Abstract
Enhancing the ability of the WRF model in simulating a large area covering the West Pacific Ocean, mainland China, and the East Indian Ocean is very important to improve prediction of the East Asian monsoon climate. The objective of this study is to identify a reasonable configuration of physical parameterization schemes to simulate the precipitation and temperature in this large area. The Mellor–Yamada–Janjic (MYJ) and Yonsei University (YSU) PBL schemes, the WSM3 and WSM5 microphysics schemes, and the Betts–Miller–Janjic (BMJ) and Tiedtke cumulus schemes are compared through simulation of the regional climate of summer 2008. All cases exhibit a similar spatial distribution of temperature as observed, and the spatial correlation coefficients are all higher than 0.95. The cases combining MYJ, WSM3/WSM5, and BMJ have the smallest biases of temperature. The choice of PBL scheme has a significant effect on precipitation in such a large area. The cases with MYJ reproduce a better distribution of rain belts, while YSU strongly overestimates the precipitation intensity. The precipitation simulated using WSM3 is similar to that using WSM5. The BMJ cumulus scheme combined with the MYJ PBL scheme has a smaller bias of precipitation. However, the Tiedtke scheme reproduces the precipitation pattern better, especially over the ITCZ.
摘要
WRF模式对包含西太平洋、东印度洋和中国大陆的大区域气候模拟能力的提高对东亚夏季风气候的预测非常重要。本文的研究目的是找出合理的物理方案组合来模拟中国大陆、东印度洋和西太平洋这一特大区域的降水和温度。通过模拟2008年夏季气候并与观测进行对比分析,主要比较了Mellor–Yamada–Janjic (MYJ) 和Yonsei University (YSU) 边界层方案、WSM3和WSM5 微物理过程方案、Betts–Miller–Janjic (BMJ)和Tiedtke积云参数化方案对这一区域的气候模拟结果。研究表明:各种物理方案的组合对于温度空间分布的模拟与观测类似;边界层方案的选择对降水影响较大,MYJ方案比YSU方案对降水的模拟效果要好;积云方案Tiedtke对雨带分布,特别是赤道辐合带(ITCZ)降水模拟更加合理。
1. Introduction
The West Pacific Ocean and the East Indian Ocean have considerable effects on the East Asian monsoon climate, as the difference between the distribution of land and ocean generates the seasonal variation of heating to the atmosphere from land and ocean (Zhang and Li Citation2004). For East Asia in boreal summer, the substantial temperature difference between the large heat source over the East Asian continent and the strong cold source over the Pacific, east and south of the East Asian continent, is the main driving force for the formation of the East Asian summer monsoon. The Asian summer monsoon transports a large amount of water vapor from the tropical oceans to the monsoon area, which is fundamental to the formation of precipitation during the Asian summer monsoon period (Zhang and Li Citation2004). Therefore, tropical ocean thermal conditions can not only affect the intensity of the summer monsoon, but also the water vapor transport in the monsoon region—by influencing monsoon airflow. Subsequently, precipitation in the monsoon region is affected, leading to droughts and floods and other meteorological disasters (Zhang and Li Citation2004). During the East Asian summer monsoon, the monsoon circulation of the Indian summer monsoon can not only directly affect the airflow in East Asia, but also the large amount of water vapor transported from the Indian Ocean to the East Asian monsoon region, which is a major source there of summer precipitation. Therefore, it is important to simulate the climate over this large region encompassing the West Pacific Ocean and East Indian Ocean.
However, in regional climate simulation, different physical process schemes vary in their performance. Hu, John, and Zhang (Citation2010) examined the simulation ability of the WRF model over Texas in July–September 2005 when configured with three different PBL schemes: the Mellor–Yamada–Janjic (MYJ) scheme, the Yonsei University (YSU) scheme, and version 2 of the Asymmetric Convective Model (ACM2). The results showed that the WRF model underestimated temperature and overestimated moisture transport near the surface. The MYJ PBL scheme resulted in the largest bias because it simulated lower temperature and higher moisture than YSU and ACM2 in the boundary layer during daytime. The YSU PBL scheme simulated higher temperature and lower moisture than MYJ and ACM2 at nighttime.
De Silva et al. (Citation2010) reported that, when using the Betts–Miller–Janjic (BMJ) cumulus parameterization scheme in simulating precipitation over the tropical Kelani River basin in Sri Lanka, the results were not as good as when using the simplified Arakawa scheme; the BMJ scheme supplied insufficient moisture. Meanwhile, Ardie et al. (Citation2012) indicated that the BMJ scheme was capable of simulating the spatial features of three heavy rainfall episodes over the southern peninsular of Malaysia during the winter monsoon of 2006/2007. Wang et al. (Citation2011) reported that a simulation using the Tiedtke cumulus scheme yielded less precipitation for stratiform rainfall compared with TRMM observation.
Huang, Chen, and Zhu (Citation2011) demonstrated the superior performance of the WSM3 microphysics scheme in predicting the moderate and heavy rain of a torrential rainfall event related to the large-scale weather system in Xinjiang region during 25–26 May 2009. The WSM5 scheme also performed very well. Indeed, more recently, Zhu, Lin, and Cao (Citation2014) reported that WSM3 was the best scheme among eight (Lin, Kessler, WSM3, WSM5, and WSM6, Eta, WDM5, WDM6) for simulating the light to heavy rainfall and downpours of a heavy rainfall event that took place in southern China during 6–7 May 2010.
Other studies have also examined the performance of the WRF model configured with different parameterization schemes. Rafael et al. (Citation2008) used the WRF model with a combination of the YSU PBL scheme, WSM6 microphysics scheme, the Noah land-surface model, the Eta GFDL longwave radiation scheme, and the MM5 shortwave radiation scheme, to simulate two one-week-long periods in the Iberian Peninsula during winter and summer 2005. Based on a spatial resolution of 3 km, their results showed that the performance of this combination of schemes was better than others that they tested.
Despite these many experiments using different parameterization schemes to evaluate the performance of the WRF model, the simulation domains have generally been quite small. Little attention has been paid to evaluating the model’s performance over larger areas, such as that covering the West Pacific Ocean, mainland China, and the East Indian Ocean. In this context, the objective of the present study is to identify a reasonable configuration of parameterization schemes for simulating the precipitation and temperature in such a large area. The study focuses on the choice of PBL, microphysics and cumulus parameterization scheme through intercomparison of the MYJ and YSU, WSM3 and WSM5, and BMJ and Tiedtke schemes, respectively. Section 2 introduces the WRF model, the data and the design of the experiment in detail. Section 3 presents the results, and conclusions are drawn in Section 4.
2. Experiments and data
This study uses version 3.6 of the WRF model to simulate the summer temperature and precipitation in 2008. The domain includes mainland China, the West Pacific Ocean, and the East Indian Ocean. The resolution is 20 km × 20 km and the central position is (22°N, 115°E). The simulation is initiated at 0000 UTC (1800 CST) 1 May 2008. The initial and lateral boundary conditions are from the NCEP GFS FNL Operational Global Analyses data. The first month is used as the spin-up period, after which we only compare June, July, and August 2008. TRMM data at 0.5° × 0.5° resolution are used to compare with the modeled precipitation results. The CRU TS v. 3.21 of high-resolution gridded data of month-by-month variation in climate (January 1901–December 2012) and NCEP-2 data, both at 2.5° × 2.5° resolution, are used to compare with the modeled temperature results.
The Noah land-surface scheme (Chen and Dudhia Citation2001) and RRTM (Iacono et al. Citation2008) are used in all simulations. The MYJ (Janjic Citation2002) and YSU (Hong, Noh, and Dudhia Citation2006) PBL schemes, the WSM3 (Hong, Dudhia, and Chen Citation2004) and WSM5 (Hong, Dudhia, and Chen Citation2004) microphysics schemes, and the BMJ (Janjic Citation1994, Citation2000) and Tiedtke (Tiedtke Citation1989) cumulus parameterization schemes are compared.
MYJ is a PBL scheme of turbulent kinetic energy prediction, which is a local closure scheme, while YSU is a first-order non-local scheme. The YSU PBL scheme has stronger boundary layer top entrainment and boundary layer inner mixing than that of MYJ (Zhang et al. Citation2012). The WSM5 microphysics scheme allows supercooled water to exist, and a gradual melting of snow falling below the melting layer (William et al. Citation2008), whereas WSM3 does not. The Tiedtke cumulus scheme is a mass flux scheme. It includes three types of convection: penetrative convection, shallow convection, and midlevel convection (Wang et al. Citation2011). However, the BMJ cumulus scheme is a convective adjustment scheme. It describes the change in total moisture at each layer in the column but does not describe the vertical moisture flux or entrainment within the convective plume (Pérez et al. Citation2011).
The different combinations of schemes tested are shown in Table , and the following temperature and precipitation figures are the results from eight cases. By comparing these results and analyzing their statistical characteristics, including model bias, correlation, and RMSE, we aim to identify a reasonable set of parameterization schemes for the simulation of this large region by the WRF model.
Table 1. Schemes of the numerical experiments.
3. Results
This study covers three main regions: the West Pacific Ocean, mainland China and the East Indian Ocean. Figure shows the three-month (June–July–August, JJA) mean surface air temperature of the observation and eight cases. The eight cases almost simulate the same spatial distribution as the observation. The observation and eight cases all present low temperature over the Tibetan Plateau.
Figure 1. Three-month (JJA) mean surface air temperature (°C) of the observation and eight cases. (a) Observation (NCEP-2); (b) MYJ_WSM3_BMJ; (c) MYJ_WSM3_Tiedtke; (d) MYJ_WSM5_BMJ; (e) MYJ_WSM5_Tiedtke; (f) YSU_WSM3_BMJ; (g) YSU_WSM3_Tiedtke; (h) YSU_WSM5_BMJ; (i) YSU_WSM5_Tiedtke.
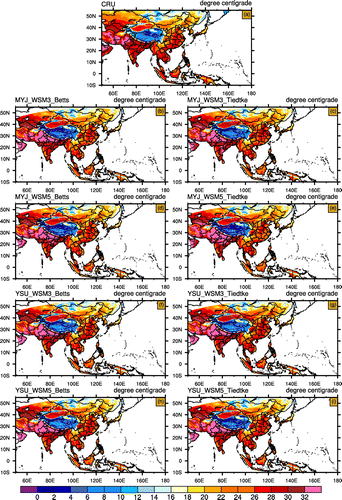
Figure shows the bias of mean surface air temperature of the eight cases. The eight cases all exhibit a considerable cold bias over the west of the Tibetan Plateau and a warm bias over northern China. However, the cases involving the MYJ PBL scheme present a colder than observed bias over southern China.
Figure 2. Bias of mean surface air temperature (°C) of the eight cases. (a) MYJ_WSM3_BMJ; (b) MYJ_WSM3_Tiedtke; (c) MYJ_WSM5_BMJ; (d) MYJ_WSM5_Tiedtke; (e) YSU_WSM3_BMJ; (f) YSU_WSM3_Tiedtke; (g) YSU_WSM5_BMJ; (h) YSU_WSM5_Tiedtke.
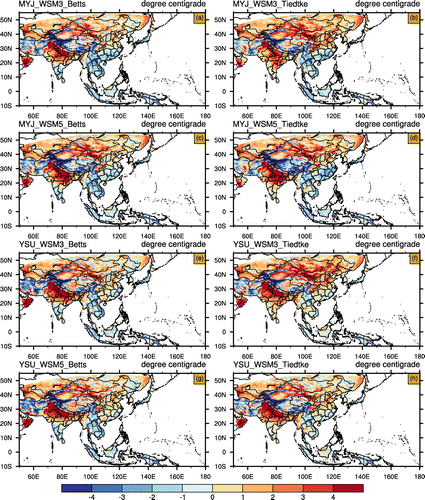
Figure shows the mean 850-hPa air temperature of the eight cases. The eight cases and the observation present progressively decreasing temperature at 850 hPa from south to north. However, over ocean, the cases involving the YSU PBL scheme yield better simulation results than those involving the MYJ PBL scheme.
Figure 3. Three-month (JJA) mean air temperature (°C) at 850 hPa of the observation and eight cases. (a) Observation (NCEP-2); (b) MYJ_WSM3_BMJ; (c) MYJ_WSM3_Tiedtke; (d) MYJ_WSM5_BMJ; (e) MYJ_WSM5_Tiedtke; (f) YSU_WSM3_BMJ; (g) YSU_WSM3_Tiedtke; (h) YSU_WSM5_BMJ; (i) YSU_WSM5_Tiedtke.
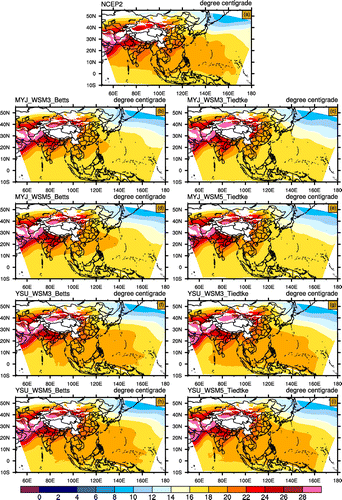
Figure shows the three main sub-regions and the three-month (JJA) mean precipitation of the observation and eight cases. Sub-region 1 is China, sub-region 2 is the East Indian Ocean, and sub-region 3 is the West Pacific Ocean. The TRMM data (Figure (b)) shows that there are some main precipitation zones; namely, an equatorial Pacific precipitation zone, a South China mainland precipitation zone, and a Northeast Indian Ocean precipitation zone. It is clear that the precipitation simulated by the WRF model when configured with the YSU PBL scheme (Figure (g) and (h)) is strongly overestimated compared to that derived from TRMM. This is because the YSU PBL scheme has stronger vertical mixing processes. The spatial distribution of precipitation simulated by the cases involving the MYJ PBL scheme is closer to the observation. For the cases with the two different microphysics schemes (WSM3 and WSM5), the results are almost the same. Meanwhile, the cases with the different cumulus schemes (BMJ and Tiedtke) simulate a different spatial distribution of precipitation. The MYJ_WSM3_BMJ (Figure (c)) and MYJ_WSM5_BMJ (Figure (e)) cases simulate the precipitation zone in the north of the East Indian Ocean and overestimate the precipitation in the West Pacific, and the situation is the reverse for MYJ_WSM3_Tiedtke (Figure (d)) and MYJ_WSM5_Tiedtke (Figure (f)). This may be attributable to the fact that the Tiedtke scheme underestimates stratiform rainfall, and the proportion of frontal precipitation reaches more than 50% in subtropical regions (Wang et al. Citation2011). The cases involving the MYJ PBL scheme reproduce the spatial distribution of the main rain belt in the region, but fail to reproduce the ITCZ rain belt.
Figure 4. Three-month (JJA) mean precipitation (mm d−1) of the observation and eight cases. (a) Sub-regions; (b) observation (TRMM); (c) MYJ_WSM3_BMJ; (d) MYJ_WSM3_Tiedtke; (e) MYJ_WSM5_BMJ; (f) MYJ_WSM5_Tiedtke; (g) YSU_WSM3_BMJ; (h) YSU_WSM3_Tiedtke; (i) YSU_WSM5_BMJ; (j) YSU_WSM5_Tiedtke.
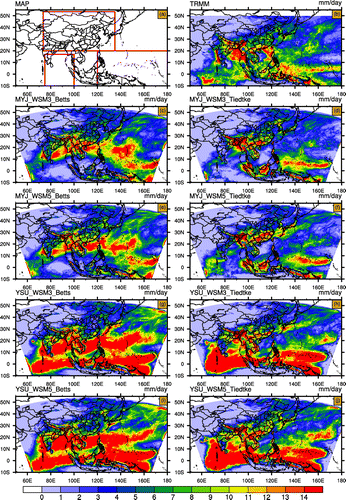
Table shows the bias, RMSE and spatial correlation coefficients of the temperature between the eight cases and observation. For surface air temperature, the statistics of the eight cases feature similar RMSE, and high correlation coefficients (<0.95). The bias of case MYJ_WSM3_BMJ is the smallest. For 850-hPa air temperature, the eight cases also feature high correlation coefficients (<0.95). The bias of case YSU_WSM5_Tiedtke is the smallest. The cases involving the YSU PBL scheme produce lower RMSE than those involving the MYJ PBL scheme.
Table 2. Statistical results of the eight cases.
Table shows the bias, RMSE, and spatial correlation coefficients of the precipitation between the eight cases and observation. It is clear that, for the whole region, the bias and RMSE of cases involving the YSU PBL scheme are much larger than those involving the MYJ PBL scheme. Among the four cases involving the MYJ PBL scheme, the MYJ_WSM5_BMJ case yields the smallest RMSE and bias (>0.1). Although the correlation coefficient of case MYJ_WSM5_BMJ is not the largest, the four cases involving the MYJ PBL scheme are very close. For the three sub-regions, the cases involving the MYJ PBL scheme feature similar correlation coefficients in sub-region 1 and sub-region 2. For sub region-3, cases MYJ_WSM3_Tiedtke and MYJ_WSM5_Tiedtke feature higher correlation coefficients than the other cases involving the MYJ PBL scheme.
Table 3. Statistical results of the eight cases.
4. Conclusion
This study evaluates the ability of the WRF model in simulating temperature and precipitation over a large region when configured with eight different combinations of physical schemes, including the MYJ and YSU PBL schemes, the WSM3 and WSM5 microphysics schemes, and the BMJ and Tiedtke cumulus parameterization schemes. The large region includes the West Pacific Ocean, mainland China, and the East Indian Ocean.
The spatial distributions of surface air temperature simulated by all cases are almost the same. The statistics indicate that they all have high correlation coefficients and similar RMSEs. However, the MYJ_WSM3_BMJ case produces the smallest bias.
The cases involving the YSU PBL scheme are better at simulating the 850-hPa air temperature pattern. The YSU_WSM5_Tiedtke case produces the smallest bias.
The choice of PBL and cumulus scheme has a significant effect on the precipitation simulation ability. The simulations involving the YSU PBL scheme simulate much more precipitation than observed. Meanwhile, the simulation abilities of the WRF model when configured with the two types of microphysics scheme (WSM3 and WSM5) are very close. The statistics indicate that the two cases of MYJ_WSM3_Tiedtke and MYJ_WSM5_Tiedtke produce higher correlation coefficients than other cases involving the MYJ PBL scheme.
In conclusion, from the statistical results, the surface and 850-hPa air temperature of the eight cases all feature considerably low biases, similar RMSEs, and high correlation coefficients. The cases of MYJ_WSM3_Tiedtke and MYJ_WSM5_Tiedtke are better than others in simulating the precipitation over this large area covering the East Indian Ocean, mainland China, and the West Pacific Ocean.
It is important to note that this work simulates the temperature and precipitation in summer 2008 only. It is necessary to simulate more years and more variables to further evaluate the performance of the WRF model with these parameterization schemes.
Disclosure statement
No potential conflict of interest was reported by the authors.
Funding
This work was funded by the National Natural Science Foundation of China [General Project, grant number 41275108]; the Strategic Priority Research Program of the Chinese Academy of Sciences [grant number XDA11010404].
Notes on contributors
Que Lin-Jing is a student of master’s degree at College of Global Change and Earth System Science (GCESS), Beijing Normal University. Her main research interest is climate simulation. Her recent publication is in Atmospheric and Oceanic Science Letters.
Que Wei-Lun works at Longjiang County Meteorological Bureau, Heilongjiang Meteorological Bureau. His main research interests are climate simulation and data analysis. His recent publication is in Atmospheric and Oceanic Science Letters.
Feng Jin-Ming is a professor at Key Laboratory of Regional Climate-Environment for East Asia (RCE-TEA), Chinese Academy of Sciences. His main research interests are climate simulation, the development of climate models, the regional impacts of urbanization and anthropogenic heat release on climate across China, meteorological statistical methods, data computation and analysis, image processing, and some programming languages. More than 60 scientific articles are published in journals of SCI and CSCD.
References
- Ardie, W. A., K. S. Sow, F. T. Tangang, A. G. Hussin, M. Mahmud, and L. Juneng. 2012. “The Performance of Different Cumulus Parameterization Schemes in Simulating the 2006/2007 Southern Peninsular Malaysia Heavy Rainfall Episodes.” Journal of Earth System Science 121 (2): 317–327.10.1007/s12040-012-0167-9
- Chen, F., and J. Dudhia. 2001. “Coupling an Advanced Land Surface-Hydrology Model with the Penn State-NCAR MM5 Modeling System. Part I: Model Implementation and Sensitivity.” Monthly Weather Review 129: 569–585.10.1175/1520-0493(2001)129<0569:CAALSH>2.0.CO;2
- De Silva, G. T., S. Herath, S. B. Weerakoon, and U. R. Rathnayake. 2010. “Application of WRF with Different Cumulus Parameterization Schemes for Precipitation Forecasting in a Tropical River Basin.” Proceedings of the 13th Asian Congress of Fluid Mechanics, December 17–21, 2010, Dhaka, Bangladesh, 513–516.
- Hong, S.-Y., J. Dudhia, and S.-H. Chen. 2004. “A Revised Approach to Ice Microphysical Processes for the Bulk Parameterization of Clouds and Precipitation.” Monthly Weather Review 132: 103–120.10.1175/1520-0493(2004)132<0103:ARATIM>2.0.CO;2
- Hong, S.-Y., Y. Noh, and J. Dudhia. 2006. “A New Vertical Diffusion Package with an Explicit Treatment of Entrainment Processes.” Monthly Weather Review 134: 2318–2341.10.1175/MWR3199.1
- Hu, X. M., W. N. G. John, and F. Q. Zhang. 2010. “Evaluation of Three Planetary Boundary Layer Schemes in the WRF Model.” Journal of Applied Meteorology & Climatology 49 (9): 1831–1844.
- Huang, H. B., C. Y. Chen, and W. N. Zhu. 2011. “Impact of Different Cloud Microphysical Process and Horizontal Resolutions of WRF Model on Precipitation Forecast Effect.” Meteorological Science and Technology 39 (5): 529–536.
- Iacono, M. J., J. S. Delamere, E. J. Mlawer, M. W. Shephard, S. A. Clough, and W. D. Collins. 2008. “Radiative Forcing by Long-Lived Greenhouse Gases: Calculations with the AER Radiative Transfer Models.” Journal of Geophysical Research 113: D13103. doi:10.1029/2008JD009944.
- Janjic, Z. I. 1994. “The Step-Mountain Eta Coordinate Model: Further Developments of the Convection, Viscous Sublayer and Turbulence Closure Schemes.” Monthly Weather Review 122: 927–945.10.1175/1520-0493(1994)122<0927:TSMECM>2.0.CO;2
- Janjic, Z. I. 2000. Comments on “Development and Evaluation of a Convection Scheme for Use in Climate Models.” Journal of the Atmospheric Sciences 57: 573–686.
- Janjic, Z. I. 2002. Nonsingular Implementation of the Mellor-Yamada Level 2.5 Scheme in the NCEP Meso model. Camp Springs, NCEP Office Note, 437, 61 pp.
- Pérez, C., K. Haustein, Z. Janjic, O. Jorba, N. Huneeus, J. M. Baldasano, T. Black, et al. 2011. “Atmospheric Dust Modeling from Meso to Global Scales with the Online NMMB/BSC-Dust Model – Part 1: Model Description, Annual Simulations and Evaluation.” Atmospheric Chemistry and Physics 11: 13001–13027.10.5194/acp-11-13001-2011
- Rafael, B., V. Alexandrov, J. Jose´ del Vas, J. Lumbreras, and E. Rodrı´guez. 2008. “A Comprehensive Sensitivity Analysis of the WRF Model for Air Quality Applications over the Iberian Peninsula.” Atmospheric Environment 42 (2008): 8560–8574.
- Tiedtke, M. 1989. “A Comprehensive Mass Flux Scheme for Cumulus Parameterization in Large-Scale Models.” Monthly Weather Review 117: 1779–1800.10.1175/1520-0493(1989)117<1779:ACMFSF>2.0.CO;2
- Wang, X. C., Q. Bao, and K. Liu, G. X. Wu, and Y. M. Liu. 2011. “Features of Rainfall and Latent Heating Structure Simulated by Two Convective Parameterization Schemes.” Science China Earth Sciences 54 (11): 1779–1788.10.1007/s11430-011-4282-2
- William C. S., J. B. Klemp, J. Dudhia, D. O. Gill, D. M. Barker, M. G. Duda, X. Y. Huang, W. Wang, and J. G. Powers. 2008. A Description of the Advanced Research WRF Version 3. Mesoscale and Microscale Meteorology Division, National Center for Atmospheric Research. Boulder, NCAR technical note, NCAR/TN–475+STR, June 2008.
- Zhang, R. H., and Q. Li. 2004. “Impact of Sea Temperature Variability of Tropical Oceans on East Asian Monsoon.” Meteorological Monthly 30 (12): 22–26.
- Zhang, B.-H., S.-H. Liu, H.-P. Liu, and Y.-Jun Ma. 2012. “The Effect of MYJ and YSU Schemes on the Simulation of Boundary Layer Meteorological Factors of WRF.” [In Chinese.] Chinese Journal of Geophysics 55 (7): 2239–2248.
- Zhu, G. L., W. T. Lin, and Y. H. Cao. 2014. “Numerical Simulation of a Rainstorm Event over South China by Using Various Cloud Microphysics Parameterization Schemes in WRF Model and Its Performance Analysis.” Chinese Journal of Atmospheric Sciences 38 (3): 513–523.