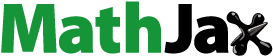
ABSTRACT
Research question
The purpose of this paper is to examine the factors affecting broadcasters’ choice of matches and audience viewership of live Chinese Super League (CSL) matches.
Research methods
Data came from 720 matches spanning 2017–2019, with 122 selected for CCTV5 telecasts. Broadcasters’ match selections and TV ratings were regressed on antecedent factors of the demand for televised CSL matches.
Results and findings
The broadcaster selection model showed broadcasters’ preferences for higher outcome uncertainty, higher athlete salaries, higher league standings, and teams contending for the CSL Championship. The viewership model indicated higher TV ratings for matches with larger fan bases, higher athlete salaries, higher-ranked teams, and those held on weekends or at night.
Implications
This study expands football media demand research and is one of the few to explore match selection and viewership factors in CSL. It offers practical insights for sport broadcasters and league managers in Asian and global contexts.
Introduction
The European-centered international football market has been expanding into Asia, in which process the evolution of the Chinese Super League (CSL) has played a significant role. CSL was launched in 2004 and has grown exponentially since 2015, when the government of China issued the National Plan for Chinese Football Reform and Development, or simply the Reform Plan, an ambitious plan to develop Chinese football to reach the highest level internationally (Cockayne et al., Citation2022; Peng et al., Citation2019).
The Reform Plan, as recognized by Peng et al. (Citation2021), was radical in its approach to balance football development across all levels, from grassroots to elite. It reduced government intervention and standardized professional club management (Peng et al., Citation2019). For instance, starting in the 2017 season, a maximum of three foreign players, regardless of Asian or non-Asian status, were allowed on the field. Additionally, in the 2018 season, the 18-player roster required at least three U-23 players, with two from the U-21 age group. These changes, initiated by the Chinese Football Association (CFA), aimed to curb excessive spending on foreign players to foster a nurturing environment for local talent (Peng et al., Citation2022).
As a result, CSL is now more visible than ever in the global football market, as reflected in various indices such as an average of 35% growth rate of CSL sponsorship investments, totaling 96 million USD in 2020 (Deloitte, Citation2020), and the enormous sum of 165 million USD acquired from selling CSL’s media rights for the 2016–2026 seasons (Xinhua News, Citation2018). The growth potential of CSL is evident. A clear understanding of fan behaviors, especially the TV demand for CSL matches, is of great importance for both managers of sport organizations who need to promote CSL matches to football fans and for sport marketers of international corporations attempting to leverage CSL as a marketing vehicle to target the vast Chinese market with its 1.4 billion population.
To date, only three studies (Li et al., Citation2022; Watanabe & Soebbing, Citation2017; Watanabe et al., Citation2019) have examined the demand for CSL matches, but their focus was on stadium attendance. Although attendance demand studies provide some useful insights to understand the TV demand for sport matches, there is a fundamental difference between stadium attendance and media viewership in that time and financial constraints are less binding on TV viewers than stadium attendees (Sung et al., Citation2019). Additionally, media demand studies need to be conducted within each context where a sport league operates because the socioeconomic environment associated with leagues in each respective country may drive different patterns of media consumption (Ryu et al., Citation2019). This calls for research to investigate CSL media demand.
Expanding TV sport demand research, this study explores factors influencing broadcasters’ match selection and TV ratings for live CSL matches on CCTV5 in China. This study contributes in three specific ways to the literature on sport demand. First, given the dearth of work on the media demand for professional football in China, at the specific time especially after the 2015 Reform Plan, the current study aims to investigate the factors affecting broadcasters’ match selection and TV ratings of CSL matches using the Heckman selection model to control for potential selection bias (Heckman, Citation1976, Citation1979). Furthermore, this study specifically focuses on CCTV5, which distinguishes itself from traditional sport broadcast companies as a state-affiliated entity with a significant market presence in China. This unique characteristic offers valuable insights into the consumption patterns within the dynamically evolving Chinese football market. Lastly, this study investigates the influence of several important yet under-researched factors in the sport demand literature. These factors include the sum and difference in goals, as well as measures of both intra-match and intra-league competitive intensity (CI) variables.
Literature review
TV audience demand for sport involves two steps: Broadcasters must choose matches from the media rights package, and viewers must select the TV channel. To analyze televised sport audience demand, both steps must be considered.
Broadcasters’ selection of team sport matches
Sport broadcasters have two interconnected roles: buying media rights from sport organizations and supplying sport programs to TV audiences (Bolotny & Bourg, Citation2006; Evens et al., Citation2013; Feuillet et al., Citation2019). Their aim to maximize revenue by selling advertising spots and acquiring program sponsorship are primarily determined by program exposure. From this perspective, broadcasters’ choice of matches for telecasts should follow the TV audience demand pattern.
However, the patterns of broadcasters’ match selections may not be as consistent with actual TV viewers’ demand. This is because broadcasters need to consider the opportunity costs associated with the selection of sport matches (Gratton & Solberg, Citation2007), which can lead them to select other programs than sport if such programs are expected to attract more TV viewers. Further, although this is less relevant to CCTV5 in the Chinese sport broadcasting market, broadcasters may simply not have access to the best games because they did not obtain the best packages, in the hand of a competitor (Feuillet & Scelles, Citation2022; Feuillet et al., Citation2019; Scelles et al., Citation2020). Therefore, an empirical investigation is needed to correctly understand factors affecting broadcasters’ match selections.
Limited research exists on the factors affecting broadcasters’ match selections. Forrest et al. (Citation2005, Citation2006) studied British broadcasters’ preferences for English Premier League (EPL) matches. They found that broadcasters favored EPL championship contenders, high-ranked teams, and games with uncertain outcomes. Additionally, they tended to choose matches with higher total wage sum, balanced team wages, local rivalries, and weekend schedules. García and Rodríguez (Citation2006) studied Spanish football match selections for telecasts during the 2000/2001 to 2002/2003 seasons. They discovered that higher relative spending (team spending divided by the season's mean total spending) and derby match status positively influenced broadcaster choices. Additionally, the population of both teams’ provinces and their league positions played significant roles in broadcaster decisions, while outcome uncertainty had no impact.
Buraimo and Simmons (Citation2015) analyzed eight seasons of EPL data using the Heckman selection model. They found that broadcasters favored matches with higher relative wages, smaller absolute differences in the relative wages, and derby status. Additionally, games with larger attendance and combined points-per-game, but smaller differences in points-per-game from the previous season, were more frequently chosen for broadcast. Outcome uncertainty did not play a role in match selection. Most recently, Kim et al. (Citation2022) investigated broadcasters’ choice of matches for live baseball telecasts. They analyzed a panel data set from the 2018 Korea Baseball Organization league pennant race by regressing broadcasters’ choice order of matches and the TV ratings on a series of antecedent factors related to game characteristics and audience preferences. The researchers found that broadcasters’ choices were based on popularity and team performance/quality. Regarding the uncertainty of outcome hypothesis, the broadcasters tended to choose games with more certain, rather than uncertain, outcomes.
Factors affecting TV viewership of team sport matches
TV ratings are typically a proxy for television viewers’ demand for sport events (Pérez et al., Citation2017; Tainsky & Jasielec, Citation2014). The earliest studies in TV ratings concerned broadcasting as a potential substitute for live attendance at stadiums (Baimbridge et al., Citation1996). This stream has since evolved into a diverse body of literature on TV audiences’ media consumption patterns for various team sport such as American football (Brown & Salaga, Citation2018), association football (Bond & Addesa, Citation2019), basketball (Mongeon & Winfree, Citation2012), and ice hockey (Paul & Weinbach, Citation2013).
Factors influencing TV sport event ratings derive from attendance research. TV demand studies have widely examined the factors identified by Borland and MacDonald (Citation2003) and Villar and Guerrero (Citation2009) except for the factors that are only applicable to the gate attendance context (e.g. seating quality). Factors, such as consumer preference (e.g. fan loyalty), competition attributes (e.g. outcome uncertainty, derby, rivalry), team attributes (e.g. star players, team rank), economic factors (e.g. substitutes, population size), and external factors (e.g. weather, schedule) have been widely examined in TV demand studies.
Tainsky (Citation2010) made one of the first attempts to apply the logic found in attendance research to TV viewership study, finding team quality, market tenure, and prime-time games boosted ratings, while income had a negative impact. Outcome uncertainty has been one of the central issues investigated in televised football demand studies. However, the empirical evidence does not provide consistent results, with some results supporting the outcome uncertainty hypothesis (Schreyer et al., Citation2018) and others reporting mixed (Buraimo & Simmons, Citation2015; Schreyer et al., Citation2017) or no support (Jang & Lee, Citation2022; Li et al., Citation2022; Sung et al., Citation2019).
Apart from outcome uncertainty, various approaches have been adopted to investigate the media demand for sporting events. Bond and Addesa (Citation2019) explored the impact of competitive intensity (CI) on TV viewership for Italian Serie A football matches, linking CI to teams’ contention for top prizes. All eight CI factors showed an increase in Italian spectators when both teams were vying for a significant championship. These results differed from those of Scelles (Citation2017), who found positive impacts only for title or Champions League-contending EPL matches. Pérez et al. (Citation2015) investigated the “general interest’ hypothesis in Spain's La Liga, examining whether regional viewership decreased when local teams were not playing. Panel regression results revealed no nationwide” ‘general interest’ except for matches involving Real Madrid CF, FC Barcelona, and local teams.
Barajas et al. (Citation2019), Mongeon and Winfree (Citation2012), and Wang et al. (Citation2018) combined TV viewership and stadium attendance in their studies. Barajas et al. (Citation2019) investigated stadium attendance's impact on TV audience size in Brazilian football, noting a positive correlation, but higher ticket prices increased TV audiences, while weekend matches saw smaller TV audiences. Mongeon and Winfree (Citation2012) compared TV and gate demand for NBA games, finding TV viewers were 4.5 times more sensitive to winning. TV audience demand decreased more with direct NBA team substitutes than gate demand, while indirect substitutes (other professional sport teams) had a greater impact on gate demand than TV audience demand. Wang et al. (Citation2018) studied Belgian football fans’ preferences for TV and stadium match scheduling, using a discrete choice experiment to assess match settings based on month, kickoff time, and opponent quality.
Gasparetto and Barajas (Citation2018) compared football broadcast demand in two Brazilian media markets, finding mostly similar patterns, with higher viewership on weekdays and for derby matches. However, one market exhibited loss aversion, while the other preferred certain wins. More recently, Wills et al. (Citation2022) analyzed TV audience determinants for the UEFA Champions League (UCL) in six major European markets (France, Germany, Italy, the Netherlands, Spain, and the United Kingdom) from 2013/14 to 2018/19. They found that outcome uncertainty had no impact on TV audience size, but the presence of star players and team quality correlated with increased TV audience demand.
Study context
This study examines factors influencing broadcaster match selections and audience viewership for 2017–2019 CSL seasons. CSL is China’s premiere professional football league, encompassing the Chinese Football Association China League (CCL), Chinese Football Association Division Two League (China League Two), and the Chinese Football Association Member Association Champions League (CMCL). It features a 16-team double round-robin format, with the bottom two teams relegated to CCL and the top two promoted. Points determine rankings (3 for win, 1 for tie, 0 for loss).
The top sporting prizes in the CSL, i.e. champion and qualification in the AFC men’s Champions League, were awarded to three to four teams via the CSL over 2017-2019. Only three teams qualified for the AFC men’s Champions League via the CSL if the Chinese FA Cup winner did not belong to the top four, e.g. Shanghai Greenland Shenhua in 2017 and 2019. Also, in 2018 and 2019, there was an incentive to be the 2nd in CSL to qualify to the group-stage of AFC men’s Champions League, rather than the 3rd which offered qualifications to play-off round only.
CSL matches are televised on CCTV5, China Central Television’s (CCTV) free sport channel. CCTV is the national television station of the People’s Republic of China and comprises a total of 59 free and pay-channels, out of which two channels mainly broadcast sport: the free CCTV5 and the pay-channel CCTV5 + . In 2016, China Sports Media Co., Ltd., bought the full media rights to CSL for 2016–2020 and distributed them to various channels, including CCTV5. CCTV5 is the most influential channel because it is the only free channel accessible from all parts of China. CCTV5 and China Sports Media Co., Ltd., reached an agreement that CCTV5 can flexibly choose to broadcast from among 240 available matches in each football season.
Methods
Model
Viewership of televised sport involves two sequentially related decisions: broadcasters choosing matches and audiences tuning in. These decisions are interdependent, creating two related selection bias issues: the first is selectivity from available matches by rights-holding broadcasters, and the second is match selections and related factors’ influence on the audience demand for televised matches as a latent variable. Owing to selection-based sampling, potential bias exists.
CCTV5 televises only a subset of available football matches in each season. If there is a difference in the characteristics of televised and non-televised matches, an endogeneity problem will occur. According to Heckman (Citation1976), if a dependent variable is estimated only from a set of nonrandom observations, then the model estimated may be biased; Heckman correction for sample selection accounts for sample-induced endogeneity (Certo et al., Citation2016; Heckman, Citation1976; Kennedy, Citation2003). Therefore, this study used the Heckman selection model to examine determinants of broadcasters’ match selections and TV ratings of televised matches.
CCTV5 televises only a subset of available football matches in each season, potentially causing an endogeneity issue if there are differences between televised and non-televised games. To address this concern, we employed the Heckman selection model, as recommended by Heckman (Citation1976) and utilized in prior research (Certo et al., Citation2016; Kennedy, Citation2003), to account for sample-induced endogeneity.
Using the Heckman selection model, broadcasters’ match selection decisions were estimated at the first stage, and the determinants of TV ratings were estimated consecutively at the second stage. Therefore, the Heckman selection model (Heckman, Citation1976, p. 1979) was a two-stage model comprising the selection model (i.e. broadcaster selection model) and the outcome model (i.e. viewership model).
The first stage identified the propensity of broadcasters’ match selections, employing a probit regression model, as follows:
(1)
(1) where
denotes the latent variable regarding broadcasters’ choice
, the observed counterpart of
;
denotes the vector of coefficients;
denotes the independent variables; and
denotes the error term.
Heckman controlled selection bias by constructing the inverse Mills ratio (IMR) in EquationEquation (1)(1)
(1) . As a correction factor, IMR,
, is expressed as follows:
(2)
(2) where the ratio represents the standard normal density by the standard normal cumulative distribution.
The second stage identified the determinants of TV ratings; the estimation model is as follows:
(3)
(3) where
represents the observed log transformed TV ratings when
of the selection model equals 1,
represents the coefficients,
represents the explanatory variables, and
represents the error term with normal distribution. Because
in EquationEquation (1)
(1)
(1) and
in EquationEquation (3)
(3)
(3) are systemically correlated upon the selection bias, the conditional expectation of
is given by the following:
(4)
(4)
where
indicates the correlation coefficient,
indicates the adjusted standard error, and
indicates the estimated coefficient of selection bias. This IMR,
, is incorporated as an exogeneous independent variable in the second phase so that the estimates can be interpreted directly, free of selection bias.
When using the Heckman selection model, if the IMR is non-significant in the second-stage linear regression model, it indicates that there is no selection bias. This in turn suggests that the estimates from the outcome model are not significantly different from those that would otherwise be derived from ordinary least squares (OLS) regression. In the current study, besides the results of Heckman selection model, we also report the estimates derived from the OLS regression. Before using OLS for data analysis, Breusch–Pagan test for heteroskedasticity was performed, which showed insignificant signs of variability in the residuals, χ2(20) = 25.29 (p = 0.191), fulfilling the assumption of OLS regression. Because the number of observations in the TV ratings models was rather limited (N = 122), OLS was applied followed by wild bootstrap standard errors (SEs) with 100,000 replications (Scelles, Citation2017).
Sample and Variables
CSL match records were collected for the 2017–2019 seasons. CSL has 16 teams, each playing 30 rounds of matches per season, resulting in 240 matches per season. Therefore, the total number of matches played during the three seasons was 720, of which 122 matches were televised on CCTV5 with national coverage. All data were collected at the individual match level.
The variables were constructed over two different stages of regression based on the Heckman selection model. The first stage involved applying the variables used in the selection model, and the second stage involved applying the variables used in the outcome model. shows the list of variables and their operational definitions.
Table 1. List of variables and operational definitions.
In the first stage with the probit model, the binary dependent variable CCTV5 was coded by assigning a value of “1” if the broadcaster selected a match, and “0” otherwise. With the bias-corrected linear model, the second dependent variable TVR was the log-transformed TV ratings of each televised match. TV ratings data were collected from KUYUN (http://pro.eye.kuyun.com), a leading multimedia big data service company recording real-time TV ratings in China. TV ratings represented the average of the minute-by-minute TV ratings over the duration of a game.
The explanatory variables in were applied differently in the first- and second-stage regression models, reflecting the exclusion restriction of the sequential regression model (Huber & Mellace, Citation2014; Morrissey et al., Citation2016). Except for one variable related to teams’ number of followers on Weibo, China’s leading social network service, all explanatory variables related to the CSL matches were collected from the official website of CFA (www.thecfa.cn). The number of Weibo followers were recorded directly from Weibo’s website (https://m.weibo.cn).
At the selection stage of the Heckman model, the independent variables included variables related to fandom (i.e. WEIBO), competition attributes (i.e. DERBY, THEIL, CSLTITLE, CSLQ, CFAQ, AFCQ, and RELEGATION), team attributes (i.e. AFC, SALARY, RANK, and STARP), match schedule (i.e. WEEKEND, NIGHT, YEAR2017–2019), and substitution (CBA). Additionally, four interaction terms were included in the independent variables of the selection model to capture the previous season’s fandom and on-field performance for the first three rounds of matches; this is because not much information is available for broadcasters to use in the early stage of each season, causing them to rely on past seasons’ records. Specifically, a value of “1” was assigned to the variable 3ROUNDS if a game was held in the first three rounds of a season, and a value of “0” was assigned otherwise. Then, four interactions terms were created by multiplying 3ROUNDS by each of the four variables reflecting the last season’s fandom (i.e. LATT) and on-field team performances (i.e. LRANK, LGOALS, and LDWIN).
At the outcome stage, a set of variables likely to affect TV ratings related to fandom (i.e. WEIBO), competition attributes (i.e. SGOAL, DGOAL, DERBY, THEIL, FLUCTUATION, CSLTITLE, CSLQ, CFAQ, AFCQ, and RELEGATION), team attributes (i.e. AFC, SALARY, RANK, and STARP), match schedule (i.e. WEEKEND, NIGHT, and YEAR2017–2019), and substitute (CBA) were included as independent variables.
Although provides details of each variable, we provide additional explanations about the two variables related to goals in the outcome model, that is, the sum of and difference in goals scored at the end of the game (i.e. SGOAL and DGOAL). One may argue that TV viewers do not find out this information until the end of the game, so it should not affect TV ratings. However, TV ratings represent the number of TV viewers who watched at least part of the game, including those who watched only the very end of the game. In this case, the use of the two goal variables may affect TV ratings.
Furthermore, both intra-match and intra-league competitive intensity (CI) variables were incorporated. The variable FLUCTUATION, which measures the number of times the score shifted from a draw to one team leading and from one team leading to a draw (Scelles et al., Citation2011), was included to represent intra-match CI, which has not been formally examined in the existing literature.
In terms of intra-league CI, five variables (CSLTITLE, CSLQ, CFAQ, AFCQ, and RELEGATION) were included. These variables indicated whether the game was a positional battle to secure one of the significant sporting prizes in the CSL (such as CSL Championship, FA Cup Championship, and AFC entry), or whether the game determined teams to be relegated to a lower division league. The five intra-league CI variables were applied only for the last 10 rounds, as games played earlier in the season have less relevance to the sporting prizes.
Following the methodology employed in Bond and Addesa (Citation2019) and Scelles (Citation2017), the five intra-league CI variables were measured using three different approaches, referred to as Index 1 through 3, respectively, in . Firstly, each intra-league CI variable was assigned a value of “4” if the outcome of the current game alone had the potential to change the position of the teams vying for the respective sporting prizes. A value of “2” was assigned if the results of the current game and the subsequent game combined could affect the teams’ positions. A value of “1” was assigned if the results of the current game, the next game, and the second subsequent game together could influence the teams’ positions. All other games were assigned a value of “0”. For instance, a game would be assigned a value of “4” if the first and second-place teams were contending for the CSL Championship, and the point difference between them was only two prior to the game, indicating that the game's outcome could impact the top-ranked team. Secondly, a value of “2” was assigned if the outcome of the current game alone could alter the teams’ positions in the race for the respective sporting prizes. A value of “1” was assigned if the results of the current game and the subsequent game combined could affect the teams’ positions. All other games were assigned a value of “0”. Thirdly, a value of “1” was assigned if the outcome of the current game alone had the potential to change the teams’ positions in the competition for the respective sporting prizes. All other games were assigned a value of “0”.
Table 2. Descriptive statistics of variables.
Findings
Descriptive statistics
presents the descriptive statistics of the key variables. It appeared that the TV ratings of CSL matches ranged from .13% to 1.09%, representing 1.8–15.2 million audience members based on 96.4% TV households among the 1.39 billion population of China in 2019 (Ding, Citation2019).
Inspection for Selection Bias
reports the analyses results. The estimate of IMR (λ), the correction variable for selection bias, did not reach a statistically significant level (λ = .466, p > .10), failing to reject the null hypothesis that the correlation between the error terms from the selection and outcome equations was not significantly different from zero. The IMR estimate indicated that there were no discerning features between matches that were broadcast and those that were not; these findings suggested that there was no selection bias in the sample. Thus, the outcome model results of the Heckman selection model should reveal a similar pattern to the results of the OLS regression.
Table 3. Results of Heckman selection and OLS models followed by wild bootstrap SEs.
Selection model for broadcasters’ match selection
The results of the selection model in indicate that five variables (i.e. THEIL, CSLTITLE, SALARY, RANK, and YEAR2019) controlled by the model had significant influences on broadcasters’ match selection.
THEIL (β = .659, p < .10), CSLTITLE (β = .061, p < .05), and SALARY (β = .556, p < .05) had a positive impact on broadcasters’ match selection; these findings indicate that broadcasters were more inclined to select games characterized by higher levels of outcome uncertainty and featuring teams competing for the CSL Championship, as well as games involving teams with higher average athlete salaries.
RANK (β = - .063, p < .01) appeared to have a negative impact on broadcasters’ match selection; that is, the higher the combined rank (i.e. smaller rank scores) of the two competing teams, the more likely broadcasters were to select the games. YEAR2019 (β = − .541, p < .05) had a negative impact on broadcasters’ match selection, suggesting that a smaller number of games were chosen for telecasts in 2019 compared to 2017. All other independent variables were not significant.
OLS models for TV ratings
Following the non-significant estimate of IMR (λ), the correction variable for selection bias (λ = .466, p > .10), OLS regression models were employed, and wild bootstrap standard errors (SEs) with 100,000 replications were utilized. The analysis in presents three regression models, with each model utilizing one of the three indices for each intra-league CI variable (CSLTITLE, CSLQ, CFAQ, AFCQ, and RELEGATION) as described in the method section.
As shown in , the results of the three models were similar. In Models 1 and 2, seven explanatory variables (WEIBO, SALARY, RANK, WEEKEND, NIGHT, YEAR2018, and YEAR2019) showed significant effects on TV ratings in the same direction. In Model 3, all the variables significant in Models 1 and 2, except for WEIBO, remained significant in Model 3 as well.
Interpreting the results of Model 1, WEIBO (β = .135, p < .10), SALARY (β = .266, p < .05), WEEKEND (β = .187, p < .05), and NIGHT (β = .656, p < .01) had positive effects on TV ratings. These findings suggest that games played by teams with a larger number of WEIBO followers and a higher sum of athlete salaries, as well as games held on weekends and nights, were associated with higher TV ratings. RANK (β = - .011, p < .05), YEAR2018 (β = − .260, p < .01), and YEAR2019 (β = − .161, p < .05) showed negative effects on TV ratings. These findings indicate that a higher combined rank (i.e. smaller rank scores) of the two competing teams was associated with higher TV ratings, and that TV ratings in 2018 and 2019 were lower compared to those in 2017, respectively.
Discussion
Comparison with previous literature and specificities of the CSL
This study showed that broadcasters and TV audiences preferred to choose and watch matches between teams that were higher ranking, and teams with higher salaries; also, for TV audiences, there was a higher demand for televised games featuring matches between teams with a larger fan base. These results are consistent with those of previous studies on audience demand for televised football matches (Mongeon & Winfree, Citation2012; Tainsky, Citation2010; Wills et al., Citation2022).
The viewership model showed that the audience demand for televised CSL matches was greater for night games (after 6 p.m), and weekend matches. The results of previous research suggest that prime-time matches are associated with higher TV ratings (e.g. Schreyer et al., Citation2018; Tainsky, Citation2010; Wang et al., Citation2018); these results are consistent with those of the current study. The literature presents mixed findings regarding the association between TV audiences and weekend versus weekday games. Barajas et al. (Citation2019) and Gasparetto and Barajas (Citation2018) reported higher TV ratings for weekday matches, while Buraimo and Simmons (Citation2015) and Scelles (Citation2017) found lower ratings for weekday games. Wang et al. (Citation2018) even advised avoiding Wednesday matches. These differences should be considered in the context of each study. In this study, weekend games had higher ratings, likely due to China's demanding workdays and limited leisure time for TV sport on weekdays.
Unlike TV viewers, broadcasters showed no preference for night or weekend games. This can be attributed to the CSL's position in the Chinese sport broadcasting market. In China, prime-time and weekend TV programs garner the largest audiences. CCTV5, the dominant sport channel, mainly televises popular sports like CBA and NBA games, given basketball's immense popularity in China. Consequently, CCTV5 broadcasters prioritize events, typically excluding CSL matches, that promise higher prime-time and weekend TV ratings.
Outcome uncertainty, as measured by the Theil index, affected broadcasters’ match selection but not the TV ratings. The results regarding the preferences among broadcasters for choosing matches with greater outcome uncertainty were consistent with those of Forrest et al. (Citation2005, p. Citation2006), whereas the null effect of outcome uncertainty on TV ratings was consistent with recent studies on football TV demand (Caruso et al., Citation2019; Schreyer et al., Citation2018; Sung et al., Citation2019), showing that TV viewers have no apparent demand for matches with greater outcome uncertainty.
Neither goal variable (i.e. SGOAL and DGOAL) was significant. This might be because complete information on SGOAL and DGOAL is not available until the game is over, so their influences on TV ratings were restricted under the statistically significant level. Additionally, even those viewers who often watch only the last part of a game may be unlikely to do so if there are many goals for the same team (no outcome uncertainty), or if there is no goal difference (outcome uncertainty) but 0-0 (which may suggest a boring game), consistent with earlier findings by Alavy et al. (Citation2010).
The status of a derby match did not impact broadcasters’ match selection or TV ratings. The literature presents mixed findings regarding the effects of derby matches. Buraimo and Simmons (Citation2015) found that broadcasters preferred to select derby matches, while TV ratings were not affected by the derby status. However, Scelles (Citation2017) found that derby matches were associated with higher TV ratings.
The study's findings about CSL derby matches should be considered in the CSL context. These derbies involve teams from the same city, but unlike traditional European football derbies, such as Liverpool vs. Everton or Juventus vs. Torino, CSL derby teams often have disparities in fan base and performance. For example, Beijing has two teams: Beijing Guoan FC, a well-established team with numerous CSL championships and a large fan base since its founding in 1992, and Beijing Renhe FC, a team with fewer fans and lower performance levels after relocating to Beijing in 2016. Consequently, matches between these Beijing teams have not attracted significant crowds.
The intra-match CI variable, FLUCTUATION, did not have an impact on viewership. This finding suggests that the mere fluctuations in the score, as indicated by the number of times the score moved from a draw to one team leading or from one team leading to a draw, may not be significant enough to affect viewers’ engagement or interest in watching the game on TV.
Similar to Bond and Addesa (Citation2019) and Scelles (Citation2017), we examined five intra-league CI variables, but none significantly predicted TV ratings. Only matches involving teams contending for the CSL Championship influenced broadcaster choices. Two potential reasons for the weak CI effect emerge: CSL fans’ strong team loyalty, exemplified by Beijing Guoan's consistent high attendance irrespective of their ranking, suggests fan interest is tied more to team loyalty than competitiveness. Additionally, a lack of competitive balance in the CSL, with teams like Guangzhou Evergrande and Shanghai SIPG dominating for years, may have made game outcomes predictable, potentially reducing TV audience interest.
Televised CSL games declined from 2017 to 2019, with lower TV ratings in 2018 and 2019, indicating reduced demand. The Chinese football reform, starting in 2015, may play a role. It emphasized domestic talent development, mandating teams to roster three U-23 players, including two U-21s, decreasing high-profile international player presence, possibly impacting interest in CSL matches.
Implications, limitations, and future directions
This study offers valuable insights for sport marketing and management. First, it provides guidance for other Chinese broadcasters. CCTV5 holds a prominent position as a national TV station with a rich history of broadcasting influential sport events, shaping viewing habits among the majority of Chinese viewers. As such, the behaviors of both broadcasters and audiences studied here with CCTV5 data can serve as a benchmark for other sport channels in China.
Second, the insights from CCTV5's analysis can be applied to contexts beyond China, including countries with channels similar to CCTV5, both in Asia and in regions with analogous fan behaviors, such as some non-Asian countries and Europe. Notably, studies comparing England and Italy, like Buraimo and Simmons (Citation2015), Scelles (Citation2017), and Bond and Addesa (Citation2019), indicate variations in determinants of TV audiences. This suggests that not all European countries exhibit uniform audience behavior patterns, with some potentially resembling China more closely than those previously studied (Scelles & François, Citation2021).
Third, this study is one of the few investigations into media demand for professional football in the Asian market. Sport fan behaviors vary across cultures, and each sport market displays unique consumption patterns. Given the expanding Asian football market and the CSL's rapid growth as a prominent Asian league, our findings hold particular relevance for scholars and practitioners interested in the Chinese football market.
This study has some limitations, pointing to potential future research directions. First, the data used predates the COVID-19 pandemic, which significantly impacted sport fan behavior. Replicating the study post-pandemic could reveal changes in CSL consumption patterns. Second, starting in 2020, CSL matches were livestreamed for free on online platforms, potentially influencing TV ratings. Future research should consider this when studying CSL media demand. Lastly, the study focused on national TV ratings, but regional variations in media demand may exist. Future research could investigate CSL match demand using regional TV ratings.
Disclosure statement
No potential conflict of interest was reported by the author(s).
References
- Alavy, K., Gaskell, A., Leach, S., & Szymanski, S. (2010). On the edge of your seat: Demand for football on television and the uncertainty of outcome hypothesis. International Journal of Sport Finance, 5(2), 75.
- Baimbridge, M., Cameron, S., & Dawson, P. (1996). Satellite television and the demand for football: A whole new ball game? Scottish Journal of Political Economy, 43(3), 317–333. https://doi.org/10.1111/j.1467-9485.1996.tb00848.x
- Barajas, A., Shakina, E., & Gasparetto, T. (2019). At the stadium or at home: the effect of broadcasting matches. Sport, Business and Management: An International Journal, 9(5), 495–505. https://doi.org/10.1108/SBM-12-2018-0112
- Bolotny, F., & Bourg, J. (2006). The demand for media coverage. In W. Andreff, & S. Szymanski (Eds.), Handbook on the economics of sport (pp. 112–133). Edward Elgar Publishing.
- Bond, A., & Addesa, F. (2019). TV demand for the Italian Serie A: Star power or competitive intensity? Economics Bulletin, 39(3), 2110–2116.
- Borland, J., & MacDonald, R. (2003). Demand for sport. Oxford Review of Economic Policy, 19(4), 478–502. https://doi.org/10.1093/oxrep/19.4.478
- Brown, K. M., & Salaga, S. (2018). NCAA football television viewership: Product quality and consumer preference relative to market expectations. Sport Management Review, 21(4), 377–390. https://doi.org/10.1016/j.smr.2017.08.008
- Buraimo, B., & Simmons, R. (2015). Uncertainty of outcome or star quality? Television audience demand for English Premier league football. International Journal of the Economics of Business, 22(3), 449–469. https://doi.org/10.1080/13571516.2015.1010282
- Caruso, R., Addesa, F., & Di Domizio, M. (2019). The determinants of the TV demand for soccer: Empirical evidence on Italian Serie A for the period 2008–2015. Journal of Sports Economics, 20(1), 25–49. https://doi.org/10.1177/1527002517717298
- Certo, S. T., Busenbark, J. R., Woo, H. S., & Semadeni, M. (2016). Sample selection bias and Heckman models in strategic management research. Strategic Management Journal, 37(13), 2639–2657. https://doi.org/10.1002/smj.2475
- Cockayne, D., Chadwick, S., & Sullivan, J. (2022). Chinese football – from a state-led past to a digital future. Journal of Global Sport Management, 7(3), 345–354. https://doi.org/10.1080/24704067.2021.1883212
- Deloitte. (2020, January). Deloitte football money league 2020. https://www2.deloitte.com/be/en/pages/technology-media-and-telecommunications/articles/deloitte-football-money-league-2020.html.
- Ding, M. (2019). China TV rating yearbook. Press of Chinese Communication University.
- Evens, T., Iosifidis, P., & Smith, P. (2013). The political economy of television sports rights. Palgrave Macmillan.
- Feuillet, A., & Scelles, N. (2022). Valuation and negotiation of sport broadcast rights. In A. Feuillet, & N. Scelles (Eds.), Sport broadcasting for managers (pp. 70–83). Routledge.
- Feuillet, A., Scelles, N., & Durand, C. (2019). A winner’s curse in the bidding process for broadcasting rights in football? The cases of the French and UK markets. Sport in Society, 22(7), 1198–1224. https://doi.org/10.1080/17430437.2018.1505869
- Forrest, D., Simmons, R., & Buraimo, B. (2006). Broadcaster and audience demand for Premier League football. In C. Jeanrenaud, & S. Késenne (Eds.), The economics of sport and the media (pp. 93–105). Edward Elgar.
- Forrest, D., Simmons, R., & Szymanski, S. (2005). Outcome uncertainty and the couch potato audience. Scottish Journal of Political Economy, 52(4), 641. https://doi.org/10.1111/j.1467-9485.2005.00360.x
- García, J., & Rodríguez, P. (2006). The determinants of TV audience for Spanish football: A first approach. In P. Rodríguez, S. Kesenne, & J. García (Eds.), Sports economics after fifty years: Essays in honour of Simon Rottenberg (pp. 147–167). Universidad de Oviedo.
- Gasparetto, T., & Barajas, Á. (2018). Fan preferences: One country, two markets and different behaviours. European Sport Management Quarterly, 18(3), 330–347. https://doi.org/10.1080/16184742.2017.1394346
- Gratton, C., & Solberg, H. A. (2007). The economics of sports broadcasting. Routledge.
- Heckman, J. J. (1976). The common structure of statistical models of truncation, sample selection and limited dependent variables and a simple estimator for such models. Annals of Economic and Social Measurement, 5(4), 475–492.
- Heckman, J. J. (1979). Sample selection bias as a specification error. Econometrica, 47(1), 153–161. https://doi.org/10.2307/1912352
- Huber, M., & Mellace, G. (2014). Testing exclusion restrictions and additive separability in sample selection models. Empirical Economics, 47(1), 75–92. https://doi.org/10.1007/s00181-013-0742-1
- Jang, H., & Lee, Y. H. (2022). Testing the uncertainty of outcome hypothesis in football leagues: An application of Italian Football League. Journal of Global Sport Management, 7(4), 529–545. https://doi.org/10.1080/24704067.2021.1951613
- Kennedy, P. (2003). A guide to econometrics (5th ed.).
- Kim, K., Sung, H., Noh, Y., & Lee, K. (2022). Broadcaster choice and audience demand for live sport games: Panel analyses of the Korea Baseball Organization. Journal of Sport Management, 36(5), 488–499. https://doi.org/10.1123/jsm.2020-0311
- Li, S., Ryu, Y., & Kim, H. (2022). A Multilevel analysis of the Chinese Super League: Examining the game level and home team level determinants of stadium attendance. Journal of Global Sport Management, 7(4), 664–681. https://doi.org/10.1080/24704067.2022.2147446
- Mongeon, K., & Winfree, J. (2012). Comparison of television and gate demand in the National Basketball Association. Sport Management Review, 15(1), 72–79. https://doi.org/10.1016/j.smr.2011.09.001
- Morrissey, K., Kinderman, P., Pontin, E., Tai, S., & Schwannauer, M. (2016). Web based health surveys: Using a two Step Heckman model to examine their potential for population health analysis. Social Science & Medicine, 163, 45–53. https://doi.org/10.1016/j.socscimed.2016.06.053
- Paul, R., & Weinbach, A. (2013). Uncertainty of outcome and television ratings for the NHL and MLS. The Journal of Prediction Markets, 7(1), 53–65. https://doi.org/10.5750/jpm.v7i1.587
- Peng, Q., Chen, Z., Li, J., Houlihan, B., & Scelles, N. (2022). The new hope of Chinese football? Youth football reforms and policy conflicts in the implementation process. European Sport Management Quarterly. Advance Online Publication, http://doi.org/10.1080/16184742.2022.2083649
- Peng, Q., Skinner, J., & Houlihan, B. (2019). An analysis of the Chinese Football Reform of 2015: Why then and not earlier? International Journal of Sport Policy and Politics, 11(1), 1–18. https://doi.org/10.1080/19406940.2018.1536075
- Peng, Q., Skinner, J., Houlihan, B., Kihl, L. A., & Zheng, J. (2021). Towards understanding change-supportive organisational behaviours in China: An investigation of the 2015 Chinese National Football Reform. Journal of Global Sport Management. Advance Online Publication, http://doi.org/10.1080/24704067.2021.1910059
- Pérez, L., Puente, V., & Rodríguez, P. (2015). Are broadcast sporting events of “general interest”? A regional panel data analysis of TV ratings for Spain's La liga. Journal of Media Economics, 28(1), 7–19. https://doi.org/10.1080/08997764.2014.997241
- Pérez, L., Puente, V., & Rodríguez, P. (2017). Factors determining TV soccer viewing: Does uncertainty of outcome really matter? International Journal of Sport Finance, 12(2), 124–139.
- Ryu, Y., Kim, K., Paik, J. W., & Cheong, Y. (2019). Determinants of audience demand for the televised professional baseball matches in Korea. International Journal of Sports Marketing and Sponsorship, 20(1), 184–202. https://doi.org/10.1108/IJSMS-12-2017-0127
- Scelles, N. (2017). Star quality and competitive balance? Television audience demand for English Premier League football reconsidered. Applied Economics Letters, 24(19), 1399–1402. https://doi.org/10.1080/13504851.2017.1282125
- Scelles, N., Dermit-Richard, N., & Haynes, R. (2020). What drives sports TV rights? A comparative analysis of their evolution in English and French men’s football first divisions, 1980–2020. Soccer & Society, 21(5), 491–509. https://doi.org/10.1080/14660970.2019.1681406
- Scelles, N., Durand, C., Bah, T. S., & Rioult, F. (2011). Intra-match competitive intensity in French football Ligue 1 and rugby Top 14. International Journal of Sport Management and Marketing, 9(3-4), 154–169. https://doi.org/10.1504/IJSMM.2011.041569
- Scelles, N., & François, A. (2021). Does a country’s income inequality affect its citizens’ quest for equality in leisure? Evidence from European men’s football. Economics and Business Letters, 10(2), 133–139. https://doi.org/10.17811/ebl.10.2.2021.133-139
- Schreyer, D., Schmidt, S. L., & Torgler, B. (2017). Game outcome uncertainty and the demand for international football games: Evidence from the German TV market. Journal of Media Economics, 30(1), 31–45.
- Schreyer, D., Schmidt, S. L., & Torgler, B. (2018). Game outcome uncertainty in the English premier league. Journal of Sports Economics, 19(5), 625–644. https://doi.org/10.1177/1527002516673406
- Sung, H., Mills, B. M., & Mondello, M. (2019). Local broadcast viewership in major league soccer. Journal of Sport Management, 33(2), 106–118. https://doi.org/10.1123/jsm.2018-0022
- Tainsky, S. (2010). Television broadcast demand for National Football League contests. Journal of Sports Economics, 11(6), 629–640. https://doi.org/10.1177/1527002509355636
- Tainsky, S., & Jasielec, M. (2014). Sports fans, identity, and socialization: Exploring the fandemonium. Journal of Sport Management, 28(1), 109–111. https://doi.org/10.1123/jsm.2012-0341
- Villar, J. G., & Guerrero, P. R. (2009). Sports attendance: A survey of the literature 1973–2007. Rivista di Diritto e di Economia Dello Sport, 5(2), 112–151.
- Wang, C., Goossens, D., & Vandebroek, M. (2018). The impact of the soccer schedule on TV viewership and stadium attendance. Journal of Sports Economics, 19(1), 82–112. https://doi.org/10.1177/1527002515612875
- Watanabe, N. M., Yan, G., Soebbing, B. P., & Fu, W. (2019). Air pollution and attendance in the Chinese Super League: Environmental economics and the demand for sport. Journal of Sport Management, 33(4), 289–302. https://doi.org/10.1123/jsm.2018-0214
- Watanabe, N., & Soebbing, B. (2017). Chinese Super League: Attendance, pricing, and team performance. Sport, Business and Management: An International Journal, 7(2), 157–174. https://doi.org/10.1108/SBM-10-2016-0055
- Wills, G., Tacon, R., & Addesa, F. (2022). Uncertainty of outcome, team quality or star players? What drives TV audience demand for UEFA Champions League football? European sport Management quarterly, 22(6), 876-894. doi:10.1080/16184742.2020.1836010
- Xinhua News. (2018, January 30). From “8 billion in 5 years” to “11 billion in 10 years”, what is the impact of the “shrinking” of the Chinese Super League's royalties? http://sports.xinhuanet.com/c/2018-01/30/c_1122337439.htm.