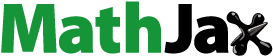
ABSTRACT
Objectives
To construct an endoplasmic reticulum stress-related prognostic risk score (RS) model to predict prognosis and perform a preliminary analysis of immune infiltration in patients with acute myeloid leukemia (AML).
Methods
The whole-genome expression data for AML and endoplasmic reticulum stress (ER stress)-related genes were downloaded from the GEO and GSEA databases, respectively. The samples were divided into death and survival groups, combined with clinical prognosis information. LASSO regression was used to construct a prognostic RS model. The Kaplan–Meier curve method was used to evaluate the association between different risk groups and actual survival prognosis information. A cox regression analysis was used to screen for independent survival prognostic clinical factors and construct a nomogram. CIBERSORT and ssGSEA was used for immune-related analysis.
Results
Eighteen ER-stress related genes were identified and a comprehensive network was constructed. Further, 5 CC, 8 MF, 17 BP, and 2 KEGG pathways were enriched. Ten optimal DEGs were obtained and a prognostic risk model was constructed. Compared to the low RS group, the OS values of the high RS group were significantly lower. A significant correlation between the different risk groups and the actual prognosis was demonstrated. Ten immune cells with significantly different distributions in different risk groups were screened. KEGG enrichment analysis showed that there were 5 signaling pathways in the high-risk group.
Conclusions
The RS model can effectively predict the prognosis and has clinical implications for the prognosis of AML, combined with the correlation between different RS groups and the immune microenvironment.
1. Introduction
Acute myeloid leukemia (AML) is a malignant disease of myeloid stem/progenitor cells. The primary causes of AML are genetic and cytogenetic alterations [Citation1]. Recent evidence indicates that the identification of novel AML biomarkers could contribute to a better understanding of the molecular basis of this disease [Citation2]. However, some patients with relapsed and refractory AML and older patients have a poor prognosis, which is currently a challenging issue. The WHO also recently released the fifth edition of the Classification of Hematolymphoid Tumours [Citation3], and the European Leukemia Net (ELN) recently published a revised system (ELN-2017) [Citation4]. The authors used molecular genetic changes as risk stratification criteria, such as the presence of NPM1, FLT3-ITD, and CEBPA, to indicate a favorable prognosis and the presence of TP53, RUNX1, and ASX1, to indicate a poor prognosis. With the development of many molecularly targeted drugs, new mutated genes to modify the genetic risk stratification system and accommodate the progression of treatment strategies must be continued.
The endoplasmic reticulum (ER) is present in various eukaryotic cells and comprises the biofilm system. It is the basis of intracellular protein synthesis and an important site for post-translational modification, folding, and transport of proteins [Citation5, Citation6]. Tumor cells are often in a microenvironment of ischemia, hypoxia, nutrient deficiency, and low pH during the growth process, which leads to the accumulation of a large number of unfolded or misfolded proteins, causing ER stress. Recent studies have confirmed that ER stress occurs in various tumor cells, leading to various pathological states. ER stress activates three major signaling pathways: the unfolded protein response (UPR), ER overload response (EOR), and sterol regulatory cascades. UPR activation also occurs during hematopoiesis, and increasing evidence supports the cytoprotective role of ER stress in the emergence and proliferation of leukemic cells. Previous studies have shown that ER stress plays an important role in AML pathogenesis [Citation7]. The increased basal UPR is induced, at least in part, by adverse growth conditions in the bone marrow, such as hypoxia [Citation8]. However, the exact role of ER-stress in AML development and progression remains unclear. Therefore, a comprehensive understanding of ER stress can contribute to a better diagnosis and help develop effective treatments for AML.
In this study, we analyzed ER-related genes in AML, explored the functions of related genes, and constructed an ER stress-related prognostic risk model. Further, the prognostic risk model was integrated with the clinical parameters, and a survival curve and normality were determined to verify the clinical predictive ability of the model. A preliminary immune infiltration analysis was also performed. The flow of the analysis is shown in . Overall, a comprehensive understanding of ER stress can contribute to a better diagnosis and prognosis of AML.
2. Materials and methods
2.1. Research objects
GSE106291[Citation9, Citation10] and GSE37642 [Citation11–13] genome-wide expression level datasets, including 250 AML bone marrow samples and 417 AML bone marrow samples with clinical survival prognosis, were downloaded from the NCBI GEO database (http://www.ncbi.nlm.nih.gov/geo/). GSE106291 and GSE37642 were used as training and validation datasets, respectively. ER stress-related genes were downloaded from the MSigDB of the Gene Set Enrichment Analysis database (http://software.broadinstitute.org/gsea/downloads.jsp) [Citation14]. The GPL18460I Illumina HiSeq 1500 (Homo sapiens) and GPL96 Affymetrix Human Genome U133A Array were used for the analysis.
2.2. Differentially expressed ER stress-related genes search
Following the screening of genes related to ER stress, the expression levels of the corresponding genes in the samples were extracted. The samples combined with the clinical prognosis information were divided into the death group and survival group [Citation15–17]. T-test in R3.6.1 was used to compare ER stress-related genes between the two groups, and P < 0.05 was considered statistically significant for the screening threshold.
2.3. Network construction and GO function and KEGG enrichment analysis
The STRING [Citation18] database (Version:11.0, http://string-db.org/) was used to search for interactions between DEGs and their protein products, and an interaction score higher than 0.4 was selected as the screening threshold. The cor function (http://77.66.12.57/R-help/cor.test.html) was calculated using the cor coefficient (PCC). Relationship pairs with a P < 0.05 and an absolute PCC > 0.3 were selected as significant correlation co-expression relationship pairs. A comprehensive network was built using Cytoscape Version 3.6.1(http://www.cytoscape.org/) [Citation19]. Finally, GO function and KEGG signaling pathway enrichment analysis of DEGs was performed using DAVID Version 6.8(The Database for Annotation, Visualization and Integrated Discovery (https://david.ncifcrf.gov/) [Citation20, Citation21].
2.4. Prognostic model construction and validation
The DEGs and clinical survival prognosis information were combined to screen overall survival prognosis using Cox regression analysis (http://bioconductor.org/packages/survivalr/) [Citation22]. Survival regression analysis was performed to determine the optimal combination of ER stress-related genes (https://cran.r-project.org/web/packages/lars/index.html) [Citation23]. According to the LASSO prognostic coefficient of each element in the optimized ER stress-related gene combination and the expression level of the target gene in the dataset, the risk score (RS) model was constructed to calculate the RS as follows:
where Coefgenes represents the LASSO prognostic coefficient of the target gene and Expgenes. represents the expression level of the target gene.
The RS values of the training and validation datasets were calculated, and using the RS median value as the dividing line, the samples were divided into high (RS score ≥ RS median value) and low (RS < RS median value) groups. The association of the actual survival prognosis information between the groups with high and low scores was evaluated using the Kaplan–Meier (KM) curve.
2.5. Relationship between risk scoring and clinical factors and construction of nomogram survival model
Analysis of the distribution of clinical information in samples in different risk groups in the training and validation datasets and screening of independent prognostic clinical factors were performed using Cox regression [Citation22]. A nomogram was constructed to predict one-, three-, and five-year survival rates combined with independent prognostic factors and risk information (https://cran.r-project.org/web/packages/rms/index.html) [Citation24, Citation25] and to calculate the C-index coefficient (C-index can be regarded as the score of all individual pairs correctly ranked based on Harrell C statistics) [Citation25] for predicting survival time[ Citation26]. (http://www.bioconductor.org/packages/release/bioc/html/survcomp.html) [Citation27].
2.6. Immunological correlation evaluation
CIBERSORT (https://cibersort.stanford.edu/index.php) [Citation28] was used to evaluate immune cell type ratios and compare differences among the immune cell distributions of various risk groups. The immune score, stromal score, and tumor purity were calculated and compared among different risk groups (http://127.0.0.1:29606/library/estimate/html/estimateScore.html) [Citation29]. Finally, the correlation between the immune cells, the four estimated scores, and the expression levels of the 10 DEGs was calculated using the cor function.
All KEGG information for related genes was acquired from the GSEA database [Citation14]. According to genome-wide expression data (http://www.bioconductor.org/packages/release/bioc/html/GSVA.html) [Citation30], quantitative analysis was performed for each KEGG signaling pathway, the distribution difference of each pathway was compared, and the correlation between significantly different signaling pathways was analyzed.
2.7. Statistical analysis
All statistical analyses were performed using R3.6.1. A Student’s t-test was used to compare the two groups. Univariate and multivariate Cox regression analyses were used for screening ER stress-related genes and independent prognostic factors for overall survival. The Wilcoxon rank-sum test was used to compare two paired groups. The cor function was calculated for correlation analysis. P < 0.05 was considered statistically significant.
3. Results
3.1. Identification of significantly DEGs
In total, 295 ER stress-related genes were identified and the expression levels of the corresponding genes in the samples were extracted from the GSE106291 expression profile data. The samples were divided into death (n = 100) and survival (n = 150) groups based on clinical prognosis information. Further, 18 ER stress-related genes were identified, including 8 downregulated genes and 10 downregulated genes (A).
Figure 2. Differential analysis ER-stress-related genes and network construction. (A) Violin plot of 18 ER-stress-related genes between survival and death groups; (B) Significantly differentially expressed ER-stress-related genes integrated network; (C) Histogram of BP, CC, MF and KEGG signaling pathways with significant gene correlation in the comprehensive network.
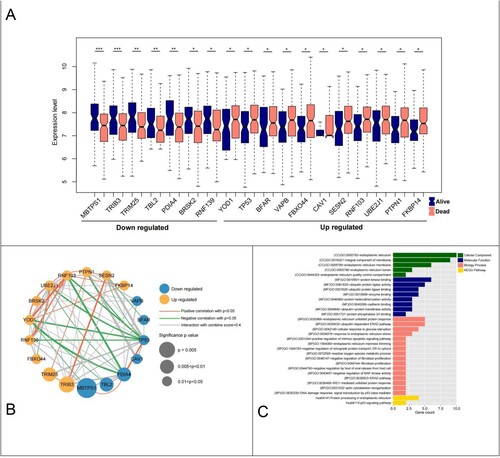
3.2. Network construction and GO function and KEGG enrichment analysis
Thirty-eight linked pairs of gene–protein relationships were obtained via network construction. The PCC of ER stress-related genes was then calculated, and 67 linked pairs of co-expression relationships were obtained and integrated to construct a comprehensive network (B).
GO function and KEGG signaling pathway enrichment showed that a total of 5 cellular components (CC), 8 molecular functions (MF), 17 biological processes (BP), and 2 KEGG pathways were screened (C). The most enriched signaling pathways in CC were ‘endoplasmic reticulum,’ ‘integral component of membrane,’ and ‘endoplasmic reticulum membrane;’ those in MF were ‘protein kinase bindin,’ ‘ubiquitin protein ligase activity,’ ‘ubiquitin protein ligase binding,’ and ‘enzyme;’ those in BP were ‘endoplasmic reticulum unfolded protein response,’ ‘ubiquitin-dependent ERAD pathway,’ and ‘cellular response to glucose starvation.’ ‘Protein processing in endoplasmic reticulum’ and ‘p53 signaling pathway’ were enriched in KEGG.
3.3. ER stress-related genes prognostic model construction
Fifteen DEGs were screened based on clinical survival prognosis information (). To prevent overfitting and eliminate highly correlated ER stress-related genes, LASSO regression analysis was performed. Ten optimal DEGs were obtained using the LASSO algorithm (A). These parameters are shown in (B). Based on the median expression of the 10 genes, the samples were divided into high- and low-expression groups. A KM curve analysis was used to investigate the correlation between expression levels and survival prognosis (C). High expression of FBXO44, TRIB3, RNF139, BRSK2, TRIM25, and FKBP14 was associated with low OS values, indicating that these six genes are risk factors, whereas high expression of MBTPS1, TBL2, VAPB, and PDIA4 was associated with high OS values, indicating that these four genes are protective factors. Based on the LASSO regression coefficients of the 10 genes and their expression levels, the following RS calculation formula was constructed:
Figure 4. (A) LASSO parameter diagram, the intersection of horizontal and vertical black lines in the following figure is the location of the optimal parameter (−4.501,0.218), and the corresponding gene is the optimal gene combination; (B) LASSO coefficients of 10 genes; (C) 10 Prognostic KM curves of the optimized ERS-related genes.
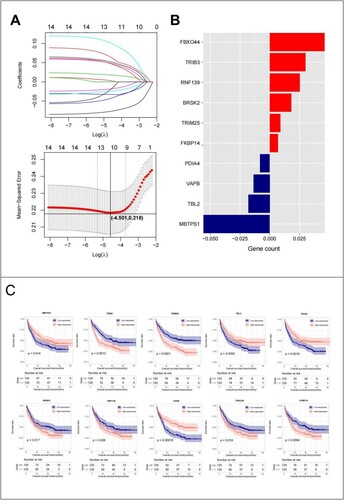
3.4. Efficacy evaluation and comparison of RS survival prognostic risk prediction model
The scatter plot of the model in the GSE106291 training set shows the samples in high- and low-risk groups. The RS value distribution and survival time distribution for each group are shown in (B). The KM curves showed that the OS of the high-RS group was significantly lower than that of the low-RS group (A). The ROC curves for the training set model yielded an acceptable AUC of 0.905 (C). Although the results of our GSE37642 validation dataset remained consistent (D,E), ROC curve analysis of the CGGA validation set model yielded an acceptable AUC value of 0.885 (F).
Figure 5. GSE106291 training set: (A) KM curves based on 10 optimized ER-stress related gene prognostic models; (B) RS distribution and survival time status; (C) The ROC curves of 10 optimal ER-stress related gene prognostic models were plotted, and the numbers in parentheses represent the corresponding ROC curves. GSE37642 validation set: (D) KM curves based on 10 optimized ER-stress related gene prognostic models; (E) RS distribution and survival time status; (F) The ROC curves of 10 optimal ER-stress related gene prognostic models were plotted, and the numbers in parentheses represent the corresponding ROC curves.
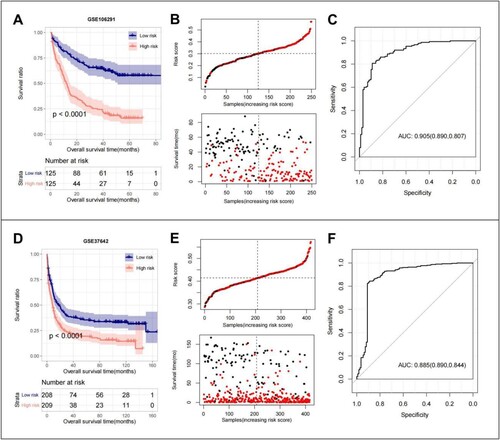
3.5. Relationship between risk score and clinical information
The differences among various RS groups with different clinical characteristics were analyzed using the R language. The results showed that the RS differed significantly according to age, runx1-runx1t1fusion, and treatment response (A–E), with patients aged >60 years having a higher RS with runx1-runx1t1_fusion and those with sensitivity to treatment response having a lower RS.
Figure 6. (A) Differential relationship between age clinical traits in risk group; (B) Differential relationship between sex clinical traits in risk group; (C) Differential relationship between runx1 mutation in risk group; (D) Differential relationship between runx1-runx1t1_fusion in risk group; (E) differential relationship between treatment response in risk group.
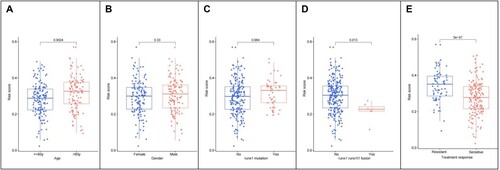
3.6. Construction of a nomogram survival model with independent prognostic factors
The screening results for independent prognostic clinical factors are shown in . Age, treatment response, and the RS model were significant independent prognostic factors (A). The nomogram survival model is shown in (B). The ‘Total points’ axis was used to predict the survival of the sample based on various clinical indicators. Calibration plots showed that the nomogram graphs were consistent with the ideal model runs by comparing the consistency between the one-, three-, and five-year survival rates (C).
Figure 7. (A) Independent prognostic Mori diagram of clinical prognostic factors; (B) Nomogram of nomogram for survival prediction model with independent prognostic factors; (C) Nomogram (left to right) Consistency plots of predicted and actual survival rates at one、three、and five years. The horizontal axis shows the predicted and the vertical axis shows the actual survival rates;
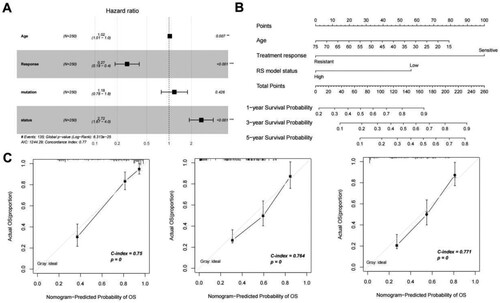
Table 1. Prognostic relevance clinical prognostic factors.
3.7. Immune infiltration analysis
Ten immune cells with significantly different distributions in the different risk groups were screened (A). (B) shows that the distribution of Stromal, Immune, and ESTIMATE Scores in the high-risk group was higher than that in the low-risk group, but TumorPurity in the low-risk group was higher than that in the high-risk group. Correlation analysis showed that TRIM25 was positively and negatively correlated with Immune Score and TumorPurity, respectively. Macrophage M2 was positively and negatively correlated with PDIA4 and T cell CD4 + memory activation, respectively (C).
Figure 8. (A) Distribution of 10 immune cells with significantly different distribution in different risk groups; (B) Distribution of the estimated scores in different risk groups; (C) Heat map of correlation between expression levels of 10 DEGs with 10 immune cells, 4 ESTIMATE scores;Scatter plot of the best positive and negative correlations on the side.
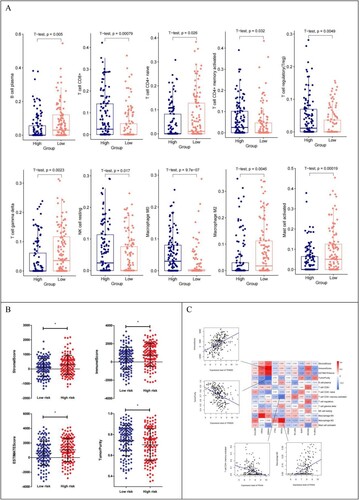
3.8. GSVA quantitative KEGG analysis based on genome-wide expression levels
We identified 21 KEGG signaling pathways with significant differences between the different risk groups (A). Twelve signaling pathways were enriched in the low-risk group, including ‘valine leucine and isoleucine biosynthesis,’ ‘aminoacyl tRNA biosynthesis,’ ‘non homologous and joining,’ ‘homologous recombination,’ ‘propanoate metabolism,’ ‘mismatch repair,’ ‘valine leucine and isoleucine degradation,’ ‘selenoamino acid metabolism,’ ‘sulfur metabolism,’ ‘cysteine and methionine metabolism,’ ‘histidine metabolism,’ and ‘DNA replication’. Nine signaling pathways were enriched in the high-risk group, including ‘cytokine cytokine receptor interaction,’ ‘thyroid cancer,’ ‘natural killer cell-mediated cytotoxicity,’ ‘folate biosynthesis,’ ‘type I diabetes mellitus,’ ‘allograft rejection,’ ‘glycosaminoglycan biosynthesis keratan sulfate,’ ‘kegg graft versus host disease,’ and ‘kegg circadian rhythm mammal’. The correlation among KEGG signaling pathways with significant differences in distribution showed that the PDIA4 gene was positively correlated with the valine, leucine, and isoleucine degradation signaling pathways and negatively correlated with the mammalian circadian rhythm signaling pathway (B).
Figure 9. (A) Heatmap of KEGG signaling pathways with significant differences between risk groups; (B) Heat map of the correlation between the quantified KEGG signaling pathways and the expression levels of the 10 genes that constitute the RS model. Scatter plot of the best positive and negative correlations on the side.
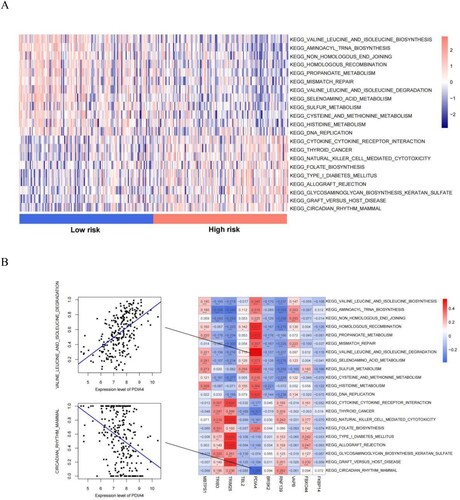
3.10. KEGG enrichment analysis related to risk groups
Based on the genome-wide expression level of the GSE106291 tumor samples, KEGG signaling pathways significantly related to risk grouping were screened. The result showed that 20 significantly related KEGG signaling pathways were screened out, of which 5 and 15 pathways were enriched in the high-risk and low-risk groups, respectively (). The five pathways in the high-risk group were ‘Allograft rejection,’ ‘glycosaminoglycan biosynthesis keratan sulfate,’ ‘graft versus host disease,’ ‘prostate cancer,’ and ‘type I diabetes mellitus.’
4. Discussion
Recent studies have shown that ER stress is involved in AML pathogenesis [Citation7]. Simultaneously, the ER stress response in myeloid leukemia cells has been associated with advanced disease stages and cell resistance [Citation31, Citation32]. Although the role of ER stress in the development of AML is well-established, the extent to which ER stress affects the prognosis of AML remains unclear.
In this study, ER stress-related genes were analyzed in AML. First, 18 ER stress-related genes were identified, including 8 downregulated genes and 10 downregulated genes. A comprehensive network was constructed, including 38 pairs of gene and protein relationships, 67 pairs of co-expression relationships A total of 5 CC, 8 MF, 17 BP, and 2 KEGG pathways were enriched. The most enriched signaling pathways in CC were ‘endoplasmic reticulum,’ ‘integral component of membrane,’ and ‘endoplasmic reticulum membrane;’ those in MF were ‘protein kinase binding,’ ‘ubiquitin protein ligase activity,’ ‘ubiquitin protein ligase binding,’ and ‘enzyme;’ those in BP were ‘endoplasmic reticulum unfolded protein response,’ ‘ubiquitin-dependent ERAD pathway,’ and ‘cellular response to glucose starvation.’ ‘Protein processing in endoplasmic reticulum’ and ‘p53 signaling pathway’ were enriched in KEGG. Further, 10 ER stress-related genes were optimized, among which MBTPS1, TBL2, VAPB, and PDIA4 were protective factors, and FBXO44, TRIB3, RNF139, BRSK2, TRIM25, and FKBP14 were risk factors. We then combined the DEGs with their corresponding clinical information to establish an RS model. In the prognostic prediction mode, MBTPS1 and FBXO44 had the greatest impact on prognosis. The higher the expression level of MBTPS1, the better the prognosis. The MBTPS1 gene, also known as the membrane-bound transcription factor peptidase site 1 gene, encodes Site-1 protease (S1P). S1P regulates lipogenesis, ER function, lysosomes, and biogenesis in mice and cultured cells. Studies have found [Citation33] that deletion of MBTPS1 can impair ER function. The investigators also developed a predictive model that included six membrane protein genes in patients with AML without cytogenetic abnormalities, among which MBTPS1 was a risk factor. However, the higher the expression level of FBXO44, the worse the prognosis. FBXO44 belongs to the F-box protein family (FBA family) and shares a conserved G domain for substrate binding. In addition to sequence homology, all members of the FBA family, except FBXO44, can recognize the glycosylation substrate FBXO44, which has a substrate-recognition function during ubiquitin-mediated proteolysis [Citation34–36]. A misfolded protein response occurs when the ER lumen misfolds or when excessive protein synthesis occurs. Studies [Citation37] have shown that FBXO44 is an E3 ubiquitin ligase responsible for BRCA1 degradation. FBXO44 promotes the silencing of DNA replication-coupled repetitive elements in cancer cells [Citation38].
In addition, we evaluated the performance of the risk prediction models. The KM curves showed that the OS of the high RS group was significantly lower than that of the low RS group. Meanwhile, we explored the relationship between the RS and clinical information, which revealed that the RS differed significantly according to age, runx1-runx1t1 fusion, and treatment response. Compared with patients with low RS scores, patients with high RS scores had worse prognosis in the training and validation groups, which further proved that ER stress-related gene markers have high prediction accuracy and robustness and that the RS is an independent prognostic factor. Furthermore, we constructed a quantitative and objective nomogram based on a multivariate analysis. We analyzed the consistency between the one-, three-, and five-year survival rates predicted by the nomogram survival model and the actual survival rates, verifying that our nomogram survival model has high predictive value and is suitable for clinical applications. The ROC curve analysis of the training set and CGGA validation set models yielded acceptable AUC values of 0.905 and 0.885, respectively. AUC values of 0.8–0.9 indicate good sensitivity and specificity. The results of the ROC analysis indicated that the metrics of our nomogram graphs are steadily predictive. Therefore, ER stress-related prognostic markers can be used as potential prognostic biomarkers for AML.
Immune escape has become an important factor affecting the long-term disease-free survival of patients with AML [Citation39]. Therefore, we conducted a preliminary study on the immune infiltration of AML, and 10 immune cells with significantly different distributions in different risk groups were screened. In the high-risk group, CD8+ T cells, CD4 + memory activated T cells, regulatory T cells (Tregs), resting natural killer (NK) cells, and Macrophage M0 immune cells infiltrated in a high proportion. Whereas, in the low-risk group, B cell plasma, CD4 + naïve T cells, T cell gamma delta, macrophage M2, and mast cells infiltrated in a high proportion. A previous study found that the T-cell and Tregs population in the bone marrow of patients with AML were higher than those in healthy donors. Moreover, targeting immune receptors provides a theoretical basis for T-cell immunotherapy in AML [Citation40]. In AML, T cells are not functionally impaired; increased PD-1 expression is observed only at relapse and is associated with shifts to memory T-cell compartments [Citation41]. Tregs play an important role in tumor immune escape and angiogenesis [Citation42]. NK cells play a key role in inducing an effective adaptive anti-tumor immune response. However, in patients with AML, significantly impaired NK cell function can prevent immune surveillance and affect prognosis [Citation43]. Various immune escape mechanisms have been reported in patients with AML, including decreased expression of NK cytotoxic receptors, downregulation of NK ligands, and secretion of soluble NK inhibitory factors [Citation44]. Monocytes and macrophages are important immune cell types involved in tumor immune escape. Monocytes have been reported to differentiate into macrophages. Stimulation with cytokines and chemokines leads to the differentiation of macrophages into classically activated macrophages (M1) and alternately activated macrophages (M2). Tumor-associated macrophages (TAMs), which are important components of tumor-infiltrating immune cells, have been associated with the growth, angiogenesis, and metastasis of a variety of cancers, most likely through TAMs polarization to M2 (an alternative phenotype). Clinical and experimental evidence has shown that highly invasive tumor tissues with TAMs are associated with poor prognosis and drug resistance. Therefore, targeting TAMs is considered a promising immunotherapeutic strategy for tumors [Citation45]. Some researchers have reported that the degree of mast cell infiltration positively correlates with tumor size, grade, and metastasis [Citation46].
Subsequently, we correlated 10 immune cells with significantly different distributions, 4 ESTIMATE scores, and the expression levels of 10 genes constituting the RS model. The results showed that the distribution of stromal, immune, and ESTIMATE scores in the high-risk group was higher than that in the low-risk group. However, TumorPurity in the low-risk group was higher than that in the high-risk group. TRIM25 positively correlated with Immune Score and negatively correlated with TumorPurity. Macrophage M2 positively correlated with PDIA4 but negatively correlated with CD4 + T-cell memory activation. Based on the GSVA quantitative KEGG analysis of genome-wide expression levels, 21 KEGG signaling pathways with significant differences among the different risk groups were identified. The results showed that PDIA4 was positively correlated with valine, leucine, and isoleucine degradation signaling pathways. There was a negative correlation with mammalian circadian rhythm signaling pathway. Valine, leucine, and isoleucine degradation play a key role in the regulation of energy homeostasis, nutrient metabolism, gut health, immunity, and disease in humans and animals. Genetic research related to the mammalian circadian rhythm signaling pathway has revealed that the circadian system is closely linked to the processes that control sleep and metabolism.
TRIM25, a member of the TRIM (tripartite motif) protein subfamily (a subfamily of Ring-type E3 ubiquitin ligases), plays an important regulatory role in carcinogenesis. Dysregulation of the ubiquitin-mediated degradation of oncogene products or tumor suppressors may be related to the etiology of cancer and leukemia [Citation47]. Studies have found that TRIM25 is the most significant inducer gene in ER-stress [Citation48]. As a feedback mechanism, TRIM25 is required for tumor cell defense against ER stress. TRIM25 promotes tumor cell survival. PDIA4 is a protein disulfide isomerase that catalyzes the formation of disulfide bonds that can activate platelets, promote thrombosis, and respond to ER stress. In addition, current studies have shown that activated platelets and ER stress responses influence tumor progression, and PDIA4 is involved in tumor development by affecting apoptosis and DNA repair mechanisms. Therefore, PDIA4 inhibitors may have great potential for inhibiting tumor progression [Citation49].
KEGG signaling pathways that were significantly related to risk groups were identified. The result showed that 20 significantly related KEGG signaling pathways were screened out, of which 5 pathways were enriched in the high-risk group. The five pathways in the high-risk group were ‘allograft rejection,’ ‘glycosaminoglycan biosynthesis keratan sulfate,’ ‘graft versus host disease,’ ‘prostate cancer,’ and ‘type I diabetes mellitus.’
Our study had some limitations. First, the results were analyzed using bioinformatics and need to be experimentally verified further. Second, the robustness of this prognostic risk model must be verified in prospective clinical studies with large sample sizes.
5. Conclusions
We developed and validated an ER stress-associated gene marker for the prognostic stratification of patients with AML. In addition, this biomarker can be used to predict patient survival, providing a new strategy for the prognostic prediction of AML. Survival outcomes and immune cell infiltration levels differed among the different RS groups. By combining prognostic risk models and clinical parameters to construct nomogram plots, our results provide new insights into the identification of prognostic biomarkers.
Supplemental Material
Download MS Word (11.8 KB)Acknowledgements
Author contributions: Lu Dong: research design and performance, data collection, data analysis and manuscript-writing; Zefeng Miao: data analysis; Haili Wang, Yanhui Yu, Dongzheng Gai, Guoxiang Zhang and Li Ge: data recheck; Xuliang Shen: research design and manuscript review.
Disclosure statement
No potential conflict of interest was reported by the author(s).
Data availability statement
Our data and related clinical information were retrieved from NCBI Gene Expression Omnibus database (GEO http://www.ncbi.nlm.nih.gov/geo/).
Additional information
Funding
References
- Grimwade D, Ivey A, Huntly BJ. Molecular landscape of acute myeloid leukemia in younger adults and its clinical relevance. Blood. 2016;127(1):29–41. Epub 20151210. doi:10.1182/blood-2015-07-604496. PubMed PMID: 26660431; PubMed Central PMCID: PMC4705608.
- Prada-Arismendy J, Ospina JA, Rthlisberger S. Molecular biomarkers in acute myeloid leukemia. Blood Rev. 2017;31(1):63–76. doi:10.1016/j.blre.2016.08.005
- Alaggio R, Amador C, Anagnostopoulos I, et al. The 5th edition of the World Health Organization classification of Haematolymphoid Tumours: lymphoid neoplasms. Leukemia. 2022;36(7):1720–1748. Epub 20220622. doi:10.1038/s41375-022-01620-2. PubMed PMID: 35732829; PubMed Central PMCID: PMC9214472.
- Harada Y, Nagata Y, Kihara R, et al. Prognostic analysis according to the 2017 ELN risk stratification by genetics in adult acute myeloid leukemia patients treated in the Japan adult leukemia study group (JALSG) AML201 study. Leuk Res. 2018;66:20–27. Epub 20180117. doi:10.1016/j.leukres.2018.01.008. PubMed PMID: 29360622.
- Wang M, Kaufman RJ. Protein misfolding in the endoplasmic reticulum as a conduit to human disease. Nature. 2016;529(7586):326–335. doi:10.1038/nature17041
- Schwarz DS, Blower MD. The endoplasmic reticulum: structure, function and response to cellular signaling. Cell Mol Life Sci. 2016;73(1):79–94. Epub 20151003. doi:10.1007/s00018-015-2052-6. PubMed PMID: 26433683; PubMed Central PMCID: PMC4700099.
- Féral K, Jaud M, Philippe C, et al. Er stress and unfolded protein response in leukemia: friend, Foe, or both? Biomolecules. 2021;11(2). Epub 20210130. doi:10.3390/biom11020199. PubMed PMID: 33573353; PubMed Central PMCID: PMC7911881.
- Rouault-Pierre K, Hamilton A, Bonnet D. Effect of hypoxia-inducible factors in normal and leukemic stem cell regulation and their potential therapeutic impact. Expert Opin Biol Ther. 2016;16(4):463–476. Epub 20160128. doi:10.1517/14712598.2016.1133582. PubMed PMID: 26679619.
- Edgar R, Domrachev M, Lash AE. Gene expression omnibus: NCBI gene expression and hybridization array data repository. Nucleic Acids Res. 2002;30(1):207–210. doi:10.1093/nar/30.1.207. PubMed PMID: 11752295; PubMed Central PMCID: PMC99122.
- Herold T, Jurinovic V, Batcha AMN, et al. A 29-gene and cytogenetic score for the prediction of resistance to induction treatment in acute myeloid leukemia. Haematologica. 2018;103(3):456–465. Epub 20171214. doi:10.3324/haematol.2017.178442. PubMed PMID: 29242298; PubMed Central PMCID: PMC5830382.
- Herold T, Metzeler KH, Vosberg S, et al. Isolated trisomy 13 defines a homogeneous AML subgroup with high frequency of mutations in spliceosome genes and poor prognosis. Blood. 2014;124(8):1304–1311. Epub 20140612. doi:10.1182/blood-2013-12-540716. PubMed PMID: 24923295.
- Li Z, Herold T, He C, et al. Identification of a 24-gene prognostic signature that improves the European LeukemiaNet risk classification of acute myeloid leukemia: an international collaborative study. J Clin Oncol. 2013;31(9):1172–1181. Epub 20130204. doi:10.1200/jco.2012.44.3184. PubMed PMID: 23382473; PubMed Central PMCID: PMC3595425.
- Kuett A, Rieger C, Perathoner D, et al. IL-8 as mediator in the microenvironment-leukaemia network in acute myeloid leukaemia. Sci Rep. 2015;5:18411. Epub 20151217. doi:10.1038/srep18411. PubMed PMID: 26674118; PubMed Central PMCID: PMC4682064.
- Subramanian A, Tamayo P, Mootha VK, et al. Gene set enrichment analysis: a knowledge-based approach for interpreting genome-wide expression profiles. Proc Natl Acad Sci U S A. 2005;102(43):15545–15550. Epub 20050930. doi:10.1073/pnas.0506580102. PubMed PMID: 16199517; PubMed Central PMCID: PMC1239896.
- Sun Y, Luo J, Qian C, et al. The value of nutritional status in the prognostic analysis of patients with AIDS-related lymphoma. Infect Drug Resist. 2021;14:1105–1113. doi:10.2147/IDR.S295077
- Lu Y, Gong Z, Jin X, et al. LncRNA MALAT1 targeting miR-124-3p regulates DAPK1 expression contributes to cell apoptosis in Parkinson's disease. J Cell Biochem. 2020. Epub 20200410. doi:10.1002/jcb.29711. PubMed PMID: 32277510.
- Zhu Y, Du Z, Zhu Y, et al. Evaluation of organ function in patients with severe COVID-19 infections. Med Clin (Engl Ed). 2020;155(5):191–196. Epub 20200919. doi:10.1016/j.medcle.2020.05.015. PubMed PMID: 32984539; PubMed Central PMCID: PMC7501795.
- Szklarczyk D, Gable AL, Nastou KC, et al. The STRING database in 2021: customizable protein-protein networks, and functional characterization of user-uploaded gene/measurement sets. Nucleic Acids Res. 2021;49(D1):D605–Dd12. doi:10.1093/nar/gkaa1074. PubMed PMID: 33237311; PubMed Central PMCID: PMC7779004.
- Shannon P, Markiel A, Ozier O, et al. Cytoscape: a software environment for integrated models of biomolecular interaction networks. Genome Res. 2003;13(11):2498–2504. doi:10.1101/gr.1239303. PubMed PMID: 14597658; PubMed Central PMCID: PMC403769.
- Huang da W, Sherman BT, Lempicki RA. Systematic and integrative analysis of large gene lists using DAVID bioinformatics resources. Nat Protoc. 2009;4(1):44–57. doi:10.1038/nprot.2008.211. PubMed PMID: 19131956.
- Huang da W, Sherman BT, Lempicki RA. Bioinformatics enrichment tools: paths toward the comprehensive functional analysis of large gene lists. Nucleic Acids Res. 2009;37(1):1–13. Epub 20081125. doi:10.1093/nar/gkn923. PubMed PMID: 19033363; PubMed Central PMCID: PMC2615629.
- Wang P, Wang Y, Hang B, et al. A novel gene expression-based prognostic scoring system to predict survival in gastric cancer. Oncotarget. 2016;7(34):55343–55351. doi:10.18632/oncotarget.10533. PubMed PMID: 27419373; PubMed Central PMCID: PMC5342421.
- Goeman JJ. L1 penalized estimation in the Cox proportional hazards model. Biom J. 2010;52(1):70–84. doi:10.1002/bimj.200900028. PubMed PMID: 19937997.
- Tibshirani R. The lasso method for variable selection in the Cox model. Stat Med. 1997;16(4):385–395. doi:10.1002/(sici)1097-0258(19970228)16:4 < 385::aid-sim380 > 3.0.co;2-3. PubMed PMID: 9044528.
- Harrell FE, Lee KL, Mark DB. Multivariable prognostic models: issues in developing models, evaluating assumptions and adequacy, and measuring and reducing errors. Stat Med. 1996;15(4):361–387. doi:10.1002/(sici)1097-0258(19960229)15:4 < 361::Aid-sim168 > 3.0.Co;2-4. PubMed PMID: 8668867.
- Mayr A, Schmid M. Boosting the concordance index for survival data–a unified framework to derive and evaluate biomarker combinations. PLoS One. 2014;9(1):e84483. Epub 20140106. doi:10.1371/journal.pone.0084483. PubMed PMID: 24400093; PubMed Central PMCID: PMC3882229.
- Shan S, Chen W, Jia JD. Transcriptome analysis revealed a highly connected gene module associated With cirrhosis to hepatocellular carcinoma development. Front Genet. 2019;10:305. Epub 20190402. doi:10.3389/fgene.2019.00305. PubMed PMID: 31001331; PubMed Central PMCID: PMC6454075.
- Chen B, Khodadoust MS, Liu CL, et al. Profiling tumor infiltrating immune cells with CIBERSORT. Methods Mol Biol. 2018;1711:243–259. doi:10.1007/978-1-4939-7493-1_12. PubMed PMID: 29344893; PubMed Central PMCID: PMC5895181.
- Hu D, Zhou M, Zhu X. Deciphering immune-associated genes to predict survival in clear cell renal cell cancer. Biomed Res Int. 2019;2019:2506843. Epub 20191207. doi:10.1155/2019/2506843. PubMed PMID: 31886185; PubMed Central PMCID: PMC6925759.
- Ye L, Zhang T, Kang Z, et al. Tumor-infiltrating immune cells act as a marker for prognosis in colorectal cancer. Front Immunol. 2019;10:2368. Epub 20191017. doi:10.3389/fimmu.2019.02368. PubMed PMID: 31681276; PubMed Central PMCID: PMC6811516.
- Wang M, Kaufman RJ. The impact of the endoplasmic reticulum protein-folding environment on cancer development. Nat Rev Cancer. 2014;14(9):581–597. doi:10.1038/nrc3800. PubMed PMID: 25145482.
- Urra H, Dufey E, Avril T, et al. Endoplasmic reticulum stress and the hallmarks of cancer. Trends Cancer. 2016;2(5):252–262. Epub 20160423. doi:10.1016/j.trecan.2016.03.007. PubMed PMID: 28741511.
- Lin SY, Miao YR, Hu FF, et al. A 6-membrane protein gene score for prognostic prediction of cytogenetically normal acute myeloid leukemia in multiple cohorts. J Cancer. 2020;11(1):251–259. Epub 20200101. doi:10.7150/jca.35382. PubMed PMID: 31892991; PubMed Central PMCID: PMC6930412.
- Glenn KA, Nelson RF, Wen HM, et al. Diversity in tissue expression, substrate binding, and SCF complex formation for a lectin family of ubiquitin ligases. J Biol Chem. 2008;283(19):12717–12729. Epub 20080118. doi:10.1074/jbc.M709508200. PubMed PMID: 18203720; PubMed Central PMCID: PMC2442310.
- Ilyin GP, Sérandour AL, Pigeon C, et al. A new subfamily of structurally related human F-box proteins. Gene. 2002;296(1-2):11–20. doi:10.1016/s0378-1119(02)00867-3. PubMed PMID: 12383498.
- Winston JT, Koepp DM, Zhu C, et al. A family of mammalian F-box proteins. Curr Biol. 1999;9(20):1180–1182. doi:10.1016/s0960-9822(00)80021-4. PubMed PMID: 10531037.
- Lu Y, Li J, Cheng D, et al. The F-box protein FBXO44 mediates BRCA1 ubiquitination and degradation. J Biol Chem. 2012;287(49):41014–41022. Epub 20121018. doi:10.1074/jbc.M112.407106. PubMed PMID: 23086937; PubMed Central PMCID: PMC3510804.
- Shen JZ, Qiu Z, Wu Q, et al. FBXO44 promotes DNA replication-coupled repetitive element silencing in cancer cells. Cell. 2021;184(2):352–369. Epub 20201223. doi:10.1016/j.cell.2020.11.042. PubMed PMID: 33357448; PubMed Central PMCID: PMC8043252.
- Zeiser R, Vago L. Mechanisms of immune escape after allogeneic hematopoietic cell transplantation. Blood. 2019;133(12):1290–1297. Epub 20181221. doi:10.1182/blood-2018-10-846824. PubMed PMID: 30578254.
- Williams P, Basu S, Garcia-Manero G, et al. The distribution of T-cell subsets and the expression of immune checkpoint receptors and ligands in patients with newly diagnosed and relapsed acute myeloid leukemia. Cancer. 2019;125(9):1470–1481. Epub 20181130. doi:10.1002/cncr.31896. PubMed PMID: 30500073; PubMed Central PMCID: PMC6467779.
- Schnorfeil FM, Lichtenegger FS, Emmerig K, et al. T cells are functionally not impaired in AML: increased PD-1 expression is only seen at time of relapse and correlates with a shift towards the memory T cell compartment. J Hematol Oncol. 2015;8:93. Epub 20150730. doi:10.1186/s13045-015-0189-2. PubMed PMID: 26219463; PubMed Central PMCID: PMC4518596.
- Facciabene A, Motz GT, Coukos G. T-regulatory cells: key players in tumor immune escape and angiogenesis. Cancer Res. 2012;72(9):2162–2171. doi:10.1158/0008-5472.Can-11-3687. PubMed PMID: 22549946; PubMed Central PMCID: PMC3342842.
- Lion E, Willemen Y, Berneman ZN, et al. Natural killer cell immune escape in acute myeloid leukemia. Leukemia. 2012;26(9):2019–2026. Epub 20120326. doi:10.1038/leu.2012.87. PubMed PMID: 22446501.
- Hong CS, Sharma P, Yerneni SS, et al. Circulating exosomes carrying an immunosuppressive cargo interfere with cellular immunotherapy in acute myeloid leukemia. Sci Rep. 2017;7(1):14684. Epub 20171031. doi:10.1038/s41598-017-14661-w. PubMed PMID: 29089618; PubMed Central PMCID: PMC5666018.
- Goswami KK, Ghosh T, Ghosh S, et al. Tumor promoting role of anti-tumor macrophages in tumor microenvironment. Cell Immunol. 2017;316:1–10. Epub 20170413. doi:10.1016/j.cellimm.2017.04.005. PubMed PMID: 28433198.
- Cherdantseva TM, Bobrov IP, Avdalyan AM, et al. Mast cells in renal cancer: clinical morphological correlations and prognosis. Bull Exp Biol Med. 2017;163(6):801–804. Epub 20171024. doi:10.1007/s10517-017-3907-7. PubMed PMID: 29063337.
- Hatakeyama S. TRIM proteins and cancer. Nat Rev Cancer. 2011;11(11):792–804. Epub 20111007. doi:10.1038/nrc3139. PubMed PMID: 21979307.
- Liu Y, Tao S, Liao L, et al. TRIM25 promotes the cell survival and growth of hepatocellular carcinoma through targeting Keap1-Nrf2 pathway. Nat Commun. 2020;11(1):348. Epub 20200117. doi:10.1038/s41467-019-14190-2. PubMed PMID: 31953436; PubMed Central PMCID: PMC6969153.
- Wang Z, Zhang H, Cheng Q. PDIA4: the basic characteristics, functions and its potential connection with cancer. Biomed Pharmacother. 2020;122:109688. Epub 20191130. doi: 10.1016/j.biopha.2019.109688. PubMed PMID: 31794946.