year is calculated in accordance with the parameterization (13).
Despite its wide use, the model FLake in its present form has one significant shortcoming. The parameterization describing the vertical temperature profile in a water column in the form currently used in the model, i.e. both formulas (10), is not able to describe the whole range of vertical temperature distributions observed in lakes. In Fig. a, the range of profiles that the model is capable to reproduce are marked by shaded area between the curves 1 and 2. The temperature profiles between curves 2 and 3, 4 and 5, the model is not describing. Meanwhile, these types of vertical temperature distributions are typical for the vast majority of shallow lakes throughout the year (Terzhevik et al., Citation2009).
The purpose of this study is to eliminate this drawback of the model, developing a ‘universal’ parameterization of the vertical temperature profile for the model FLake, which will allow considering the entire range of profiles, including those between curves 1 and 3 in Fig. a and curves 4 and 5 in Fig. b.
2. Site of study, materials and methods
Most of observational data taken into analysis in a present paper, were collected in Lake Vendyurskoe, hereinafter LV. LV (62°10 N, 33°10′E) is a small shallow lake of glacial origin located in Karelia, Russia. The area of the lake surface is 10.4 km2, the average volume is 54.8 × 106 m3, the average residence time is 0.3 yr, and the maximal and mean depths are 13.4 and 5.3 m, respectively. Ice-on occurs from the first days of November to mid-December and ice-off from 1 to 19 May in different years; the duration of the ice season varies from 146 to 192 days with the ice thickness from 0.4 to 0.8 m. In (Terzhevik et al., Citation2009), it was shown that LV is a typical representative of a large class of shallow Karelian lakes of glacial origin (more than 30% of lakes in consideration) with due regard to their thermal regime.
Long-term observations of water temperature profiles (Tw) have been performed in 2007–2008. The data loggers TR-1060 (RBR Ltd, Canada) with accuracy/resolution 0.002/0.00005 °C were autonomously used for registrations one minute apart at a location 11 m deep. The observational set-up was as follows. All instruments were connected at different distances from the lake bottom to a line with a weight at its lower end and a floatable buoy atop. The depths of temperature loggers were 0.05, 0.10, 0.14, 0.16, 0.18, 0.20, 1.81, 2.82, 3.82, 4.79, 5.76, 6.75, 7.74, 8.19, and 8.56 m measured from the bottom. The observations lasted from 4 September 2007 until 26 May 2008, with a break to downloading collected data during 18–20 October. The general scheme of location of measuring equipment in the lake is shown in Fig. .
Methodically, the data processing was the same as it was proposed by Kitaigorodskii and Miropolskiy (Citation1970). Initially, all the measured temperature profiles were daily averaged and analysed in order to detect the thickness of the upper quasi homogeneous (‘mixed’) layer with respect to temperature. Then the data were processed according to representation (3). The dimensionless data was plotted as a functional dependence of temperature on dimensionless depth. Finally, the attempts to approximate the ‘cloud’ of experimental points by polynomial were undertaken. Description of the main results and brief discussion are presented below.
3. Main results and discussion
The data obtained during year-round observations were processed in accordance with (3). Results are presented in dimensionless form in Fig. . The first thing to note is the difference between ‘winter’ and ‘summer’ temperature profiles. At the beginning of the freezing period the shape of temperature profiles corresponds to the curve 4 in Fig. a. Over time they gradually aligned and at the end of the ice season the vertical distribution of temperature in the lake becomes close to linear, indicating that the process of redistribution of heat between the sediments and the water column – the so-called ‘under-ice warming’ – becomes quasi-stationary (Malm et al., Citation1996; Malm et al., Citation1997). Among the parameterizations presented in Table such evolution can be described only by formula (6) including time-dependent parameter B(t) that allows changing the shape of the profile. Summer temperature profiles do not have any regularity in its variability, which has a physical justification. In the open water period, the main processes that form the vertical distribution of temperature in a lake are incoming solar radiation, wind mixing, and density convection. These processes possess a high degree of variability, which leads in turn to the variability of the shape of temperature profiles reflected in the scatter of data in Fig. . In this situation, none of the parameterizations presented in Table is able to cover the whole range of variability of the vertical temperature distributions in the lake because they do not include parameters changing in time (the parameterization (11) is able to describe only the ‘winter’ temperature profiles). Finally, all the data taken together (Fig. ) almost exactly correspond to the area bounded by the curves 1 and 3 in Fig. a. Thus, self-similar representation of the temperature profile in the form (3) proposed Kitaigorodskii and Miropolsky (Citation1970) and modified by other authors, has very limited applicability under natural conditions. It is obvious that in order to formulate the new parametrization describing the maximum possible number of types of vertical temperature distributions, it must contain the time-dependent parameters. To obtain a new parametric representation of the temperature profile in a lake, we will use a procedure similar to that used to obtain the parameterization (11).
Fig. 4. Relationship between different parameterizations. Here empty circles mark ‘winter’ data, black squares stand for data, obtained during the open water period; upper solid line coincides with parameterization (16) at A = 3 and B = 0, the lower solid line coincides a new parameterization (16) at A = 0 and B = 3. Intermediate thin lines correspond to parameterizations (8)–(13).
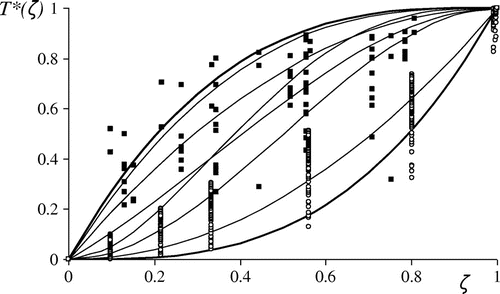
As noted above, the function T*(ζ) satisfies two obvious boundary conditions, namely:(14)
(14)
Let us add to the conditions (14) two additional, determining derivatives of the function T*(ζ) at ζ = 0 and ζ = 1:(15)
(15)
Now, we have four boundary conditions, under which a new function T*(ζ) can be represented as a polynomial of the third degree,(16)
(16)
To determine the physical meaning of parameters A(t) and B(t), we use the expression for the vertical heat flux in the form(17)
(17)
Here, Q is the kinematic vertical heat flux, [K m s−1]; λeff is the ‘effective’ coefficient of heat conductivity, [m2 s−1];
is the vertical gradient of temperature [K m−1].
We rewrite (3) in the form(18)
(18)
After differentiating (18) with respect to z and taking into account boundary conditions (15) Equation (Equation17(17)
(17) ) can be rewritten in the form
(19)
(19)
Now the expressions for A(t) and B(t) are as follows(20)
(20)
Here, Qh and QD are the heat fluxes at z = h and z = D, respectively, λh,eff, λh,eff are the effective temperature conductivity at z = h and z = D, respectively.
Thus, the parameters A(t) and B(t) represent the ratio between the real heat fluxes at z = h and z = D and the heat fluxes corresponding to the linear vertical distribution of temperature, i.e. A(t) and B(t) represent a kind of Nusselt number (Nu).
Introduction of time-dependent parameters A(t) and B(t) in the parameterization (16) allows solving the problem of the variability of the temperature profile shape in time, but generates another problem. Equation (Equation20(20)
(20) ) involve two coefficients of effective temperature conductivity – λd,eff, λh,eff which are associated with turbulent heat transfer. If in respect to λDeff there are the recommendations for its parameterizing in the bottom boundary layer (see, for example, Golosov et al., Citation2007), then the necessity of determining λh,eff brings us back to the solution of the equation of thermal diffusivity in the form (1), which casts doubt on the feasibility of new parameterization (16). The problem of determining the parameters A(t) and B(t) can be solved in the framework of the analysis of the behaviour of the function T*(ζ) within the domain of definition, i.e. from 0 to 1.
T*(ζ) should be monotonic and rising within its definition domain. This requirement to the function T*(ζ) strictly limits the range of variability of the parameters A(t) and B(t). It is easy to demonstrate that the function T*(ζ) in form (11) fills these requirements if values of A(t) and B(t) lie in the range from 0 to 3.
As mentioned above, currently two types of profiles are used in a model FLake in accordance with the parameterization (10). For the transition from one type to another in the model there is a special relaxation procedure (Mironov, Citation2008). The same procedure can be applied for the calculation of intermediate values of the parameters A(t) and B(t). Here, another question arises. Is there a connection between the parameterization in the form (16) and previously proposed ones?
First, as it is shown in Fig. , the curves corresponding to earlier proposed parameterizations are located between two limiting cases described by (16). It means that the representation (16) can cover the maximally possible scatter of field data observed in natural conditions as compared to earlier suggested parameterizations.
Moreover, all the curves corresponding to previously suggested parameterizations can be graphically reproduced from (16) with certain fixed values of parameters A(t) and B(t) (see Table ).
Table 2. Relationship between different parameterizations.
The only exception is the second formula in (10) the curve of which can not be obtained from (16). The explanation is very simple. This formula was not derived analytically from physically grounded boundary conditions but came from processing of experimental data by means of statistical methods, resulted in the polynomial approximation of the forth power.
Another important issue is to be discussed. Parameterization (16) was verified against the observational data from a single lake. The question arises on the applicability of (16) to other water bodies experiencing different natural conditions. Partially the answer to this question is given in the section Materials and methods, where it is said that the LV is a typical representative for 30% of all lakes in the Republic of Karelia. Considering that in Karelia there are more than 73,000 lakes with an area more than 0.1 ha, it is possible to talk about the applicability of the parameterization (16) to tens of thousands of the water bodies. Moreover, the verification of the parameterization (8) has been based on oceanic data obtained at station Papa, located in the Pacific Ocean, the parameterization (10) – on observational data from measurements in the Baltic Sea and lakes of Finland. Given both parameterizations are particular cases of (16) and the fact that these regions clearly do not belong to Karelia, then it is safe to say that the applicability of the parameterization (16) is not limited by a geographical location of water bodies. The limiting factor is rather the spatial water temperature heterogeneity and heat advection by currents, which may disrupt the vertical thermal structure similar to that shown in Fig. .
4. Conclusions
A new parameterization of the ETP in frames of FLake model, also based on self-similarity of the temperature-depth curve, is proposed. It is demonstrated that a new parameterization is capable to reproduce most of the ETP types observed in natural environments. The curves corresponding to earlier proposed parameterizations are located between two limiting cases described by (16). It means that the representation (16) can cover the maximally possible scatter of field data observed as compared to earlier suggested parameterizations. The extent to which a new parameterization is able to improve the quality of the model can be estimated quantitatively after an appropriate change in the model code and its validation against field data obtained in lakes at different latitudes and external parameters. As a preliminary estimate, we can expect the essential improvement in simulating the thermal regime of lakes during the ice season. In particular, it might be crucial in calculating the dates of ice-on and ice-off important for assessing the effect of lakes on the parameters and structure of the atmospheric boundary layer.
Disclosure statement
No potential conflict of interest was reported by the authors.
Funding
This study was supported by the Russian Academy of Sciences (collection of data) and the Russian Scientific Fund [project no. 14-37-00053].
Acknowledgements
The authors are grateful to Andrei Mitrokhov, Nikolai Palshin, Galina Zdorovennova, and Roman Zdorovennov (NWPI) for their efforts in collecting and pre-processing data.
References
- Arsenyev, S. and Felzenbaum, A. I. 1977. Integral model of the active ocean layer. Izv. Akad. Nauk SSSR. Fizika Atmosfery i Okeana 13, 1034–1043 (in Russ).
- Golosov, S. and Kirillin, G. 2010. A parameterized model of heat storage by lake sediments. Environ. Model. Softw. 25, 793–801.10.1016/j.envsoft.2010.01.002
- Golosov, S., Zverev, I., Terzhevik, A., 1998. Modelling thermal structure and heat interaction between a water column and bottom sediments. Report No. 3220 [LUTVDG/(TVVR-3220) 1-41(1998)], Dept. of Water Resources Engineering, Inst. of Technology, Univ. of Lund, Lund, Sweden, 41 p.
- Golosov, S., Zverev, I., Terzhevik, A. Yu. 2003. Thermal structure and heat exchange in ice-water column-sediment system. In: Proceedings of the 7th Workshop on Physical Processes in Natural Water (ed. A. Terzhevik), Karelian Scientific Centre, Russian Academy of Sciences, Petrozavodsk, Russia, 2003, pp. 144–148.
- Golosov, S., Zverev, I., Terzhevik, A. Yu, Kirillin, G., Engelhardt, C. 2007. On the effective thermal diffusivity in ice covered lakes. In: Proceedings of the 11th Workshop on Physical Processes in Natural Water (eds. L. Umlauf and G Kirillin), 3–6 September 2007, Warnemunde, Germany, pp. 157–167.
- Kitaigorodskii, S. A. and Miropolsky, Yu Z. 1970. On the theory of the open ocean active layer. Izv. Akad. Nauk SSSR. Fizika Atmosfery i Okeana 6, 178–188.
- Malkki, P. and Tamsalu, R. E. 1985. Physical features of the Baltic sea. Finn Mar Res. 252, Helsinki, 110 p.
- Malm, J., Terzhevik, A., Bengtsson, L., Boyarinov, P., Glinsky, A., and co-authors. 1996. A field study of thermo- and hydrodynamics in three small Karelian lakes during winter 1994/1995. Rep. № 3197, Lund Univ., Sweden, 216 p.
- Malm, J., Terzhevik, A., Bengtsson, L., Boyarinov, P., Glinsky, A. and co-authors. 1997. Temperature and hydrodynamics in Lake Vendyurskoe during winter 1995/1996. Rep. № 3213, Lund Univ., Sweden, 203 p.
- Mironov, D. 2008. Parameterization of lakes in numerical weather prediction. Deutsher Wetter Dienst, Technical Report № 11, 44 p.
- Mironov, D., Heise, E., Kourzeneva, E., Ritter, B., Schneider, N. and co-authors. 2010. Implementation of the lake parameterisation scheme FLake into the numerical weather prediction model COSMO. Boreal Env. Res. 15, 218–230.
- Terzhevik, A., Golosov, S., Palshin, N., Mitrokhov, A., Zdorovennov, R. and co-authors. 2009. Some features of the thermal and dissolved oxygen structure in boreal, shallow ice-covered Lake Vendyurskoe, Russia. Aquat. Ecol. 43, 617–627.10.1007/s10452-009-9288-x