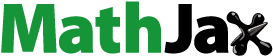
Abstract
In the search for new metabolite inhibitors, a natural product activity and species source (NPASS) database was virtually screened using AutoDock software to identify potential NRP1 inhibitors. NPASS compounds complexed with NRP1 were subjected to molecular dynamics (MD) simulations. Furthermore, NPASS-NRP1 binding affinities were calculated using the MM-GBSA approach. Based on calculated binding energies, kamolonol (NPC146388) demonstrated lower NRP1 binding affinity than the co-crystallized HRG/Arg-1 ligand with binding energy (ΔGbinding) values of –34.5 and –32.0 kcal/mol, respectively. Structural and energetic analysis showed high stability for kamolonol and HRG/Arg-1 with NRP1 over the 200 ns MD simulations. The studied physicochemical properties of kamolonol and HRG/Arg-1 revealed that these compounds obey Lipinski's rule of five. ADMET characteristics of kamolonol and HRG/Arg-1 were predicted, and kamolonol showed better ADMET properties compared to HRG/Arg-1. Based on these results, kamolonol is a promising NRP1 inhibitor worthy of further experimental assays as anti-carcinoma remediation.
1. Introduction
Biological alteration that results in unregulated cell growth [Citation1] is referred to as cancer and is a worldwide health challenge with poor clinical outcomes [Citation2,Citation3]. Approximately 10 million deaths were reported in 2020 by global cancer statistics [Citation4]. Despite ongoing progress in surgery and radiotherapy, patients with advanced tumours still have poor prognoses [Citation5]. Moreover, there is only a small number of newly approved cancer medications released annually by the US Food and Drug Administration (FDA) [Citation6]. With resistance to therapeutic drugs a substantial problem in treating cancer [Citation7], there is a critical need for novel cancer therapies.
Numerous cancers, including gastric, urinary, bladder, breast, lung, and esophageal, have high levels of neuropilin-1 (NRP1) protein [Citation8–11]. NRP1 is a cell surface receptor that binds vascular endothelial growth factor (VEGF), a potent mediator of endothelial permeability, chemotaxis, and proliferation. It is also a regulator for tumour development, proliferation, invasion, metastases, and prognosis [Citation12–16]. NRP1 enhances angiogenesis by forming a direct complex with endothelial growth factors A and 2 (VEGFA and VEGFR2) [Citation17]. It also promotes the tumour's development after binding with the VEGFA due to its ability to promote RhoA activation [Citation18]. Besides, NRP1 has the ability to accelerate neoplasm progression by steadying the role of regulatory T cells (Tregs) and banning the tumour-associated macrophages (TAM) from penetrating normoxic cancer areas [Citation19]. Although NRP1 protein has received considerable critical attention as a potential therapeutic target for cancer therapy [Citation20,Citation21], no drug has been approved as an NRP1 inhibitor.
Natural products (NPs) retrieved from microbes and plants have a vital role in drug discovery, particularly against carcinoma and contagious illnesses [Citation22,Citation23]. Several drugs are natural products from plant sources, such as paclitaxel, morphine, and vinblastine [Citation24]. Therefore, there is abundant opportunity for mining novel anticancer drug candidates as NRP1 inhibitors from the Natural Product Activity and Species Source (NPASS) database. In the current study, NPASS database was screened for promising NRP1 inhibitors utilizing molecular docking computations [Citation25]. Subsequently, promising NPASS compounds were selected and subjected to molecular dynamics (MD) simulations and the corresponding binding energies were estimated using the MM-GBSA approach. The stability and tightness of the most potent NPASS compounds within NRP1 were selected. Thus, the current study highlights the potentiality of NPASS candidates as NRP1 inhibitors and illustrates a promising drug candidate for clinical consideration.
2. Computational methodology
2.1. NRP1 preparation
The neuropilin-1 b1 domain (NRP1) atomic coordinates in complex with L-homoarginine (HRG/Arg-1) were retrieved from the protein databank (PDB code: 5ijr; resolution: 1.52 Å [Citation26]) and considered as a template for all in-silico computations. The crystallographic water molecules, ions, and ligand were eliminated from the NRP1 protein, keeping only the amino acids. The protonation state of the amino acids was investigated using the H++ server, and all missing hydrogen atoms were inserted [Citation27].
2.2. Database preparation
The Natural Product Activity and Species Source (NPASS) database, containing more than 35,000 natural compounds, was downloaded in SDF format [Citation25]. The duplicated compounds were eliminated based on the International Chemical Identifier (InChIKey) [Citation28]. The three-dimensional (3D) structures of the NPASS compounds were generated using Omega2 software [Citation29,Citation30] and minimized utilizing the MMFF94S force field integrated inside SZYBKI software [Citation31,Citation32]. The protonation state of the NPASS compounds was examined using fixpka algorithm within the QUACPAC software, respectively [Citation33]. The Gasteiger-Marsili method was used for the determination of the partial charges of NPASS compounds [Citation34]. The prepared files are present in a CompChem database (www.compchem.net/ccdb) that is publically accessible. The schematic diagram of the utilized computational approaches is depicted in Figure .
2.3. Molecular docking
AutoDock Vina1.1.2 software was used for executing molecular docking calculations [Citation35]. The NRP1 protein was converted into pdbqt format using MGL tools (version 1.5.7) [Citation36]. The details of the employed protocol for the docking calculations are described elsewhere [Citation37–39]. Except the exhaustiveness parameter, which was set to 50 and 200 in fast and expensive calculations, respectively, the remaining parameters were preserved at the default settings. The grid box was tailored to fit the active site of NRP1 protein by taking the centroid of HRG/Arg-1 as a centre of the grid, with a grid size of 15 Å × 15 Å × 15 Å and a value of spacing equal to 1 Å. The grid was centred at 32.485, –11.49, and 27.507 (in x, y, and z dimensions, respectively). The top nine scoring poses were estimated via visual investigation and the lowest docking score was selected as a representive mode.
2.4. Molecular dynamics simulations
The most effective NPASS compounds complexed with the NRP1 protein underwent molecular dynamics (MD) simulations using AMBER16 software [Citation40]. The details of MD simulations are described elsewhere [Citation41–45]. Briefly, the inspected NPASS compounds were defined utilizing the general AMBER force field (GAFF2) [Citation46], while the NRP1 protein was characterized using the AMBER force field of 14SB [Citation47].
MD simulations were conducted employing both implicit and explicit water solvents. In the implicit water solvent MD simulations, the atomic partial charges of the inspected NPASS compounds were estimated using an AM1-BCC method with the aid of the Antechamber tool within the AMBER16 [Citation48]. Periodic boundary conditions were not utilized, and the cutoff value was set to 999 Å. Additionally, the solvent model (igb = 1) was used to present the solvation contributions [Citation49]. All the investigated systems were first minimized for 500 steps. The minimized complexes were warmed progressively from 0 to 300 K over 10 ps. Thereafter, the NPASS-NRP1 complexes were subjected to an equilibrium stage of 50 ps, followed by a production stage of 1 ns.
In explicit water solvent MD simulations, the restricted electrostatic potential (RESP) approach was employed to determine the partial charge of each atom of the inspected NPASS compounds at the HF/6-31G* level of theory [Citation50]. Quantum mechanics calculations were performed using Gaussian09 software [Citation51]. TIP3P water molecules were utilized to solvate NPASS-NRP1 complexes in an octahedron box with a minimal distance of 12 Å [Citation52]. All investigated systems were minimized by employing 5000 cycles before heating from 0 to 300 K over 50 ps under the NVT ensemble. NPASS-NRP1 complexes were then equilibrated over 1 ns under the NPT ensemble. The equilibrated coordinates of the systems were subjected to production runs of 10 and 200 ns. Atomic coordinates were recorded every 10 ps for post-dynamics analyses and binding free energy estimations. The Particle Mesh Ewald (PME) approach was applied to treat long-range electrostatic interactions [Citation53]. Berendsen barostat with a 2 ps relaxation duration was employed to adjust the atmospheric pressure [Citation54]. To keep temperature steady at 298 K, Langevin dynamics with gamma_ln set to 1.0 for collision frequency was applied [Citation55]. The SHAKE algorithm was applied to constrain all bonds involving hydrogen bonds at the time step of 2 fs [Citation56]. The GPU version of pmemd (pmemd.cuda) was applied to perform MD simulations. The molecular visualizations were generated with the help of BIOVIA Visual Studio2020 [Citation57].
2.5. MM-GBSA binding energy
Binding energies of inspected NPASS compounds in complex with NRP1 were calculated using a molecular mechanics-generalized Born surface area (MM-GBSA) approach [Citation58]. An updated generalized born model including Onufriev (igb = 2) was used for computing the polar solvation energy [Citation59]. The MM-GBSA binding energies were computed based on statistically independent snapshots gathered from MD simulations in accordance with the following equation:
The energy term (G) was determined as:
Through employing generalized born (GB) models, the electrostatic solvation energy (ΔGGB) in the abovementioned equation was determined with GSA standing for nonpolar solvation-free energy. Eele refers to electrostatic energy. Evdw is van der Waals energy. The entropic contributions are often disregarded because of high computational costs [Citation60,Citation61].
2.6. Drug-likeness characteristics
The physicochemical characteristics of the identified compound were estimated utilizing a SwissADME online website (http://www.swissadme.ch). The estimated characteristics included topological polar surface area (TPSA), relative molecular weight (MW), number of hydrogen bond donors (nOHNH), number of hydrogen bond acceptors (nON), and the partition coefficient (MlogP) [Citation62].
2.7. ADMET characteristics
ADMET properties of the identified compounds were evaluated by an online pkCSM web server [Citation63]. The absorption (A) was estimated by human intestinal absorption (HIA) and Caco-2 permeability. Distribution (D) was predicted based on blood–brain barrier (BBB) permeability and steady-state distribution volume (VDss). The metabolism (M) was detected using CYP3A4 inhibitor/substrate. Excretion (E) is specified according to the total clearance of the drug. Finally, the toxicity (T) was determined based on hepatotoxicity.
3. Results and discussion
3.1. In-silico protocol assessment
The performance of AutoDock Vina1.1.2 software in predicting the correct ligand-NRP1 binding pose was assessed. For assessment purposes, the co-crystallized HRG/Arg-1 inhibitor was re-docked in NRP1 binding site, and the predicted docking pose was compared to the experimental binding mode (PDB code: 5ijr [Citation26]) (Figure ). As depicted in Figure , the docking pose was similar to the native structure of the co-crystallized HRG/Arg-1 ligand with an RMSD value of 0.23 Å. AutoDock Vina results showed a docking score of –5.6 kcal/mol, forming six significant hydrogen bonds with TRP301 (2.01 Å), ASP320 (1.65, 3.11 Å), SER346 (2.60 Å), GLU348 (2.44 Å), and ILE415 (2.79 Å). Based on these results, AutoDock Vina software was utilized to screen the NPASS database for potential NRP1 inhibitors.
Figure 2. (a) (i) Superimposed structures of the co-crystalized mode (in grey) and the predicted docking pose (in green) and (ii) 2D molecular interaction of L-homoarginine (HRG/Arg-1). (b) (i) 3D and (ii) 2D representations of the predicted binding mode for kamolonol (NPC146388) within the NRP1 active site. The predicted docking score in kcal/mol is also listed.
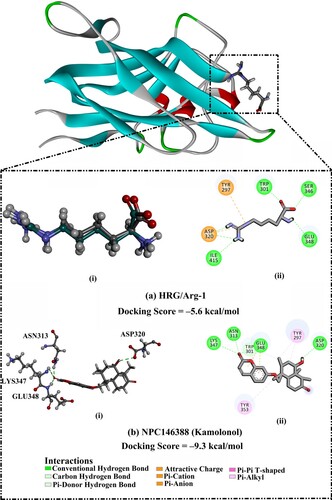
3.2. Virtual screening of the NPASS database
Molecular docking calculations were executed via two stages of accuracy that were named fast and expensive docking stages (see computational methodology section for details). The NPASS database, containing greater than 35,000 compounds, was virtually screened using fast docking computations. The docking scores of NPASS compounds were predicted and compared to that of the co-crystallized HRG/Arg-1 ligand (calc. –5.6 kcal/mol). NPASS compounds with docking scores <−7.0 kcal/mol were selected and subjected to expensive docking computations, giving a total number of 1,332 compounds. Due to a large number of investigated compounds, a threshold value of –7.0 kcal/mol was selected to shortlist the prospective NRP1 inhibitors. Table S1 provides the evaluated fast and expensive docking scores for the top 1,332 NPASS compounds against the NRP1 protein. Figure S1 depicts 3D and 2D molecular interactions of the top four potent NPASS compounds with essential residues within the active site of the NRP1 protein. Table summarizes the obtained docking scores, 2D chemical structures, and binding features of the top four potent NPASS compounds within the active site of NRP1.
Table 1. Computed fast and expensive docking scores (in kcal/mol), 2D chemical structures, and binding features for the top four potent NPASS compounds against the NRP1 protein.Table Footnotea
Notably, those four NPASS compounds were filtered according to further binding energy calculations over 10 ns MD simulations. As illustrated in Table and Figure S1, the four NPASS compounds and HRG/Arg-1 demonstrated approximately the same binding modes within the NRP1 active site. The most favorable interactions between the identified NPASS compounds and NRP1 active site were conventional hydrogen bonds and π-based interactions.
Kamolonol (NPC146388), isolated from F. assafoetida gum resin [Citation64], belongs to the class of organic compounds known as coumarins. Kamolonol possesses antiplasmodial characteristics, anti-cellular hypertrophic, and anti-fibrotic effects [Citation65,Citation66]. Besides, kamolonol demonstrated a promising docking score towards NRP1 protein with a value of –9.3 kcal/mol (Table ). The potency of kamolonol as an NRP1 inhibitor can be traced to its ability to exhibit multiple hydrogen bonds with the key amino acids of the NRP1 active site (Figure ). Inspecting the binding mode of kamolonol revealed that the hydroxyl (OH) group of 2-methylcyclohexan-1-ol ring formed a hydrogen bond with the carbonyl (C = O) group of ASP320 amino acid with a bond length of 2.68 Å (Figure ). Besides, the carbonyl (C = O) group of the methylhexanone ring exhibited three hydrogen bonds with the NH group of ASN313 and GLU348 and NH2 group of LYS347 with bond lengths of 2.25, 2.41, and 3.01 Å, respectively (Figure ). In addition, kamolonol demonstrated π-donor hydrogen bond with TRP301 residue and π-alkyl interactions with TYR297 and TYR353 residues.
3.3. Molecular dynamics simulations
Molecular dynamics (MD) simulations of the target-inhibitor complex are essential for inspecting conformational steadiness and changes, internal motions, and the reliability of target-inhibitor binding affinities [Citation67,Citation68]. Consequently, MD simulations were employed herein to investigate the stability and binding affinity of the most potent NPASS compounds complexed with NRP1 protein. Considering computational cost and time, the top 5% promising NPASS compounds (i.e. 67 compounds with expensive docking scores < –8.1 kcal/mol) in complex with NRP1 were subjected to implicit water solvent MD simulations. Additionally, the corresponding MM-GBSA binding energies (ΔGbinding) were estimated and listed in Table S2. As data enrolled in Table S2, four NPASS compounds demonstrated greater binding affinity compared to the co-crystallized HRG/Arg-1 ligand (calc. –28.7 kcal/mol) with binding energies with values in the range of –21.5 to –29.8 kcal/mol. Those four potent NPASS compounds in complex with NRP1 were subjected to explicit water solvent MD simulations for 10 ns for more reliable binding affinities. The corresponding binding energies (ΔGbinding) were calculated and illustrated in Figure .
Figure 3. Estimated MM-GBSA binding energies for the four promising NPASS compounds against the NRP1 active site over 1 ns in implicit water solvent MD simulations and 10 and 200 ns in explicit water solvent MD simulations.
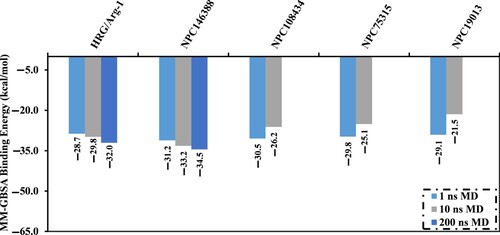
Interestingly, only kamolonol exposed binding energy lower than that of the HRG/Arg-1 (calc. –29.8 kcal/mol) over the MD course of 10 ns. A MD simulation for kamolonol was also prolonged to 200 ns, and the corresponding binding energy (ΔGbinding) was calculated (Figure ). No discernible variation in the predicted binding energies of kamolonol within the NRP1 active site over the different simulated times of 10 and 200 ns MD simulations. Compared to HRG/Arg-1, kamolonol complexed with NRP1 demonstrated lower binding energy over 200 ns MD simulation with ΔGbinding values equal to –34.5 and –32.0 kcal/mol, respectively (Figure ).
Inherent driving forces in the binding of kamolonol and the HRG/Arg-1 were analyzed utilizing MM-GBSA binding energy decomposition (Figure ). Remarkably, the van der Waals forces (ΔEvdw) demonstrated a significant contribution to the binding energy of kamolonol- and HRG/Arg-1-NRP1 complexes, with values of –38.3 and –32.5 kcal/mol, respectively (Figure ).
Figure 4. MM-GBSA binding energy components for kamolonol (NPC146388) and the co-crystallized HRG/Arg-1 ligand with the NRP1 protein over the 200 ns MD simulations.
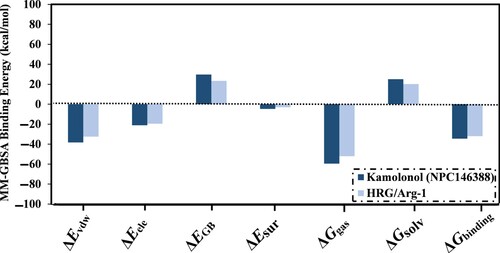
The electrostatic forces (ΔEele) were also adequate for kamolonol and HRG/Arg-1 complexed with NRP1, with average values of –21.2 and –19.6 kcal/mol, respectively (Figure ). The abovementioned findings give statistical information on the binding energies of kamolonol as a putative NRP1 inhibitor.
To examine kamolonol-NRP1 interactions and the contribution of essential residues inside the active site of NRP1, estimated ΔGbinding values were divided into individual amino acid participations (Figure a). Particularly, amino acids with energy contributions less than –0.5 kcal/mol were depicted in Figure a. Per-residue energy decomposition analysis demonstrated that TRP301, SER346, ILE415, and ASP320 interacted with kamolonol and HRG/Arg-1 (Figure a). For instance, ASP320 amino acid residue participated in the total binding energy (ΔGbinding) with values of –2.6 and –2.1 kcal/mol, for kamolonol- and HRG-NRP1 complexes, respectively (Figure a). The average structures for kamolonol and HRG/Arg-1 inside the NRP1 active site over the 200 ns MD simulations are also displayed in Figure b. Kamolonol and HRG/Arg-1 formed four and two hydrogen bonds, respectively, with the key residues of the active site of NRP1 protein throughout the 200 ns MD simulations.
Figure 5. (a) The energy contribution of the most essential amino acids to the total MM-GBSA binding energy and (b) 2D representations of the anticipated binding modes for (i) kamolonol (NPC146388) and (ii) HRG/Arg-1 with the NRP1 active site in accordance with the average structure of the 200 ns MD simulations.
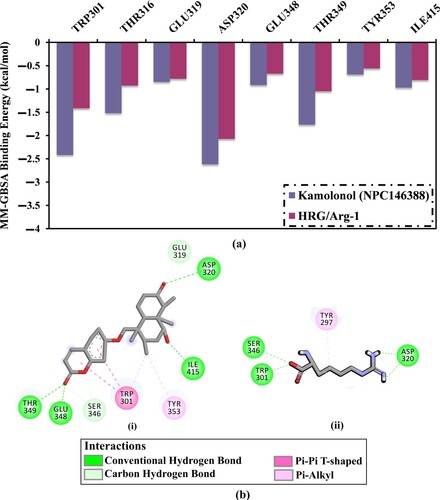
Inspecting the average structure for kamolonol-NRP1 complex showed that kamolonol demonstrated essential hydrogen bonds with key amino acid residues over the MD simulation. Notably, these interactions were absent in the initial predicted binding modes from docking computations. This demonstrates the importance of molecular dynamics simulations toward reliable results. Among these interactions are hydrogen bonds with ASP320, ILE415 and GLU348 (Figure b).
3.4. Post-dynamics analyses
3.4.1. Binding energy per-frame
To investigate the stability of kamolonol within the NRP1 active site, the correlation between the binding energy and simulation time was inspected (Figure ). As illustrated in Figure , kamolonol and HRG/Arg-1 were generally stable from 32 ns until the termination of the simulation, demonstrating binding energy equal to –34.5 and –32.0 kcal/mol, respectively. These study results elucidated the steadiness of the investigated complexes throughout the 200 ns MD simulations.
3.4.2. Hydrogen bond analysis
The hydrogen bonding of the ligands with the NRP1 protein was estimated throughout the MD course of 200 ns. The correlation between the number of hydrogen bonds and simulation time was inspected and presented in Figure S2. The most striking aspect of Figure S2 is that the average number of hydrogen bonds was 3 and 2 for the kamolonol and HRG/Arg-1. The current findings clearly supported the steady for the kamolonol and HRG/Arg-1.
3.4.3. Centre-of-mass distance
Centre-of-mass (CoM) distance was used to inspect the steadiness of the kamolonol and HRG/Arg-1 within NRP1. The CoM distance was measured between the kamolonol and HRG/Arg-1 and the ASP320 residue over the 200 ns MD simulations (Figure ). The CoM distance was steady for kamolonol and HRG/Arg-1 complexed with NRP1 at average distances of 10.7 and 8.6 Å, respectively. The presented results demonstrated the high stability of kamolonol and HRG/Arg-1 with NRP1.
3.4.4. Root-mean-square deviation
To examine the structural changes of NRP1 after complexation with kamolonol and HRG/Arg-1, the root-mean-square deviation (RMSD) analysis was measured (Figure ). The average RMSD values were 0.13 and 0.10 nm for the kamolonol- and HRG/Arg-1-NRP1 complexes, respectively, over the 200 ns MD simulations (Figure ). The RMSD analysis showed that the two complexes preserved their stability until the end of the simulations. These results confirmed that kamolonol and HRG/Arg-1 are tightly bonded and do not disturb the overall structural constancy of NRP1 besides keeping structural integrity.
3.5. Drug-likeness characteristics
The SwissADME website was utilized to predict the drug-likeness characteristics of kamolonol compared to HRG/Arg-1. For kamolonol the MLogP value was found to be less than five (calc. 3.0), indicating that this compound has high lipophilicity. The molecular weight of kamolonol was found to be less than 500 (calc. 398.49 g/mol). Additionally, the number of hydrogen bond acceptors (nON) was 5, and the number of hydrogen bond donors (nOHNH) was 1 (Figure ). The TPSA of the kamolonol was 76.74, which indicates that kamolonol has good bioavailability (Figure ). Finally, the calculated %ABS was 82.5%, explaining that kamolonol has good cell membrane permeability and oral bioavailability. Comparing the drug-likeness characteristics of kamolonol with those of HRG/Arg-1 demonstrated that kamolonol has better physiochemical properties and oral bioavailability than HRG/Arg-1.
3.6. ADMET characteristics
The pharmacokinetic properties of kamolonol were predicted and compared to HRG/Arg-1 utilizing the pkCSM online server. The pharmacokinetic properties included absorption, in which kamolonol demonstrated high Caco2 permeability (calc. 0.839) and high human intestinal absorption (HIA) (calc. 98.3), suggesting that kamolonol would be highly absorbed (Table ). In contrast to HRG/Arg-1, HRG/Arg-1 manifested poor Caco2 permeability and HIA with values of –0.351 and 24.6, respectively (Table ). To examine the drug distribution, the VDss value was expected with values of 0.351 and –0.438 for kamolonol and HRG/Arg-1, respectively (Table ). The metabolism results showed that kamolonol could work as an inhibitor for the CYP3A4 enzyme; however, HRG/Arg-1 was not an inhibitor for the CYP3A4 enzyme (Table ). The excretion was predicted based on the total clearance with values of 0.433 and 0.589 for kamolonol and HRG/Arg-1, respectively (Table ). According to hepatotoxicity, both kamolonol and HRG/Arg-1 were not toxic (Table ). The most striking observation to emerge from the data comparison was the superior ADMET features for kamolonol over HRG/Arg-1. These findings confirmed that the kamolonol could be used as a promising anticancer drug candidate.
Table 2. The expected ADMET properties for the kamolonol (NPC146388) and HRG/Arg-1.
3.7. Kamolonol vs. EG00229
EG00229, (S)−2-(3-(benzo[c][1,2,5]thiadiazole-4-sulfonamido)thiophene-2-carboxamido)−5-guanidinopentanoic acid, is an experimental inhibitor of NRP1 with IC50 value of 3 μM [Citation69]. To estimate the prospectivity of the identified NPASS compound, the binding score and mode of EG00229 towards NRP1 protein were evaluated and compared to kamolonol (NPC146388). Furthermore, MD simulations over 200 ns pursued by binding affinity estimations were performed for EG00229 complexed with NRP1 (Table and Figure S3). As depicted in Figure S3, the docking pose of EG00229 within the binding pocket of NRP1 was similar to the binding mode of kamolonol, exhibiting seven hydrogen bonds with ASP320 (2.54, 2.68 Å), SER346 (2.78, 2.82 Å), THR349 (1.91 Å), and ILE415 (2.96, 3.01 Å). Compared to kamolonol (docking score = −9.3 kcal/mol), EG00229 demonstrated a good docking score against NRP1 with a value of −6.1 kcal/mol (Table ).
Table 3. Predicted docking score (in kcal/mol) and MM/GBSA binding energies during the 200 ns MD course for kamolonol and EG00229 complexed with NRP1 protein.
To gain more reliable results, EG00229 in complex with NRP1 was subjected to 200 ns MD simulations, and the corresponding MM-GBSA binding affinity was computed (Table ). From Table , the average MM-GBSA binding energy (ΔGbinding) for EG00229 in complex with NRP1 was –28.7 kcal/mol, compared to –34.5 kcal/mol for the kamolonol-NRP1 complex. Similar to kamolonol, the binding affinity of EG00229 complexed with NRP1 protein was predominated by van der Waals (Evdw) with an average value of –30.0 kcal/mol (Table ).
4. Conclusion
In the current study, the NPASS database containing more than 35,000 natural products was virtually screened against the NRP1 protein for identifying potent inhibitors as anticancer drug candidates using the molecular docking technique. Based on docking computations, 67 compounds with expensive docking scores < –8.1 kcal/mol in complex with NRP1 were subjected to MD simulations. The computed MM-GBSA binding energy over an MD course of 200 ns demonstrated superior binding affinity of kamolonol (NPC146388) compared to the HRG/Arg-1 ligand with NRP1, demonstrating ΔGbinding values of –34.5 and –32.0 kcal/mol, respectively. During 200 ns MD simulations, energetic and structural analyses indicated perfect constancy for the kamolonol. Furthermore, kamolonol elucidated favorable physiochemical and pharmacokinetic properties compared to HRG/Arg-1. Finally, these results confirmed that the kamolonol could be used as a promising NRP1 inhibitor and hold promise for further experimental assays toward cancer treatment.
Supplemental Material
Download MS Word (11.8 MB)Acknowledgements
Ahmed M. Shawky would like to thank the Deanship of Scientific Research at Umm Al-Qura University for supporting this work by Grant Code: (22UQU4331174DSR43). The computational work was completed with resources supported by the Science and Technology Development Fund (STDF-Egypt, Grants No. 5480 and 7972), and Bibliotheca Alexandrina (http://hpc.bibalex.org). Dr. Mahmoud A. A. Ibrahim extends his appreciation to the Academy of Scientific Research and Technology (ASRT), Egypt, for funding Graduation Projects conducted at CompChem Lab, Egypt.
Disclosure statement
No potential conflict of interest was reported by the author(s).
Data availability statement
The data that support the findings of this study are available in the Supporting Information Material of this paper.
Additional information
Funding
References
- Szent-Gyoergyi A. Cell-division and cancer. Science. 1965;149:34–37. doi:10.1126/science.149.3679.34.
- Ma X, Yu H. Global burden of cancer. Yale J Biol Med. 2006;79:85–94.
- Mortality GBD. Causes of death, C. global, regional, and national life expectancy, all-cause mortality, and cause-specific mortality for 249 causes of death, 1980-2015: a systematic analysis for the Global Burden of Disease Study 2015. Lancet. 2016;388:1459–1544. doi:10.1016/S0140-6736(16)31012-1.
- Sung H, Ferlay J, Siegel RL, et al. Global cancer statistics 2020: GLOBOCAN estimates of incidence and mortality worldwide for 36 cancers in 185 countries. CA: Cancer J Clin. 2021;71:209–249. doi:10.3322/caac.21660.
- Pecorelli S, Benedet JL, Creasman WT, et al. FIGO staging of gynecologic cancer. Int J Gynaecol Obstet. 1999;64:5–10. doi:10.1016/s0020-7292(98)00234-3.
- Benjamin DJ, Xu A, Lythgoe MP, et al. Cancer drug approvals that displaced existing standard-of-care therapies, 2016-2021. JAMA Netw Open. 2022;5:e222265. doi:10.1001/jamanetworkopen.2022.2265.
- Longley DB, Johnston PG. Molecular mechanisms of drug resistance. J Pathol. 2005;205:275–292. doi:10.1002/path.1706.
- Shi F, Shang L, Yang LY, et al. Neuropilin-1 contributes to esophageal squamous cancer progression via promoting P65-dependent cell proliferation. Oncogene. 2018;37:935–943. doi:10.1038/onc.2017.399.
- Luo M, Hou L, Li J, et al. VEGF/NRP-1 axis promotes progression of breast cancer via enhancement of epithelial-mesenchymal transition and activation of NF-κB and β-catenin. Cancer Lett. 2016;373:1–11. doi:10.1016/j.canlet.2016.01.010.
- Hong TM, Chen YL, Wu YY, et al. Targeting neuropilin 1 as an antitumor strategy in lung cancer. Clin Cancer Res. 2007;13:4759–4768. doi:10.1158/1078-0432.CCR-07-0001.
- Li LH, Jiang X, Zhang Q, et al. Molecular targeted therapy for the treatment of gastric cancer. J Exp Clin Cancer Res. 2016;35:1–12. doi:10.1186/s13046-015-0276-9.
- Hang C, Yan HS, Gong C, et al. MicroRNA-9 inhibits gastric cancer cell proliferation and migration by targeting neuropilin–1. Exp Ther Med. 2019;18:2524–2530. doi:10.3892/etm.2019.7841.
- Zhou R, Curry JM, Roy LD, et al. A novel association of neuropilin-1 and MUC1 in pancreatic ductal adenocarcinoma: role in induction of VEGF signaling and angiogenesis. Oncogene. 2016;35:5608–5618. doi:10.1038/onc.2015.516.
- Hendricks C, Dubail J, Brohee L, et al. A novel physiological glycosaminoglycan-deficient splice variant of neuropilin-1 Is anti-tumorigenic In vitro and In vivo. PLoS One. 2016;11:e0165153. doi:10.1371/journal.pone.0165153.
- Al-Shareef H, Hiraoka SI, Tanaka N, et al. Use of NRP1, a novel biomarker, along with VEGF-C, VEGFR-3, CCR7 and SEMA3E, to predict lymph node metastasis in squamous cell carcinoma of the tongue. Oncol Rep. 2016;36:2444–2454. doi:10.3892/or.2016.5116.
- Jimenez-Hernandez LE, Vazquez-Santillan K, Castro-Oropeza R, et al. NRP1-positive lung cancer cells possess tumor-initiating properties. Oncol Rep. 2017;39:349–357. doi:10.3892/or.2017.6089.
- Soker S, Takashima S, Miao HQ, et al. Neuropilin-1 is expressed by endothelial and tumor cells as an isoform-specific receptor for vascular endothelial growth factor. Cell. 1998;92:735–745. doi:10.1016/S0092-8674(00)81402-6.
- Yoshida A, Shimizu A, Asano H, et al. VEGF-A/NRP1 stimulates GIPC1 and Syx complex formation to promote RhoA activation and proliferation in skin cancer cells. Biol Open. 2015;4:1063–1076. doi:10.1242/bio.010918.
- Sun L, Chen B, Jiang R, et al. Resveratrol inhibits lung cancer growth by suppressing M2-like polarization of tumor associated macrophages. Cell Immunol. 2017;311:86–93. doi:10.1016/j.cellimm.2016.11.002.
- Liu SD, Zhong LP, He J, et al. Targeting neuropilin-1 interactions is a promising anti-tumor strategy. Chin Med J. 2021;134:508–517. doi:10.1097/CM9.0000000000001200.
- Chuckran CA, Liu C, Bruno TC, et al. Neuropilin-1: a checkpoint target with unique implications for cancer immunology and immunotherapy. J Immunother Cancer. 2020;8:e000967. doi:10.1136/jitc-2020-000967.
- Harvey AL, Clark RL, Mackay SP, et al. Current strategies for drug discovery through natural products. Expert Opin Drug Discov. 2010;5:559–568. doi:10.1517/17460441.2010.488263.
- Harvey AL. Natural products in drug discovery. Drug Discov Today. 2008;13:894–901. doi:10.1016/j.drudis.2008.07.004.
- Patridge E, Gareiss P, Kinch MS, et al. An analysis of FDA-approved drugs: natural products and their derivatives. Drug Discov Today. 2016;21:204–207. doi:10.1016/j.drudis.2015.01.009.
- Zeng X, Zhang P, He W, et al. NPASS: natural product activity and species source database for natural product research, discovery and tool development. Nucleic Acids Res. 2018;46:D1217–D1222. doi:10.1093/nar/gkx1026.
- Mota F, Fotinou C, Rana RR, et al. Architecture and hydration of the arginine-binding site of neuropilin-1. FEBS J. 2018;285:1290–1304. doi:10.1111/febs.14405.
- Gordon JC, Myers JB, Folta T, et al. H++: a server for estimating pKas and adding missing hydrogens to macromolecules. Nucleic Acids Res. 2005;33:W368–W371. doi:10.1093/nar/gki464.
- Heller SR, McNaught A, Pletnev I, et al. Inchi, the IUPAC international chemical identifier. J Cheminformatics. 2015;7:23, doi:10.1186/s13321-015-0068-4.
- OMEGA. 2.5.1.4; openeye scientific software. Santa Fe, NM, USA, 2013.
- Hawkins PC, Skillman AG, Warren GL, et al. Conformer generation with OMEGA: algorithm and validation using high quality structures from the Protein Databank and Cambridge Structural Database. J Chem Inf Model. 2010;50:572–584. doi:10.1021/ci100031x.
- SZYBKI. 1.9.0.3, openeye scientific software. Santa Fe, NM, USA, 2016.
- Halgren TA. MMFF VI. MMFF VI. MMFF94s option for energy minimization studies. J Comput Chem. 1999;20:720–729. doi:10.1002/(SICI)1096-987X(199905)20:7<720::AID-JCC7>3.0.CO;2-X.
- QUACPAC. 1.7.0.2; openeye scientific software. Santa Fe, NM, USA, 2016.
- Gasteiger J, Marsili M. Iterative partial equalization of orbital electronegativity—a rapid access to atomic charges. Tetrahedron. 1980;36:3219–3228. doi:10.1016/0040-4020(80)80168-2.
- Trott O, Olson AJ. AutoDock Vina: improving the speed and accuracy of docking with a new scoring function, efficient optimization, and multithreading. J Comput Chem. 2009;31:455–461. doi:10.1002/jcc.21334.
- Morris GM, Huey R, Lindstrom W, et al. AutoDock4 and AutoDockTools4: Automated docking with selective receptor flexibility. J Comput Chem. 2009;30:2785–2791. doi:10.1002/jcc.21256.
- Cetin A. In silico studies on stilbenolignan analogues as SARS-CoV-2 Mpro inhibitors. Chem Phys Lett. 2021;771:138563, doi:10.1016/j.cplett.2021.138563.
- Cetin A, Bursal E, Türkan F. 2-methylindole analogs as cholinesterases and glutathione S-transferase inhibitors: Synthesis, biological evaluation, molecular docking, and pharmacokinetic studies. Arab J Chem. 2021;14:103449, doi:https://doi.org/10.1016/j.arabjc.2021.103449.
- Cetin A, Donmez A, Dalar A, et al. Tetra-substituted pyrazole analogues: synthesis, molecular docking, ADMET prediction, antioxidant and pancreatic lipase inhibitory activities. Med Chem Res. 2023;32:189–204. doi:10.1007/s00044-022-03005-7.
- Case DAB, Cerutti DS, Cheatham TE, et al. AMBER 2016. San Francisco, USA: University of California.
- Ibrahim MAA, Badr EAA, Abdelrahman AHM, et al. In Silico targeting human multidrug transporter ABCG2 in breast cancer: Database screening, molecular docking, and molecular dynamics study. Mol Inform. 2022;41:2060039. doi:10.1002/minf.202060039.
- Ibrahim MAA, Badr EAA, Abdelrahman AHM, et al. Prospective drug candidates as human multidrug transporter ABCG2 inhibitors: An in silico drug discovery study. Cell Biochem Biophys. 2021;79:189–200. doi:10.1007/s12013-021-00985-y.
- Ibrahim MAA, Abdelrahman AHM, Badr EAA, et al. Naturally occurring plant-based anticancerous candidates as prospective ABCG2 inhibitors: an in silico drug discovery study. Mol Divers. 2022: 3255–3277. doi:10.1007/s11030-022-10389-6.
- Ibrahim MAA, Abdeljawaad KAA, Abdelrahman AHM, et al. Non-β-Lactam allosteric inhibitors target methicillin-resistant staphylococcus aureus: An In silico drug discovery study. Antibiotics (Basel). 2021;10:934–956. doi:10.3390/antibiotics10080934.
- Ibrahim MAA, Abdelrahman AHM, Jaragh-Alhadad LA, et al. Exploring toxins for hunting SARS-CoV-2 main protease inhibitors: molecular docking, molecular dynamics, pharmacokinetic properties, and reactome study. Pharmaceuticals. 2022;15:153. doi:10.3390/ph15020153.
- Wang J, Wolf RM, Caldwell JW, et al. Development and testing of a general amber force field. J Comput Chem. 2004;25:1157–1174. doi:10.1002/jcc.20035.
- Maier JA, Martinez C, Kasavajhala K, et al. ff14SB: Ff14sb: improving the accuracy of protein side chain and backbone parameters from ff99SB. J Chem Theory Comput. 2015;11:3696–3713. doi:10.1021/acs.jctc.5b00255.
- Jakalian A, Jack DB, Bayly CI. Fast, efficient generation of high-quality atomic charges. AM1-BCC model: II. Parameterization and validation. Parameterization and Validation J Comput Chem. 2002;23:1623–1641. doi:10.1002/jcc.10128.
- Roux B, Simonson T. Implicit solvent models. Biophys Chem. 1999;78:1–20. doi:10.1016/S0301-4622(98)00226-9.
- Bayly CI, Cieplak P, Cornell WD, et al. A well-behaved electrostatic potential based method using charge restraints for deriving atomic charges: the RESP model. J Phys Chem. 1993;97:10269–10280. doi:10.1021/j100142a004.
- Frisch MJ, Trucks GW, Schlegel HB, et al. Gaussian 09, revision E01; Gaussian09, Gaussian Inc. Wallingford, CT, USA: pn; 2009.
- Jorgensen WL, Chandrasekhar J, Madura JD, et al. Comparison of simple potential functions for simulating liquid water. J Chem Phys. 1983;79:926–935. doi:10.1063/1.445869.
- Darden T, York D, Pedersen L. Particle mesh Ewald: AnN⋅log(N) method for Ewald sums in large systems. J Chem Phys. 1993;98:10089–10092. doi:10.1063/1.464397.
- Berendsen HJC, Postma JPM, Vangunsteren WF, et al. Molecular dynamics with coupling to an external bath. J Chem Phys. 1984;81:3684–3690. doi:10.1063/1.448118.
- Izaguirre JA, Catarello DP, Wozniak JM, et al. Langevin stabilization of molecular dynamics. J Chem Phys. 2001;114:2090–2098. doi:10.1063/1.1332996.
- Miyamoto S, Kollman PA. Settle: An analytical version of the SHAKE and RATTLE algorithm for rigid water models. J Comput Chem. 1992;13:952–962. doi:10.1002/jcc.540130805.
- Dassault Systèmes. BIOVIA, discovery studio visualize, version 2019; dassault systèmes: San Diego, CA, USA, 2019.
- Massova I, Kollman PA. Combined molecular mechanical and continuum solvent approach (MM-PBSA/GBSA) to predict ligand binding. Perspect Drug Discov. 2000;18:113–135. doi:10.1023/A:1008763014207.
- Onufriev A, Bashford D, Case DA. Exploring protein native states and large-scale conformational changes with a modified generalized born model. Proteins. 2004;55:383–394. doi:10.1002/prot.20033.
- Hou T, Wang J, Li Y, et al. Assessing the performance of the molecular mechanics/Poisson Boltzmann surface area and molecular mechanics/generalized Born surface area methods. II. The accuracy of ranking poses generated from docking. II. The accuracy of ranking poses generated from docking. J Comput Chem. 2011;32:866–877. doi:10.1002/jcc.21666.
- Wang E, Sun H, Wang J, et al. End-point binding free energy calculation with MM/PBSA and MM/GBSA: Strategies and applications in drug design. Chem Rev. 2019;119:9478–9508. doi:10.1021/acs.chemrev.9b00055.
- Daina A, Michielin O, Zoete V. SwissADME: a free web tool to evaluate pharmacokinetics, drug-likeness and medicinal chemistry friendliness of small molecules. Sci Rep. 2017;7:42717, doi:10.1038/srep42717.
- Pires DE, Blundell TL, Ascher DB. pkCSM: Pkcsm: predicting small-molecule pharmacokinetic and toxicity properties using graph-based signatures. J Med Chem. 2015;58:4066–4072. doi:10.1021/acs.jmedchem.5b00104.
- Kim MS, Oh KS, Lee JH, et al. Kamolonol suppresses angiotensin II-induced stress fiber formation and cellular hypertrophy through inhibition of Rho-associated kinase 2 activity. Biochem Biophys Res Commun. 2013;438:318–323. doi:10.1016/j.bbrc.2013.07.069.
- Dastan D, Salehi P, Reza Gohari A, et al. Disesquiterpene and sesquiterpene coumarins from Ferula pseudalliacea, and determination of their absolute configurations. Phytochemistry. 2012;78:170–178. doi:10.1016/j.phytochem.2012.02.016.
- Dixit D, Reddy CRK, Trivedi MH, et al. Non-targeted metabolomics approach to assess the brown marine macroalga Dictyota dichotoma as a functional food using liquid chromatography with mass spectrometry. Sep Sci Plus. 2020;3:140–149. doi:10.1002/sscp.201900109.
- Kerrigan JE. Molecular dynamics simulations in drug design. In: Kortagere S, editor. silico models for drug discovery. Totowa, NJ: Humana Press; 2013. p. 95–113.
- De Vivo M, Masetti M, Bottegoni G, et al. Role of molecular dynamics and related methods in drug discovery. J Med Chem. 2016;59:4035–4061. doi:10.1021/acs.jmedchem.5b01684.
- Jarvis A, Allerston CK, Jia H, et al. Small molecule inhibitors of the neuropilin-1 vascular endothelial growth factor A (VEGF-A) interaction. J Med Chem. 2010;53:2215–2226. doi:10.1021/jm901755g.