ABSTRACT
Background
Galectin (Gal) is considered a promising immune checkpoint molecule. More and more studies have shown that high expression levels of galectins in hematologic cancer are positively correlated with poor clinical prognosis. However, the exact prognostic significance of galectins remains unclear.
Methods
PubMed, Embase, Web of Science, and Cochrane Library were searched for studies addressing the correlation of galectin expression levels with prognosis of hematologic cancers. Stata software was used to estimate hazard ratios (HR) and 95% confidence intervals (CI).
Result
Hematologic cancer patients with high galectin expression levels showed poor overall survival (OS, HR = 2.43, 95% CI: 1.95, 3.04), disease-free survival (DFS, HR = 3.29, 95% CI: 1.61, 6.71), and event-free survival (EFS, HR = 2.20, 95% CI: 1.47, 3.29) outcomes. Subgroup analysis revealed that high expression levels of galectins pointed to relatively poor OS in MDS (HR = 5.44, 95% CI: 2.09, 14.18), as compared to AML, CHL and CLL. No correlation was found between galectins and OS in NHL and MM. Among the three galectins, Gal-9 (HR = 3.60, 95% CI: 2.03, 6.38) showed higher correlation with poor prognosis than Gal-1 and Gal-3. In addition, use of peripheral blood (HR = 2.96, 95% CI: 2.07, 4.22) samples and qRT-PCR (HR = 2.80, 95% CI: 1.96, 4.01) method for galectin detection were shown to improve its prognostic correlation in hematologic cancers.
Conclusion
Meta-analysis revealed high expression of galectins was associated with poor prognosis in hematologic cancer patients and galectins can be considered a promising prognostic predictive marker.
1. Introduction
Hematologic cancer is one of the most significant public health concerns and an important cause for mortality worldwide. Its characteristics include rapid progression, high malignancy, systemic dissemination, limited treatment options, and a tendency to form minimal residual disease (MRD) [Citation1–3]. The main types of hematologic cancers include leukemia, malignant lymphoma, multiple myeloma (MM), and myelodysplastic syndrome (MDS). Compared to solid tumors, hematologic cancers are challenging to diagnose and treat, thus leading to significant physical and emotional suffering for affected individuals [Citation4–6].
According to Globocan 2020 data released by the International Agency for Research on Cancer (IARC) of the World Health Organization (WHO), it was estimated that there were 1278362 new cases of hematological cancers to be diagnosed worldwide in 2020, accounting for 6.6% of all cancer cases [Citation7]. This indicates a 7.73% increase in the number of new cancer cases worldwide as compared to 2018, suggesting that the growing incidence of hematologic cancers remains a threat and challenge to public health systems [Citation8]. As the majority of hematologic cancers can't be detected by conventional imaging techniques or treated by surgery, early diagnosis and timely intervention of hematologic cancers are crucial for improving the prognosis. Therefore, it is imperative to develop new biomarkers for the diagnosis and prognosis of hematologic cancers.
Galectin (Gal) is a β-galactose binding protein encoded by LGALS genes. It has high affinity for β-galactose residues on polysaccharides. Belonging to the superfamily of animal lectins, galectins are widely distributed in animals [Citation9]. To date, a total of 16 galectins have been identified since first identification in 1976 [Citation10, Citation11]. Galectins can be categorized into three types based on the carbohydrate recognition domain (CRD) in the molecule [Citation12]. The first type is prototypic galectin with a single CRD, including Gal-1, Gal-2, Gal-5, Gal-7, Gal-10, Gal-11, Gal-13, Gal-14, Gal-15, and Gal-16. The second type is chimeric galectin, in which the CRD is linked to a collagen-like N-domain and Gal-3 is the only member. The third type is a tandem-repeat galectin comprising two distinct CRDs; its members include Gal-4, Gal-6, Gal-8, Gal-9, and Gal-12.
Previous studies have demonstrated the vital role of galectins in various biological and pathophysiological processes in vivo, including infection, inflammation, angiogenesis, immune escape, and tumor progression [Citation13]. Galectins were observed to be expressed to varying degrees in heart disease [Citation14–17], kidney disease [Citation18–20] and various cancers [Citation21–28]. Studies have shown that galectin can serve as a biomarker for diagnosis and prognosis, as well as a potential therapeutic target in multiple diseases [Citation10]. Gal-3, as an early biomarker for thyroid cancer, is valuable in assisting the clinical diagnosis of thyroid cancer [Citation29]. Gal-1 [Citation30–33], Gal-3 [Citation34–36], Gal-9 [Citation37–39] and Gal-12 [Citation40] also showed prognostic potential in acute myeloid leukemia (AML), chronic lymphocytic leukemia (CLL), classic Hodgkin lymphoma (CHL), non-Hodgkin lymphoma (NHL) and other hematologic cancers. However, a systematic review assessing the effect of galectins in the prognosis of hematologic cancers is still lacking.
For this, our study evaluated the association between galectin overexpression and prognosis of hematologic cancers through meta-analysis, aiming to elucidate the clinical application value of galectins as a prognostic biomarker for hematologic cancers. Our findings provided references for hematology departments to select appropriate biomarkers to estimate the prognosis of hematologic cancers.
2. Materials and methods
2.1. Literature search
Four databases, i.e. PubMed, Embase, Web of Science, and Cochrane Library, were searched from inception to March 7, 2023 to collect studies on the association between high galectin expression and the prognosis of hematologic cancers. Screening was conducted by two reviewers independently based on the titles and abstracts as well as the full texts of articles. Disagreements were resolved by a third reviewer through consensus. The following terms used in literature search were respectively ‘galectin’, ‘leukemia’, ‘lymphoma’, ‘multiple myeloma’, ‘myelodysplastic syndromes’, ‘prognosis’, etc. Only English-language articles were included. This systematic review was conducted following the recommendations of Preferred Reporting Items for Systematic Reviews and Meta-Analysis (PRISMA) [Citation41] and Meta-analysis of Observational Studies in Epidemiology (MOOSE) [Citation42]. An ethical approval was not required since no human or animal trial was involved in this work.
2.2. Eligibility criteria
The PECOS (population, exposure, comparison, outcome, and study design) format was applied in this review, shown as follows: (1) Population: patients diagnosed with hematologic cancers including leukemia, lymphoma, MM and MDS by cytology and histopathology; (2) Exposure: galectin overexpression at diagnosis; (3) Comparison: comparison between patients with galectin overexpression and those with low expression; (4) Outcome: the hazard ratios (HR) and 95% confidence intervals (CI) of overall survival (OS), disease-free survival (DFS), relapse-free survival (RFS, counted as a distinct form of DFS in this review) and event-free survival (EFS); (5) Study design: observational studies.
The exclusion criteria included: (1) non-English studies; (2) studies with duplicate data already included in this review; (3) studies with incorrect statistical methods; (4) studies exploring the correlation between low galectin expression and prognosis of hematologic cancers; (5) reviews, conference articles, animal studies, case reports and other studies without survival data; (6) studies with HR and 95% CI unavailable. Literature evaluation was independently summarized by two reviewers, and any disagreement was resolved through a consensus judgement by a third reviewer.
2.3. Data extraction
Two reviewers independently extracted the data from eligible articles as follows: author, region, publication year, hematologic cancer type, galectin type, sample size, sample type, galectin detection method, follow-up time, cut-off value of galectins, outcome end-point. As for studies that did not report HR and 95% CI directly, survival curves were used to calculate them by means of GetData Graph Digitaler 2.25 (GetData Pty Ltd., Kogarah, Australia).
2.4. Quality assessment
Each study was independently scored by two reviewers according to Newcastle-Ottawa Scale (NOS). Studies with NOS scores ≥ 7 were considered of high quality, indicating a low risk of bias. Review Manager 5.4 (The Cochrane Collaboration, Oxford, UK) was utilized to produce a risk of graph and risk of bias summary.
2.5. Data analysis
Stata 15.0 (Stata Corp, College Station, TX, U.S.A.) was applied to analyze the data and generate the forest plots. Pooled HR and 95% CI were used to assess the association between high galectin expression and prognosis of hematologic cancers. Heterogeneity among the included studies was evaluated by the I2 statistic and an I2 < 50% indicated non-significant heterogeneity among studies. If so, the fixed-effects model was then applied for meta-analysis. Otherwise, the random-effects model was applied. A subgroup analysis and a sensitivity analysis were performed to address studies with significant clinical heterogeneity. Begg's test, Egger's test and funnel plot asymmetry were used to evaluate the publication bias of included studies, and a p-value of less than 0.05 was considered funnel plot asymmetry, suggesting statistically significant publication bias.
3. Results
3.1. Literature screening process and results
A total of 1329 articles were identified by literature searching. Among them, 472 articles were removed due to duplication; 285 articles, including reviews, conference articles, animal studies and case reports, were excluded due to absence of survival data. After an exclusion of 536 articles by reason of their irrelevance to this review, there remained 36 articles that underwent further screening for eligibility based on the inclusion criteria. Resultantly, a total of 17 articles, which were published between 2000 and 2022, were included in the systematic review and meta-analysis. Out of these, 6 studies were subject to the qualitative review [Citation21, Citation30, Citation33, Citation35, Citation37, Citation39], while 11 studies to the quantitative meta-analysis [Citation31, Citation32, Citation34, Citation36, Citation38, Citation43–48]. The flow diagram of the literature screening procedure is shown in .
3.2. Basic characteristics and quality assessment
The 11 articles for quantitative meta-analysis comprised 1544 patients from China [Citation34, Citation44, Citation45, Citation47], Denmark [Citation31, Citation32], South Korea [Citation36, Citation38], the United States [Citation46], Japan [Citation43], and Poland [Citation48] respectively. The patients were diagnosed with AML [Citation34, Citation44–47], CLL [Citation48], CHL [Citation32, Citation36], NHL [Citation31], MM [Citation38], and MDS [Citation43], covering the majority of hematologic cancers in clinical practice. The characteristics of the included studies are shown in . Based on NOS assessment results, the overall quality of the included studies was deemed to be satisfactory for meta-analysis. The risk chart and risk of bias summary are shown in .
Table 1. The main characteristics of the eligible literatures included in the meta-analysis (n = 11). Studies are ordered by author names.
3.3. Meta-analysis results
3.3.1. OS results
OS data was included in all 11 studies. Results of heterogeneity analysis (I2 = 5.6%, p = 0.389) indicated there was no statistically significant heterogeneity among studies. As shown in , the pooled HR was 2.43 (95% CI: 1.95, 3.04), which indicated that high expression levels of galectins were significantly correlated with poor OS. Sensitivity analysis revealed that the pooled HR was not altered via removing one study at a time, indicating the stability and reliability of the results (). Begg's test showed a risk of bias (p = 0.019) while Egger's test indicated no risk of bias (p = 0.122). No publication bias was observed in the funnel plot ().
3.3.2. DFS results
Two studies contained DSF data. Non-significant heterogeneity was found in I2 test analysis (I2 = 15.7%, p = 0.305). As can be seen in (A), pooled HR for DFS was calculated to be 3.29 (95% CI: 1.61, 6.71), showing a significant association between high expressions of galectins and poor DFS.
3.3.3. EFS results
Two studies had EFS data. Similar to OS and DFS, non-significant heterogeneity (I2 = 14.4%, p = 0.280) was found between the two studies. Meanwhile, the pooled HR was 2.20 (95% CI: 1.47, 3.29), which also indicated the significant association between high galectin expressions and poor EFS ((B)).
3.3.4. Subgroup results
Subgroup analyses were further conducted for cancer types, galectin types, sample types and detection methods based on OS results. The forest plots of subgroup analysis are shown in .
Figure 7. Forest plots of the subgroup analysis of cancer types (A), galectin types (B), sample types (C) and detection methods (D). MDS: myelodysplastic syndrome, AML: acute myeloid leukemia, NHL: non-Hodgkin lymphoma, CHL: classic Hodgkin lymphoma, MM: multiple myeloma, CLL: chronic lymphocytic leukemia, PB: peripheral blood, BM: bone marrow.
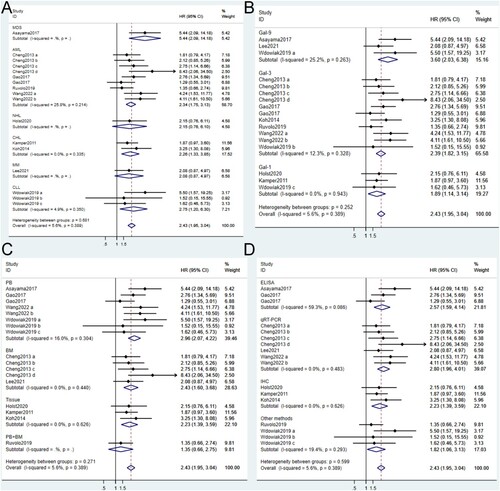
Cancer types. The involved hematologic cancers included MDS, AML, NHL, CHL, MM and CLL. Results showed that high galectin expressions were significantly correlated with poor OS in patients with MDS (HR = 5.44, 95% CI: 2.09, 14.18), AML (HR = 2.34, 95% CI: 1.75, 3.13), CHL (HR = 2.26, 95% CI: 1.33, 3.85), and CLL (HR = 2.75, 95% CI: 1.20, 6.30), while no correlation was found in patients with NHL (HR = 2.15, 95% CI: 0.76, 6.10) and MM (HR = 2.08, 95% CI: 0.87, 4.97). This may be attributed to the relatively small number of related studies and presence of a degree of bias for NHL and MM. The pooled HR results indicated that high galectin expressions were of greater value for poor prognosis in MDS than in other hematologic cancers.
Galectin types. Subgroup analysis for galectin types showed that high expression levels of Gal-1, Gal-3, and Gal-9 were significantly correlated with poor OS in patients with hematologic cancers, and Gal-9 (HR = 3.60, 95% CI: 2.03, 6.38) was superior to Gal-1 (HR = 1.89, 95% CI: 1.14, 3.14) and Gal-3 (HR = 2.39, 95% CI: 1.82, 3.15) in its correlation with the outcome in hematologic cancers.
Sample types. Peripheral blood (PB), bone marrow (BM) and solid tissue samples were utilized for the single or/and combined detection of galectins. Except for the PB and BM-combined detection, all single detection results revealed a statistically significant correlation between high galectin expressions and inferior OS in patients with hematologic cancer. Meanwhile, compared with BM (HR = 2.43, 95% CI: 1.60, 3.68) and tissue (HR = 2.23, 95% CI: 1.39, 3.59), PB-derived galectins (HR = 2.96, 95% CI: 2.07, 4.22) showed superior potential for prognosis prediction.
Detection methods. ELISA (enzyme-linked immunosorbent assay), qRT-PCR (quantitative real-time PCR), IHC (immunohistochemistry) and other methods were used to assess the galectin expressions. The pooled HRs were calculated to be 2.57 for ELISA (95% CI: 1.59, 4.14), 2.80 for qRT-PCR (95% CI: 1.96, 4.01), 2.23 for IHC (95% CI: 1.39, 3.59) and 1.82 for other methods(95% CI: 1.06, 3.13). This means that all methods were considered effective in evaluating correlation of high galectin expressions with poor OS in hematologic cancers, and qRT-PCR was deemed as the optimal detection method.
4. Discussion
The global cancer burden remains on the rise [Citation49]. Compared to solid tumors, hematologic cancers often pose a challenge in terms of early diagnosis due to the limitations of traditional diagnostic methods such as non-specific symptoms like fever, anemia, fatigue, and bone pain [Citation50, Citation51], as well as the inability for CT or MRI to locate lesion sites [Citation52]. Therefore, new effective biomarkers are urgently needed to improve the early diagnosis and prognosis of hematologic cancers. A growing number of studies have shown that high expressions of Gal-1, Gal-3, Gal-9 and other molecules are positively correlated with poor prognosis in patients with hematologic cancer, suggesting that galectins might be a prognostic biomarker for hematologic cancers. Our work is the first systematic review and meta-analysis to evaluate the association between over-expression of galectins and poor prognosis of hematologic cancers. Our results revealed a significant correlation of high galectin expressions with poor prognosis in hematologic cancers.
In this meta-analysis, the pooled HR and 95% CI of OS, DFS and EFS indicated the correlation of high galectin expressions with poor outcome in hematologic cancers. All included studies were considered of high quality according to NOS. There was no statistically significant heterogeneity of publication bias in-between studies.
In subgroup analysis, we found that high galectin expressions had better correlation with poor prognosis in MDS than in other tumors, while no correlation was observed in NHL and MM. In a NHL study included the present review, the authors observed a significant difference in OS between Gal-1 high and low expression groups in the CD30-positive subcohort instead of the entire cohort [Citation31]. Similarly, in a MM study included, there was no statistical difference in OS between the gal-9 positive and negative groups in the whole cohort. However, in PD-L1 overexpression subcohort, patients with gal-9 positivity had significantly worse OS than the galectin-negative compartment [Citation38]. Therefore, we inferred that galectins’ correlation with OS was context-dependent in NHL and MM. Or, other factors than galectins, like CD30 and PD-L1, affected OS as well, thus obscuring the real OS- correlation of galectins. Zhang et al. discovered a positive correlation between gal-9 and proteasome subunit beta type-8 (PSMB8). Meanwhile, these two biomarkers had a synergistic effect on the survival of cells, cell signaling, and the progression of AMLs [Citation53]. This indicates that combined detection of galectin and other molecules (PSMB8) will contribute to better prognosis prediction for hematologic cancers.
Gal-9 exhibited better correlation with poor prognosis than Gal-1 and Gal-3 in this review, indicating the superior prognosis potential of Gal-9 in hematologic cancers. In recent years, there have been several reports underlying the mechanisms of Gal-9/Tim-3 signaling up-regulation in hematologic cancers. TIM-3 was over-expressed in bone marrow-resident T cells of AML patients with treatment failure, leading to increased number of Gal9+ CD34- T cells, as compared with AML patients with complete response [Citation54]. Additionally, gal-9 was found to stimulate TIM-3 to co-activate NF-κB and β-catenin signaling pathways in the microenvironment of leukemia, thus accelerating the leukemia progression through TIM-3/Gal-9 autocrine rings [Citation55]. Similarly, the Gal-9/TIM-3 signaling pathway was also activated in CLL, which promoted Treg cell-mediated immune escape through inhibiting CD4+ T cells [Citation56]. On the other hand, inhibition of the Gal-9/TIM-3 axis in combination with chemotherapy suppressed the inflammatory response and proliferation of tumor tissues, thus delaying the recurrence of AML [Citation57]. Besides, inhibition of Gal-9 alone or in combination with other antibodies (anti-PD-L1, anti-GITR) could suppress tumor proliferation and prolong OS in varying degrees in syngeneic tumor mice models [Citation58]. All these supported our finding that Gal-9 was significantly correlated with poor outcome in patients with hematologic cancers, affirming the prognosis prediction value of Gal-9. So far, only Gal-1, Gal-3, and Gal-9 have been extensively studied. Whether expressions of other galectins affect the prognosis of hematologic cancers and the underlying mechanism require to be further investigated.
The optimal sample source for galectins was PB, compared to BM and tissue. This is surprising, as we thought BM-derived galectins would be more convincing for prognosis prediction since most hematologic cancers are BM-originated. However, galectins are known to be produced and secreted by diverse cell types including cancer cells (melanoma, prostate cancer, ovary carcinomas, AML, etc.) [Citation59] and non-cancer cells (monocytes, T cells, B cells, DCs, macrophages, stroma cells, etc.) [Citation60, Citation61]. Additionally, they function both intercellularly and extracellularly [Citation62–64]. Therefore, we speculated that both medullary and extramedullary-sourced galectins, which contributed to its enrichment in PB, participated in the malignant progress of hematologic cancers [Citation65], leading to the better prognosis correlation of PB-derived ones. Still, further large-scale observational studies and mechanism research are required to verify this result. In this review, qRT-PCR was found to be the best method for galectin detection, compared to IHC, ELISA, and other methods. Despite highly sensitive, qRT-PCR reflect the transcriptional levels of galectin expression while ELISA and IHC detect the proteins levels. Because galectins function in the protein form, such methods as ELISA was necessary for detection of galectin protein levels.
Limitations of our work: (1) the retrospective nature of the included studies may generate recall, measurement, and reporting biases in the study design; (2) there was a small number of DFS- or/and EFS-related studies, and more studies are required for better evaluation of galectins’ prognostic value in hematologic cancers; (3) due to the lack of the original HR and 95% CI data in 7 of the 11 cohort studies, we extracted related data and calculate HR and 95% CI based on the survival curve, during which errors might be inevitably caused; (4) different cut-off values were set for galectins in different studies, which could affect the accuracy of correlation analysis; (5) there was lack of correlation analysis between high galectin expressions and other prognostic factors like cytogenetic and molecular abnormalities.
In summary, Our results suggested that high expression levels of galectins were associated with poor OS, DFS and EFS in hematologic cancer patients. Subgroup analysis showed that galectins had the greatest prognostic value in MDS, with Gal-9 exhibiting optimal prognostic correlation. Meanwhile, the use of PB samples and qRT-PCR method for galectin detection contributed to its prognostic correlation. All these findings may be helpful for the departments of hematology to select appropriate biomarkers for prognosis prediction. In the next step, we will confirm the correlations of high Gal-9 expression with poor prognosis in AML using clinical samples and animal models. In the meantime, we are hoping to identify new prognosis-related biomarkers in AML. On the other hand, an association analysis will be conducted between galectin expressions and molecular abnormalities in AML using online databases, followed by experimental validation using clinical samples.
Acknowledgements
Haotian Liu and Tiantian She designed the study. Qiuying Dai and Yixian Li conducted literature search. Zhenfei Tang and Qiuying Dai performed the data collection. Haotian Liu was in charge of using the software. Haotian Liu and Tiantian She analyzed the data and composed the final version. This work was supported by Tianjin Municipal Education Commission [Grant No.2021KJ262].
Disclosure statement
No potential conflict of interest was reported by the author(s).
Data availability
The data could be obtained by contacting corresponding author.
Additional information
Funding
References
- Van Dongen JJ, Seriu T, Panzer-Grümayer ER, et al. Prognostic value of minimal residual disease in acute lymphoblastic leukaemia in childhood. Lancet. 1998;352(9142):1731–1738. DOI:10.1016/S0140-6736(98)04058-6
- Raff T, Gökbuget N, Lüschen S, et al. Molecular relapse in adult standard-risk ALL patients detected by prospective MRD monitoring during and after maintenance treatment: data from the GMALL 06/99 and 07/03 trials. Blood. 2007;109(3):910–915. DOI:10.1182/blood-2006-07-037093
- Ben Lassoued A, Nivaggioni V, Gabert J. Minimal residual disease testing in hematologic malignancies and solid cancer. Expert Rev Mol Diagn 2014;14(6):699–712. DOI:10.1586/14737159.2014.927311
- O'Brien S, Del Giglio A, Keating M. Advances in the biology and treatment of B-cell chronic lymphocytic leukemia. Blood. 1995;85(2):307–318. DOI:10.1182/blood.V85.2.307.307
- Noren DP, Long BL, Norel R, et al. A crowdsourcing approach to developing and assessing prediction algorithms for AML prognosis. PLoS Comput Biol. 2016;12(6):e1004890. DOI:10.1371/journal.pcbi.1004890
- Burkhardt B, Hermiston ML. Lymphoblastic lymphoma in children and adolescents: review of current challenges and future opportunities. Br J Haematol. 2019;185(6):1158–1170. DOI:10.1111/bjh.15793
- Siegel RLJ, et al. Global cancer statistics 2020: GLOBOCAN estimates of incidence and mortality worldwide for 36 cancers in 185 countries. CA Cancer J Clin. 2021;71(3):209–249. DOI:10.3322/caac.21660
- Bray F, Ferlay J, Soerjomataram I, et al. Global cancer statistics 2018: GLOBOCAN estimates of incidence and mortality worldwide for 36 cancers in 185 countries. CA Cancer J Clin. 2018;68(6):394–424. DOI:10.3322/caac.21492
- Rabinovich GA, Toscano MA. Turning ‘sweet’ on immunity: galectin-glycan interactions in immune tolerance and inflammation. Nat Rev Immunol. 2009;9(5):338–352. DOI:10.1038/nri2536
- Dong R, Zhang M, Hu QY, et al. Galectin-3 as a novel biomarker for disease diagnosis and a target for therapy. Int J Mol Med 2018;41(2):599–614.
- Kaminker JD, Timoshenko AV. Expression, Regulation, and functions of the galectin-16 gene in human cells and tissues. Biomolecules. 2021;11:12.
- Sciacchitano S, Lavra L, Morgante A, et al. Galectin-3: One molecule for an alphabet of diseases, from A to Z. Int J Mol Sci. 2018;19:2.
- Liu FT, Rabinovich GA. Galectins as modulators of tumour progression. Nat Rev Cancer. 2005;5(1):29–41. DOI:10.1038/nrc1527
- De Boer RA, Voors AA, Muntendam P, et al. Galectin-3: a novel mediator of heart failure development and progression. Eur J Heart Fail 2009;11(9):811–817. DOI:10.1093/eurjhf/hfp097
- Shah RV, Chen-Tournoux AA, Picard MH, et al. Galectin-3, cardiac structure and function, and long-term mortality in patients with acutely decompensated heart failure. Eur J Heart Fail 2010;12(8):826–832. DOI:10.1093/eurjhf/hfq091
- De Boer RA, Van Veldhuisen DJ, Gansevoort RT, et al. The fibrosis marker galectin-3 and outcome in the general population. J Intern Med 2012;272(1):55–64. DOI:10.1111/j.1365-2796.2011.02476.x
- Chow SL, Maisel AS, Anand I, et al. Role of biomarkers for the prevention, assessment, and management of heart failure. A scientific statement from the American heart association. Circulation. 2017;135(22):E1054–E1E91.
- O'Seaghdha CM, Hwang SJ, Ho JE, et al. Elevated galectin-3 precedes the development of CKD. J Am Soc Nephrol. 2013;24(9):1470–1477.
- Chen SC, Kuo PL. The role of galectin-3 in the kidneys. Int J Mol Sci. 2016;17:4.
- Desmedt V, Desmedt S, Delanghe JR, et al. Galectin-3 in renal pathology: more than just an innocent bystander? Am J Nephrol. 2016;43(5):305–317. DOI:10.1159/000446376
- Plattel WJ, Alsada ZND, Van Imhoff GW, et al. Biomarkers for evaluation of treatment response in classical Hodgkin lymphoma: comparison of sGalectin-1, sCD163 and sCD30 with TARC. Br J Haematol 2016;175(5):868–875. DOI:10.1111/bjh.14317
- Welton JL, Khanna S, Giles PJ, et al. Proteomics analysis of bladder cancer exosomes. Mol Cell Proteom. 2010;9(6):1324–1338. DOI:10.1074/mcp.M000063-MCP201
- Arcolia V, Journe F, Wattier A, et al. Galectin-1 is a diagnostic marker involved in thyroid cancer progression. Int J Oncol 2017;51(3):760–770. DOI:10.3892/ijo.2017.4065
- Arcolia V, Journe F, Renaud F, et al. Combination of galectin-3, CK19 and HBME-1 immunostaining improves the diagnosis of thyroid cancer. Oncol Lett. 2017;14(4):4183–4189. DOI:10.3892/ol.2017.6719
- Wang JT, Liu YD, Yang YF, et al. High expression of galectin-7 associates with poor overall survival in patients with non-metastatic clear-cell renal cell carcinoma. Oncotarget. 2106;7(27):41986–41995. DOI:10.18632/oncotarget.9749
- Xu YY, Li CF, Sun JH, et al. Antitumor effects of galectin-3 inhibition in human renal carcinoma cells. Exp Biol Med 2016;241:1365–1373. DOI:10.1177/1535370216630839
- Aboulhagag NA, El-Deek HEM, Sherif MF. Expression of galectin-1 and galectin-3 in renal cell carcinoma; immunohistochemical study. Ann Diagn Pathol. 2018;36:31–37. DOI:10.1016/j.anndiagpath.2018.06.005
- Shen JJ, Person MD, Zhu JJ, et al. Protein expression profiles in pancreatic adenocarcinoma compared with normal pancreatic tissue and tissue affected by pancreatitis as detected by two-dimensional gel electrophoresis and mass spectrometry. Cancer Res 2004;64(24):9018–9026. DOI:10.1158/0008-5472.CAN-04-3262
- Kalfert D, Ludvikova M, Kholova I, et al. Combined use of galectin-3 and thyroid peroxidase improves the differential diagnosis of thyroid tumors. Neoplasma. 2020;67(1):164–170. DOI:10.4149/neo_2019_190128N86
- Cedeno-Laurent F, Watanabe R, Teague JE, et al. Galectin-1 inhibits the viability, proliferation, and Th1 cytokine production of nonmalignant T cells in patients with leukemic cutaneous T-cell lymphoma. Blood. 2012;119(15):3534–3538. DOI:10.1182/blood-2011-12-396457
- Holst JM, Ludvigsen M, Hamilton-Dutoit SJ, et al. High intratumoural galectin-1 expression predicts adverse outcome in ALK- ALCL and CD30+ PTCL-NOS. Hematol Oncol. 2020;38(1):59–66. DOI:10.1002/hon.2702
- Kamper P, Ludvigsen M, Bendix K, et al. Proteomic analysis identifies galectin-1 as a predictive biomarker for relapsed/refractory disease in classical Hodgkin lymphoma. Blood. 2011;117(24):6638–6649. DOI:10.1182/blood-2010-12-327346
- Ouyang J, Plutschow A, Von Strandmann EP, et al. Galectin-1 serum levels reflect tumor burden and adverse clinical features in classical Hodgkin lymphoma. Blood. 2013;121(17):3431–3433. DOI:10.1182/blood-2012-12-474569
- Cheng CL, Hou HA, Lee MC, et al. Higher bone marrow LGALS3 expression is an independent unfavorable prognostic factor for overall survival in patients with acute myeloid leukemia. Blood. 2013;121(16):3172–3180. DOI:10.1182/blood-2012-07-443762
- Iurisci I, Tinari N, Natoli C, et al. Concentrations of galectin-3 in the sera of normal controls and cancer patients. Clin Cancer Res. 2000;6(4):1389–1393.
- Koh YW, Jung SJ, Park CS, et al. LGALS3 as a prognostic factor for classical Hodgkin's lymphoma. Mod Pathol. 2014;27(10):1338–1344. DOI:10.1038/modpathol.2014.38
- Alame M, Cornillot E, Cacheux V, et al. The immune contexture of primary central nervous system diffuse large B cell lymphoma associates with patient survival and specific cell signaling. Theranostics. 2021;11(8):3565–3579. DOI:10.7150/thno.54343
- Lee BH, Park Y, Kim JH, et al. Prognostic Value of Galectin-9 Relates to Programmed Death-Ligand 1 in Patients With Multiple Myeloma. Front Oncol. 2021;11: 669817. DOI:10.3389/fonc.2021.669817
- Nakajima R, Miyagaki T, Kamijo H, et al. Possible therapeutic applicability of galectin-9 in cutaneous T-cell lymphoma. J Dermatol Sci 2019;96(3):134–142. DOI:10.1016/j.jdermsci.2019.09.004
- El Leithy AA, Helwa R, Assem MM, et al. Expression profiling of cancer-related galectins in acute myeloid leukemia. Tumor Biol. 2015;36(10):7929–7939. DOI:10.1007/s13277-015-3513-0
- Moher D, Liberati A, Tetzlaff J, et al. Preferred reporting items for systematic reviews and meta-analyses: the PRISMA statement. Br Med J. 2009;339:b2535. DOI:10.1136/bmj.b2535
- Stroup DF, Berlin JA, Morton SC, et al. Meta-analysis of observational studies in epidemiology – a proposal for reporting. J Am Med Assoc. 2000;283(15):2008–2012.
- Asayama T, Tamura H, Ishibashi M, et al. Functional expression of Tim-3 on blasts and clinical impact of its ligand galectin-9 in myelodysplastic syndromes. Oncotarget. 2017;8(51):88904–88917. DOI:10.18632/oncotarget.21492
- Gao N, Wang XX, Sun JR, et al. Clinical impact of galectin-3 in newly diagnosed t (15;17)(q22;q21)/PML-RARa acute promyelocytic leukemia treated with all-trans retinoic acid and arsenic trioxide-based regimens. Ann Hematol 2017;96(5):711–718. DOI:10.1007/s00277-017-2948-3
- Gao N, Yu WZ, Guo NJ, et al. Clinical significance of galectin-3 in patients with adult acute myeloid leukemia: a retrospective cohort study with long-term follow-up and formulation of risk scoring system. Leuk Lymphoma. 2017;58(6):1394–1402. DOI:10.1080/10428194.2016.1243677
- Ruvolo PP, Hu CW, Qiu YH, et al. LGALS3 is connected to CD74 in a previously unknown protein network that is associated with poor survival in patients with AML. Ebiomedicine. 2019;44:126–137. DOI:10.1016/j.ebiom.2019.05.025
- Wang JY, Gao N, Wang XX, et al. Prognostic Factors in Acute Myeloid Leukemia with t(8;21)/AML1-ETO: Strategies to Define High-Risk Patients. Ind J Hematol Blood Trans. 2022;38(4):631–637. DOI:10.1007/s12288-021-01507-9
- Wdowiak K, Gallego-Colon E, Francuz T, et al. Increased serum levels of Galectin-9 in patients with chronic lymphocytic leukemia. Oncol Lett. 2019;17(1):1019–1029.
- The global burden of cancer attributable to risk factors, 2010-19: a systematic analysis for the Global Burden of Disease Study 2019. Lancet. 2022;400(10352):563-591.
- Steele M, Narendran A. Mechanisms of defective erythropoiesis and anemia in pediatric acute lymphoblastic leukemia (ALL). Ann Hematol. 2012;91(10):1513–1518. DOI:10.1007/s00277-012-1475-5
- Chennamadhavuni A, Lyengar V, Mukkamalla SKR, et al. Leukemia. StatPearls. Treasure Island (FL); StatPearls Publishing Copyright © 2022, StatPearls Publishing LLC. 2022.
- Heilmeier B, Spiekermann K, Bohlander S, et al. Current methods for diagnosing leukemia in adults. Deutsch Med Wochenschr. 2009;134(23):1222–1226. DOI:10.1055/s-0029-1222598
- Zhang YP, Xue S, Hao Q, et al. Galectin-9 and PSMB8 overexpression predict unfavorable prognosis in patients with AML. J Cancer. 2021;12(14):4257–4263. DOI:10.7150/jca.53686
- Dama P, Tang M, Fulton N, et al. Gal9/Tim-3 expression level is higher in AML patients who fail chemotherapy. J Immunother Cancer. 2019;7(1):175. DOI:10.1186/s40425-019-0611-3
- Kikushige Y, Miyamoto T, Yuda J, et al. A TIM-3/Gal-9 autocrine stimulatory loop drives self-renewal of human myeloid leukemia stem cells and leukemic progression. Cell Stem Cell. 2015;17(3):341–352. DOI:10.1016/j.stem.2015.07.011
- Pang NN, Alimu X, Chen R, et al. Activated Galectin-9/Tim3 promotes Treg and suppresses Th1 effector function in chronic lymphocytic leukemia. FASEB J. 2021;35:7.
- Nasri F, Sadeghi F, Behranvand N, et al. Oridonin could inhibit inflammation and T-cell immunoglobulin and Mucin-3/Galectin-9 (TIM-3/Gal-9) autocrine loop in the acute myeloid leukemia cell line (U937) as compared to doxorubicin. Iran J Allergy Asthma Immunol. 2020;19(6):602–611.
- Yang RY, Sun LL, Li CF, et al. Galectin-9 interacts with PD-1 and TIM-3 to regulate T cell death and is a target for cancer immunotherapy. Nat Commun. 2021;12:1.
- Banh A, Zhang J, Cao H, et al. Tumor galectin-1 mediates tumor growth and metastasis through regulation of T-cell apoptosis. Cancer Res. 2011;71(13):4423–4431. DOI:10.1158/0008-5472.CAN-10-4157
- Salazar F, Sewell HF, Shakib F, et al. The role of lectins in allergic sensitization and allergic disease. J Allergy Clin Immunol. 2013;132(1):27–36. DOI:10.1016/j.jaci.2013.02.001
- Yi L, Feng Y, Chen D, et al. Association between galectin-13 expression and eosinophilic airway inflammation in chronic obstructive pulmonary disease. Copd. 2023;20(1):101–108. DOI:10.1080/15412555.2022.2162377
- Rotshenker S. Galectin-3 (MAC-2) controls phagocytosis and macropinocytosis through intracellular and extracellular mechanisms. Front Cell Neurosci. 2022;16:949079. DOI:10.3389/fncel.2022.949079
- Sewgobind NV, Albers S, Pieters RJ. Functions and inhibition of galectin-7, an emerging target in cellular pathophysiology. Biomolecules. 2021;11:11.
- Mathew MP, Donaldson JG, Hanover JA. Evaluating the role of galectins in clathrin-independent endocytosis. Methods Mol Biol. 2022;2442:391–411. DOI:10.1007/978-1-0716-2055-7_21
- Fei F, Zhang M, Tarighat SS, et al. Galectin-1 and galectin-3 in B-cell precursor acute lymphoblastic leukemia. Int J Mol Sci. 2022;23:22.