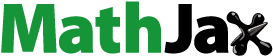
ABSTRACT
Objective
This study aims to construct a nomogram model for predicting poor prognosis in acute leukemia with trisomy 8.
Methods
A retrospective analysis was conducted on 244 patients with primary acute leukemia with trisomy 8 who received treatment in our hospital, and they were randomly divided into the modeling group (122 cases, including 78 cases with good prognosis and 44 cases with poor prognosis) and the verification group (122 cases). R software was used to construct a nomogram model for predicting poor prognosis in acute leukemia with trisomy 8.
Results
The results of multivariate analysis showed that age >51 years old, and white blood cell count ≤20 × 109/L were risk factors for poor prognosis in acute leukemia patients with trisomy 8 (P < 0.05), and chemotherapy + transplantation was a protective factor for poor prognosis in acute leukemia patients with trisomy 8 (P < 0.05). The nomogram for poor prognosis of acute leukemia patients with trisomy 8 was constructed using the above four risk predictors. The Hosmer-Lemeshow goodness of fit test was = 6.371, P = 0.497, and the area under the ROC curve was 0.817 (95%CI: 0.742-0.892). The slope of the calibration curve of external validation was close to 1, Hosmer-Lemeshow goodness of fit test was = 6.507, P = 0.448, and the area under the ROC curve was 0.834 (95%CI: 0.748-0.921).
Conclusion
The nomogram model constructed in this study for predicting poor prognosis among acute leukemia patients with trisomy 8 demonstrates excellent discrimination and consistency.
1. Introduction
Acute leukemia, a malignancy caused by massive malignant proliferation of immature myeloid hematopoietic stem cells, can infiltrate lymph nodes, liver and other tissues and organs, inhibit normal hematopoiesis in the body, and decrease blood cell production [Citation1, Citation2]. Chromosomal abnormalities play an important role in hematological malignancies, including chromosomal number abnormalities and structural abnormalities. Some studies have shown that chromosomal abnormalities are the basis for the development of acute leukemia [Citation3]. Trisomy 8 is a frequently observed chromosomal abnormality in cases of acute leukemia [Citation4]. It has been shown that cytogenetic heterogeneity has a significant impact on the prognosis of acute leukemia patients, with complete remission achieved in 90% cases of acute leukemia children, but the overall survival rate of patients is about 70% due to a high relapse rate [Citation5, Citation6]. Therefore, it is clinically important to make early prediction of the prognosis of acute leukemia patients with trisomy 8. Nomogram is a visual clinical prediction model that has been used for the early prediction of prognosis in acute myeloid leukemia patients with biallelic CEBPA mutation [Citation7]. Therefore, we retrospectively collected clinical data of 244 primary acute leukemia patients with trisomy 8 admitted to our hospital between January 2014 and January 2020, explored the risk factors affecting patients’ prognosis, and constructed an individualized nomogram model for predicting the prognosis of acute leukemia patients with trisomy 8. The purpose of this study is to establish a theoretical foundation for prognostic risk prediction in acute leukemia patients with trisomy 8, validate the clinical applicability of the model, and offer guidance for the development of targeted sex therapy programs. Ultimately, these efforts aim to mitigate the occurrence of unfavorable prognoses.
2. Data and methods
2.1. Research object
A retrospective collection was conducted on a total of 244 primary acute leukemia patients with trisomy 8 who received treatment at our hospital between January 2014 and January 2020. The study cohort consisted of 139 males and 105 females, ranging in age from 30 to 75 years, with a mean age of (51.72 ± 9.65) years. The study was approved by the ethics committee of our hospital and gained informed consent from the patients and their families. Using the random number table method, 244 patients were randomly divided into modeling group (122 cases) and validation group (122 cases) according to a 1:1 ratio.
Inclusion criteria were as follows: ① confirmation of acute leukemia diagnosis (including acute myeloid leukemia and acute lymphoblastic leukemia) through MICM typing diagnosis (cytogenetics, morphology, immunology, molecular biology), with diagnostic criteria based on the 2017 CAP/ASH guidelines [Citation8]; ② availability of complete clinical data after admission; ③ no history of antibiotic usage in the month prior to admission. Exclusion criteria were: ① presence of combined liver and renal dysfunction; ② presence of other concomitant malignancies; ③ presence of psychiatric abnormalities; ④ M3 typing in patients with acute myeloid leukemia ().
2.2. Clinical data collection
Age, gender, peripheral blood primitive cell ratio at initial diagnosis, bone marrow primitive cell ratio, disease remission status after the first induction, white blood cell count, neutrophil count, lymphocyte, platelet count, hemoglobin, lactate dehydrogenase (positive /negative), albumin level, and risk stratification were collected from the enrolled patients, and information about the patients’ subsequent treatment modalities was also collected.
2.3. Methods
2.3.1. Sample collection
Bone marrow samples of 2–4 ml were collected from patients prior to treatment, with the specific amount extracted depending on the peripheral blood leukocyte count of each patient.
2.3.2. Cytogenetic analysis
The collected bone marrow cells were cultured for 18∼24 h. Cells at mitosis metaphase were collected, processed and fixed, and karyotype analysis was performed using the chromosomal R banding technique, with approximately 20 mid-mitotic phases analyzed. Chromosomes were analyzed and described according to the International System for the Designation of Human Cytogenetics (ISCN 2013).
2.4. Patient treatment regimen and follow-up
For chemotherapy regimens, patients with acute myeloid leukemia received intravenous infusion of daunorubicin, 25–45 mg/m2 on d1∼d3; received intravenous infusion of cytarabine 100 mg/m2 on d1∼d7. Patients with acute lymphoblastic leukemia received intravenous infusion of vincristine 1.5 mg/m2 on dl, d8, d15, d22, received intravenous infusion of daunorubicin, 30∼40 mg/m2 on dl∼d3, d15∼d17; orally took prednisone 40∼60 mg/m2 on dl∼d14 (dose was reduced from dl5 and the drug was discontinued on d28). The induction remission status was evaluated in both groups after 1 course of treatment regimen. Patients undergoing transplantation were treated with hematopoietic stem cell transplantation after the disease remission.
Complete remission (CR): ① the patient's clinical symptoms disappeared; ② the absolute value of neutrophils in peripheral blood was > l.6 × 109/L; ③ the platelet count was >100 × 109/L; ④ the content of promyelocytes and myeloblasts in bone marrow was less than 5%. Partial remission (PR): one of the complete remission items did not meet the corresponding criteria. Non-remission (NP): None of the 4 criteria in complete remission were met. Incomplete remission was PR + NP. Follow-up was performed by case query or phone call for a maximum of 3 years until follow-up cutoff on 1 February 2023. Overall survival time started from initiation of treatment to patient death or follow-up cutoff. The modeling group was divided into 78 cases with a good prognosis and 44 cases with a poor prognosis based on the patient prognosis at the time of follow-up, while the validation group was divided into 74 cases with a good prognosis and 40 cases with a poor prognosis. Good prognosis was determined if patients gained CR after treatment without recurrence or disease deterioration.
2.5. Statistical methods
SPSS 21.0 was used to process the data, and the count data were described by n. The test was used for comparison between the two groups. The Kaplan-meier method was used to fit the survival curves, and the Log-rank method was used for comparison between groups. The COX proportional risk model was used to screen the risk factors affecting the poor prognosis of acute leukemia patients with trisomy 8. The identified risk factors were introduced into R 3.6.3 software and the rms package to build nomogram model for predicting poor prognosis in acute leukemia patients with trisomy 8. The Hosmer-Lemeshow goodness of fit test and calibration curves were used to assess consistency, and ROC curves were plotted to assess the discrimination. Differences were considered statistically significant if P < 0.05.
3. Results
3.1. Univariate analysis of poor prognosis in acute leukemia patients with trisomy 8
A univariate analysis was performed on the data of 44 patients with poor prognosis and 78 patients with good prognosis in the modeling group. The results showed that the proportion of age >51 years, incomplete disease remission after the first induction, white blood cell count ≤20 × 109/L, ALB < 35 g/L, high risk stratification and chemotherapy alone were significantly higher in the poor prognosis group than in the good prognosis group (P < 0.05), as shown in .
Table 1. Univariate analysis of poor prognosis in acute leukemia patients with chromosome 8 abnormalities.
3.2. Multivariate COX regression analysis of poor prognosis in acute leukemia patients with trisomy 8
Whether poor prognosis occurred in acute leukemia patients with trisomy 8 was used as the dependent variable (good prognosis = 0, poor prognosis = 1), and the statistically significant indicators in univariate analysis (age, disease remission status after the first induction, white blood cell count, treatment modality, albumin < 35 g/L, and risk stratification) were used as independent variables, with variable assignment shown in . The results of multivariate analysis showed that age > 51 years, incomplete disease remission after the first induction, white blood cell count ≤ 20 × 109/L were risk factors for poor prognosis in acute leukemia patients with trisomy 8 (P < 0.05), and chemotherapy + transplantation was a protective factor for poor prognosis in acute leukemia patients with trisomy 8 (P < 0.05), as shown in .
Table 2. Variable assignment methods.
Table 3. Multivariate analysis of poor prognosis in acute leukemia patients with chromosome 8 abnormalities.
3.3. Survival analysis of the factors
In this study, age, disease remission status after the first induction, white blood cell count, and treatment modality were found to be correlated with survival in acute leukemia patients with trisomy 8 ().
3.4. Establishment of nomogram model to predict poor prognosis in acute leukemia patients with trisomy 8
A nomogram prediction model was constructed for poor prognosis in acute leukemia patients with trisomy 8 using R software, as shown in . The impact of each factor on the poor prognosis of acute leukemia patients with trisomy 8 was presented in the form of score, with 86.5 for age >51 years, 77.5 for incomplete remission after the first induction, 89 for white blood cell count ≤ 20 × 109/L, and 100 for chemotherapy alone. If one patient was >51 years old (86.5 points), had incomplete disease remission after the first induction (77.5 points), had a white blood cell count >20 × 109/L (0 points) and had chemotherapy alone (100 points), then the patient had a total score of 264. A vertical line was made at the total score coordinate of 264, corresponding to a predicted probability of approximately 60%, then the acute leukemia patient with trisomy 8 had a predicted value of 60% for poor prognosis.
3.5. Internal validation of the nomogram model for predicting poor prognosis in acute leukemia patients with trisomy 8
The internal validation results of the nomogram model showed that the predicted values on calibration curve of the nomogram prediction model (as shown in (A)) for poor prognosis in acute leukemia patients with trisomy 8 was in good agreement with the actual values. In Hosmer-Lemeshow goodness-of-fit test, = 6.371, P = 0.497, showing good consistency. The area under the ROC curve was 0.817 (95% CI: 0.742∼0.892) with a good discrimination, as shown in (B).
3.6. External validation of the nomogram model for predicting poor prognosis in acute leukemia patients with trisomy 8
The external validation results of the nomogram model are displayed in the calibration curve of the nomogram prediction model, as shown in (A). In Hosmer-Lemeshow goodness-of-fit test, = 6.507, P = 0.448, showing good agreement between the actual risk and risk of poor prognosis in acute leukemia patients with trisomy 8 as predicted by the model. The area under the ROC curve for external validation was 0.834 (95% CI: 0.748∼0.921) with a good discrimination, as shown in (B).
4. Discussion
Acute leukemia is a common cancer in children and adults under 35 years of age, which has a high degree of heterogeneity. So far, there are more than 2 million children with leukemia in China, and the number of new cases continues to grow at a rate of 30,000–40,000 per year [Citation9]. Complex chromosome abnormalities have been reported in most leukemia patients [Citation10]. Acute leukemia is characterized by cytogenetic and molecular biological abnormalities and patients have varying susceptibility to treatment, leading to different prognosis in acute leukemia patients [Citation11]. Chromosome 8 contains multiple genetic disease pathogenic genes, accounting for approximately 1.5% of the total number of genes, 16% of which are associated with cancer development [Citation12]. Trisomy 8 means common cytogenetic alterations in acute leukemia, with an incidence of 10%∼15%. Chromosome 8 is strongly associated with alterations in DNA methylation genes, spliceosome complex genes and myeloid transcription factor genes in acute leukemia, and these alterations may have a significant impact on the development and prognosis of leukemia [Citation13]. Studies in the past decades have shown that survival rates of acute leukemia patients have not improved, with an overall 5-year survival rate of about 27% in adult patients. Although acute myeloid leukemia patients are treated with standard treatments such as anthracyclines and cytarabine therapy, it is difficult to treat patients with relapsed and refractory acute myeloid leukemia, with a 3-year survival rate below 10% for patients with relapsed/refractory acute myeloid leukemia [Citation14, Citation15].
The present study showed that the proportion of patents aged >51 years was significantly higher in the poor prognosis group than in the good prognosis group. Studies have shown that 60%∼85% of adults under 60 years of age can achieve complete remission after effective treatment, but in older patients over 60 years of age, the complete remission rate is only 40%∼60% [Citation16]. The 5-year overall survival rate for young and middle-aged acute myeloid leukemia patients is <50%, compared with only about 10% for acute myeloid leukemia patients over 60 years of age [Citation17]. This study showed that the proportion of incomplete disease remission after the first induction, the proportion of white blood cell count ≤ 20 × 109/L, and the proportion of chemotherapy alone were significantly higher in the poor prognosis group than in the good prognosis group. Chemotherapy is a common clinical treatment modality for acute leukemia, but even when patients achieve CR of their disease, the disease-free survival rate is only 40%∼50%. Clinicians believe that this outcome is associated with the development of chemotherapy resistance in patients [Citation18]. In addition, chemotherapy may lead to acute complications in patients with acute myeloid leukemia. Allogeneic stem cell transplantation is an appropriate approach to reduce the leukemia relapse risk compared to standard chemotherapy, though it increases the risk of serious complications [Citation19]. It has also been shown that white blood cell count is an important predictor of prognosis in patients with acute myeloid leukemia [Citation20].
In this study, multivariate COX regression analysis showed that age >51 years, incomplete disease remission after the first induction, and white blood cell count ≤ 20 × 109/L were risk factors for poor prognosis in acute leukemia patients with trisomy 8, and chemotherapy + transplantation was a protective factor for poor prognosis in acute leukemia patients with trisomy 8. In this study, we constructed a nomogram model for predicting poor prognosis in acute leukemia patients with trisomy 8 based on four influencing factors: age, disease remission status after the first induction, white blood cell count, and treatment modality. It was found by internal and external validation that the risk of poor prognosis predicted by the model was in good agreement with the actual incidence risk and the model demonstrated good discrimination. Studies have shown that nomogram may quantify the impact of influencing factors on poor prognosis in acute leukemia patients with trisomy 8 in a more accurate and convenient way. So far, nomogram has been used to estimate survival rates in acute leukemia children [Citation20]. Nomogram shows that age >51 years, incomplete disease remission after the first induction, white blood cell count ≤ 20 × 109/L, and chemotherapy alone contribute 86.5, 77.5, 89, and 100 points of impact weight to poor prognosis in acute leukemia patients with trisomy 8, respectively.
5. Conclusion
In conclusion, based on age, disease remission status after the first induction, white blood cell count, and treatment modality, this study constructs a nomogram model regarding poor prognosis in acute leukemia patients with trisomy 8. With good consistency, discrimination, and clinical applicability, the model provides guidance for clinical identification of acute leukemia patients with trisomy 8 who are at high risk of poor prognosis.
Declaration of conflicting interests
Authors declared no conflict of interest.
Consent for publication
All authors give consent for publication
Author contributions
Tianxia Deng:Project development, Data Collection, Manuscript writing. Sheng Wang and Qiulian Liu: Data collection. Zhuonan Ran: Data analysis. Mingxia Wang: Project development, Data collection, Data analysis,Manuscript editing.
Research involving human participants and/or animal
This retrospective study involving human participants was in accordance with the ethical standards of the institutional research committee and with the 1964 Helsinki Declaration and its later amendments or comparable ethical standards. The study was approved by our institution’s ethics review board.
Disclosure statement
No potential conflict of interest was reported by the authors.
Data availability statement
The [DATA TYPE] data used to support the findings of this study are included within the article.
References
- Pemberton-Whiteley Z, Nier S, Geissler J, et al. Understanding quality of life in patients With acute leukemia, a global survey. J Patient Cent Res Rev. 2023;10(1):21–30. doi:10.17294/2330-0698.1951
- Tebbi CK. Etiology of acute leukemia: A review. Cancers (Basel). 2021;13(9):2256. doi:10.3390/cancers13092256
- de Oliveira Lisboa M, Brofman PRS, Schmid-Braz AT, et al. Chromosomal instability in acute myeloid leukemia. Cancers (Basel). 2021;13(11):2655. doi:10.3390/cancers13112655
- Gagnon M-F, Berg HE, Meyer RG, et al. Typical, atypical and cryptic t(15;17)(q24;q21) (PML::RARA) observed in acute promyelocytic leukemia: A retrospective review of 831 patients with concurrent chromosome and PML::RARA dual-color dual-fusion FISH studies. Genes Chromosomes Cancer. 2022;61(10):629–634. doi:10.1002/gcc.23070
- Quessada J, Cuccuini W, Saultier P, et al. Cytogenetics of pediatric acute myeloid leukemia: A review of the current knowledge. Genes (Basel). 2021;12(6):924. doi:10.3390/genes12060924
- Li K, Chen L, Zhang H, et al. High expression of COMMD7 is an adverse prognostic factor in acute myeloid leukemia. Aging. (Albany NY). 2021;13(8):11988–12006. doi:10.18632/aging.202901
- Xu X, Cai W, Cai P, et al. Prognostic nomogram for acute myeloid leukemia patients With biallelic CEBPA mutations. Front Oncol. 2021;11:628248. doi:10.3389/fonc.2021.628248
- Arber DA, Borowitz MJ, Cessna M, et al. Initial diagnostic workup of acute leukemia: guideline from the college of American pathologists and the American society of hematology. Arch Pathol Lab Med. 2017;141(10):1342–1393. doi:10.5858/arpa.2016-0504-CP
- Li R, Ma J, Chan Y, et al. Symptom clusters and influencing factors in children With acute leukemia during chemotherapy. Cancer Nurs. 2020;43(5):411–418. doi:10.1097/NCC.0000000000000716
- Arniani S, Pierini V, Pellanera F, et al. Chromothripsis is a frequent event and underlies typical genetic changes in early T-cell precursor lymphoblastic leukemia in adults. Leukemia. 2022;36(11):2577–2585. doi:10.1038/s41375-022-01671-5
- Cai SF, Levine RL. Genetic and epigenetic determinants of AML pathogenesis. Semin Hematol. 2019;56(2):84–89. doi:10.1053/j.seminhematol.2018.08.001
- Yao H, Wang Z, Yang J, et al. Simultaneous in situ detection of protein expression of multiple tumor markers of circulating tumor cells and heteroploid of chromosome 8 in primary lung cancer. Ann Transl Med. 2021;9(24):1772, doi:10.21037/atm-21-6346
- Hemsing AL, Hovland R, Tsykunova G, et al. Trisomy 8 in acute myeloid leukemia. Expert Rev Hematol. 2019;12(11):947–958. doi:10.1080/17474086.2019.1657400
- Zeidan AM, Podoltsev NA, Wang X, et al. Patterns of care and clinical outcomes with cytarabine-anthracycline induction chemotherapy for AML patients in the United States. Blood Adv. 2020;4(8):1615–1623. doi:10.1182/bloodadvances.2020001728
- Carter JL, Hege K, Kalpage HA, et al. Targeting mitochondrial respiration for the treatment of acute myeloid leukemia. Biochem Pharmacol. 2020;182:114253. doi:10.1016/j.bcp.2020.114253
- Qiu Q, Zhang P, Zhang N, et al. Development of a prognostic nomogram for acute myeloid leukemia on IGHD gene family. Int J Gen Med. 2021;14:4303–4316. doi:10.2147/IJGM.S317528
- Chen X-X, Li Z-P, Zhu J-H, et al. Systematic analysis of autophagy-related signature uncovers prognostic predictor for acute myeloid leukemia. DNA Cell Biol. 2020;39(9):1595–1605. doi:10.1089/dna.2020.5667
- Shallis RM, Wang R, Davidoff A, et al. Epidemiology of acute myeloid leukemia: Recent progress and enduring challenges. Blood Rev. 2019;36:70–87. doi:10.1016/j.blre.2019.04.005
- Qu Y, Zhang S, Qu Y, et al. Novel gene signature reveals prognostic model in acute myeloid leukemia. Front Genet. 2020;11:566024. doi:10.3389/fgene.2020.566024
- Jiang F, Yu X, Wu C, et al. A simple-to-Use nomogram for predicting survival in children with acute myeloid leukemia. Biomed Res Int. 2021;2021:7264623. doi:10.1155/2021/7264623