ABSTRACT
Online Acceptance and Commitment Therapy (ACT) interventions use websites and smartphone apps to deliver ACT exercises and skills. The present meta-analysis provides a comprehensive review of online ACT self-help interventions, characterizing the programs that have been studied (e.g. platform, length, content) and analyzing their efficacy. A transdiagnostic approach was taken, including studies that addressed a range of targeted problems and populations. Multi-level meta-analyses were used to nest multiple measures of a single construct within their respective studies. A total of 53 randomized controlled trials were included (n = 10,730). Online ACT produced significantly greater outcomes than waitlist controls at post-treatment for anxiety, depression, quality of life, psychological flexibility, and all assessed outcomes (i.e. omnibus effect), which were generally maintained at follow-up. However, only psychological flexibility and all assessed outcomes at post-treatment were found to be significantly greater for online ACT when compared to active controls, with no significant follow-up effects. Overall, these results further clarify that ACT can be effectively delivered in an online format to target a wide range of mental health concerns, although it is less clear if and when online ACT is more efficacious than other online interventions.
An estimated 21% of adults (52.9 million) in the United States experienced a diagnosable mental illness in 2020 (SAMHSA, Citation2021). Due to the COVID-19 pandemic amongst other factors, mental health symptoms have been rising over the past few years (Czeisler et al., Citation2020; National Center for Health Statistics, Citation2022). Anxiety and depressive disorder symptom prevalence increased by three (25% versus 8.1%) and four times (24.3% versus 6.5%) respectively within the second quarter of 2019 (Czeisler et al., Citation2020). Despite increase in need for mental health services, there are gaps in access to mental health care. Of adults experiencing a diagnosable mental illness in the United States, 30.5% reported an unmet need for mental health services (SAMHSA, Citation2021).
Online self-help treatment programs, such as those delivered over websites and smartphone apps, are a step towards addressing these gaps in mental health services (Casey & Clough, Citation2015; Casey et al., Citation2014). Such interventions can reduce structural barriers prohibiting access, such as transportation difficulties, provider availability, or cost of treatment. Attitudinal barriers such as treatment seeking stigma, or low perceived need, may be addressed by online programs as well (Choi et al., Citation2015; Wallin et al., Citation2018). Mobile health applications in particular are becoming increasingly popular around the world, with half a billion people downloading at least one of the over 100,000 mobile health apps available (Dorsey et al., Citation2017). Empirically-based online self-help interventions demonstrate significant effects on mental health outcomes as compared to waitlist controls, with several trials suggesting near equivalent effects as compared to face-to-face therapy (Ebert et al., Citation2018). While synchronous treatment from a therapist is generally perceived as more acceptable than online self-help interventions (Cuijpers et al., Citation2019), these online interventions help reach a sub-population of individuals who are unwilling to see a therapist (e.g. Levin et al., Citation2018).
While there has been limited research comparing different self-help formats (e.g. online versus printed books), one study found that online self-help programs are equally effective as compared to print self-help such as books (French et al., Citation2017). The previously discussed benefits of online programs apply to print self-help as well. However, there remains a need to investigate online self-help programs specifically, isolated from print self-help. Differences in engagement, uptake, and who is using which may exist between the two. One study found similar rates of uptake, with 17% of college students having ever used a self-help book, 15% having ever used a self-help website, and 8% having ever used a self-help app (Levin et al., Citation2017). However, another study had found that 53% of college students have ever downloaded a self-help app (Melcher et al., Citation2020). The content and function of online versus print self-help can differ as well, with many self-help apps being oriented towards brief daily skills practice as opposed to longer chapters featured in books. Reminder prompts and interactive elements are easier to integrate into online self-help, resulting in potential differences in engagement patterns. Given recent booms in digital healthcare (Carl et al., Citation2022), we can expect online interventions to continue increasing in popularity, with research on online self-help already eclipsing self-help book studies regarding rates of publications.
Among online self-help interventions, there is variation in the therapeutic orientation underlying the program content. Most online interventions are grounded in Cognitive Behavioral Therapy (CBT), with the majority of these using traditional CBT techniques (e.g. cognitive restructuring, progressive muscle relaxation, etc.; Ebert et al., Citation2018; Fleming et al., Citation2018). However, it is important to consider the implementation of other therapeutic approaches in the development of online interventions as well. This is highlighted by the finding supported by one meta-analysis that clients are more likely to experience greater treatment satisfaction, completion rates, and clinical outcomes when provided with a choice in treatment (Lindhiem et al., Citation2014).
Acceptance and commitment therapy (ACT; Hayes et al., Citation2012) is one therapeutic approach within the CBT tradition that has been used as the underlying orientation for several online self-help interventions. ACT addresses a wide range of mental health and related problems through a set of key therapeutic processes including defusing from thoughts and accepting experienced emotions (i.e. being open), being in the present moment and engaging in self-as-context (i.e. being centered), and contacting values and committing to valued actions (i.e. being engaged). These six processes, grouped into pairs of two that make up the open, centered, and engaged pillars of ACT, comprise the construct of psychological flexibility (i.e. the ability to engage in valued activities while being mindfully aware and accepting of whatever internal experiences are present). The primary focus in ACT is to improve psychological flexibility to increase engagement in valued living (i.e. increased quality of life) in the context of whatever challenging thoughts, feelings or other internal experiences may be present. Consistent with this transdiagnostic approach, ACT has been found effective when delivered by a therapist for a wide range of mental health and related problems including anxiety disorders, obsessive compulsive related disorders, depression, substance use, eating disorders, serious mental illness, chronic pain, and coping with chronic health conditions (ACBS, Citation2022; Gloster et al., Citation2020).
The structure of ACT lends itself particularly well to online interventions, for similar reasons that CBT has been widely implemented through online interventions. Treatment manuals for ACT are broken down by session topics that translate conveniently to individual modules for online programs (e.g. acceptance, values, commitment, etc.). Online interventions may also offer unique features to facilitate delivery of ACT, such as ecological momentary assessment for tailoring skill coaching (Levin et al., Citation2019) or the ability to track skills practice (Heffner et al., Citation2015). Previous studies, including meta-analyses and systematic reviews, have demonstrated the efficacy of ACT-based online interventions for depression and anxiety (Brown et al., Citation2016; Herbert et al., Citation2022; Kelson et al., Citation2019; O’Connor et al., Citation2018; Sierra et al., Citation2018; Thompson et al., Citation2021).
This study aims to update the literature on online ACT interventions through a brief review and meta-analysis. While previous studies have addressed this as well, it is important to consider that the most recent meta-analysis of online ACT interventions as a transdiagnostic intervention included studies that were published up until June 2019 (Thompson et al., Citation2021). Publications in the area of online mental health interventions have been rising, particularly on account of the COVID-19 pandemic, calling for another review to incorporate more recent findings. Additionally, heterogeneity among online mental programs, such as differences in supports provided, usability of the program, etc., are important to account for when considering the evidence for online ACT programs on a broader level. Better understanding these variables among the online ACT literature provides context for future research in this area, and sheds light on what has been tried so far in the development of evidence-based online ACT programs. Thus, our review will clarify the state of the literature on online ACT self-help apps and websites, including characteristics of studied interventions (e.g. format, targeted problem area) and variables related to engagement (e.g. usability, adherence). We will also conduct meta-analyses to assess the efficacy of ACT regarding anxiety, depression, quality of life, psychological flexibility, as well as a comprehensive omnibus effect on all outcomes measured by the included studies.
Method
Search process and procedure
A thorough search in the PsycINFO and Google Scholar was conducted using the following search strategy in July 2022: (“computer-based” OR “internet” OR “online” OR “web-based” OR “app*”) AND (“acceptance and commitment therapy”). Studies included in previous meta-analyses and systematic reviews regarding online ACT interventions were also reviewed for inclusion (Han & Kim, Citation2022; Kelson et al., Citation2019; Linardon, Citation2020; O’Connor et al., Citation2018; Sierra et al., Citation2018; Thompson et al., Citation2021), as well as studies listed on the Association of Contextual Behavioral Science’s website’s list of ACT based technology RCTs (ACTing with Technology SIG, Citation2021). Forward and backward searches through found articles were also utilized to increase the thoroughness of the literature review.
To be included in our review, articles had to have (a) tested an intervention delivered through a phone or website (b) that could be completed individually and included interactivity other than reading (e.g. feedback on module completion status), (c) was based upon ACT for the purpose of treating or enhancing mental health, (d) used a RCT design with a non-ACT comparison or control group, (e) published in a peer-reviewed journal, (e) reported pre-treatment and post-treatment outcomes, or outcomes shared by author upon contact, and (f) be in English. Studies that investigated digital self-help book interventions, or other intervention formats that offered limited interactivity (e.g. DVDs, PowerPoint slide decks), were excluded. To be classified as being based on ACT, the intervention must have included at least two of the three columns of ACT (open, centered, engaged). Studies were coded as including “open” if content addressed either acceptance or defusion, “centered” if content addressed either present-moment-awareness or self-as-context, and “engaged” if content addressed either values or committed action. This allowed for inclusion of studies that did not explicitly label the intervention as ACT but functionally served a similar purpose through similar means, as well as exclude studies that labeled the intervention as ACT but did not cover enough elements of ACT to serve as a functional representation in our meta-analysis.
Our search was conducted by two researchers (KSK and GSM), who independently searched for and retrieved articles using discussed strategy and criteria. Regarding our search in PsychINFO, it should be noted that the advanced search settings “Apply related words” and “Peer Reviewed” were selected, and that the search string was entered in the broadest field category (TX All Text). Search results were exported using PsychINFO’s built-in tool and the Publish or Perish software to develop a comprehensive list of potentially eligible articles. Duplicates were identified and removed based on DOI and article title. Remaining articles were screened based on title and abstract to identify relevant articles to be assessed for inclusion with greater depth. These articles were hence retrieved, and articles meeting full inclusion criteria were selected. The lists of selected articles produced through this process by KSK and GSM were compared for discrepancies, which were handled through discussion. It should be noted that the present study was not formally registered.
Data extraction
Pre-established data from each of the finalized articles was extracted by the three researchers KSK, GSM, and MNM. Extracted data was cross-checked amongst each researcher’s data sheets to identify errors or subjective differences in coding. Interrater reliability was appropriate, with coders agreeing on 84% of coded data prior to resolving disagreements through discussion. The following data was extracted from each article: (1) article information: (a) citation reference, (b) intervention name; (2) intervention goals: (a) targeted problem/disorder, (b) primary outcome measure(s), (c) if there was an elevated symptoms criterion; (3) intervention structure: (a) delivery platform (e.g. website or app), (b) if a modular format was used (e.g. program was composed of multiple sequential modules), (c) number of modules, (d) number of weeks the intervention was available to participant; (4) ACT components: (a) inclusion of “open” content, (b) inclusion of “centered” content, (c) inclusion of “engaged” content, (d) inclusion of other elements (e.g. behavioral activation, health behavior tracking); (5) population location: (a) country, (b) continent; (6) additional support elements (e.g. coaching, feedback from therapist); (7) RCT design elements: (a) comparison condition(s) (e.g. waitlist control, face-to-face treatment), (b) post-treatment timepoint, (c) follow-up timepoint; (8) usage and satisfaction data: (a) percent of participants who completed 100% of modules, (b) statistics (mean and SD) of number of modules completed, (c) satisfaction scores, (d) SUS usability rating; and (9) effect size data for each reported outcome (including sample size, mean, and SD for pre-treatment, post-treatment, and follow-up if reported). Intent-to-treat data was selected over completer data when possible. If at least one piece of sought after data was missing from the original article, the corresponding author was contacted and requested to provide the data. A total of 32 authors were contacted (across 41 studies), with data being successfully retrieved for 15 studies. See Appendix C for our coding sheet, which delineates our decision-making process for each coded variable.
Analyses
Statistical analyses were conducted with R (v 4.2, R Core Team, Citation2022) in RStudio (v 2022.02.2 + 485, RStudio Team, Citation2022). Summary statistics were calculated for studies’ reported adherence and satisfaction scores. Satisfaction measures varied across studies, thus scores and corresponding standard deviations were all rescaled to have uniform lower and upper bounds of 0 and 10. Given that participant-level data was not available to analyze, this transformation was applied to study-level means. Reported summary statistics were not weighted by sample size.
Effect size computations accounted for between-group effects by way of comparisons between post-treatment scores of online ACT and control groups, as well as follow-up scores of online ACT and control groups. Collected data used an intent-to-treat approach to analyses, thus the calculated effect sizes reflect data collected from all participants regardless of level of participation with the intervention. In cases where there were more than one online ACT or control group, groups were combined using formulae recommended by the Cochrane Handbook for Systematic Reviews of Interventions (J. P. Higgins et al., Citation2022).
Between-group meta-analyses were conducted for multiple outcome subgroups, including depression, anxiety, quality of life, and psychological flexibility. All instances of each individual outcome were included in the subgroup meta-analysis, whether or not it was the primary outcome of the study (e.g. a chronic pain study’s reported outcomes on anxiety were still included in the anxiety subgroup analyses). Additionally, we conducted a meta-analysis to calculate a total omnibus effect that included all the previously mentioned outcomes, as well as any other outcomes assessed within included studies. Meta-analyses were carried out in a multi-level fashion, to account for multiple measures of a single construct being nested within a single study (3-level meta-analyses), and multiple constructs being nested within a single study in the case of the omnibus effect (4-level meta-analysis) using the rma.mv() function from the metafor package (Viechtbauer, Citation2010). It is important to note that constructs could repeat across different studies. For example, if one study contained three measures of stress, and another study contained two measures of stress, together this would compose five effects, nested within one construct, nested within two studies. Regarding psychological flexibility, any measures of subsuming processes (e.g. mindfulness, values, cognitive fusion, acceptance, etc.) were grouped together within the construct of psychological flexibility alongside more encompassing measures that were intended to assess all processes.
I2 percentages were calculated for anxiety, depression, quality of life, and psychological flexibility analyses using the var.comp() function from the dmetar package (Harrer et al., Citation2019), representing the proportion of variance in observed effects that represent variance among true effects, as opposed to sampling error. τ was used to report heterogeneity for omnibus analyses given that the var.comp() function is not compatible with 4-level models, performing as a measure of standard deviation of true effects. Analyses were conducted separately for control condition comparisons (e.g. waitlist control), and active condition comparisons (e.g. placebo treatment, treatment-as-usual). Comparison groups that consisted of an ACT-based intervention (e.g. face-to-face ACT) were not included in analyses. As included studies focused on a variety of populations based upon the targeted disorder, a random-effects model was used. Hedge’s g was used to compute effect sizes using the group samples sizes, means, and standard deviations extracted from each study.
To assess for publication bias, contour-enhanced funnel plots were visually examined and Egger’s regression tests were conducted on each model from post-treatment assessment with both waitlist and active control comparisons. To conduct the latter, meta-analytic models from post-test assessments were re-run with sample variance added as a moderator. No other assessments of publication bias or sensitivity analyses (e.g. trim-and-fill) were conducted given the limitations of the rma.mv() function used to conduct the multi-level meta-analysis.
Results
Study selection
A total of 2,565 articles were retrieved after conducting a thorough search, with 1,355 stemming from PsychNet, 1,180 from Google Scholar, and 50 from other sources (i.e. ACTing with Technology SIG website, previous meta-analyses and systematic reviews, forward/backward searches within articles). Of those articles, 460 were identified as duplicates, 14 were article corrections, and 2 were miscellaneous materials; the remaining 1,930 articles were then screened. Three hundred and thirty-five articles were investigated further based on their title and abstract. Finally, 53 articles were determined eligible for inclusion (see and Appendix A).
Sample characteristics
Collectively, there were 10,730 participants among the 53 studies with sample sizes for each study ranging from 23 to 2,415 (median = 92). See Appendix B for a full listing of sample characteristics by study. Among the articles, 37 studies required participants to meet a specific elevated symptomology (e.g. meeting criteria for diagnosis on DSM) in order to be eligible for their respective study, while 16 studies did not have requirements for elevated symptomology. Targeted problems among studies included well-being/stress (n = 17), mental health affected by a chronic health condition (n = 12), depression (n = 6), smoking cessation (n = 6), anxiety (n = 4), health behaviors (i.e. diet and exercise; n = 3), eating disorders (n = 2), insomnia (n = 1), parenting skills (n = 1), and inflammation and stress biomarkers (n = 1). The majority of studies recruited participants from Europe (n = 31), followed by North America (n = 16), Australia (n = 3), transcontinental (i.e. Cyprus; n = 2), and Asia (n = 1). United States (n = 14) was recognized as the country where most of the studies recruited their participants from, second most was Sweden (n = 12), and then Finland (n = 7). The remaining articles recruited participants from the Netherlands (n = 4), United Kingdom (n = 2), Australia (n = 3), Germany (n = 2), Cyprus (n = 2), Canada (n = 1), Republic of Ireland (n = 1), Norway (n = 1), Portugal (n = 1), Denmark (n = 1), China (n = 1), and both US and Canada (n = 1).
Study design characteristics
Of the included studies, 37 had implemented a waitlist control condition, 14 had implemented an active control condition, and 2 had implemented both. Regarding the 16 active control conditions, 9 of these were treatment as usual, 5 were a placebo treatment (i.e. online discussion forum or expressing writing program), and 2 were online intervention using a different form of therapy than ACT (i.e. traditional CBT and compassion-focused therapy). While the following conditions were not evaluated in the meta-analysis portion of this article, two studies additionally included a face-to-face group ACT condition, five studies included a condition that consisted of the online intervention with additional features (i.e. orientation/termination sessions, personalized feedback from a coach), and four studies included a condition that was a simplified version of the online intervention (i.e. online program without tailoring, online program with content from only one of the pillars of ACT).
Studies had an average of 2.17 (SD = 1.60) months between the baseline and post-treatment assessment. Of the 34 studies that collected follow-up data, studies had an average of 4.93 (SD = 3.46) months between post-treatment assessment and follow-up assessment. With studies that included multiple follow-up assessment timepoints, only data was entered for the timepoint that best matched average follow-up time of other included studies. This approach was chosen given that combining data from multiple timepoints poses a unit-of-analysis problem, and the alternative of choosing the longer timepoint introduces unnecessary heterogeneity (J. P. Higgins et al., Citation2022).
Interventions
Among the included studies, 38 used a website to deliver their respective intervention, 10 used an app, and 5 used a combination of at least two delivery platforms (i.e. website and workbook). The five interventions that used a combination of platforms all implemented a website, with three of these additionally integrating a physical workbook and audio CD, one of these additionally integrating a physical workbook and no audio CD, and one of these being a website that could be delivered either through a computer or mobile device (e.g. could essentially function as an app). Interventions on average took place across a period of 9.27 weeks (SD = 9.05; median = 8), with this time span ranging from two weeks to a full year. However, it is notable that the two interventions that took place over a full year were outliers (Bricker et al., Citation2018, Citation2020), with the second longest intervention taking place over 14 weeks. The majority of studied interventions (n = 42) were divided into discrete modules that were to be completed over multiple sessions, while the remaining interventions functioned more like a typical app and were to be accessed in repeated, brief periods to be guided through a quick ACT skill (n = 10). One intervention was an exception to both of these categories, and was designed to be completed within two hours (either in a single session, or several shorter session) but was not divided into discreet modules (Sagon et al., Citation2018). Of the 42 studies that investigated a modular program, interventions were composed of 6.38 (SD = 2.22) modules on average (median = 6; mode = 6), ranging from 2 to 12 modules. A total of 49 interventions included all three pillars of ACT (i.e. open, centered, engaged), with the remaining 4 interventions containing at least two of the pillars as designated by our inclusion criteria. Eight interventions additionally included psychoeducation elements (e.g. on sleep hygiene, diet/fitness, knowledge about the chronic condition that is being addressed, etc.).
Additional support for interventions came in the form of prompts to use the program (e.g. regularly sent supportive text messages or reminder to use the program; n = 30), written “one-way” (e.g. the participant was not expected to reply back) feedback from a coach/therapist/research staff on activities provided by the intervention (n = 26), synchronous orientation or termination session (delivered face-to-face or phone-call; n = 17), two-way coaching (e.g. regular phone calls, emails, or text messages with a coach to discuss intervention progress; n = 12), or another forms of support (e.g. online forum for discussion with other participants, consultation with a psychologist; n = 9). Of note, not all interventions included additional support and some interventions had multiple forms of support. Only 15 interventions had either only automated prompts or no additional support, indicating that the majority of interventions involved some non-automated, human contact component.
Adherence & satisfaction data
Among the studies that provided adherence data, the average number of participants that completed all modules was 57.63% (n = 23; SD = 18.45%; median = 57%) with a broad range of 27.5% to 94.7%. Participants typically completed a total of 74% (n = 13; SD = 11%; median = 72.86%) of all modules, with a range of 53.33% to 94.5%.
On average across studies, satisfaction was rated at 7.27 on a scale of 0–10 (n = 14; between-study SD = 0.59; range = 6.36–8.02), with an average SD of 1.56 within-study. Participants reported an average System Usability Score of 79.19 on a scale of 0 to 100 (n = 8; between-study SD = 5.46; range = 71.13–85.63), with an average SD of 13.74 within-study. Thus, all studies approximately met or approached cut-off criteria for “good” (≥72.75) to “excellent” (≥85.58) usability (Bangor et al., Citation2008).
Subgroup meta-analyses
See for a full table of results, including effect size (g), 95% confidence intervals, effect size p-values, Cochran’s Q p-values (Cochran, Citation1954), Higgins and Thompson’s I2 for both between-study and within-study (J. P. T. Higgins & Thompson, Citation2002), and 95% prediction intervals (IntHout et al., Citation2016).
Table 1. Between-group meta-analyses results (subgroups).
Anxiety
When comparing online ACT to waitlist control groups for anxiety measures during the post-treatment assessment (k = 22 effects across 17 studies), a small, significant effect size of g = 0.30 (95% CI [0.17, 0.43]) was observed. Moderate between-studies heterogeneity (I2 = 42.9%) was present, with no within-studies heterogeneity (I2 = 0%; see ). Follow-up assessment for anxiety measures when comparing online ACT to waitlist control conditions (k = 6 effects across five studies) had a small, significant pooled effect size of g = 0.32 (95% CI [0.12, 0.52]), with no between or within-studies heterogeneity (I2 = 0%).
Figure 2. (a) Forest plot of meta-analysis results at post-treatment (Waitlist controls; Anxiety). (b) Forest plot of meta-analysis results at post-treatment (Waitlist controls; Depression). (c) Forest plot of meta-analysis results at post-treatment (Waitlist controls; Quality of life). (d) Forest plot of meta-analysis results at post-treatment (Waitlist controls; Psychological flexibility).
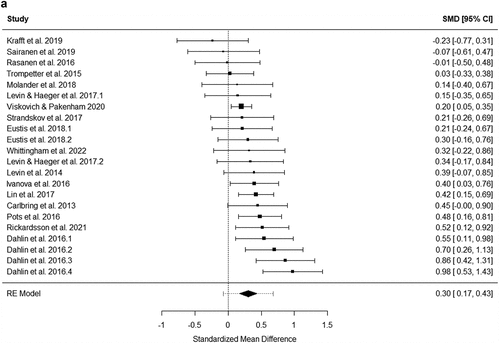
In contrast, when comparing online ACT to active control groups at post-treatment assessment (k = 10 effects across eight studies), no significant effect was found (g = 0.21; 95% CI [−0.07, 0.50]. Between-studies heterogeneity was substantial (I2 = 54.9%), and within-studies heterogeneity was negligible (I2 = 9.6%; see ). Similarly, no significant effect was found when comparing experiment to active control groups at follow-up assessment (k = 8 effects across six studies), with substantial between-studies heterogeneity (I2 = 56.6%) and negligible within-studies heterogeneity (I2 = 16.3%).
Figure 3. (a) Forest plot of meta-analysis results at post-treatment (Active controls; Anxiety). (b) Forest plot of meta-analysis results at post-treatment (Active controls; Depression). (c) Forest plot of meta-analysis results at post-treatment (Active controls; Quality of Life). (d) Forest plot of meta-analysis results at post-treatment (Active controls; Psychological flexibility).
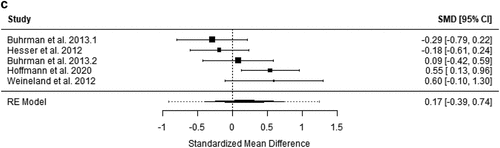
Depression
The pooled effect size for the studies comparing online ACT to waitlist control groups for depression measures during the post-treatment assessment (k = 27 effects across 24 studies) was a small, significant effect of g = 0.44 (95% CI [0.32, 0.57]), with substantial between-studies heterogeneity (I2 = 53.4%) and no within-studies heterogeneity (I2 = 0%; see ). The studies that captured follow-up data on depression (k = 7 effects across seven studies) had a small, significant pooled effect size of g = 0.49 (95% CI [0.30, 0.68]), with negligible between-studies heterogeneity (I2 = 3.8%) and within-studies heterogeneity (I2 = 3.8%) when comparing online ACT to waitlist control conditions.
Results were less promising when comparing online ACT and active control conditions at post-treatment (k = 10 effects across eight studies), with no significant effect found (g = 0.15; 95% CI [−0.03, 0.32]). Between-studies heterogeneity was negligible (I2 = 17%), and no within-studies heterogeneity was present (I2 = 0%; see ). No significant effect was found when comparing online ACT and active control conditions at follow-up (k = 7 effects across seven studies; g = 0.05; 95% CI [−0.32, 0.43]), and moderate heterogeneity was present (I2 = 35.2%).
Quality of life
When comparing online ACT and waitlist control conditions, quality of life measures assessed at post-treatment (k = 24 effects across 15 studies) revealed a significant, small pooled effect size of g = 0.20 (95% CI [0.09, 0.30]), with negligible between-studies heterogeneity (I2 = 12.4%) and no within-study heterogeneity (I2 = 0%; see ). However, no significant effect was found when comparing experiment and waitlist control conditions at follow-up (k = 6 effects across three studies; g = 0.11; 95% CI [−0.14, 0.36]), with no between or within-studies heterogeneity being present (I2 = 0%).
No significant effect was found for quality of life when comparing online ACT and active control conditions at post-treatment (k = 5 effects across four studies; g = 0.17; 95% CI [−0.39, 0.74]), and between-studies heterogeneity was substantial (I2 = 59.8%) while within-studies heterogeneity was negligible (I2 = 3.9%; see ). Similarly, when comparing online ACT and active control conditions at follow-up (k = 2 effects across two studies), no significant effect was found (g = 0.13; 95% CI [−6.68, 6.93]), and heterogeneity was moderate (I2 = 45.1%).
Psychological flexibility
Finally, psychological flexibility at post-treatment assessment, when comparing online ACT to waitlist control conditions (k = 69 effects across 23 studies), had a significant, small pooled effect size of g = 0.29 (95% CI [0.21, 0.38]). Negligible between-studies heterogeneity was exhibited (I2 = 10.3%), however substantial within-studies heterogeneity was present (I2 = 50.1%; see ). Among the studies that investigated psychological flexibility at follow-up when comparing experiment and active control conditions (k = 26 effects across seven studies), a small pooled effect size of g = 0.37 (95% CI [0.24, 0.49]) was found, with no between-studies heterogeneity (I2 = 0%) but substantial within-studies heterogeneity (I2 = 53.9%).
When comparing psychological flexibility measures between online ACT and active control groups at post-treatment (k = 33 effects across 15 studies), a significant, small pooled effect size of g = 0.16 (95% CI [0.02, 0.31]) was found, with substantial between-studies heterogeneity (I2 = 60.6%) and no within-studies heterogeneity (I2 = 0%; see ). This effect was not maintained into follow-up though, as indicated by no significant found when comparing online ACT and active control groups at this time point (k = 25 effects across eight studies; g = 0.05; 95% CI [−0.15, 0.24]).
Omnibus meta-analyses
See for a full table of results, which includes the previously discussed statistics that were reported for omnibus meta-analyses. However, as opposed to reporting I2, heterogeneity is reported using τ at each level of analysis (i.e. between-studies, within-studies, within-constructs).
Table 2. Between-group meta-analyses results (Omnibus Effect).
Waitlist control comparisons
A total of 274 effects, nested within 157 constructs, nested within 36 studies were included in the meta-analysis of online ACT and waitlist control comparisons as measured at post-treatment. Online ACT had a significant, small pooled effect of g = 0.31 (95% CI [0.24, 0.39]) on observed outcomes, and a between-studies standard deviation of τ = 0.04, a within-studies standard deviation of τ < 0.01, and a within-constructs standard deviation of τ = 0.01 (see ). When analyzing online ACT and waitlist control comparisons at follow-up, a total of 89 effects, nested within 41 constructs, nested within 11 studies were included. At this time point, online ACT had a significant, small pooled effect of g = 0.30 (95% CI [0.19, 0.42]), and a between-studies standard deviation of τ = 0.17, a within-studies standard deviation of τ < 0.01, and a within-constructs standard deviation of τ < 0.01.
Active control comparisons
A meta-analysis of 105 effects, nested within 67 constructs, nested within 16 studies regarding online ACT and active control comparisons revealed that online ACT had a significant, small pooled effect of g = 0.18 (95% CI [0.09, 0.28]) on outcomes. The between-studies standard deviation was τ = 0.13, the within-studies standard deviation was τ = 0.18, and the within constructs standard-deviation was τ < 0.01 (see ). At follow-up, online ACT did not have a significant effect on outcomes when comparing online ACT and active control conditions (g = 0.10; 95% CI [−0.11, 0.31]), which consisted of 70 effects, nested within 44 constructs, nested within 8 studies. For this pooled effect, the standard deviation was τ = 0.28 between-studies, τ = 0.07 within-studies, and τ < 0.01 within constructs.
Publication bias
Egger’s test indicated significant asymmetry in funnel plots for post-treatment omnibus effects when comparing online ACT and waitlist control conditions (p = .017) and online ACT and active control conditions at post-treatment (p = .003). Additionally, significant asymmetry was found in anxiety (p = .012), depression (p = .005), quality of life (p < .001), and psychological flexibility (p = .005) when comparing online ACT and waitlist control conditions at post-treatment. No significant asymmetry was found in these subgroups when comparing online ACT and active control conditions at post-treatment (all p > .05). Examination of contour-enhanced funnel plots supported these findings, with studies being biased towards significant positive effect sizes (see ).
Discussion
The current study provides an up-to-date reflection on the current state of the literature on online ACT self-help interventions, as well as their effectiveness regarding depression, anxiety, quality of life, psychological flexibility, and all assessed outcomes more broadly (i.e. omnibus effect size). A total of 53 studies (n = 10,730) met inclusion criteria and were divided into studies that used a waitlist control (n = 39) and studies that used an active control (n = 16) prior to conducting meta-analyses. Additionally, studies were characterized to provide a better understanding of empirically researched online ACT interventions, regarding factors such as delivery platform (e.g. website or app), treatment length, additional supports, included ACT processes, and adherence and satisfaction rates.
Study and intervention characteristics
Websites were found to be the most commonly research delivery platform for interventions (n = 38), while apps were less common (n = 10). The number of researched ACT website interventions increases to 43 when considering programs that used a combination of a website plus another platform (e.g. workbook). This is a key finding that reflects a discrepancy between the ACT literature and real-world practice, given that apps are a more commonly accessed method of self-help (Stawarz et al., Citation2019). While a previous study conducted in 2017 found only four publicly available ACT apps (Torous et al., Citation2017), this has since increased to 11 publicly available ACT apps, as compared to only 9 publicly available ACT website interventions (ACTing with Technology SIG, Citation2021). ACT apps appear to be increasing in popularity over time and may eventually follow the trend of traditional CBT apps of which there are hundreds, vastly outnumbering traditional CBT websites (Stawarz et al., Citation2019; Torous et al., Citation2017). While websites are typically more feasible for researchers to build, apps are generally easier to distribute directly to market through interfaces such as app stores. Given that the majority of researched ACT website interventions are not deployed to the public, while the majority of mental health apps are created by developers and are thus not formally researched (Torous et al., Citation2017), a gap exists in which published research on online interventions does not reflect the online interventions that the public is using. Thus, further research on ACT-based apps is called for to address this discrepancy.
Similarly, the frequent inclusion of additional supports in ACT online self-help research such as personalized feedback from therapists, synchronous coaching, or orientation and termination sessions may also fail to reflect the realities of how ACT-based programs are used. In the present meta-analysis, a total of 38 of the 53 included online ACT interventions involved a form of non-automated human support. However, it is often not feasible to provide these resource-heavy supports to a large user base when services are offered outside the context of research at a broader scale, and many individuals using self-help will not have access to such supports. Additionally, findings are mixed regarding the impact of these human-supports on adherence and treatment efficacy (Musiat et al., Citation2021; Shim et al., Citation2017). More research taking place in a naturalistic context without additional supports is called for to improve the external validity of this field of research, in addition to research regarding when additional supports enhance program usage and how to deliver these supports in a scalable manner.
Treatment adherence was found to be somewhat variable, with the percentage of participants completing the entire intervention ranging from 27.5% to 94.7% (M = 57.63%, SD = 18.45%), and the average number of modules completed ranging from 53.33% to 94.5% (M = 74%, SD = 11%). A prior meta-analysis of online ACT found average adherence rates of 75.77% for number of modules completed, indicating consistency of our findings with prior online ACT literature (Thompson et al., Citation2021). Given the high heterogeneity regarding how adherence, attrition, and engagement are reported in research on online interventions, comparison with other online programs based in other orientations is somewhat difficult (Beintner et al., Citation2019). However, these ACT adherence rates appear to be generally similar to those found with other online CBTs (Borghouts et al., Citation2021; Linardon & Fuller-Tyszkiewicz, Citation2020). In considering the wide range of adherence rates, it is important to note heterogeneity in factors such as availability of human-support and length of the program. It is still unknown what ideal dosage levels (e.g. program length) are necessary for change, and how ideal dosage level differs among individuals.
Efficacy of online ACT
Between-group effect sizes for post-treatment assessment showed online ACT to be an efficacious intervention for anxiety, depression, quality of life, psychological flexibility, and all assessed outcomes (i.e. omnibus effect) when compared to a waitlist control group. Follow-up between-group effect sizes for all outcomes except for quality of life were also found to be significant. In contrast, when comparing to active control groups (e.g. treatment-as-usual, placebo intervention, online traditional CBT), only psychological flexibility and all assessed outcomes were found to be significantly greater for online ACT at post-treatment assessment, with no significant effects at follow-up.
A prior meta-analysis of online ACT interventions similarly found waitlist control comparisons produced a greater number of significant effects, however these were more promising than our found results given that significant effects were still found for depression and anxiety against active controls (O’Connor et al., Citation2018). However, it is important to note that active controls and alternative interventions (e.g. online traditional CBT) were grouped separately in that meta-analysis, with effect sizes for alternative intervention groups being non-significant. It is interesting that quality of life at follow-up was found to be non-significant for both waitlist and active control comparisons, despite a previous meta-analysis finding support for these outcomes (Thompson et al., Citation2021). The significant effect sizes for waitlist control comparisons found within our study (ranging from g = 0.20–0.49) approximately matched those of prior meta-analyses which grouped waitlist and active controls (ranging from g = 0.24–0.38), however significant effect sizes for active control comparisons were much lower (ranging from g = 0.16 to 0.18; Thompson et al., Citation2021).
Regarding psychological flexibility, a prior meta-analysis analyzing process variables of online ACT found generally higher effect sizes for psychological flexibility at post-test (SMD = 0.38, compared to g = 0.29) and follow-up (SMD = 0.41, compared to g = 0.37) when comparing waitlist conditions, and for post-treatment when comparing active controls (SMD = 0.60, compared to g = 0.16; Han & Kim, Citation2022). Similar to our study, no significant effect was found for follow-up when comparing active controls. However, it is worth noting that for the purpose of our meta-analysis all measures of psychological flexibility constructs (e.g. cognitive defusion, mindfulness, etc.) were included within our psychological flexibility analyses, while the effect sizes listed for the compared study are limited to measures that are intended to capture all facets of the psychological flexibility (sub-constructs were analyzed separately).
It is worth comparing the results of our study to prior meta-analyses of face-to-face ACT as well. A prior meta-analysis found that face-to-face ACT had a medium pooled effect-size of SMD = 0.59 on depression at post-assessment (Bai et al., Citation2020). We had found a smaller effect size of g = 0.44 when comparing waitlist control, and a non-significant effect size when comparing active controls. Another meta-analysis had found medium pooled effect sizes of g = 0.63 for waitlist controls, g = 0.59 for placebo, and g = 0.55 for treatment-as-usual control comparisons when looking at primary outcomes in face-to-face ACT trials (Öst, Citation2014). Our approach differed in that we analyzed all assessed outcomes to compute an omnibus effect size as opposed to selecting only primary outcomes, producing small effect sizes of g = 0.31 for waitlist controls and g = 0.18 for active controls. While our effect sizes were not as high across varying outcomes, our results are still comparable given that effect sizes can be expected to be higher when analyzing primary outcomes.
Given the comparability of our results with prior online ACT meta-analyses, we have further evidence supporting the effectiveness of such technologically aided interventions. However, results suggest smaller effect sizes for online ACT as compared to face-to-face interventions, reinforcing that online programs are not necessarily a replacement for synchronous therapy, but still have the potential to perform as efficacious interventions. We can have further confidence as a field in the ability of online ACT self-help to serve as intermediate treatments while waiting for therapist availability, as follow-up care once treatment has terminated, or as stand-alone interventions for those who do not have the need, preference, or means to see a mental health provider synchronously. More research is called for on dissemination, implementation, and moderation effects for online ACT programs in order to carry us into an era where such interventions are well known and often used, by both the general public as well as by clinics and mental health professionals in their work with clients. Specifically, further investigation on differences between delivery platforms, when and how human-support is helpful, as well as adherence-related effects and necessary dosage levels to achieve improvements are called for.
Additionally, it is important to consider the present results within the context of the publication bias that was detected. Our results suggest potential publication bias among both waitlist and active control comparisons when examining an omnibus effect, and among waitlist control comparisons only when examining all individual outcomes (i.e. depression, anxiety, quality of life, psychological flexibility). However, prior meta-analyses that have parsed apart waitlist from active control studies have found contradictory results, with asymmetry in funnel plots being found among waitlist control conditions only for depression, and among active control conditions only for anxiety, with no asymmetry found for quality of life (O’Connor et al., Citation2018). It is somewhat unclear why this is the case, however this discrepancy should not be attributed to an increase in publication bias across time, given that no relationship was visually observed between bias and year of publication. Regardless, the results presented may offer a more optimistic view of online ACT self-help as compared to how these programs perform in reality, either due to publication bias or other factors that cause funnel plot asymmetry such as heterogeneity among studies.
Limitations
Limitations to this study may include heterogeneity among studies regarding variables such as delivery platform, availability of additional human supports, and treatment length, making it difficult to generalize our findings to any one specific program. Additionally, while all reviewed studies were included in our meta-analyses, several specific outcomes were missing and could not be retrieved from authors. Specifically, 21 post-treatment effects across 5 studies, and 27 follow-up effects across 4 studies could not be retrieved and included. There were also 25 included studies missing data related to program adherence, and 11 included studies missing data related to satisfaction. Thus, this meta-analysis might not give a complete, full picture of the literature available and research conducted. The outcomes assessed (i.e. anxiety, depression, and all included outcomes) also did not always directly map onto the primary outcome of the intervention (e.g. a depression outcome may have been included in the meta-analysis even if anxiety was the targeted problem), thus effect sizes may be diluted as compared to assessing only primary outcomes. It is likely that psychological flexibility is the “active ingredient” process that contributes to these effect sizes, given that effects on psychological flexibility were significant as well.
It should also be taken into consideration that by way of including only RCTs, results may reflect online intervention use under more ideal conditions as compared to natural use (Baumel et al., Citation2019). For example, participants may be motivated to adhere to and complete the intervention due to compensation offered, demand characteristics, or additional support offered such as coaching, which may not be available under usual circumstances of program use. This bias limits the external validity of the study, with results inflating adherence compared to what would be seen in typical use. Furthermore, the studies had varying follow-up time points therefore, there is limited information on the trajectory of the long-term effects of the intervention. There is also a significant risk of publication bias from the waitlist control comparison studies included, which reduces the strength of the evidence. Most of the studies included were from the Western world (Europe and North America), therefore the results may not generalize to other populations. Finally, the study excluded studies that were not in English which may have contributed to selection bias. Future research should include studies published in other languages.
Conclusions
Overall, results show an online version of ACT to be an effective self-help intervention for improving mental health generally as well as addressing anxiety and depression more specifically, particularly when observing studies that used a waitlist control. While significant effect sizes were small, and smaller than the medium effect sizes found for face-to-face therapy (Bai et al., Citation2020; Öst, Citation2014), these were comparable to prior effect sizes found by similar online ACT meta-analyses (O’Connor et al., Citation2018; Thompson et al., Citation2021). Effect sizes were less promising when comparing active control groups, as only psychological flexibility and omnibus effects were found to be significant at post-treatment, with marginal effect sizes. This raises questions regarding if and when online ACT has superior effectiveness to other online interventions, and calls for research regarding mechanisms of change and moderation in online programs. In addition, online ACT generally demonstrates comparable adherence levels to other online interventions, with acceptable satisfaction and usability scores. Considering the number of difficulties individuals have connecting with a therapist face-to-face, online ACT could bridge the gap to make therapy more available, accessible, and affordable (Bennett et al., Citation2020).
Supplemental Material
Download MS Word (71.3 KB)Disclosure statement
No potential conflict of interest was reported by the author(s).
Supplementary data
Supplemental data for this article can be accessed online at https://doi.org/10.1080/16506073.2023.2178498
References
- ACBS. (2022, June 9). ACT Randomized Controlled Trials (1986 to present). https://contextualscience.org/act_randomized_controlled_trials_1986_to_present
- ACTing with Technology SIG. (2021, October 4). Research and Clinical Trials. ACTing with Technology SIG. https://actingwithtech.wordpress.com/research-and-clinical-trials/
- Bai, Z., Luo, S., Zhang, L., Wu, S., & Chi, I. (2020). Acceptance and commitment therapy (ACT) to reduce depression: A systematic review and meta-analysis. Journal of Affective Disorders, 260, 728–737. https://doi.org/10.1016/j.jad.2019.09.040
- Bangor, A., Kortum, P. T., & Miller, J. T. (2008). An empirical evaluation of the system usability scale. International Journal of Human-Computer Interaction, 24(6), 574–594. https://doi.org/10.1080/10447310802205776
- Baumel, A., Edan, S., & Kane, J. M. (2019). Is there a trial bias impacting user engagement with unguided e-mental health interventions? A systematic comparison of published reports and real-world usage of the same programs. Translational Behavioral Medicine, ibz147. https://doi.org/10.1093/tbm/ibz147
- Beintner, I., Vollert, B., Zarski, A.-C., Bolinski, F., Musiat, P., Görlich, D., Ebert, D. D., & Jacobi, C. (2019). Adherence reporting in randomized controlled trials examining manualized multisession online interventions: Systematic review of practices and proposal for reporting standards. Journal of Medical Internet Research, 21(8), e14181. https://doi.org/10.2196/14181
- Bennett, C. B., Ruggero, C. J., Sever, A. C., & Yanouri, L. (2020). eHealth to redress psychotherapy access barriers both new and old: A review of reviews and meta-analyses. Journal of Psychotherapy Integration, 30(2), 188–207. https://doi.org/10.1037/int0000217
- Borghouts, J., Eikey, E., Mark, G., De Leon, C., Schueller, S. M., Schneider, M., Stadnick, N., Zheng, K., Mukamel, D., & Sorkin, D. H. (2021). Barriers to and facilitators of user engagement with digital mental health interventions: Systematic review. Journal of Medical Internet Research, 23(3), e24387. https://doi.org/10.2196/24387
- Bricker, J. B., Mull, K. E., McClure, J. B., Watson, N. L., & Heffner, J. L. (2018). Improving quit rates of web-delivered interventions for smoking cessation: Full-scale randomized trial of WebQuit.Org versus smokefree.Gov. Addiction, 113(5), 914–923. https://doi.org/10.1111/add.14127
- Bricker, J. B., Watson, N. L., Mull, K. E., Sullivan, B. M., & Heffner, J. L. (2020). Efficacy of smartphone applications for smoking cessation: A randomized clinical trial. JAMA Internal Medicine, 180(11), 1472. https://doi.org/10.1001/jamainternmed.2020.4055
- Brown, M., Glendenning, A., Hoon, A. E., & John, A. (2016). Effectiveness of web-delivered acceptance and commitment therapy in relation to mental health and well-being: A systematic review and meta-analysis. Journal of Medical Internet Research, 18(8), e221. https://doi.org/10.2196/jmir.6200
- Carl, J. R., Jones, D. J., Lindhiem, O. J., Doss, B. D., Weingardt, K. R., Timmons, A. C., & Comer, J. S. (2022). Regulating digital therapeutics for mental health: Opportunities, challenges, and the essential role of psychologists. British Journal of Clinical Psychology, 61(S1), 130–135. https://doi.org/10.1111/bjc.12286
- Casey, L. M., & Clough, B. A. (2015). Making and keeping the connection: Improving consumer attitudes and engagement in e-mental health interventions. In G. Riva, B. K. Wiederhold, & P. Cipresso (Eds.), The psychology of social networking Vol.1: Personal experience in online communities. De Gruyter Open Poland. https://doi.org/10.1515/9783110473780
- Casey, L. M., Wright, M.-A., & Clough, B. A. (2014). Comparison of perceived barriers and treatment preferences associated with internet-based and face-to-face psychological treatment of depression. International Journal of Cyber Behavior, Psychology and Learning, 4(4), 16–22. https://doi.org/10.4018/ijcbpl.2014100102
- Choi, I., Sharpe, L., Li, S., & Hunt, C. (2015). Acceptability of psychological treatment to Chinese- and Caucasian-Australians: Internet treatment reduces barriers but face-to-face care is preferred. Social Psychiatry and Psychiatric Epidemiology, 50(1), 77–87. https://doi.org/10.1007/s00127-014-0921-1
- Cochran, W. G. (1954). Some methods for strengthening the common χ 2 tests. Biometrics, 10(4), 417. https://doi.org/10.2307/3001616
- Cuijpers, P., Noma, H., Karyotaki, E., Cipriani, A., & Furukawa, T. A. (2019). Effectiveness and acceptability of cognitive behavior therapy delivery formats in adults with depression: A network meta-analysis. JAMA Psychiatry, 76(7), 700. https://doi.org/10.1001/jamapsychiatry.2019.0268
- Czeisler, M. É., Lane, R. I., Petrosky, E., Wiley, J. F., Christensen, A., Njai, R., Weaver, M. D., Robbins, R., Facer-Childs, E. R., Barger, L. K., Czeisler, C. A., Howard, M. E., & Rajaratnam, S. M. W. (2020). Mental health, substance use, and suicidal ideation during the COVID-19 pandemic—United States. MMWR Morbidity and Mortality Weekly Report, 69 (32), 1049–1057. June 24–30, 2020. https://doi.org/10.15585/mmwr.mm6932a1.
- Dorsey, E. R., “Yvonne Chan, Y. -F., McConnell, M. V., Shaw, S. Y., Trister, A. D., & Friend, S. H. (2017). The use of smartphones for health research. Academic Medicine, 92(2), 157–160. https://doi.org/10.1097/ACM.0000000000001205
- Ebert, D. D., Van Daele, T., Nordgreen, T., Karekla, M., Compare, A., Zarbo, C., Brugnera, A., Øverland, S., Trebbi, G., Jensen, K. L., Kaehlke, F., & Baumeister, H. (2018). Internet- and mobile-based psychological interventions: Applications, efficacy, and potential for improving mental health: A report of the efpa e-health taskforce. European Psychologist, 23(2), 167–187. https://doi.org/10.1027/1016-9040/a000318
- Fleming, T., Bavin, L., Lucassen, M., Stasiak, K., Hopkins, S., & Merry, S. (2018). Beyond the trial: Systematic review of real-world uptake and engagement with digital self-help interventions for depression, low mood, or anxiety. Journal of Medical Internet Research, 20(6), e199. https://doi.org/10.2196/jmir.9275
- French, K., Golijani-Moghaddam, N., & Schröder, T. (2017). What is the evidence for the efficacy of self-help acceptance and commitment therapy? A systematic review and meta-analysis. Journal of Contextual Behavioral Science, 6(4), 360–374. https://doi.org/10.1016/j.jcbs.2017.08.002
- Gloster, A. T., Walder, N., Levin, M. E., Twohig, M. P., & Karekla, M. (2020). The empirical status of acceptance and commitment therapy: A review of meta-analyses. Journal of Contextual Behavioral Science, 18, 181–192. https://doi.org/10.1016/j.jcbs.2020.09.009
- Han, A., & Kim, T. H. (2022). The effects of internet-based acceptance and commitment therapy on process measures: Systematic review and meta-analysis. Journal of Medical Internet Research, 24(8), e39182. https://doi.org/10.2196/39182
- Harrer, M., Adam, S. H., Baumeister, H., Cuijpers, P., Karyotaki, E., Auerbach, R. P., Kessler, R. C., Bruffaerts, R., Berking, M., & Ebert, D. D. (2019). Internet interventions for mental health in university students: A systematic review and meta-analysis. International Journal of Methods in Psychiatric Research, 28(2), e1759. https://doi.org/10.1002/mpr.1759
- Hayes, S. C., Strosahl, K. D., & Wilson, K. G. (2012). Acceptance and Commitment Therapy: The Process and Practice of Mindful Change (Second ed.). The Guilford Press.
- Heffner, J. L., Vilardaga, R., Mercer, L. D., Kientz, J. A., & Bricker, J. B. (2015). Feature-level analysis of a novel smartphone application for smoking cessation. The American Journal of Drug and Alcohol Abuse, 41(1), 68–73. https://doi.org/10.3109/00952990.2014.977486
- Herbert, M. S., Dochat, C., Wooldridge, J. S., Materna, K., Blanco, B. H., Tynan, M., Lee, M. W., Gasperi, M., Camodeca, A., Harris, D., & Afari, N. (2022). Technology-supported acceptance and commitment therapy for chronic health conditions: A systematic review and meta-analysis. Behaviour Research and Therapy, 148, 103995. https://doi.org/10.1016/j.brat.2021.103995
- Higgins, J. P., Li, T., & Deeks, J. J. 2022. Chapter 6: Choosing effect measures and computing estimates of effect. In J. P. Higgins, J. Thomas, J. Chandler, M. Cumpston, T. Li, M. Page, & V. Welch (Eds.), Cochrane handbook for systematic reviews of interventions. version 6.3 (updated February 2022). Cochrane. http://www.training.cochrane.org/handbook
- Higgins, J. P. T., & Thompson, S. G. (2002). Quantifying heterogeneity in a meta-analysis. Statistics in Medicine, 21(11), 1539–1558. https://doi.org/10.1002/sim.1186
- IntHout, J., Ioannidis, J. P. A., Rovers, M. M., & Goeman, J. J. (2016). Plea for routinely presenting prediction intervals in meta-analysis. BMJ Open, 6(7), e010247. https://doi.org/10.1136/bmjopen-2015-010247
- Kelson, J., Rollin, A., Ridout, B., & Campbell, A. (2019). Internet-delivered acceptance and commitment therapy for anxiety treatment: Systematic review. Journal of Medical Internet Research, 21(1), e12530. https://doi.org/10.2196/12530
- Levin, M. E., Haeger, J., & Cruz, R. A. (2019). Tailoring acceptance and commitment therapy skill coaching in the moment through smartphones: Results from a randomized controlled trial. Mindfulness, 10(4), 689–699. https://doi.org/10.1007/s12671-018-1004-2
- Levin, M. E., Krafft, J., & Levin, C. (2018). Does self-help increase rates of help seeking for student mental health problems by minimizing stigma as a barrier? Journal of American College Health, 66(4), 302–309. https://doi.org/10.1080/07448481.2018.1440580
- Levin, M. E., Stocke, K., Pierce, B., & Levin, C. (2017). Do college students use online self-help? A survey of intentions and use of mental health resources. Journal of College Student Psychotherapy, 32(3), 181–198. https://doi.org/10.1080/87568225.2017.1366283
- Linardon, J. (2020). Can acceptance, mindfulness, and self-compassion be learned by smartphone apps? A systematic and meta-analytic review of randomized controlled trials. Behavior Therapy, 51(4), 646–658. https://doi.org/10.1016/j.beth.2019.10.002
- Linardon, J., & Fuller-Tyszkiewicz, M. (2020). Attrition and adherence in smartphone-delivered interventions for mental health problems: A systematic and meta-analytic review. Journal of Consulting and Clinical Psychology, 88(1), 1–13. https://doi.org/10.1037/ccp0000459
- Lindhiem, O., Bennett, C. B., Trentacosta, C. J., & McLear, C. (2014). Client preferences affect treatment satisfaction, completion, and clinical outcome: A meta-analysis. Clinical Psychology Review, 34(6), 506–517. https://doi.org/10.1016/j.cpr.2014.06.002
- Melcher, J., Camacho, E., Lagan, S., & Torous, J. (2020). College student engagement with mental health apps: Analysis of barriers to sustained use. Journal of American College Health, 70(6), 1–7. https://doi.org/10.1080/07448481.2020.1825225
- Musiat, P., Johnson, C., Atkinson, M., Wilksch, S., & Wade, T. (2021). Impact of guidance on intervention adherence in computerised interventions for mental health problems: A meta-analysis. Psychological Medicine, 52(2), 229–240. https://doi.org/10.1017/S0033291721004621
- National Center for Health Statistics. (2022, March 23). Mental Health—Household Pulse Survey—COVID-19. https://www.cdc.gov/nchs/covid19/pulse/mental-health.htm
- O’Connor, M., Munnelly, A., Whelan, R., & McHugh, L. (2018). The efficacy and acceptability of third-wave behavioral and cognitive ehealth treatments: A systematic review and meta-analysis of randomized controlled trials. Behavior Therapy, 49(3), 459–475. https://doi.org/10.1016/j.beth.2017.07.007
- Öst, L.-G. (2014). The efficacy of acceptance and commitment therapy: An updated systematic review and meta-analysis. Behaviour Research and Therapy, 61, 105–121. https://doi.org/10.1016/j.brat.2014.07.018
- R Core Team. (2022). R: A language and environment for statistical computing. R Foundation for Statistical Computing. https://www.R-project.org/
- RStudio Team. (2022). RStudio: Integrated development environment for R. RStudio, PBC. http://www.rstudio.com/
- Sagon, A. L., Danitz, S. B., Suvak, M. K., & Orsillo, S. M. (2018). The mindful way through the semester: Evaluating the feasibility of delivering an acceptance-based behavioral program online. Journal of Contextual Behavioral Science, 9, 36–44. https://doi.org/10.1016/j.jcbs.2018.06.004
- SAMHSA. (2021). Key Substance Use and Mental Health Indicators in the United States: Results from the 2020 National Survey on Drug Use and Health 156. https://www.samhsa.gov/data/sites/default/files/reports/rpt35325/NSDUHFFRPDFWHTMLFiles2020/2020NSDUHFFR1PDFW102121.pdf
- Shim, M., Mahaffey, B., Bleidistel, M., & Gonzalez, A. (2017). A scoping review of human-support factors in the context of internet-based psychological interventions (IPIs) for depression and anxiety disorders. Clinical Psychology Review, 57, 129–140. https://doi.org/10.1016/j.cpr.2017.09.003
- Sierra, M. A., Ruiz, F. J., Universitaria Konrad Lorenz, F., & Flórez, C. L. (2018). A systematic review and meta-analysis of third-wave online interventions for depression. Revista Latinoamericana de Psicología, 50(2), 126–135. https://doi.org/10.14349/rlp.2018.v50.n2.6
- Stawarz, K., Preist, C., & Coyle, D. (2019). Use of smartphone apps, social media, and web-based resources to support mental health and well-being: Online survey. JMIR Mental Health, 6(7), e12546. https://doi.org/10.2196/12546
- Thompson, E. M., Destree, L., Albertella, L., & Fontenelle, L. F. (2021). Internet-based acceptance and commitment therapy: A transdiagnostic systematic review and meta-analysis for mental health outcomes. Behavior Therapy, 52(2), 492–507. https://doi.org/10.1016/j.beth.2020.07.002
- Torous, J., Levin, M. E., Ahern, D. K., & Oser, M. L. (2017). Cognitive behavioral mobile applications: Clinical studies, marketplace overview, and research agenda. Cognitive and Behavioral Practice, 24(2), 215–225. https://doi.org/10.1016/j.cbpra.2016.05.007
- Viechtbauer, W. (2010). Conducting meta-analyses in R with the meta for package. Journal of Statistical Software, 36(3). https://doi.org/10.18637/jss.v036.i03
- Wallin, E., Maathz, P., Parling, T., & Hursti, T. (2018). Self-stigma and the intention to seek psychological help online compared to face-to-face. Journal of Clinical Psychology, 74(7), 1207–1218. https://doi.org/10.1002/jclp.22583