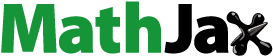
Abstract
In this article, a new class of weighted exponential distribution called Entropy-Based Exponential weighted distribution (EBEWD) is proposed. The main idea of the new lifetime distribution is in using the Shannon entropy measure as a weighted function. The mathematical properties of the EBEWD, such as reliability measures, moment measures, variability measures, shapes measures, entropy measures and Fisher’s information, were derived. The unknown parameters of the proposed distribution were estimated using the maximum likelihood estimation method. The efficiency and flexibility of the EBEWD were examined numerically and then by a real-life data application. The results revealed that the EBEWD outperforms some existing distributions in terms of their test statistics.
1. Introduction
The method of weighted distributions as proposed by Fisher’s [Citation1] models of ascertainment on how recorded the probabilities of the events. Weighted distributions are used to select the suitable models for observed data with a technique for fitting models and suitable fits for the data. Rao [Citation2] provides a method of ascertainment concept and how the general formula of weighted distribution is useful for standardizing statistical distributions.
Many researchers start using the concept ofweighted distribution and used the idea of weighted distributions on how effect the original distribution with the new weighted function. Cox [Citation3] suggested using and calling the new distribution by length-biased weighted distribution. Rao [Citation2] used the weight function
and proposed the so-called size-biased weighted distribution of order
. Different weight functions are used in the literature; illustrates some of these weight functions with some related references.
Table 1. Improper weight functions.
The main idea of this paper is to introduce a new weight function that is used in quantifying the maximum amount of information in a random variable called entropy [Citation4] to the context of weighted distributions. Accordingly, the article is organized as follows: the next section introduces the new weigh function. The new exponential probability density function is given in Section 3. The statistical measures of the modified exponential distribution are given from Section 3 to Section 10. Parameter estimation is given in Section 11 while in Section 12 the benefit of fitting data to the new distribution model based on a real case study is discussed. The article ends with a concluding remark section.
2. Proposed weighted function
Let X be a random variable with probability density function (pdf) where
is an unknown parameter, then the corresponding weighted density function is defined as follows:
(1)
(1)
where
is a non-negative weighted function; and
is the expected value of the weighted function. It is assumed that the expected value of the weighted function exists, and it is positive. The problem in formulating the weighted distribution occurs in selecting a suitable weighted function such that the new distribution is more suitable than the original distribution
, and provides a better fit for the data than some existing distributions.
The suggested weighted function in this article is entropy, which is defined by Shannon [Citation5] as a negative average of the logarithm of a pdf and called the amount of self-information for a given random variable. Statistically, let X be a continuous random variable with pdf , then the entropy is defined as follows:
(2)
(2)
Based on (2), the suggested weight function will be
Then using this function in (1), we will have the new formulation of the weighted distribution as follows:
(3)
(3)
3. Entropy-based weighted exponential distribution
The exponential distribution is the most known and used continuous distribution for lifetime data applied in many sciences, such as engineering, economics, medicine, biomedical and in many industrial applications. However, the exponential distribution does not provide a significant fit for some real life-time applications, where the hazard rate function and the mean residual life function of exponential distribution are always constant. Therefore, a more suitable distribution is needed; one of the creative ideas is to form a weighted form of the exponential distribution with a given weight function.
Definition 3.1:
A non-negative continuous random variable X is said to have an exponential distribution if it has a pdf and CDF:
(4)
(4)
and
(5)
(5)
where
is the distribution parameter and called the rate of the distribution. When
, then the distribution is the standard exponential.
Corollary 3.1:
Let X be a non-negative continuous random variable X with pdf as given in 4, then the entropy is
This result can be used to develop a new pdf. Therefore, using (1), the entropy-based weighted exponential distribution (EB-WED) will be
(6)
(6)
The cumulative distribution function CDF of the EB-WED is
(7)
(7)
Note that, the pdf given in (6) is a non-negative real-valued function, which means
. This is true iff
Based on this fact, some restriction on the pdf range depends on the parameter value. Three cases were obtained as follows:
if
; then
if
; then
if
; then
shows that the pdf is skewed to the right. However, when belongs to the interval
or
, then the pdf has a clear shoulder as
increases. When
the pdf has shrinkages and does not have a clear shoulder. Moreover, the CDF is given in noting that
must be held.
4. Moments and related measures of the EB-WED
The rth moment of the EB-WED random variable is defined as
Now, based on three cases the pdf given in (7) the rth moment of X is
Theorem 4.1:
Let X be a random variable following the EB-WED. Then, the rth moment of X is
where
Proof:
The rth moment of the EB-WED can be obtained using the mathematical expectation:
which can be simplified to
which can be simplified to
End of the proof for case 1.
Now case 3 has
The integration within this expected value can be evaluated easily based on the incomplete gamma function and using the following:
Therefore,
which can be simplified to
which ends the proof of case 3. Noting that Case 2 can be obtained directly by finding the differences between case 1 and case 3.
To reduce the number of cases due to the dependence of the variable range on the parameter value, and for the similarities of steps in getting the results, we will consider the results in this article when .
Theorem 4.2:
Assume that the random variable X follows the EB-WED, then the moment-generating function, (t), of X is defined as
Proof
The moment-generating function is defined as
Therefore,
This can be simplified to
After distributing the terms we have
Then
This ends the proof.
Theorem 4.3:
The harmonic mean of EB-WED distribution is defined as
Proof:
The following integral is used to compute the Harmonic mean
Therefore
After rearranging the terms, we have
This can be simplified to
Then
where
This ends the proof.
Theorem 4.4:
The mode of the EB-WED distribution is
Proof
The pdf of the EB-WED distribution is and its logarithm
After rearranging the terms, we have
Finding the first derivative with respect to x, we have
Setting to zero and solving for x to get
This ends the proof.
5. Measures of variability and distribution shape of the EB-WED
Measures of variability or relative variability can be obtained using the first two moments, based on Theorem 1:
The mean E(X) of the EB-WED is
The second moment E(
) of the EB-WED is
The third moment E(
) of the EB-WED is
The fourth moment E(
) of the EB-WED is
is defined as
Moreover, the coefficient of kurtosis as
for EB-WED is defined as
gives a numerical illustration of all statistical measures of the EB-WED with different parameter values. The results indicated that the population means values increase as the value of
increases, noting that when
, then EB-WED is the standard exponential distribution. While the values of the variance, coefficient of variation, skewness and kurtosis decrease as
increases.
Table 2. Numerical values of all statistical measures for the EB-WED.
6. Measures of reliability
In this section, the Measure of Reliability of the EB-WED distribution is derived.
Corollary 6.1:
Suppose that X is a non-negative continuous random variable that has the pdf and CDF as given in 7 and 8, respectively, then
The hazard function is
The reliability functions
The reversed hazard rate function
The odds function
The above figures indicate that as increases the hazard rate function and the odd function increases while it decreases with the other reliability measures.
7. Stochastic orderings
Let X and Y be two continuous random variables with probability density functions and
, and the associated cumulative distributions functions are
, then
Stochastic order
if
for all x;
Hazard rate order
if
for all x;
Mean residual life order
if
for all x;
Likelihood ratio order
if
decreases in x.
Thus,
Theorem 7.1:
Let and
and corresponding distribution functions
and
. If
then
and
,
and
Proof:
Stochastic order: needs to show that
for all x
For then
For all x, then X is stochastically smaller than Y based on stochastic order
Hazard rate order: needs to show that
for all x
For then
For all x, then X is smaller in the hazard rate ordering than Y-based hazard rate order
Mean residual life order: needs to show that
for all x
For then
For all x, then
is smaller in the mean residual life order than
-based mean residual life order
.
Likelihood ratio order: needs to show that
is a decreasing function of x
8. Information measures
The information measure of the EB-WED random variable is derived as follows:
Theorem 8.1:
Let X be a continuous random variable that has EB-WED distribution, then the Rényi entropy of X is defined as
Proof
Using the Rényi entropy as defined by Rényi [Citation22]
,then we have
which can be simplified to
After rearranging the terms,
Now if assume that
, then du =
dx
Replace x with u, then the integral will be
which can be simplified to
Using integral on gamma function, we have
After rearranging the terms
This ends the proof.
Theorem 8.2:
Let X be a continuous random variable that has EB-WED distribution, then the Shannon entropy of X is defined as follows:
Proof
Using Shannon entropy , then the Shannon entropy of EB-WED can be written in terms of integration:
Then using the properties of the ln function, we have
This is can be written in terms of expected value:
where
E(X) =
;
;
and
which can be obtained by setting u = then
Therefore
which is equivalent to
Noting that
is the logarithmic integral function that is defined by
This ends the proof.
9. Extreme order statistics
In this section, the pdf of the minimum and the maximum order statistic of the EB-WED is given. Let X(1:n), X(2:n), … , X(n:n) denote the order statistics of a random sample of size n; X1, X2 … , Xn; from a continuous population with pdf and CDF
, then the pdf of the ith order statistic X(i:n) is defined as follows:
Using 7 and 8 will be derived; the pdf of the minimum and the maximum order statistic will be
10. Fisher’s information
Derive Fisher’s information of the EB-WED random variable based on
Theorem 10.1:
Let X follow the EB-WED distribution, and then its Fisher’s information, FIEB-WED (λ) is
Proof
Fisher’s information is defined as
Now, the log of the pdf of the EB-WED is
By differentiating the last equation with respect to
, then
The second differentiation is
Now
This ends the proof.
provides some value of Fisher’s information for EB-WED when λ = 0.1, 0.2, … , 1.3. The results indicated that as increases as
decreases, hence the data provide a lot of “information” about λ
Table 3. Fisher’s information values of the EB-WED for λ value.
11. Parameter estimation
In this section, we use the maximum likelihood estimation method to find a point estimator of the unknown parameter in the EB-WED. Suppose that is a random sample of size n (independent and identically distributed) following the EB-WED, as given in 7. Using MLE, then the likelihood function is given as follows:
which is equivalent to
Finding the logarithm of the likelihood function
By differentiating with respect to
The maximum likelihood
of
is obtained by equating the above equation to zero. Since the equation is complicated, the point estimator can be found by employing a numerical method to find the point estimator numerically under the following condition:
12. Application
In this section, real data analysis will be considered to study the performance of the new distributions. Then a comparison will be made with some fitted lifetime distributions: The Marshall Olkin Esscher transformed Laplace distribution, Lindley distribution and exponential distribution.
The one-parameter Marshall – Olkin Esscher transformed Laplace distribution (MOETLD) was provided by George and George [Citation23] with pdf
Lindley distribution was provided by Lindley [Citation24] with pdf
12.1. Goodness of fit measures
Maydeu et al [Citation25]. The goodness of fit is some of the statistical model for summarize the fit of observation, and how perform model comparisons, where in there mathematical formulas have the value of the log-likelihood function k is the number of estimated parameters and n is the sample size.
(I) Information Criterion Measures:
Bayesian Information Criterion (BIC), Schwarz [Citation26]:
It is a statistical measure used to compare between the performances of a set of models, the lower the value the better the model.
Akaike Information Criterion (AIC), Akaike [Citation27]:
How well the data are explained by the model suitability and complexity of the model, and the performance of a model is predicted by minimizing the function. The lower the value, the better the model.
Consistent Akaike Information Criterion (CAIC), Bozdogan [Citation28]:
It is a model selection criteria provide an asymptotically how will the model fit the data, and give unbaised estimate of the order of the true model. The model with more parameters fits better than the model that has fewer parameters
Hannan – Quinn Information Criterion (HQIC) – Hannan and Quinn [Citation29]: the autoregressive of the model, HQIC is strongly consistent; the lower the value the better the model.
Maximized log-likelihood (MLL), Heggland andLindqvist [Citation30]:
The likelihood ratio function is expressing the relative likelihood of the data given two competing models. The equation of EB-WED:
(II) Test of Hypothesis
Anderson – Darling Criterion (A–D), Stephens [Citation31]:
Test, if the sample of data has specific distributionand use it in calculating critical value.
Camér – von Mises Criterion (C – M), Cramér [Citation32]:
Compare the goodness of fit of a probability distribution to empirical distributions
Chi-Square test, Chakravarty et al. [Citation33]: A measure of goodness of fit to test if the data are distributed according to the distribution used.
Oi = an observed frequency for bin i
Ei = an expected frequency for bin i
Dataset: Murthy et al [Citation34], Aircraft windshield components should have high-performance material and must be able to bear high temperatures and pressures, the company needs to test its product and make it high quality and suitable for different aircraft models. It finds failures items that result from the damaged Windshield, and then the time of repairing faulty items is recorded as service time Wallace et al. [Citation35]. In this study, the data are used to measure the service times for Aircraft windshields. The remaining 63 are service times of windshields. The unit for measurement is 1000 h, with mean 2.08, variance 1.55, min 0.046, max 5.14, skewness 0.439 and kurtosis −0.267, reported as
The distribution fitting results are given in Tables and . In addition, visual comparisons between the empirical distribution and the theoretical distribution are given in .Table 4. Estimation and information criterions for aircraft data.
Table 5. Distribution fitting tests for the aircraft data.
Based on the values of the AIC, BIC, CAIC and HQIC tests the Entropy-Based Exponential Weighted Distribution provides the best fit for the data. Based on the chi-square test for distributions, the proposed weighted distribution shows a significant result to fit the Aircraft data with p-values of 0.45 for EB-WED. This means the proposed distributions are more suitable than the other distributions in fitting the Aircraft data.
13. Concluding remarks
In this article, we proposed a new exponential type of probability density function called the Entropy-Based Weighted Exponential Distribution. Several of its statistical properties, such as the moments’ measures, variability and distribution measures, reliability, hazard, reversed hazard and odds functions, are provided and studied. Moreover, Fisher information and the Maximum-likelihood estimators of the model parameters are obtained. Finally, a real dataset on the Aircraft’s windshield component is analysed and the results are compared to several life-time models. The results showed that the proposed entropy-based exponential model is more flexible and has a better fit for the suggested data.
In this article, we used the Shannon entropy to formulate the weighted distribution. The proposed distribution could be considered a new value distribution that could be used for fitting real-life data. Note that dealing with entropy measures in formulating a new lifetime distribution is not an easy task; however, the window of further research is still open, for example, one can use other entropy measures such as Renyi or Tsallis in formulating the weighted distribution; and the results could be used to compare the new suggested models with the proposed model in this article in terms of model flexibility and bathtub and other reliability measures.
Disclosure statement
No potential conflict of interest was reported by the author(s).
References
- Fisher RA. The effects of methods of ascertainment upon the estimation of frequencies. Ann Eugen. 1934;6(1):13–25.
- Rao CR. On discrete distributions arising out of methods of ascertainment. In: Patil GP, editor. Classical and contagious discrete distribution. Calcutta: Pergamon Press and Statistical Publishing Society; 1965, 27(2); p. 311–324.
- Cox DR. Some sampling problems in technology. In: Johnson NL, Smith H, editor. New developments in survey sampling. New York: John Wiley and Sons; 1968. p. 506–527.
- Al-Nasser AD, Al-Omari A, Ciavolino E. A size-biased Ishita distribution and application to real data. Qual Quant. 2019;53(1):493–512.
- Shannon CE. A mathematical theory of communication. Reprinted with Corrections from the Bell Syst Tech J. 1948;27(3):379–423.
- Rezzoky A, Nadhel Z. Mathematical study of weighted generalized exponential distribution. Journal of the College of Basic Education. 2015;21(89):165–172.
- Mir K, Ahmed A, Reshi J. Structural properties of length biased Beta distribution of first kind. American Journal of Engineering Research. 2013;2:01–06.
- Mudasir S, Ahmad S. Structural properties of length-biased Nakagami distribution. International Journal of Modern Mathematical Sciences. 2015;13(3):217–227.
- Shi X, Broderick O, Pararai M. Theoretical properties of weighted generalized Rayleigh and related distributions. Theoretical Mathematics and Applications. 2012;2:45–62.
- Ye Y, Oluyede B, Pararai M. Weighted generalized Beta distribution of the second kind and related distributions. Journal of Statistical and Econometric Methods. 2012;1:13–31.
- Das K, Roy T. Applicability of length biased generalized Rayleigh distribution. Advances in Applied Science Research. 2011;2:320–327.
- Jabeen S, Jan T. Information measures of size biased generalized Gamma distribution. International Journal of Scientific Engineering and Applied Science. 2015;1:2395–3470.
- Aleem M, Sufyan M, Khan N. A Class of Modified weighted Weibull distribution and its properties. American Review of Mathematics and Statistics. 2013;1:29–37.
- Castillo J, Casany M. Weighted Poisson distribution for over dispersion and under dispersion solutions. Annals of Institute of Statistical Mathematics. 1998;50:567–585.
- Larose D, Dey D. Weighted distributions viewed in the context of model selection: A bayesian perspective. Test. 1996;5(1):227–246.
- Mahfoud M, Patil G. On weighted distributions. In: Kallianpur G. et al. editors. Statistics and probability essays in honor of C. R. Rao. Amsterdam: North-Holland Publishing Company. 1982; p. 479–492.
- Al-Kadim A, Hussein A. New proposed length biased weighted exponential and rayleigh distributions with application. Mathematical Theory and Modeling. 2014;4(7):137–52.
- Al-Kadim K, Hantoosh A. Double weighted distribution and double weighted exponential distribution. Mathematical Theory and Modeling. 2013;3(5):124–34.
- Bashir S, Rasul M. Some properties of the weighted Lindley distribution. International Journal of Economics and Business Review. 2015;3:11–17.
- Modi K. Length-biased weighted Maxwell distribution. Pakistan Journal of Statistics and Operation Research. 2015;11:465–472.
- Kharazmi O, Mahdavi A, Fathizadeh M. Generalized weighted exponential distribution. Communications in Statistics – Simulation and Computation. 2014;44(6):1557–1569.
- Renyi A. On measures of entropy and information. In: Jerzy Neyman editor. Proceedings of the 4th Berkeley symposium on mathematical statistic and probability. Vol. I. Contributions to Probability and Theory. Berkeley: University of California Press; 1961. p. 547–561.
- George D, George S. Marshall–Olkin Esscher transformed Laplace distribution and processes. Braz J Prob Stat. 2013;27(2):162–184.
- Lindley DV. Fiducial distributions and Bayes’ Theorem. J R Stat Soc. 1958;20:102–107.
- Maydeu A, Garci A, Forero C. Goodness-of-Fit testing. International Encyclopedia of Education. 2010;7:190–196.
- Schwarz G. Estimating the dimension of a model. Ann Stat. 1978;6(2):461–464.
- Akaike H. A New look at the statistical model identification. IEEE Trans Autom Control. 1974;19(6):716–723.
- Bozdogan H. Model selection and Akaike’s information criterion (AIC): the general theory and its analytical extensions. Psychometrika. 1987;52(3):345–370.
- Hannan EJ, Quinn BG. The determination of the order of an autoregression. J R Stat Soc B Methodol. 1979;41(2):190–195.
- Heggland K, Lindqvist BH. A nonparametric monotone maximum likelihood estimator of time trend for repairable systems data. Reliab Eng Syst Saf. 2007;92:575–584.
- Stephens MA. EDF statistics for goodness of Fit and some comparisons. J Am Stat Assoc. 1974;69:730–737.
- Cramér H. On the composition of elementary errors. Scand Actuar J. 1928;1928(1):13–74.
- Laha C, Laha RG. Handbook of methods of applied statistics. New York: John Wiley and Sons; 1967. 1; p. 392–394.
- Murthy DNP, Min X, Jiang R. Weibull models. New York: John Wiley and Sons; 2004. 1047(22).
- Blischke WR, Prabhakar Murthy DN. Reliability: modeling, prediction, and optimization. New York: John Wiley and Sons; 2011.