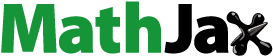
Abstract
The degree of type-1 fuzzy sets membership function cannot express the linguistic variable of a complex problem. The type-2 fuzzy sets as a problem solver such that more fuzziness for constructing membership functions can be handled. Recently, many multi-criteria decision making (MCDM) methods have been expanded using type-2 fuzzy sets. Analytical Hierarchy Process (AHP) is one of the well-known MCDM that can take into account multiple and conflicting criteria at the same time. Our goal is to develop an interval type-2 trapezoidal fuzzy AHP through the new proposed ranking i.e. the modified total integral value. Based on the illustrative examples for trapezoidal type-2 fuzzy sets, the new proposed ranking has a well-performance in ranking. Furthermore, we apply the new trapezoidal type-2 fuzzy AHP to a supplier selection problem. Based on the results of the application, the new fuzzy AHP has the same ranking results as the existing fuzzy AHP.
1. Introduction
In a decision support system on conventional multi-criteria decision making (MCDM) problems, the perceptions of experts called linguistic variables are represented by crisp numbers. The judgment of perception is strongly influenced by the subjectivity factor of an expert in decision making. However, several real problems, the judgment's expert that is fuzziness and vagueness can be an impact on the accuracy of data. Evaluation on criteria is subjective and qualitative, it is very difficult for decision makers to represent it in numbers [Citation1]. The conventional MCDM approach is considered less effective to overcome the fuzziness and vagueness value of linguistic variables [Citation2]. Analytical Hierarchy Process (AHP) was introduced by Saaty [Citation3] is well-known MCDM, having structured multi-criteria technique in organising and analysing complex decisions including conflicting criteria. The fuzzy AHP technique is rated as an advanced analysis method that is developed from conventional AHP is capable to overcome fuzziness and vagueness in the decision maker's judgment [Citation4].
Based on the references, linguistic variables of fuzzy AHP are represented by type-1 fuzzy sets had been discussed. Triangular Fuzzy AHP based logarithmic least squares method to calculate fuzzy eigenvectors [Citation5]. Extended analysis of triangular fuzzy AHP [Citation6,Citation7]. Fuzzy Programming method based geometrical representation of the prioritisation process to enhanced consistency of pairwise comparison matrix in fuzzy AHP [Citation8]. Direct fuzzification eigenvector of fuzzy AHP uses Lambda-Max method [Citation9]. Fuzzy geometric row means to improve the consistency of pairwise comparison matrices [Citation10]. Group fuzzy preference programming based on combines the group synthesis and prioritisation on fuzzy AHP [Citation11]. Eigenvector method to calculate interval fuzzy weight from pairwise comparison matrices of trapezoidal and triangular fuzzy AHP [Citation12]. A logarithmic fuzzy preference programming to compute the fuzzy weight of fuzzy AHP [Citation13]. Consistency analysis and fuzzy priority on pairwise comparison matrices of triangular fuzzy AHP use fuzzy arithmetic operations based on transitivity equations where this equation reflects consistency by using geometric means and modal mean [Citation14]. Fuzzy AHP based eigenvector to improve consistency of pairwise comparison matrix and expected values to decision making [Citation15,Citation16].
Normally, the degree of type-1 fuzzy sets (T1FS) membership function is evaluated by crisp numbers. However, for various complexity problems, the degree of membership function will be difficult to express the linguistic variables of the problem. Therefore, the concept of type-2 fuzzy sets (T2FS) is extended from T1FS was introduced by Zadeh [Citation17] can be handled. T2FS is very compatible of the problems that is difficult to evaluate by the degree of T1FS membership function, such that T2FS can be expressed the different of linguistic the expert's judgment [Citation18]. The main difference between T1FS and T2FS lies in the dimension of membership function value, where T1FS is two dimensions, while T2FS is three dimensions [Citation19]. The new three dimensions provide an additional degree of membership function which allows for direct expression of fuzziness [Citation20].
Furthermore, the interval type-2 fuzzy sets (IT2FS) is a special case of T2FS and has been implemented in fuzzy MCDM [Citation19,Citation21–27], especially MCDM problems. The modified of Buckley's fuzzy AHP with pairwise comparison matrix represented by IT2FS [Citation28]. Best Non-fuzzy Performance (BNP) method to rank IT2FS of pairwise comparison matrices and was applied on the modified of Buckley's fuzzy AHP [Citation20]. The modified of Van Laarhoven and Pedrycz's fuzzy AHP with interval type-2 trapezoidal fuzzy number (IT2TrFN) and was applied in new product development project screening [Citation29]. The application of Kahraman et al.'s fuzzy AHP with IT2TrFN to analysed business project priorities [Citation30], for event log-based fraud ranking [Citation31] and for green supplier selection with case studies of household appliance manufacturers [Citation32]. Buckley's fuzzy AHP is based on Z-numbers to evaluate social sustainable development factors [Citation33]. In addition, many researchers have combined IT2FS fuzzy AHP with other MCDM methods [Citation34], such as the combination of Kahraman et al.'s fuzzy AHP and fuzzy TOPSIS for decision making in maritime transport engineering [Citation35], to evaluate the quality of life in 28 EU countries and other 6 countries [Citation36] and to determine the main priority in distribution [Citation37]. The combination of the modified of Buckley's fuzzy AHP based balanced score card and fuzzy TOPSIS to strategy selection problem [Citation38]. Buckley's fuzzy AHP is based on Abbasimehr et al.'s ranking and fuzzy VIKOR to evaluate online review [Citation39].
The main research contribution is the development of type-1 trapezoidal fuzzy AHP was proposed by Prascevi and Prascevi [Citation16] to be interval type-2 fuzzy AHP with linguistic expert's judgment represented by IT2TrFN [Citation20]. Furthermore, the new proposed ranking based left, right and total integral value is presented on the ranking process. Numerical examples are provided to illustrate the capability of the new proposed ranking and a case study is provided to demonstrate the new interval type-2 fuzzy AHP.
The paper is ordered as follows: Section 2 is interval type-2 trapezoidal fuzzy sets, and the ranking methods for interval type-2 trapezoidal fuzzy sets are discussed in Section 3. Then, in Section 4, the new interval type-2 trapezoidal fuzzy AHP is presented. Section 5 describes in practice of the new interval type-2 trapezoidal fuzzy AHP. The conclusion is provided in Section 6.
2. Interval Type-2 Trapezoidal Fuzzy Sets
This section provides an overview of the concept of interval type-2 fuzzy sets (IT2FS) as follows [Citation40]:
Let is a type 2 fuzzy set (T2FS) with membership function denoted by
where
and
. Therefore,
can be defined as follows [Citation17]:
(1)
(1)
where
is the primary level membership function on x and
for
,
is a secondary or second level membership function. When the membership function of
is continue, so
can be represented as follows:
(2)
(2)
where
denoted by union
in feasible area and the membership function is discrete, it notation can be changed with
.
Based on Equation (Equation1(1)
(1) ),
is T2FS with support
(real number). Both of them are normal and convex. So, support
or
can be defined as follows [Citation41]:
(3)
(3)
From Equation (Equation3
(3)
(3) ),
for all
can be defined as IT2FS, such that Equation (Equation2
(2)
(2) ) can be changed to membership function of IT2FS
which is represented as follows:
(4)
(4)
For simplicity, given
is IT2FS which is limited by two T1FS are
is LMF (lower membership function) and
is UMF (upper membership function). The membership function graph of interval type-2 trapezoidal fuzzy set (IT2TrS) can be seen in Figure with FOU (footprint of uncertainty) is uncertainty in primary membership function of IT2TrS
consists of a restricted area. FOU can be denoted by
.
Figure 1. Interval type-2 trapezoidal fuzzy set [Citation40].
![Figure 1. Interval type-2 trapezoidal fuzzy set K~~=(K~L,K~U) [Citation40].](/cms/asset/8331c4ef-85f2-41a1-abd5-748305c82e4d/tfie_a_1952760_f0001_ob.jpg)
The generalised interval type-2 trapezoidal fuzzy number (GIT2TrFN) define [
,
]. The value LMF is equal to
in
,
, respectively. Whereas, the value of UMF is equal to
in
,
, respectively. Therefore, GIT2TrFN
is denoted by
and its membership function as follows
and
For special case, if
and
then GIT2TrFN is called generalised interval type 2 trapezoidal flat fuzzy number and perfect IT2TrFN [Citation42].
Next, the arithmetic operation of GIT2TrFN is given as follows [Citation25,Citation42,Citation43]:
Definition 2.1
Given and
are GIT2TrFN and c is crisp number. Some arithmetic operations on GIT2TrFN explained as follows:
Addition:
Subtraction:
Multiplication:
where
Unique case, if
,
,
and
are flat generalised interval type-2 trapezoidal fuzzy number, then
Scalar multiplication:
if c ≥ 0 then
if c ≤ 0 then
Example 2.1
Let three perfect GIT2TrFN as follows
Based on Definition 2.1 we get that,
3. Ranking Methods for Interval Type-2 Trapezoidal Fuzzy Sets
The ranking process of interval type-2 trapezoidal fuzzy sets is divided into two steps. First step, interval type-2 trapezoidal fuzzy sets as determined as type-1 trapezoidal fuzzy sets by using reduction process. Second step, the ranking methods of type-1 trapezoidal fuzzy sets use to find equality of interval type-2 trapezoidal fuzzy sets [Citation18]. In the following, we present several ranking methods that are already in the references as well as the new proposed ranking.
3.1. Centroid of a Type-2 Fuzzy Set
Centroid of T2FS is union from all of centroid
embedded T1FS
[Citation18].
(5)
(5)
where
(6)
(6)
(7)
(7)
Karnik and Mendel [Citation18] presented the iterative algorithm to compute Equations (Equation6
(6)
(6) ) and (Equation7
(7)
(7) ). The iterative algorithm can be shown in Algorithm 1.
3.2. Indices of a Type-2 Fuzzy Set
Niewiadomski et al. [Citation44] presented proposed optimistic, moderate, pessimistic and weighted average indices which obtain different points of view for the type reduction of interval type-2 fuzzy sets. If is an interval-valued fuzzy set in the universe X. The indices reduction of optimistic from
denoted by
, moderate from
denoted by
and pessimistic from
denoted by
and weighted average from
denoted by
.
(10)
(10)
(11)
(11)
(12)
(12)
(13)
(13)
where
3.3. Lee and Chen's Ranking Value of Interval Type-2 Fuzzy Sets
Lee and Chen [Citation45] proposed the concept of ranking values GIT2TrFS. Given is GIT2TrFN, the ranking values that is denoted by
of
is defined as follows:
(14)
(14)
where
is the average of elements
and
with
,
,
is standard deviation of the elements
,
,
is membership degree of the element
in the trapezoidal membership function
and
.
3.4. Chen and Lee's Likelihood Ranking
Chen and Lee [Citation46] presented the following ranking method for type-2 fuzzy sets. They first calculate the likelihood of by Equation (Equation14
(15)
(15) )
(15)
(15)
Next, the Chen and Lee's likelihood ranking values for upper and lower membership functions are given by Equations (Equation15
(16)
(16) ) and (Equation16
(17)
(17) ), respectively:
(16)
(16)
and
(17)
(17)
and lastly, the Chen and Lee's likelihood ranking values of
be computed by Equation (Equation18
(18)
(18) )
(18)
(18)
3.5. Kahraman et al.'s Ranking
Kahraman et al. [Citation20] was adjusted the centre of area method Best Non-fuzzy Performance value for ranking IT2TrFS. They presented the ranked interval type-2 trapezoidal fuzzy set (RTrT) approach as follows:
(19)
(19)
3.6. The New Proposed Ranking
The modified total integral value is proposed to rank GIT2TrFN. The new proposed ranking uses the novel left and right of integral value as follows.
Given is GIT2TrFN with membership function on Equations (5) and (Equation6
(6)
(6) ). The novel left and right of integral value ranking consist of two membership functions based on LMF and UMF in accordance with the graph of GIT2TrFN membership function in Figure . The novel left and right of integral value based on LMF as follows.
(20)
(20)
(21)
(21)
where
,
and
Figure 2. The generalised interval type-2 trapezoidal fuzzy number [Citation42].
![Figure 2. The generalised interval type-2 trapezoidal fuzzy number [Citation42].](/cms/asset/e950665a-ff27-4530-8754-c90c98424fbd/tfie_a_1952760_f0002_ob.jpg)
The novel total integral value of LMF with index of optimistic or based Equations (Equation19
(20)
(20) ) and (Equation20
(21)
(21) ) as follows.
(22)
(22)
Meanwhile, the novel left and right of integral value based on UMF as follows.
(23)
(23)
(24)
(24)
where
,
and
The novel total integral value of UMF with index of optimistic or based Equations (Equation23
(23)
(23) ) and (Equation24
(24)
(24) ) as follows
(25)
(25)
Next, finding the average of novel total integral value as follows
(26)
(26)
Furthermore, given
and
. Then, Algorithm 2 can be used to compare
and
.
In the following four GIT2TrFN cases with different hight are given in Figure and by using the modified integral value in Algorithm 2 with moderate value of optimistic index or can be found
,
and
. So, the new proposed ranking gives ranking of 3a>3d>3c>3b of GIT2TrFN, whereas the existing rankings give ranking 3a = 3b = 3c = 3d [Citation18], 3a = 3b = 3c = 3d [Citation44], 3b>3a>3c>3d [Citation45,Citation46] and 3b>3a = 3c>3d [Citation20]. From the results of these ranking, the new proposed ranking succeeded to rank numerical examples of GIT2TrFN as with Lee and Chen's ranking [Citation45] and Chen and Lee's likelihood ranking[Citation46], while proposed ranking who is proposed by Karnik and Mendel [Citation18], Niewiadomski et al. [Citation44], and Kahraman et al. [Citation20] failed to rank numerical examples of GIT2TrFN. In detail, the comparison ranking result of the methods can be seen in Table .
Figure 3. Illustrative numerical examples of GIT2TrFN [Citation20].
![Figure 3. Illustrative numerical examples of GIT2TrFN [Citation20].](/cms/asset/62fc5e36-08b8-425b-8870-532afb9a86d9/tfie_a_1952760_f0003_oc.jpg)
Table 1. The comparison result of ranking methods.
4. The New Interval Type-2 Trapezoidal Fuzzy AHP
In this section, in order to the concept of interval type-2 trapezoidal fuzzy AHP can be easily understood, we will first give a brief overview of type-1 trapezoidal fuzzy AHP and its algorithms. Then, the new interval type-2 trapezoidal fuzzy AHP is presented.
4.1. Type-1 Trapezoidal Fuzzy AHP
Sometimes, subjective and qualitative decision criteria are uncertain, so that the decision makers find it difficult to express the strengths of preference and demonstrating the exact pairwise comparison. So. The crisp number is not suitable for representing the uncertainty of pairwise comparison. Therefore, the judgment of the decision maker or experts team is uncertain and imprecise. It will be much better to represent the value of pairwise comparison matrices as fuzzy numbers instead of crisp numbers. Due to the existing complexity and uncertainty of problems in the real life. Therefore, it is impossible to make decisions that can provide the exact judgment according to the problem [Citation43].
To overcome these shortcomings due to the crisp number, the fuzzy AHP was developed to solve MCDM. Prascevic and Prascevic [Citation16] developed a type-1 trapezoidal fuzzy AHP based on eigenvalues and expected value. The linguistic value of fuzzy AHP into type-1 trapezoidal fuzzy number on the criteria and alternatives in shown Table . The steps of Prascevic and Prascevic's fuzzy AHP are given in Algorithm 3.
Table 2. Linguistic scale type-1 trapezoidal fuzzy number for weight matrix [Citation6].
4.2. The New Interval Type-2 Trapezoidal Fuzzy AHP
In this section, Prascevic and Prascevic's type-1 trapezoidal fuzzy AHP method will be modified by using interval type-2 fuzzy sets. The linguistic value of fuzzy AHP into interval type-2 trapezoidal fuzzy number on the criteria and alternatives in shown Table . In detail, the steps of this fuzzy AHP method are explained in Algorithm 4.
Table 3. Linguistic scale generalised interval type-2 trapezoidal fuzzy number for weight matrix [Citation20].
5. In Practice
Based on the case study presented by Kahraman et al. [Citation20] regarding the problem of selecting a supplier with two alternatives (SP1 and SP2) and four criteria, namely price (PC), quality (QY), delivery (DV) and capacity(CP) with the hierarchy structure presented in Figure and the abbreviations of the linguistic variables are expressed in Table .
Figure 4. Illustrative the hierarchy of supplier selection problem [Citation20]
![Figure 4. Illustrative the hierarchy of supplier selection problem [Citation20]](/cms/asset/4cbfc08e-f2d8-4e33-8ebb-ebd0ae73ba87/tfie_a_1952760_f0004_ob.jpg)
We apply the steps of the proposed trapezoidal interval type-2 fuzzy AHP in Algorithm 4 as follows.
Creation of the pairwise comparison matrices. Tables and present criteria and alternatives of pairwise comparison matrices using linguistic variables term.
Consistency ratio test of pairwise comparison matrices for criterion (
) by using Equations (36) and (37) are obtained
Calculate fuzzy weight priority of criteria
by using Equation (39) are resulted
Obvious that using Algorithm 2,
and by Equations (45) and (46) with n = 4 is resulted CR = 0.1 such that pairwise comparison matrix of
is consistent.
Consistency ratio test of pairwise comparison matrices for alternatives
by solving Equation (47).
For J = PC, so
For J = QY, so
For J = DV, so
For J = CP, so
Clear that n = 2 such that RI = 0. Hence, all of
are 0 such that all of
pairwise comparison matrices are consistent.
Determine fuzzy weight vector of
by using Equation (48) are obtained
If j = PC then
If j = QY then
If j = PC then
If j = PC then
Calculate fuzzy weight global
by using Equations (43), (44) and (45) are obtained If
and
then
If
and
then
If
and
then
If
and
then
Calculate ranking of alternatives according to mean value (
) by using Equation (53) and Coefficient of variance (
) by using Equation (46). Moreover, ranking of alternatives based on the distance
by using Equation (59). The results of ranking for alternatives can be seen in Tables and . Based on these tables, supplier 2 is selected with the best ranking according to the mean value, the coefficient of variance and the distance of the original point to the centroid point.
In order to compare our results with Prascevic and Prascevic's type-1 fuzzy AHP, we solve the same problem using type-1 fuzzy sets. The comparison ranking Prascevic and Prascevic's type-1 fuzzy AHP and our type-2 fuzzy AHP can be seen in Table .
Table 4. The pairwise comparison matrix of criteria.
Table 5. The pairwise comparison matrix of alternatives.
Table 6. The result of ranking for alternatives based on mean value and STD.
Table 7. The result of ranking for alternatives based on the distance.
Table 8. The comparison ranking of type-1 and type-2 fuzzy AHP.
Both methods give different ranking results. Type-1 and type-2 fuzzy AHP use pairwise comparison matrices under fuzziness. But, type-2 fuzzy AHP sustains us to construct membership functions with the longest flexibility. Moreover, We also compare the type-2 fuzzy AHP proposed with type-2 fuzzy AHP was proposed by Kahraman et al. [Citation20] shown in Table and this table shows that the ranking result by Kahraman et al.'s fuzzy AHP equal to the new type-2 fuzzy AHP.
Table 9. The comparison ranking of existing and the new of type-2 trapezoidal fuzzy AHP.
6. Conclusion
Type-1 fuzzy sets have difficulties when constructing complex membership functions due to the many vagueness and fuzziness of the expert team's judgment. Type-2 fuzzy set as a problem solver. The modification of Prascevic and Prascevic's type-1 trapezoidal fuzzy AHP to be interval type-2 trapezoidal fuzzy AHP has been developed and discussed. Based on the study case provided, the ranking value produced by the new interval type-2 trapezoidal fuzzy AHP is equal to ranking value of Kahraman et al.'s fuzzy AHP and is different from the ranking value Prascevic and Prascevic's type-1 trapezoidal fuzzy AHP. Furthermore, there are limited number of ranking methods for trapezoidal interval type-2 fuzzy sets in the references. The modified total integral value is proposed and compared with the existing ranking methods. Based on the four numerical examples, the new proposed ranking is superior to three existing ranking methods and is equal to two existing methods.
Disclosure statement
No potential conflict of interest was reported by the author(s).
Additional information
Funding
Notes on contributors
Muhammad Sam'an
Muhammad Sam'an received Bachelor Degree from Universitas Negeri Semarang and Master Degree from Universitas Diponegoro in Mathematics 2010 and 2016 respectively and now, he is studying in the postgraduate student, Faculty of Technology Management and Business, Universiti Tun Hussein Onn Malaysia (UTHM). His research interests are in Optimization, Fuzzy Mathematics and Computational Mathematics.
Yosza Dasril
Yosza Dasril received PhD and Master degree in Applied Mathematics from Universiti Putra Malaysia in 2003 and 1999 respectively and Bachelor Degree in Mathematics from Universitas Riau, Indonesia in 1994. From 1999 to 2006, he was a lecturer in the Universiti Malaysia Terengganu (UMT). In the middle of 2006 into Nov 2019, he serve as a Senior Lecturer at the Faculty of Electronic and Computer Engineering, Universiti Teknikal Malaysia Melaka (UTeM). Currently, he is a Senior Lecturer at Faculty of Technology Management and Business, Universiti Tun Hussein Onn Malaysia (UTHM). His research interests are in Optimization, Engineering Mathematics and Computational Mathematics.
Much Aziz Muslim
Much Aziz Muslim received Bachelor Degree from Universitas Stiekubank, Semarang and Master Degree from Universitas Gadjah Mada in computer science and now, he is studying in the postgraduate Student, Faculty of Technology Management and Business, Universiti Tun Hussein Onn Malaysia (UTHM) and a lecturer in the Universitas Negeri Semarang. His research interests are in Optimization and Data mining.
References
- Tseng ML, Lin YH, Chiu AS, et al. Fuzzy AHP-approach to TQM strategy evaluation. Ind Eng Manag Syst. 2008;7:34–43.
- Kahraman C, Ruan D, Dogan I. Fuzzy group decision-making for facility location selection. Inform Sci. 2003;157:135–153.
- Saaty TL. The analytic hierarchy process: planning, priority setting, resource allocation. New York: McGraw-Hill; 1980.
- Chen SJ, Hwang CL, Hwang FP. Fuzzy multiple attribute decision making: methods and applications. Berlin: Springer; 1992.
- Van Laarhoven PJM, Pedrycz WA. Fuzzy extension of Saaty's priority theory. Fuzzy Sets Syst. 1983;11:229–241.
- Chang DY. Extent analysis and synthetic decision, optimization techniques and applications. Singapore: World Scientific; 1992.
- Chang DY. Application of the extent analysis method on fuzzy AHP. Eur J Oper Res. 1996;95:649–655.
- Mikhailov L. A fuzzy programming method for deriving priorities in analytic hierarchy process. J Oper Res Soc. 2000;51:341–349.
- Csutora R, Buckley JJ. Fuzzy hierarchical analysis: the Lambda-Max method. Fuzzy Sets Syst. 2001;120:181–195.
- Buckley JJ, Eslami E, Feuring T. Fuzzy mathematics in finance and engineering. Berlin: Springer; 2002.
- Mikhailov L. Group prioritization in the AHP by fuzzy preference programming method. Comput Oper Res. 2004;31:293–301.
- Wang JM, Chin KS. An eigenvector method for generalizing normalized interval and fuzzy weights. Appl Math Comput. 2006;181:1257–1275.
- Wang JM, Chin KS. Fuzzy analytic hierarchy process: a logarithmic fuzzy preference programming methodology. Int J Approx Reason. 2011;52:541–553.
- Wang ZJ. Consistency analysis and priority derivation of triangular fuzzy preference relations based on modal value and geometric mean. Inform Sci. 2015;314:169–183.
- Prascevic Z, Prascevic N. Application of fuzzy AHP method based on eigenvalues for decision making in construction industry. Tech Gazette. 2016;23:57–64.
- Prascevic Z, Prascevic N. Application of fuzzy AHP for ranking and selection of alternatives in construction project management. J Civil Eng Manag. 2017;23:1123–1135.
- Zadeh LA. The concept of a linguistic variable and its application to approximate reasoning-I. Inform Sci. 1975;8:199–249.
- Karnik NN, Mendel JM. Operations on type-2 fuzzy sets. Fuzzy Sets Syst. 2001;122:327–348.
- Wu DR, Mendel JM. Aggregation using the linguistic weighted average and interval type-2 fuzzy sets. IEEE Trans Fuzzy Syst. 2007;15:1145–1161.
- Kahraman C, Oztaysi B, Sari IC, et al. Fuzzy analytic hierarchy process with interval type-2 fuzzy sets. Knowl Based Syst. 2014;59:48–57.
- Mendel JM. An architecture for making judgements using computing with words. Int J Appl Math Comput Sci. 2002;12:325–335.
- Ozen T, Garibaldi JM. Effect of type-2 fuzzy membership function shape on modelling variation in human decision making. In: Proceedings of the IEEE International Conference on Fuzzy Systems; Budapest, Hungary; 2004. p. 971–976.
- Sevastjanov P, Figat P. Aggregation of aggregating modes in MCDM: synthesis of type-2 and level-2 fuzzy sets. Omega. 2007;35:505–523.
- Wu D, Mendel JM. Uncertainty measures for interval type-2 fuzzy sets. Inform Sci. 2007;177:5378–5393.
- Yager RR. Fuzzy subsets of type-2 in decisions. J Cybern. 1980;10:137–159.
- Huynh VN, Nakamori Y, Lawry J. A probability-based approach to comparison of fuzzy numbers and applications to target-oriented decision making. IEEE Trans Fuzzy Syst. 2008;16:371–387.
- Chen SY, Wang CY. Fuzzy decision making systems based on interval type-2 fuzzy sets. Inform Sci. 2013;242:1–21.
- Kahraman C, Oztaysi B, Sari IC, et al. Fuzzy analytic hierarchy process with type-2 fuzzy sets. In: World Scientific Proceedings Series on Computer Engineering and Information Science Uncertainty Modeling in Knowledge Engineering and Decision Making; 2012. p. 201–206.
- Chiao KP. Interval type-2 fuzzy sets extension of analytic hierarchy process with application to new product development project screening. In: Proceedings of the IEEE International Conference on Fuzzy Theory and its Applications; Kaohsiung, Taiwan; 2014. p. 111–116.
- Oztaysi B, Cevik Onar S, Kahraman C. Prioritization of business analytics projects using interval type-2 fuzzy AHP. In: Springer International Conference on European Society for Fuzzy Logic and Technology; Warsaw, Poland; 2017. p. 106–117.
- Pane ES, Wibawa AD, Purnomo MH. Event log-based fraud rating using interval type-2 fuzzy sets in fuzzy AHP. In: Proceedings of the IEEE International Conference on TENCON; Singapura, Singapura; 2016. p. 1965–1968.
- Ecer F. Multi-criteria decision making for green supplier selection using interval type-2 fuzzy AHP: a case study of a home appliance manufacturer. Oper Res Int J. 2020. doi:https://doi.org/10.1007/s12351-020-00552-y.
- Yildiz N, Kahraman C. Evaluation of social sustainable development factors using Buckley's fuzzy AHP based on Z-numbers. In: Springer International Conference on Intelligent and Fuzzy Techniques in Big Data Analytics and Decision Making; Istanbul, Turkey; 2020. p. 770–778.
- Riaz M, Hashmi MR. Linear Diophantine fuzzy set and its applications towards multi-attribute decision making problems. J Intell Fuzzy Syst. 2019;37:5417–5439.
- Celik E, Akyuz E. An interval type-2 fuzzy AHP and TOPSIS methods for decision-making problems in maritime transportation engineering: the case of ship loader. Ocean Eng. 2018;155:371–381.
- Yonar A, Pehlivan NY. Evaluation of life quality by integrated method of AHP and TOPSIS based on interval type-2 fuzzy sets. Hacettepe J Math Stat. 2017;46:511–523.
- Yilmaz H, Kabak O. Prioritizing distribution centers in humanitarian logistics using type-2 fuzzy MCDM approach. J Enterp Inf Manag. 2020;33:1199–1232.
- Ozdemir YS, Uskudar A. Strategy selection by using interval type-2 fuzzy MCDM and an application. J Eng Res. 2020;8:172–189.
- Abbasimehr H, Nourani E, Shabani M. A hybrid framework for ranking reviewers based on interval type-2 fuzzy AHP and VIKOR. Int J Intell Eng Inform. 2020;8:95–116.
- Mendel JM, John RI, Liu F. Interval type-2 fuzzy logic systems made simple. IEEE Trans Fuzzy Syst. 2006;14:808–821.
- Jain R. Decision-making in the presence of fuzzy variables. IEEE Trans Syst Man Cybern. 1976;6:698–703.
- Javanmard M, Mishmast Nehi H. Rankings and operations for interval type-2 fuzzy numbers: a review and some new methods. J Appl Math Comput. 2019;59:597–630.
- Wang YJ, Lee HS. The revised method of ranking fuzzy numbers with an area between the centroid and original points. Comput Math Appl. 2008;55:2033–2042.
- Niewiadomski A, Ochelska J, Szczepaniak PS. Interval-valued linguistic summaries of databases. Control Cybern. 2006;35:415–443.
- Lee LW, Chen SM. Fuzzy multiple attributes group decision-making based on the extension of TOPSIS method and interval type-2 fuzzy sets. In: Proceedings of the 2008 International Conference on Machine Learning and Cybernetic; Kunming, China; 2008. p. 3260–3265.
- Chen SM, Lee LW. Fuzzy multiple attributes group-decision making based on the ranking values and the arithmetic operations of interval type-2 fuzzy sets. Expert Syst Appl. 2010;37:824–833.
- Cheng CH. A new approach for ranking fuzzy numbers by distance method. Fuzzy Sets Syst. 1998;95:307–317.