ABSTRACT
Background:
Cuprotosis is a mode of cell death triggered by copper accumulation. There is a lack of studies on the role of cuprotosis-related lncRNA in acute myeloid leukaemia (AML).
Methods:
Expression data and corresponding clinical data of lncRNA and mRNA were sourced from TCGA database. Pearson’s correlation, differential expression, univariate Cox regression, and least absolute shrinkage and selection operator (LASSO) analysis were performed to screen for a cuprotosis-related lncRNA signature and to evaluate its prognostic significance. A prognostic model was constructed, and patients were categorized into high- and low-risk groups according to their calculated risk scores. The model's performance was then assessed in internal training, and internal and external testing sets. The high- and low- risk groups were examined to explore their involvement in AML. The relationship between the risk score and various clinical parameters, mutational landscapes, immune cell scores, and drug sensitivity were investigated.
Results:
Five cuprotosis-related lncRNAs (AC020571.3, CTD-2325M2.1, RP11-802O23.3, RP11-474N24.6, and UCA1) were identified, which were differentially expressed in AML datasets in comparison to normal controls and significantly associated with prognosis. Consistent with the results obtained from the training and testing sets, the high-risk group had a poor prognosis with good predictive power. The high- and low-risk groups differed significantly in immune-related biological processes and the IC50 values of WH-4.023, mitomycin C, navitoclaxin, and PD-0325901.
Conclusion:
Five cuprotosis-related lncRNA signatures were screened as prognostic predictors to provide new insights into lncRNA-based diagnostic and therapeutic strategies for AML.
Introduction
Acute myeloid leukaemia (AML) is a predominant form of acute leukaemia and is characterized by a high incidence rate. Despite advancements in treatment, the overall prognosis of AML remains heterogeneous and suboptimal, with a 5-year survival rate of less than 50% [Citation1]. Several prognostic risk models for AML have been developed, and the updated European Leukaemia Network risk stratification is widely implemented in clinical practice, playing a crucial role in the clinical decision-making process and clinical management [Citation2]. Long non-coding RNAs (lncRNAs), comprising more than 200 nucleotides, lack protein-coding capacity but regulate gene expression at the transcriptional, post-transcriptional, and epigenetic levels [Citation3]. LncRNAs have emerged as critical regulators of tumourigenesis, exhibiting potential as prognostic markers across various malignancies [Citation4,Citation5]. Additionally, they exert significant control over haematopoietic stem cell (HSC) maintenance and govern the fate determination of HSCs into precursor and progenitor blood cells [Citation6,Citation7]. Recent investigations have unveiled the intricate involvement of lncRNAs in AML initiation, progression, and drug resistance, fuelling the exploration of lncRNA, targeting a prospective therapeutic avenue for AML [Citation8]. Mounting evidence supports the independent prognostic value of IncRNAs in patients with AML, leading to the development of multiple prognostic lncRNA signatures.
Copper is an essential trace element that plays vital roles in growth, development, metabolism, and reproduction [Citation9]. Under normal conditions, intracellular copper concentrations are tightly regulated and remain relatively stable. The absorption and excretion of copper are intricately regulated in mammals; inadequate copper levels compromise metalloenzyme function, while copper levels exceeding the homeostatic threshold induces cell death [Citation10]. Cuprotosis differs from programmed cell death and depends on mitochondrial respiration. Copper directly binds to lipidated fractions of the tricarboxylic acid cycle, triggering protein lipoylation and loss of iron–sulphur cluster proteins, thus causing proteotoxic stress that induces cell death [Citation11]. Copper seems to be more essential for cancer cell proliferation and survival than for healthy cells [Citation12]. Previous studies have shown that copper is essential for tumour progression, angiogenesis, immune escape, and chemoresistance [Citation13]; blocking copper transport can inhibit tumour cell proliferation, which is a potential anticancer strategy [Citation14]. Serum copper levels are elevated in haematological malignancies during relapse or disease progression, while normal copper levels are associated with remission or stable disease [Citation15]. Elevated serum copper levels are indicative of an adverse prognosis in chronic lymphocytic leukaemia, showing a positive correlation with absolute peripheral blast cells [Citation16].
Cuprotosis-associated lncRNA signatures can indicate immune microenvironmental features, serving as independent prognostic markers in soft tissue sarcoma [Citation17]. Furthermore, a cuprotosis-related lncRNA signature can be a promising biomarker for predicting prognosis and drug sensitivity in head and neck cancer [Citation18]. These findings highlight the potential of cuprotosis-associated lncRNA signatures as prognostic indicators. Accordingly, this, study aimed to screen cuprotosis-associated lncRNA prognostic signatures by mining the lncRNA data obtained from genome-wide profiles and clinical data in the TCGA database. Univariate Cox and LASSO Cox analyses were performed to identify novel prognostic biomarkers and construct a prognostic risk model. Additionally, the association between the lncRNA model and clinical features and molecular characteristics was explored. The ultimate goal of this study was to develop a novel prognostic risk model and provide valuable guidance for the clinical diagnosis and prognosis of AML.
Material and methods
Instruments and reagents
The following reagents and instruments were used: foetal bovine serum (FBS) (Gibco-BRL, USA), IMDM (Gibco-BRL, USA), 1640 (Gibco-BRL, USA), penicillin/streptomycin (P/S) (Gibco-BRL, USA), CO2 incubator (Heal Force, China), Spin Column RNA Cleanup & Concentration Kit (Sangon Biotech, China), nuclease-free water (Sparkjade, China), SweScript All-in-One First-Strand cDNA Synthesis SuperMix for qPCR (Sparkjade, China), 2×SYBR Green qPCR Master Mix (Sparkjade, China), desktop high speed refrigerated centrifuge (DragonLab, China), NanoDrop 2000 (NanoDrop, USA), quantitative fluorescence PCR instrument (Bio-rad, USA), Ultra-micro spectrophotometer (Thermo, USA), and refrigerated centrifuge (Hitachi, Japan). Primers were synthesized by Sangon Biotech (Sangon, China).
Cell culture
The human AML cell line KG-1a, the acute monocytic leukaemia cell line THP-1, and the immortalized normal hepatocyte cell line LO2 were purchased from the Chinese Academy of Sciences cell library. THP-1 and LO2 cells were cultured in 10% FBS-RPMI 1640 at 37°C and 5% CO2. KG-1a was cultured in IMDM supplemented with 10% FBS and 1% P/S at 37°C in humidified air with 5% CO2.
Data collection and processing
TCGA and GTEx clinical data and sequencing data for lncRNAs and mRNA were obtained from the Toil RNA-seq recompute data hub on the UCSC Xena server (https://xena.ucsc.edu/) [Citation19]. For the sequencing data, the raw reads obtained from each library were normalized to the TPM. The ‘tinyarray’ package was used to convert the probe ID to the gene name SYMBOL, with Homo sapiens as the selected species. Data derived from Vizome database (http://vizome.org/) were used to validate external data validation.
Identification of cuprotosis-related lncRNA
Thirteen cuprotosis-related genes (ATP7A, ATP7B, DLST, DBT, DLD, DLAT, LIPT1, LIAS, PDHA1, PDHB, FDX1, GCSH, and SLC31A1) were obtained from a published study [Citation11]. Pearson correlation coefficients were calculated between lncRNA expression and cuprotosis-related genes with a threshold of |R| > 0.4 and p < 0.05. Repeated lncRNAs were then removed, and the original candidate lncRNAs were obtained. The ‘survival’ package was used to perform univariate Cox analysis to identify survival-associated lncRNA biomarkers. The ‘limma’ package was further applied to obtain differentially expressed prognostic lncRNAs with the criteria of p < 0.05 and |logFC| > 1.5. Based on the above screening, LASSO analysis was performed to screen and shrink the data using the ‘glmnet’ package with 10-fold cross-validation.
Real-time quantitative PCR (RT-qPCR)
The expression level of screened lncRNA was validated in the human AML cell lines, KG-1a and THP-1, and the normal human liver cell line, LO2. RNA was extracted from cells using the column-based system RNAeasy mini kit (Qiagen) according to the manufacturer’s instructions. RNA concentration and purity were measured using NanoDrop 2000. Excessively high RNA concentrations were diluted to a final concentration of 200 ng/μL. Reverse transcription was performed in a reaction system containing 10 μL RNA, 1 μL gDNA remover, and 4 μL 5×SweScript All-in-One SuperMix for qPCR, and the volume was made up to 20 µL with RNase free water. The reaction was gently mixed and centrifuged briefly. For PCR, the following primers were used: AC020571.3 forward primer 5′GGA GAC AAC CAG AAG CAG CAA T-3′ and AC020571.3 reverse primer 5′- GTGAATGTGCCACTGAACTGTATCT′; CTD-2325M2.1 forward primer 5′- AGAGATGGGGTTTCACTATGTTGG-3′ and CTD-2325M2.1 reverse primer 5′- TGCCTGTAATCCCAGCACTTTG-3′; UCA1 forward primer 5′-TGTTTGTTGTTAGAGGGCTTGG-3′, and UCA1 reverse primer 5′-ATTCCCTGTTGCTAAGCCGAT-3′; RP11-474N24.6 forward primer 5′-ATCCAGGTATTAGGGTCCATCCA-3′; and RP11-474N24.6 reverse primer 5′-CACCTCTCACTCCCAGGAATTTC-3′; RP11-802O23.3 forward primer 5′-AGAGTGCCTGCACTACACAC-3′; and RP11-802O23.3 reverse primer 5′-AACAAAGTTGCCACCCCCAT-3′; GAPDH forward primer 5′-GGAAGCTTGTCATCAATGGAAATC-3′, and GAPDH reverse primer 5′-TGATGACCCTTTTGGCTCCC-3′. RT-qPCR assay was performed, and reaction system was configured according to the kit. Relative lncRNA expression was calculated using the comparative ΔΔCt method, and values were expressed as 2-ΔΔCt.
Construction and validation of the prognostic risk model
The LASSO Cox model of screened cuprotosis-related lncRNAs was built based on the entire AML cohort from TCGA to predict the risk scores of samples and divide them into low- and high-risk groups based on the optimal cut-offs (R package ‘glmnet’). The risk score for each patient was determined based on the following risk formula: risk score = (lncRNA1 expression × lncRNA1 Coef) + (lncRNA2 expression × lncRNA2 Coef) + (lncRNAn expression × lncRNAn Coef). The survival differences between the low- and high-risk groups were assessed using Kaplan-Meier (KM) survival curves. Receiver-operating characteristic (ROC) curves were used to assess the accuracy of the prognostic risk models. Time-dependent receiver-operating characteristic (ROC) analysis was used to assess the predictive potential of the prognostic risk models. The entire AML cohort was partitioned into the internal training and internal testing sets at an approximate ratio of 7:3 using the ‘caret’ package, which were used to test the robustness of the constructed prognostic risk model. An external testing set was obtained from Vizome database to validate the diagnostic and prognostic predictive power.
Correlation between the prognostic risk model and age, morphology, cytogenetics risk and blast cell percentage
Univariate and multivariate Cox proportional hazards analyses were conducted to analyse the correlation between the prognostic risk model and clinical parameters, including age, morphology, cytogenetic risk, and blast cell percentage. Survival differences between the high- and low-risk groups in the different subgroups were analysed using KM survival curves. Overall survival (OS) at one, three, and five years was predicted using a prognostic nomogram model based on the ‘rms’ package. Calibration and decision curve analysis (DCA) were used to assess the calibration and clinical utility of the model, respectively.
Functional enrichment analysis
To assess the potential differences of biological functions between the high- and low-risk groups, differentially expressed genes (DEGs) between the high- and low-risk groups were screened using ‘limma’ package, which were used for gene set enrichment analysis (GSEA). Then, DEGs between the high-risk group and normal samples, as well as DEGs between the low-risk group and normal samples were collected using the criteria of p < 0.05 and |logFC|> 1.5. Kyoto Encyclopaedia of Genes and Genomes (KEGG) analysis of DEGs was performed using g:profiler [Citation20] to further explore the molecular biological processes of the high- and low-risk groups.
Association between mitochondria-associated pathways and screened lncRNAs
In MitoCarta 3.0, there are 1,136 human genes listed that encode proteins with evidence of mitochondrial localization. These genes are further categorized based on their sub-mitochondrial compartment. Additionally, the genes are assigned to a custom ontology consisting of 149 mitochondrial pathways, which helps in understanding their functional roles within the mitochondria [Citation21]. Considering the tight link between mitochondrial metabolism and cuprotosis, the association between the screened lncRNAs and mitochondria-associated pathways was further explored. Mitochondria-associated pathways were sourced from MitoCarta3.0. The enrichment scores of mitochondria-associated pathways were calculated using single sample GSEA (ssGSEA). Pearson’s correlation analysis was performed to assess the relationship between the screened lncRNAs and mitochondrial-associated pathways.
Immune infiltration, mutational and drug sensitivity analysis
The xCell algorithm was used based on transcriptomic data to compare immune cell fractions between the high- and low-risk groups [Citation22]. The ‘Maftoolto’ package was used to assess the somatic mutation between high- and low- risk groups. The ‘pRRophetic’ package was implemented for chemotherapeutic response prediction.
Result
Screened for cuprotosis-related lncRNAs associated with AML prognosis
Pearson correlation analysis was conducted using lncRNA and mRNA expression data, leading to the identification of 2,171 cuprotosis-related lncRNAs. After removing redundant genes, a total of 1,684 lncRNAs were retained for further analysis. LncRNAs with a zero value in more than 50% of the samples were filtered out, resulting in 1,321 lncRNAs for subsequent investigation. Univariate Cox analysis identified 205 lncRNAs significantly associated with prognosis. Differential expression analysis revealed 19 differentially expressed prognostic lncRNAs ((a)). Using LASSO analysis, the following lncRNAs were identified as being significantly associated with prognosis: AC020571.3, CTD-2325M2.1, RP11-802O23.3, RP11-474N24.6, and UCA1 ((b,c)). Compared to normal controls, patients with AML exhibited elevated expression of RP11-802O23.3 and decreased expression of AC020571.3, CTD-2325M2.1, RP11-474N24.6, and UCA1 ((a)). Consistent findings were obtained from the Vizome database, where RP11-802O23.3 was increased, and the other four lncRNAs exhibited differential upregulation and downregulation in 357 AML samples compared to 19 healthy control samples ((b)). The expression levels of the selected lncRNAs were validated in the KG-1a, THP-1, and LO2. The results showed that AC020571.3, CTD-2325M2.1, RP11-474N24.6, and UCA1 were downregulated in THP-1 and KG-1a, while RP11-802O23.3 was upregulated in THP-1 and KG-1a compared to that in LO2 (Figure 2(c)). According to data from TCGA, decreased expression of AC020571.3, RP11-802O23.3, and UCA1, as well as elevated expression of CTD-2325M2.1 and RP11-474N24.6, was associated with poor prognosis ((d–h)).
Figure 1. Cuprotosis-related lncRNAs associated with AML prognosis in TCGA database. (a) Heatmap of 19 cuprotosis-related lncRNAs differentially expressed in normal and AML. (b and c) Five cuprotosis-related lncRNAs signatures obtained by using a LASSO cross-validation dimension reduction.
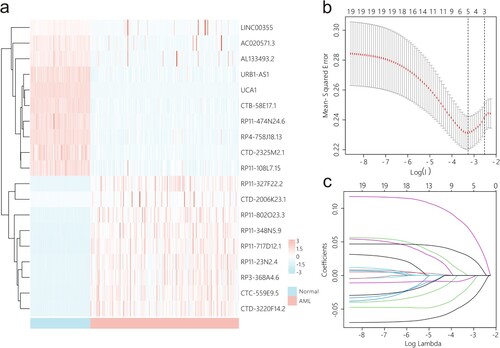
Figure 2. Expression pattern and prognostic significance of cuprotosis-related lncRNAs. (a) The expression pattern of screened cuprotosis-related lncRNAs in AML samples from TCGA and normal controls from GTEx. (b) The expression levels of screened cuprotosis-related lncRNAs were validated in AML samples and healthy controls from Vizome. (c) The expression levels of screened cuprotosis-related lncRNAs were validated in the human AML cell line KG-1a, the acute monocytic leukaemia cell line THP-1, and the immortalized normal hepatocyte cell line LO2 by using qRT-PCR. (d–h) Prognostic significance of cuprotosis-related lncRNAs. *: p < 0.05, **: p < 0.01, ***: p < 0.001, ****: p < 0.0001; #: p < 0.05, ##: p < 0.01, ###: p < 0.001.
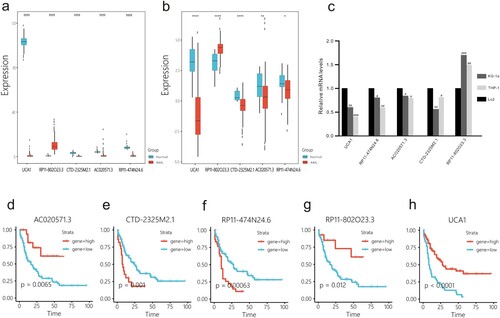
Construction and validation of prognostic risk model
A prognostic risk model for cuprotosis-related lncRNAs was constructed using the entire AML cohort. Patients were grouped into high- and low-risk groups according to the risk score and optimal cut-off point (0.56) ((a,b)), and risk score = (−0.040) × expression of AC020571.3 + (0.071) × expression of CTD-2325M2.1 + 0.029 × expression of RP11-474N24.6 + (−0.018) × expression of RP11-802O23.3 + (−0.005) × expression of UCA1. The model was capable of predicting prognosis in the entire AML cohort (P < 0.0001; (c)). The ROC curve showed that the model could better screen patients with poor prognosis with an AUC of 0.727 ((d)). The predictive power of the model was demonstrated using a time-dependent ROC curve ((e)).
Figure 3. Prognostic risk model was constructed based on LASSO Cox analysis in the training cohort. (a) The optimal cut-off value for this model was 0.27. (b) Risk plot between the high- and low-risk groups. (c) KM curve of the patients classified in high- and low-risk groups. (d) ROC for the training set. (e) Time-dependent ROC curve shows good performance of the model on 1-, 3-, and 5-year OS.
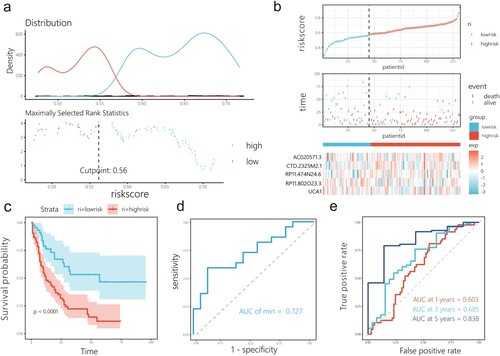
Using the caret package, the entire AML cohort in TCGA was randomly divided into internal and external training sets. In the internal training set, patients were grouped into high- and low-risk groups according to risk score ((a)). The KM curves showed that the high-risk groups had a worse prognosis than the low-risk groups (p < 0.0001; (b)). The ROC curves illustrate the differential ability of the model, with an AUC of 0.753 ((c)). The AUC of the prognostic risk model in the testing set for OS was 0.700 at 1 year, 0.856 at 3 years, and 0.809 at 5 years ((d)). Internal and external test sets were used to assess the predictive ability of the model ((e,i)). Similar to the findings from the internal training set, there was a significant difference in prognosis between the two groups in the internal and external testing sets, with the high-risk group having a worse prognosis ((f,j)), and features obtained through LASSO Cox analyses led to AUCs of 0.733 and 0.709 in the internal testing set and external testing set, respectively. ((g,k)). Additionally, the time-dependent ROC curve showed good performance of the model for 1, 3, and 5-year OS ((h,l)).
Figure 4. Prognostic risk model is tested in the internal training set and validated in the internal and external testing sets. (a, e, i) Risk plot between the high- and low-risk score groups in the internal training set, and internal and external testing sets. (b, f, j) KM curve shows high-risk score group has shorter OS than low-risk group in the internal training set, and internal and external testing sets. (c, g, k) ROC for the internal training set, and internal and external testing sets. (d, h, l) The time-dependent ROC was used to assess the performance for survival prediction in the internal training set, and internal and external testing sets.
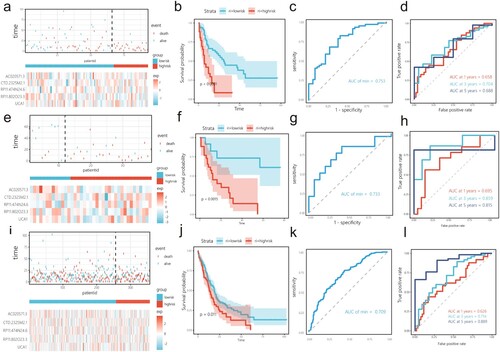
Clinical significance of the prognostic risk signature
Univariate and multivariate Cox analyses of the relationship between clinical variables and risk scores showed that age and risk score could be used as independent prognostic factors (). In the subgroup, age ≥ 60 occupied a relatively larger proportion in the high-risk group ((a)). Additionally, in the age subgroup, the high-risk group was associated with poor prognosis ((b,c)). The approximate survival rates at 1, 3, and 5 years for patients with AML were estimated by calculating the nomogram score ((d)). C-index = 0.680, and the time-dependent calibration curve and DCA confirmed the prediction superiority of the nomogram model ((e–h)).
Figure 5. Clinical correlations among cuprotosis-related lncRNAs included in the risk score and clinical characteristics. (a) Relationship between subgroup of age and risk score. The low-risk score group had a higher survival rate than the high-risk score group in age < 60 years (b) and age ≥ 60 (c). (d) A nomogram to quantitatively predict 1-, 3-, and 5-year survival. (e) Calibration curve for 1-, 3-, and 5-year OS plot to compare the actual event with the predicted event. An ideal calibration would lie along the 45° grey line. The clinical application value of the nomogram model was evaluated via decision curve analysis (DCA) at one year (f), three years (g), and five years (h).
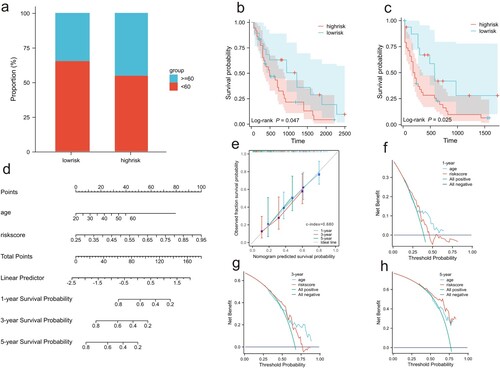
Figure 6. Underlying mechanisms related to risk score subgroup. (a) GSEA of the DEGs between the high- and low-risk groups. (b) KEGG enrichment analysis of DEGs between high-risk and normal samples, as well as between the low-risk group and normal sample.
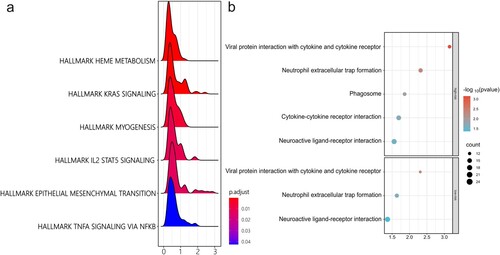
Table 1. Univariate and multivariate Cox analyses of the prognostic risk model.
Functional analyses result
To further investigate the biological processes involved in the prognostic risk model, GSEA was performed based on the logFC obtained from the results of the differential expression analyses between the low- and high-risk groups. The results showed that HALLMARK HEME METABOLISM, HALLMARK KRAS SIGNALING, HALLMARK MYOGENESIS, HALLMARK IL2 STAT5 SIGNALING, HALLMARK EPITHELIAL MESENCHYMAL TRANSITION, and HALLMARK TNFA SIGNALING VIA NFKB had different biological functions in the high- and low-risk groups ((a)).
Figure 7. Relationship between cuprotosis-related lncRNAs and mitochondria-associated pathways. (a) Relationship between cuprotosis-related lncRNAs and angiogenesis-associated genes. (b) The colour gradation from red to blue corresponds to positive and negative correlations. *p < 0.05, **p < 0.01.
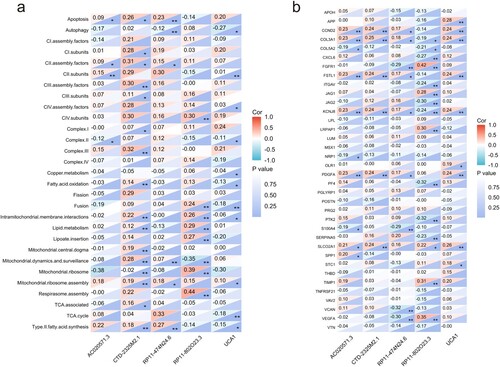
A total of 637 DEGs between the high-risk group and normal controls were screened and found to be enriched in viral protein interactions with cytokines and cytokine receptors, neutrophil extracellular trap formation, phagosomes, cytokine-cytokine receptor interactions, and neuroactive ligand-receptor interactions. A total of 658 DEGs were screened between the low-risk group and normal controls, which were enriched in viral protein interactions with cytokines and cytokine receptors, neutrophil extracellular trap formation, and neuroactive ligand-receptor interactions ((b)).
Molecular features of cuprotosis-related lncRNA
Given that copper is significant in the process of neoplastic angiogenesis and that results from GSEA indicated that the prognostic risk model is associated with angiogenesis, we explored the relationship between angiogenesis-associated genes and cuprotosis-related lncRNAs. Angiogenesis-associated genes were obtained from the hallmark gene set (MSigDB). The results showed that angiogenesis-associated genes, including CCND2, COL3A1, FSTL1, KCNJ8, and PDGFA, were positively related to AC020571.3, RP11-474N24.6, CTD-2325M2.1, and UCA1, and negatively related to RP11-802O23.3 ((a)).
Figure 8. Different distributions of somatic mutations between low- and high-risk groups. (a and b) Mutation profile of low-risk group. (c and d) Mutation profile of high-risk group.
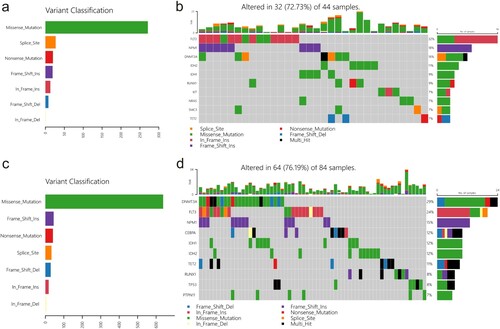
A correlation analysis between mitochondria-associated pathways and screened lncRNAs showed that there is significant link between mitochondrial function and five cuprotosis-related lncRNAs. CTD-2325M2.1 was positively related to most of mitochondrial functions and most significantly related to complex III (r = 0.32, p < 0.01), CIII assembly factors (r = 0.30, p < 0.01), mitochondrial dynamics and surveillance (r = 0.28, p < 0.01), intramitochondrial membrane interactions (r = 0.22, p < 0.01), mitochondrial ribosome assembly (r = 0.19, p < 0.01), mitochondrial central dogma (r = 0.19, p < 0.01), type II fatty acid synthesis (r = 0.18, p < 0.01), lipid metabolism (r = 0.12, p < 0.01), and fatty acid oxidation (r = 0.14, p < 0.01) ((b)).
Result of immune mutational analysis, infiltration analysis, and drug sensitivity analysis
‘Maftools’ package was utilised to analyse the mutational landscape differences between the low- and high-risk groups; the result revealed that the frameshift insertion of FLT3 is observed in the low-risk group ((a,b)), and missense mutation of DNMT3A is frequent in the high-risk score group ((c,d)). Immune infiltration analysis indicated significant differences in Th1 cell, GMP, and CMP levels between the high- and low-risk groups ((a)). Furthermore, drug response prediction was performed using the R package ‘pRRophetic’. The results demonstrated that WH-4.023 and mitomycin C decreased the IC50 values in the high-risk group compared to those in the low-risk group, whereas navitoclaxin and PD-0325901 decreased the IC50 values in the low-risk group compared to those in the high-risk group ((b)).
Discussion
LncRNAs are widely involved in leukaemogenesis. In clinical practice, a prognostic risk model based on lncRNA signature gene expression levels with high discrimination power is required to effectively predict the prognosis of individual patients [Citation23]; however, a single lncRNA biomarker often lacks diagnostic and prognostic predictive value [Citation24]. A prognostic model that integrates multiple genes synergistically interacting in biological processes has the potential to further improve prediction accuracy. Copper plays a crucial role as a co-factor in many enzymes involved in maintaining the proper functions of the body [Citation25]. Imbalances in copper homeostasis and metabolic abnormalities cause a range of disorders [Citation26]. Copper concentration exceeding a safe dispensing threshold leads to cell death, a process known as cuprotosis [Citation11]. Previous studies have reported a cuprotosis-related lncRNA prognostic risk model, a predictor of survival in tumours [Citation17,Citation18]; however, cuprotosis-related lncRNA models based on lncRNAs in AML are limited. Based on previous findings on cuprotosis and the functions of lncRNAs, a risk prediction model was constructed to predict the prognosis of patients with AML and explored the relationship between cuprotosis-related lncRNAs and clinical characteristics of AML, as well as the underlying mechanism of the model.
Five cuprotosis-related lncRNAs (AC020571.3, CTD-2325M2.1, RP11-802O23.3, RP11-474N24.6, and UCA1) exhibiting prognostic importance and dysregulation in AML were identified. Based on these findings, a risk prediction model was developed and evaluated in an internal training set, and validated in both internal and external testing sets. Distinct mutational landscapes were observed across different groups, with frequent missense mutations in DNMT3A observed in the high-risk group, and nonsense mutations in FLT3 detected in the low-risk group. Univariate and multivariate Cox regression analyses involving the risk score and clinicopathological factors demonstrated the independent prognostic value of age and the risk score. The nomogram and calibration curve exhibited consistent predictions of survival rates at 1, 3, and 5 years. AML predominantly affects older patients (≥60 years) with a median age of 67 years, and the incidence of AML increases with age [Citation27]. Furthermore, older age often indicates poorer outcomes [Citation28]. The older age group exhibited a higher proportion of high-risk samples than the low-risk group, indicating a positive correlation between the risk score and age. A higher risk score corresponded to a more malignant phenotype. Notably, within the subgroup aged ≥60 years, the high-risk category correlated with shorter survival time than the subgroup aged <60 years, underscoring the model's importance in predicting prognosis for patients aged ≥60 years. Drug response prediction revealed the sensitivity of the low-risk group to BCL-2 and BCL-XL inhibitor navitoclaxin, as well as the MEK inhibitor PD-0325901. Conversely, the high-risk group exhibited sensitivity to WH-4.023 and mitomycin C. Extensive studies have demonstrated the promising efficacy of navitoclaxin in small cell lung cancer and acute lymphocytic leukaemia (ALL) [Citation29]. Mitomycin C, an antitumour antibiotic, functions as a bifunctional alkylating agent [Citation30]. WH-4.023, a potent and selective Lck and Src inhibitor, induces cell death in glucocorticoid-resistant T-ALL cells, with co-treatment with dexamethasone effectively reversing GC resistance [Citation31].
Furthermore, the mechanisms described by the model were explored. Among the screened lncRNAs, UCA1, the first identified in bladder transitional cell carcinoma [Citation32], has been shown to promote autophagy [Citation33] and repress the expression of the cell cycle regulators p27kip1 [Citation34], CXCR4, and CYP1B1 [Citation35] to sustain AML cell proliferation. Additionally, UCA1 acts as an miRNA sponge to regulate the miR-126 [Citation32] and miR-296-3p/Myc axes [Citation33] to modulate AML cell proliferation. Targeting UCA1 has the potential to be a novel strategy for overcoming drug resistance, as UCA1 overcomes the chemoresistance of paediatric AML by suppressing glycolysis [Citation36] and increases the resistance of leukaemia cells to daunorubicin by regulating the PI3K/AKT pathway [Citation37]. AC020571.3, CTD-2325M2.1, RP11-802O23.3, and RP11-474N24.6 have never been investigated previously; however, GSEA revealed that groups with high- and low-risk scores were significantly different in some immune-related biological processes and angiogenesis. CTD-2325M2.1 positively related to angiogenesis-associated genes (CCND2, COL3A1, FSTL1, KCNJ8, and PDGFA). Copper and angiogenesis are closely associated, and copper affects tumour progression by affecting angiogenesis, metastasis, and other processes [Citation38]. Thus, CTD-2325M2.1 is not only involved in cuprotosis, but also in angiogenesis. Mitochondria are the primary target of copper toxicity [Citation39], resulting in oxidative stress, impairment of the electron transport chain, and ultimately, apoptosis of cells with mitochondrial overload [Citation40]. CTD-2325M2.1 is related to most mitochondrial functions and most significantly related to complex III and CIII assembly factors, mitochondrial dynamics and surveillance, intramitochondrial membrane interactions, and mitochondrial ribosome assembly. CTD-2325M2.1 has been shown to play a crucial role in cuprotosis progression and has the potential to be involved in both mitochondria function and angiogenesis. Specifically, neutrophil extracellular trap formation was a common biological process related to the high- and low-risk groups. Activated neutrophils release fibrous structures known as neutrophil extracellular traps, which act as host defence mechanisms and protect immune cells and pathogens through physical barriers [Citation41]. In malignant tumours, the extracellular trap of neutrophils is regarded as a double-edged sword [Citation42]. Neutrophils are attracted to tumour microenvironments through chemokines released by cancer cells and are called tumour-associated neutrophils (TANs) [Citation43]. TANs can polarize into N1 and N2 populations, N1 exerts antineoplastic effects via indirect cytotoxicity and induces tumour cell apoptosis [Citation44]. N2 supports tumour growth, invasion, angiogenesis, and metastasis of cancer cells [Citation45]. Given the significant relationship between the risk score and immune-related biological processes based on the results of the above studies, copper is reportedly related to normal lymphocyte maturation and immune function regulation [Citation46], and differences in immune infiltration were observed between high- and low-risk groups. Elevated Th1 cell numbers were observed in the high-risk group. Elevated Th1 cell levels promote apoptosis and inhibit the proliferation of leukaemia cells via IFN-γ secretion, both in vitro and in vivo [Citation47]. Low levels of Th1 cells were detected in the high-risk group, suggesting a potential relationship between the risk score and the malignant phenotype.
In this study, a prognostic model based on lncRNAs was developed, and the inclusion of five cuprotosis-related lncRNAs in the classification demonstrated the ability to predict clinical recurrence in the internal validation cohort and independent external cohort. However, there are certain limitations to consider. Owing to the scarcity of relevant clinical data, all essential clinical variables into our predictive model were unable to incorporated. Furthermore, the prognostic power of the risk models may be diminished due to the limited size of the external validation set. Future studies with a larger sample size and comprehensive clinical data will help optimize and refine this predictive model. Additionally, our screening identified CTD-2325M2.1 as a cuprotosis-related lncRNA involved in mitochondrial function and angiogenesis. Nonetheless, further experimental investigations, including overexpression and knockdown experiments, are necessary to validate its impact on mitochondrial function and angiogenesis-related phenotypes.
Conclusions
Five cuprotosis-related lncRNA signatures were screened for prognostic risk model construction, which showed good predictive performance and discrimination ability, and provided a novel method for predicting the prognosis for patients with AML.
Authors’ contributions
Q.W. conceived the study. Q.W. contributed to the data acquisition and analyses. Q.W. drafted the manuscript.
Acknowledgements
I would like to thank those who made their invaluable data publicly available.
Disclosure statement
No potential conflict of interest was reported by the author(s).
References
- Short NJ, Rytting ME, Cortes JE. Acute myeloid leukaemia. Lancet. 2018;392(10147):593-606. doi:10.1016/S0140-6736(18)31041-9
- Riva L, Luzi L, Pelicci PG. Genomics of acute myeloid leukemia: the next generation. Front Oncol. 2012;2:40. doi:10.3389/fonc.2012.00040
- Zhang R, Xia LQ, Lu WW, Zhang J, Zhu JS. LncRNAs and cancer (Review). Oncol Lett. 2016;12(2):1233-1239. doi:10.3892/ol.2016.4770
- Tan YT, Lin JF, Li T, et al. LncRNA-mediated posttranslational modifications and reprogramming of energy metabolism in cancer. Cancer Commun (Lond). 2021;41(2):109–120. doi:10.1002/cac2.12108
- Schwerdtfeger M, Desiderio V, Kobold S, et al. Long non-coding RNAs in cancer stem cells. Transl Oncol. 2021;14(8):101134. doi:10.1016/j.tranon.2021.101134
- Alvarez-Dominguez JR, Lodish HF. Emerging mechanisms of long noncoding RNA function during normal and malignant hematopoiesis. Blood. 2017;130(18):1965-1975. doi:10.1182/blood-2017-06-788695
- Dahariya S, Paddibhatla I, Kumar S, et al. Long non-coding RNA: classification, biogenesis and functions in blood cells. Mol Immunol. 2019;112:82–92. doi:10.1016/j.molimm.2019.04.011
- Gourvest M, Brousset P, Bousquet M. Long noncoding RNAs in acute myeloid leukemia: functional characterization and clinical relevance. Cancers (Basel). 2019;11(11):1638. doi:10.3390/cancers11111638
- Banci L, Bertini I, Cantini F, et al. Cellular copper distribution: a mechanistic systems biology approach. Cell Mol Life Sci. 2010;67(15):2563–2589. doi:10.1007/s00018-010-0330-x
- Scheiber I, Dringen R, Mercer JF. Copper: effects of deficiency and overload. Met Ions \Life Sci. 2013;13:359-387. doi:10.1007/978-94-007-7500-8_11
- Tsvetkov P, Coy S, Petrova B, Dreishpoon M, Verma A, Abdusamad M, Rossen J, Joesch-Cohen L, Humeidi R, Spangler RD, Eaton JK, Frenkel E, Kocak M, Corsello SM, Lutsenko S, Kanarek N, Santagata S, Golub TR. Copper induces cell death by targeting lipoylated TCA cycle proteins. Science. 2022;375(6591):eabq4855. doi:10.1126/science.abf0529
- Brady DC, Crowe MS, Turski ML, et al. Copper is required for oncogenic BRAF signalling and tumorigenesis. Nature. 2014;509(7501):492–6. doi:10.1038/nature13180
- Li Y. Copper homeostasis: emerging target for cancer treatment. IUBMB Life. 2020;72(9):1900–1908. doi:10.1002/iub.2341
- De Luca A, Barile A, Arciello M, et al. Copper homeostasis as target of both consolidated and innovative strategies of anti-tumor therapy. J Trace Elem Med Biol. 2019;55:204–213. doi:10.1016/j.jtemb.2019.06.008
- Kaiafa GD, Saouli Z, Diamantidis MD, Kontoninas Z, Voulgaridou V, Raptaki M, Arampatzi S, Chatzidimitriou M, Perifanis V. Copper levels in patients with hematological malignancies. Eur J Intern Med. 2012;23(8):738-741. doi:10.1016/j.ejim.2012.07.009
- Zuo XL, Chen JM, Zhou X, et al. Levels of selenium, zinc, copper, and antioxidant enzyme activity in patients with leukemia. Biol Trace Elem Res. 2006;114(1-3):41–54. doi:10.1385/BTER:114:1:41
- Han J, Hu Y, Liu S, et al. A newly established cuproptosis-associated long non-coding RNA signature for predicting prognosis and indicating immune microenvironment features in soft tissue sarcoma. J Oncol. 2022;2022:1. doi:10.1155/2022/8489387
- Yang L, Yu J, Tao L, et al. Cuproptosis-Related lncRNAs are biomarkers of prognosis and immune microenvironment in head and neck squamous cell carcinoma. Front Genet. 2022;13:947551. doi:10.3389/fgene.2022.947551
- Goldman MJ, Craft B, Hastie M, et al. Visualizing and interpreting cancer genomics data via the Xena platform. Nat Biotechnol. 2020;38(6):675–678. doi:10.1038/s41587-020-0546-8
- Raudvere U, Kolberg L, Kuzmin I, et al. g:Profiler: a web server for functional enrichment analysis and conversions of gene lists (2019 update). Nucleic Acids Res. 2019;47(W1):W191–W198. doi:10.1093/nar/gkz369
- Rath S, Sharma R, Gupta R, et al. MitoCarta3.0: an updated mitochondrial proteome now with sub-organelle localization and pathway annotations. Nucleic Acids Res. 2021;49(D1):D1541–D1547. doi:10.1093/nar/gkaa1011
- Aran D, Hu Z, Butte AJ. xCell: digitally portraying the tissue cellular heterogeneity landscape. Genome Biol. 2017;18(1):220), doi:10.1186/s13059-017-1349-1
- Wan J, Guo C, Fang H, et al. Autophagy-related long non-coding RNA is a prognostic indicator for bladder cancer. Front Oncol. 2021; 11:647236. doi:10.3389/fonc.2021.647236
- Wu B, Wang K, Fei J, Bao Y, Wang X, Song Z, Chen F, Gao J, Zhong Z. Novel three-lncRNA signature predicts survival in patients with pancreatic cancer. Oncol Rep. 2018;40(6):3427-3437. doi:10.3892/or.2018.6761
- Grubman A, White AR. Copper as a key regulator of cell signalling pathways. Expert Rev Mol Med. 2014;16:e11. doi:10.1017/erm.2014.11
- Ruiz LM, Libedinsky A, Elorza AA. Role of copper on mitochondrial function and metabolism. Front Mol Biosci. 2021;8:711227. doi:10.3389/fmolb.2021.711227
- Edmund J, Thaliath LJ, Meleveedu K. Acute myeloid leukemia in the medically unfit elderly patients. Leukemia Res 2023; 130:107306. doi:10.1016/j.leukres.2023.107306
- Fleischmann M, Scholl S, Frietsch JJ, et al. Clinical experience with venetoclax in patients with newly diagnosed, relapsed, or refractory acute myeloid leukemia. J Cancer Res Clin Oncol. 2022;148(11):3191–3202. doi:10.1007/s00432-022-03930-5
- Mohamad Anuar NN, Nor Hisam NS, Liew SL, Ugusman A. Clinical review: navitoclax as a pro-apoptotic and anti-fibrotic agent. Front Pharmacol. 2020;11:564108. doi:10.3389/fphar.2020.564108
- Bradner WT. Mitomycin C: a clinical update. Cancer Treat Rev. 2001;27(1):35–50. doi:10.1053/ctrv.2000.0202
- Serafin V, Capuzzo G, Milani G, et al. Glucocorticoid resistance is reverted by LCK inhibition in pediatric T-cell acute lymphoblastic leukemia. Blood 2017; 130(25):2750–2761. doi:10.1182/blood-2017-05-784603
- Wang XS, Zhang Z, Wang HC, et al. Rapid identification of UCA1 as a very sensitive and specific unique marker for human bladder carcinoma. Clin Cancer Res. 2006;12(16):4851–4858. doi:10.1158/1078-0432.CCR-06-0134
- Li JJ, Chen XF, Wang M, et al. Long non-coding RNA UCA1 promotes autophagy by targeting miR-96-5p in acute myeloid leukaemia. Clin Exp Pharmacol Physiol. 2020;47(5):877–885. doi:10.1111/1440-1681.13259
- Hughes JM, Legnini I, Salvatori B, et al. C/EBPα-p30 protein induces expression of the oncogenic long non-coding RNA UCA1 in acute myeloid leukemia. Oncotarget. 2015;6(21):18534–18544. doi:10.18632/oncotarget.4069
- Li J, Li Z, Bai X, et al. Lncrna UCA1 promotes the progression of AML by upregulating the expression of CXCR4 and CYP1B1 by affecting the stability of METTL14. J Oncol. 2022;2022:1. doi:10.1155/2022/2756986
- Zhang Y, Liu Y, Xu X. Knockdown of LncRNA-UCA1 suppresses chemoresistance of pediatric AML by inhibiting glycolysis through the microRNA-125a/hexokinase 2 pathway. J Cell Biochem. 2018;119(7):6296–6308. doi:10.1002/jcb.26899
- Yao Q, Zhang L, Wang Y, Liu J, Yang L, Wang Y. LncRNA UCA1 elevates the resistance of human leukemia cells to daunorubicin by the PI3K/AKT pathway via sponging miR-613. Biosci Rep. 2021;41(6):BSR20201389. doi:10.1042/BSR20201389
- Wang Z, Jin D, Zhou S, Dong N, Ji Y, An P, Wang J, Luo Y, Luo J. Regulatory roles of copper metabolism and cuproptosis in human cancers. Front Oncol. 2023;13:1123420. doi:10.3389/fonc.2023.1123420
- Mehta R, Templeton DM, O'brien PJ. Mitochondrial involvement in genetically determined transition metal toxicity. Chem Biol Interact. 2006;163(1-2):77-85. doi:10.1016/j.cbi.2006.05.011
- Vriens A, Nawrot TS, Janssen BG, Baeyens W, Bruckers L, Covaci A, De Craemer S, De Henauw S, Den Hond E, Loots I, Nelen V, Schettgen T, Schoeters G, Martens DS, Plusquin M.. Exposure to environmental pollutants and their association with biomarkers of aging: a multipollutant approach. Environ Sci Technol. 2019;53(10):5966-5976. doi:10.1021/acs.est.8b07141
- Pruchniak MP, Demkow U. Potent NETosis inducers do not show synergistic effects in vitro. Cent Eur J Immunol. 2019;44(1):51-58. doi:10.5114/ceji.2019.84017
- Demkow U. Neutrophil extracellular traps (NETs) in cancer invasion, evasion and metastasis. Cancers (Basel). 2021;13(17):4495. doi:10.3390/cancers13174495
- Masucci MT, Minopoli M, Carriero MV. Tumor associated neutrophils. their role in tumorigenesis, metastasis, prognosis and therapy. Front Oncol. 2019;9:1146. doi:10.3389/fonc.2019.01146
- Lee J, Lee D, Lawler S, et al. Role of neutrophil extracellular traps in regulation of lung cancer invasion and metastasis: structural insights from a computational model. PLoS Comput Biol. 2021;17(2):e1008257. doi:10.1371/journal.pcbi.1008257
- Shaul ME, Fridlender ZG. Cancer-related circulating and tumor-associated neutrophils – subtypes, sources and function. FEBS J. 2018;285(23):4316–4342. doi:10.1111/febs.14524
- Percival SS. Copper and immunity. Am J Clin Nutr. 1998;67(5 Suppl):1064S-1068S. doi:10.1093/ajcn/67.5.1064S
- Liu Q, Hua M, Zhang C, et al. NLRP3-activated bone marrow dendritic cells play antileukemic roles via IL-1β/Th1/IFN-γ in acute myeloid leukemia. Cancer Lett. 2021;520:109–120. doi:10.1016/j.canlet.2021.06.014