The commentary by Billieux and Fournier (Citation2022) is an excellent example of how constructive open dialogue can help a field to progress rapidly. In response to our pre-registered confirmatory findings that supported ontological diversity of the ‘gaming disorder’ construct(s) by prevalence and group overlap (Karhulahti, Vahlo et al. Citation2022), Billieux and Fournier approached the same research question and data using network analysis. As a methodological benefit, network analysis allows for scrutinizing construct relationships on an item-level: every item of each operationalized construct can be assessed based on their association with other items across constructs (e.g. Borsboom et al. Citation2021).
As items that cluster together can be considered to form useful construct lines, Billieux and Fournier (Citation2022) simulated two models that illustrate different possibilities of items representing constructs: without notable overlap (their Figure 1a) and with notable overlap (their Figure 1b). By reusing the data of our study, Billieux and Fournier were able to show that a network model of the four ‘gaming disorder’ scales (their Figure 2) fits the overlap type better than the non-overlap type. It is a justified interpretation of these results that the four scales (and the constructs they represent) significantly overlap, ‘therefore measuring substantially homogeneous constructs after all’ (Billieux and Fournier Citation2022, abstract).
Figure 1. Visualization of the networks. A = Original data, ggmModSelect estimator, tuning = 0; B = Original data, EBICglasso estimator, tuning = .25; C = Dichotomized data, IsingFit estimator, tuning =.25; D = Non-paranormal transformation, ggmModSelect estimator, tuning = 0; E = Non-paranormal transformation, EBICglasso estimator, tuning =.25.
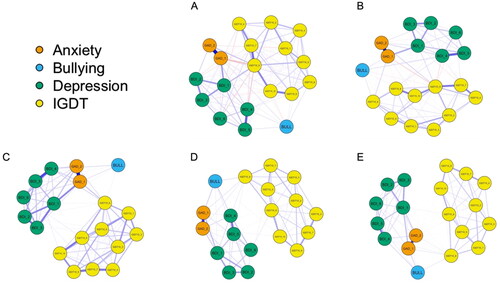
As Billieux and Fournier correctly point out, the above presented findings should lead us to collectively better define or further elaborate on what ‘ontological diversity’ is or could be. We completely agree with this conclusion as well as their related suggestion that item-level construct overlap is theoretically explainable due to historical similarities (and differences) in gaming-related measurement development. In order to take this dialogue one more step further, we also carried out network analyses using the same dataset. As the dataset also included previously unreported measures for non-gaming constructs, our goal was to test whether network overlap might similarly occur with other constructs that are historically distinct. To do this, we chose two highly established mental disorder constructs—anxiety and depression—with one of the original gaming measures:
Anxiety: General Anxiety Disorder 2 (Finnish validation by Kujanpää et al. Citation2014)
Depression: Beck Depression Inventory 6 (Finnish validation by Aalto et al. Citation2012)
Gaming: Internet Gaming Disorder Test 10 (Finnish validation by Männikkö et al. Citation2019)
For experimental reasons, we additionally included a fourth construct that measures experiences of having been bullied in life (single-item instrument created by us), which is not part of any leading diagnostic manuals, but our recent phenomenological findings indicated to be of importance for gaming-related health problems (Karhulahti, Siutila et al. Citation2022).
Because the first three constructs are independent in the light of psychiatric nosology (despite comorbidity, see below) and they do not share strong conceptual origins (like mutual ‘addiction’ measurement), the network produced by the items of these constructs should, following the same principle, fit the non-overlap model rather than the overlap model. Alas, the results of network analysis generate model(s) that involve notable overlap between constructs (; notice outcome differences between approaches) (Supplement 1). On the one hand, the findings may be considered surprising because the scales and their validated items measure constructs (anxiety, bullying, depression, gaming-related health problems) with widely acknowledged independence that have their own diagnostic and research histories; on the other hand, the areas of correlational and/or causal overlap between anxiety, bullying, depression, and gaming-related health problems are somewhat established (e.g. Kumpulainen Citation2008; Ropovik et al. Citation2022), for which one might also expect significant associations and correlations (even regularized partial correlations) to occur. It is possible that the network approach is not optimal for precise identification of construct differences, at least with the present data in the current methodological state.
To investigate the possible homogeneity of the originally used four gaming scales (Karhulahti, Vahlo et al. Citation2022; Billieux and Fournier Citation2022) from one more perspective, we also carried out confirmatory factor analyses with all the items of each such scale (Supplement 1). A single factor structure was not supported (χ2(209) = 11161.73, p < .001; CFI = 0.85; TLI = 0.84; RMSEA = 0.09, 95% CI [0.09, 0.10]; SRMR = 0.05). Although the four-factor structure (i.e. each gaming scale modeled as a separate factor) yielded a significantly better fit (Δχ2(5) = 688.05, p < .001), the model was still disconfirmed. To exploratively investigate the ideal number of factors, a parallel analysis was run, and the results suggest that the optimal solution has even more (eight) factors than the number of theoretical constructs included in the model. If the latter is correct, the four scales cannot reliably measure all factors, as multiple items are needed for a factor to be identified (e.g. Brown Citation2015; see Supplement 1 for cross-loadings).Footnote1
By combining our original results, those found by Billieux and Fournier, and the present findings, we find the state of art to be several steps ahead from where we started. In the original study, we set out to investigate two dimensions of ontological diversity: ‘What criteria define the disorder?’ and ‘How criteria define the disorder?’ (Karhulahti et al. Citation2022, p. 2). Although our results generally confirmed such diversity in terms of prevalence and group overlap, Billieux and Fournier (Citation2022) convincingly suggested that critical item-level similarities may still be observed. Because our present results imply such item-level similarities to occur also between constructs with established nosological and other differences, a larger question remains: at what point and in what way should a construct be identified with independent ontology or be considered homogenous with related constructs? Keeping in mind that construct overlap can also be a product of the crud factor or method bias (e.g. Orben and Lakens Citation2020), or relate to the indexicality of constructs (e.g. Kendler Citation2017), detailed conceptual analysis, content validity assessment, and real-life consequences should outdo statistical estimations. Essentially, the independence of constructs becomes a pragmatic and political question: what benefits and harms would follow from setting the bar of construct independence high or low? This is a question that will undoubtedly be revisited by decision-makers and researchers in the future.
It is important to remember that diagnostic manuals, which currently tend to serve as the main ontological source for psychopathology research, are not necessarily designed to guide the development of statistical measures. For instance, while the ICD coding system specifically serves as a ‘tool for recording, reporting and grouping conditions and factors that influence health’ (reference guide at https://icd.who.int/en), the DSM-5-TR explicitly states that the ‘primary purpose of DSM-5 is to assist trained clinicians in the diagnosis of mental disorders’ (APA Citation2022, p. 21). Accordingly, when statisticians develop and use different scales based on such standardized systems with varying functionalities and premises, it is arguably unsurprising that different prevalence rates and groups are identified. This is not necessarily a problem, however, if researchers clearly define their constructs of interest and carry out comparisons across constructs with a critical awareness of differences. For instance, as Billieux and Fournier (Citation2022) correctly exemplify, one can ask: is the measured gaming construct built on symptoms such as tolerance and withdrawal, which are no longer part of ‘gaming disorder’ in the ICD-11-based construct and its measures? With such reflexivity, the interpretation of both clinical and epidemiological findings will be improved.
This will not, however, solve the main ontological dilemma. In order to provide a practical example of how new related challenges will keep ensuing, it is currently unclear whether problems related to financial spending during gaming are part of ‘gaming disorder’ even according to the improved criteria outlined in the ICD-11. Although the ICD-11 criteria do not address financial spending at all and related measures do not identify this dimension as part of the construct, there are case studies (e.g. Ra and Das Citation2020) and large-scale clinical analyses (e.g. Karhulahti, Nuutinen et al. Citation2022) that show how people already seek treatment because of their problematic spending of money in videogames. If one day this financial dimension is added to the ontological structure of ‘gaming disorder’, for instance, by means of new criteria in the official diagnostic manuals, it is evident that the updated measures will once again address new populations and thus produce different prevalence rates and group overlap discrepancies. The ontological challenge of ‘gaming disorder’—perhaps unlike those of anxiety and depression—is that the human-technology relationship is rapidly ever-evolving and will remain difficult to domesticate into stiff nosological structures. Footnote2
To conclude, we completely share Billieux and Fournier’s (Citation2022) positive sentiment on the possibilities that endorsing open science practices enables for our research fields. Transparent reporting practices—allowing us to have constructive conversations through different methodological interpretations of data and materials—will remain a major benefit and, in the long run, help the research community to pursue robust, cumulative scientific outcomes.
Author contributions
MA carried out all statistical analyses in conversation and with feedback from VMK and MaMa. VMK wrote the first version of the manuscript. All authors commented/read the manuscript and accepted the final version.
Acknowledgements
This reply was improved in a long constructive dialogue with the commenting authors Billieux and Fournier. We also received helpful feedback from Eiko Fried. We are truly thankful for the opportunity to learn in this process.
Disclosure statement
No potential conflict of interest was reported by the author(s).
Data availability statement
The data and materials used in this study are openly available in OSF: https://osf.io/v4cqd/.
Additional information
Funding
Notes
1 For all reported network and factor analyses, we did not test other measures or combinations thereof. It is possible that other combinations yield results with different implications.
2 During the collaborative review of this reply, Billieux and Fournier correctly noted that technological development also concerns biotechnology and thus affects the ontology of substance use disorders. Currently, the DSM-5-TR (APA Citation2022) recognizes ten separate classes of drugs, which are generally the same ones as in the preceding DSM-IV (APA Citation1994). Over the same three decades, gaming products have gone through numerous industry-wide changes, such as the emergence of massive online games, mobile games, esports games, subscription-based monetization, and (currently) microtransaction-driven design with loot boxes as well as virtual reality applications. We agree that our notion of “rapidly ever-evolving” is a matter of subjective assessment, and only time will show how long such evolution remains as rapid as it has been so far.
References
- Aalto AM, Elovainio M, Kivimäki M, Uutela A, Pirkola S. 2012. The Beck Depression Inventory and General Health Questionnaire as measures of depression in the general population: a validation study using the Composite International Diagnostic Interview as the gold standard. Psychiatry Res. 197(1-2):163–171.
- [APA] American Psychiatric Association 1994. The diagnostic and statistical manual of mental disorders. 4th ed. Washington, DC: APA.
- [APA] American Psychiatric Association 2022. The Diagnostic and Statistical Manual of Mental Disorders: DSM-5-TR. Washington, DC: APA.
- Billieux J, Fournier L. 2022. Commentary on Karhulahti et al (2022): Addressing ontological diversity in gaming disorder measurement from an item-based psychometric perspective. Addict Res Theory. https://doi.org/10.1080/16066359.2022.2125508.
- Borsboom D, Deserno MK, Rhemtulla M, Epskamp S, Fried EI, McNally RJ, Robinaugh DJ, Perugini M, Dalege J, Costantini G, et al. 2021. Network analysis of multivariate data in psychological science. Nat Rev Met Primers. 1(1):1–18.
- Brown TA. 2015. Confirmatory factor analysis for applied research. 2nd ed. New York: the Guilford Press.
- Karhulahti VM, Vahlo J, Martončik M, Munukka M, Koskimaa R, von Bonsdorff M. 2022. Ontological diversity in gaming disorder measurement: a nationally representative registered report. Addict Res Theory. https://doi.org/10.1080/16066359.2022.2115033.
- Karhulahti VM, Siutila M, Vahlo J, Koskimaa R. 2022. Phenomenological strands for gaming disorder and esports play: a qualitative registered report. Collabra: psychol. 8(1):38819.
- Karhulahti VM, Nuutinen S, Lukka L. 2022. Why do adults seek treatment for gaming (disorder)? A qualitative study. Preprint. https://doi.org/10.31234/osf.io/e7a6k.
- Kendler KS. 2017. DSM disorders and their criteria: how should they inter-relate? Psychol Med. 47(12):2054–2060.
- Kujanpää T, Ylisaukko-Oja T, Jokelainen J, Hirsikangas S, Kanste O, Kyngäs H, Timonen M. 2014. Prevalence of anxiety disorders among Finnish primary care high utilizers and validation of Finnish translation of GAD-7 and GAD-2 screening tools. Scand J Prim Health Care. 32(2):78–83.
- Kumpulainen K. 2008. Psychiatric conditions associated with bullying. Int J Adol Med Health. 20(2):121–132.
- Männikkö N, Ruotsalainen H, Tolvanen A, Kääriäinen M. 2019. Psychometric properties of the Internet Gaming Disorder Test (IGDT‐10) and problematic gaming behavior among Finnish vocational school students. Scand J Psychol. 60(3):252–260.
- Orben A, Lakens D. 2020. Crud (re)defined. Adv Methods Pract Psychol Sci. 3(2):238–247.
- Ra K, Das S. 2020. Excessive Spending in a “Free‐to‐Play” Smartphone Game. Am J Addiction. 29(6):528–530.
- Ropovik I, Martončik M, Babincak P, Baník G, Vargová L, Adamkovic M. 2022. Risk and protective factors for (internet) gaming disorder: A meta-analysis. Preprint.