Abstract
Drought is one of the extreme events that can be caused by internal climate variability (ICV) and external forcing (EF). Here, the authors investigate the relative contributions of ICV and EF to meteorological drought changes in China using 40 members from the Community Earth System Model Large Ensemble (CESE_LE) project for historical simulations (in response to greenhouse gases and other EF) and future simulations under the RCP8.5 scenario. The authors use the Standardized Precipitation Index (SPI) to represent meteorological drought, and then define and analyze four drought parameters (frequency, severity, duration, and maximum duration) over eight regions of China. For historical periods, the ICV plays a dominant role in drought variation, while with global warming under the RCP8.5 scenario the EF becomes the prominent factor for drought characteristics. With the global warming signal, the effect of ICV varies with the drought parameters. This study suggests that the ICV should be taken into account when climate model simulations are used to investigate drought—in particular, for historical periods.
摘要
干旱受气候内部变率和外部强迫共同影响。本文利用地球系统模式CESM对历史时期和RCP8.5下的40个集合模拟的降水资料,并结合实际观测,研究了上述两因子对气象学干旱–标准化降水指数变化的贡献。通过对干旱频率、强度、持续时间、及最长持续时间的变化分析发现:在历史时期,气候内部变率对干旱变化起主要影响,而在未来(RCP8.5)情景下,外部强迫变得更为重要。本文建议,在利用模式模拟结果研究干旱变化时应考虑气候内部变率的影响。
1. Introduction
Drought is one of the natural disasters affecting agriculture, water resources, and society, and it also causes large economic losses over almost every region in every year. As an extreme climate phenomenon, drought reflects below-normal conditions of land surface hydrological variables such as precipitation, soil moisture, and stream flow, over a period ranging from several weeks to decades (e.g. Wang et al. Citation2009; Wang, Lettenmaier, and Sheffield Citation2011; Dai Citation2013). For example, the severe drought over southwestern China from fall 2009 to spring 2010 caused considerable damage to crops, as well as human casualties (Song, Yang, and Li Citation2011). With the increased global warming in the twenty-first century, global models have projected an increase in global mean precipitation and an accelerated hydrological cycle and intensified drought over many regions (e.g. Dai Citation2013). Studies have also shown that global drylands have expanded in the past several decades and will probably continue to expand throughout the twenty-first century (Feng and Fu Citation2013).
The Earth’s climate changes due to internal climate variability (ICV) and external forcing (EF) or both. The former is caused by the interaction of intrinsic dynamical processes within the earth system and is difficult to predict (e.g. Deser et al. Citation2012, 2014). External climate forcing factors include volcanic eruptions, solar variability, anthropogenic emissions of greenhouse gases and pollutants, and land use change, and can be directly observed or observationally constrained (e.g. Zeng and Geil Citation2016). On regional scales, ICV is a dominant factor for drought and precipitation changes, even though the EF signal is detectable in global-mean drought indices (Dai Citation2013; Dai and Zhao Citation2016; Zhao and Dai Citation2016). The ICV may be as important as anthropogenic climate change at regional scales in particular, at middle and high latitudes and for precipitation and other hydroclimate variables (Hawkins and Suttond Citation2009; Deser et al. Citation2012, 2014).
One way to quantify ICV is to produce a large number of climate change realizations through coupled climate system modeling with different initial conditions. The Community Earth System Model Large Ensemble (CESM_LE) project is one such project. It provides a set of 40-ensemble simulations for the period 1920–2100 using historical forcing and the RCP8.5 scenario (Kay et al. Citation2015). The CESM_LE products contain long-term and high-resolution meteorological and hydrological variables, which facilitate the investigation of the relative attributions of climate change due to ICV and EF, respectively. In the CESM_LE project, the differences across ensemble members are entirely caused by slightly different initial conditions, which lead to different evolutions of climate variability that can affect decadal precipitation over many land regions (e.g. Dong and Dai Citation2015). The ensemble mean (ENS) represents the model-simulated response to the EF, while the spread among ensemble members manifests the ICV effects (Kay et al. Citation2015). This is in contrast to the ensemble simulations from multiple climate models (e.g. Zhao and Dai Citation2016), which include both ICV and inter-model differences.
The goal of this study is to employ the CESM_LE-simulated precipitation to construct an index for meteorological drought and then to analyze regional drought characteristics and their changes contributed by ICV and EF, respectively. Two questions will be addressed: (1) What is the relative importance of ICV and EF in different drought parameters over different regions in China in the twentieth century? (2) How do they change with global warming in the twenty-first century? Section 2 briefly introduces the CESM_LE project, drought definition, and analysis method, while Section 3 discusses the changes in drought parameters due to both ICV and EF. A conclusion and discussion is presented in Section 4.
2. Data and method
The CESM_LE project uses CESM, version 1.1, which consists of the Community Atmosphere Model, version 5; the Community Land Model, version 4; the Parallel Ocean Program, version 2, and the Los Alamos Sea Ice Model, version 4 (Hurrell et al. Citation2013). The CESM-LE product has a horizontal resolution of 0.9° × 1.25° and is available for 1920–2100. Under the protocols of the Coupled Model Intercomparison Project, Phase 5 (CMIP5) (Taylor, Stouffer, and Meehl Citation2012), all ensemble simulations have the same specified historical EF for 1920-2005 and future scenario with RCP8.5 for 2006–2100. The ensemble member 1 was run directly from 1850 to 2100, while the ensemble members 2–40 were started on January 1920 using the ensemble 1 generated initial condition with slight perturbations (Kay et al. Citation2015). Differences among all CESM_LE members only come from the internal variability of the climate system since the influence of the initial condition would be demised only for couple weeks (e.g. Lorenz Citation1963; Deser et al. Citation2012, 2014). Assuming that the 40-member ensemble is large enough to account for most fluctuations in the atmospheric circulation induced by ICV, the ENS simulation from all CESM_LE members could be treated as the responses to EF only since the effect of ICV would be offset by different ensemble members in computing the ENS.
To evaluate the ability of CESM_LE to represent drought in China, we use a 0.5° × 0.5° gridded precipitation dataset (CN05.1; referred to as OBS) constructed from daily measurements of more than 2400 meteorological stations during 1961–2014 within mainland China (Wu and Gao Citation2013). The monthly OBS precipitation data are disaggregated into a 0.9° × 1.25° resolution using bilinear interpolation to match that of CESM_LE.
To focus on drought variations over large areas (rather than small spatial scale drought variations), a common approach is to divide mainland China into several regions (Wang, Lettenmaier, and Sheffield Citation2011; Ma et al. Citation2015; Li, Zhou, and Chen Citation2015). Following the climate division defined in Ma et al. (Citation2015), mainland China is divided into seven regions (Figure (a)): northwest (NW), northeast (NE), North Center (NC), Yangtze River valley (YZ), southeast (SE), southwest (SW), and Western Tibet (WT). Monthly precipitation from both CESM_LE simulations and OBS are regionally averaged before they are further analyzed. The following analyses are conducted over all eight regions (seven regions plus mainland China as a whole).
Figure 1. Annual precipitation for 1961–2005 from (a) observation (OBS); (b) the difference between simulations from the CESM-LE 40-member ensemble mean (ENS) and OBS; (c) the ENS difference between 2071–2100 and 1971–2000; and (d) the CESM_LE spread (in percentage terms), computed as the ratio of the standard deviation of annual precipitation differences between 2071–2100 and 1971–2000 across the 40 CESM-LE members to their ENS differences.
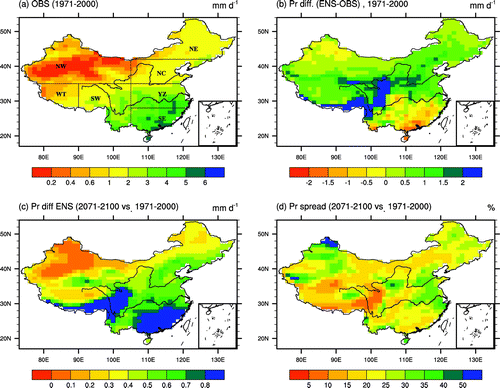
The Standardized Precipitation Index (SPI) is widely used to indicate abnormal dryness and wetness according to antecedent precipitation deficiency at different time scales, ranging from weeks to months (McKee, Doesken, and Kleist Citation1993). The original SPI was computed by fitting the precipitation in preceding months or weeks to a two-parameter Gamma distribution, and then transforming the time series into the standard normal distribution (McKee, Doesken, and Kleist Citation1993). For a specific region over a long-term computed period, the SPI value has zero mean and unity standard deviation, which facilitates the intercomparison of the dry/wet states over different climate regimes. Guttman (Citation1999) compared several different probability distributions and suggested that the three-parameter Pearson Type III (PE3) distribution is most appropriate to fit the precipitation sums. Therefore, the PE3 distribution is employed to compute SPI in this study.
Here, a drought event is defined as consecutive months with SPI below a critical value (−1.0) for a certain time scale (i.e. 3, 6, 12, and 24 months). Drought characteristics, including frequency, severity, and mean and maximum duration, are then computed for each CESM_LE member over each region. Drought frequency is the number of drought events divided by the total months during the study period. Drought duration is defined as the number of months for each drought event, while the maximum drought duration is the longest period of consecutive months under drought during the studied period. Drought severity is defined by the departure of the mean SPI from the critical value of −1.0 (i.e. −1.0 minus SPI) in each drought event, so that it is greater than zero and a higher value indicates a more severe drought. Wu et al. (Citation2005) found that the SPI value somehow depended on the data period used to derive parameters in the probability distribution. For a consistent comparison, the monthly sums of precipitation for the period 1971–2000 were used as a reference period to fit the PE3 distribution for both OBS and the CESM_LE simulations. We then computed the SPI for the period 1920–2100 from CESM_LE, and for the period 1961–2005 from OBS. The drought parameters were then computed from each ensemble member, and the ENS was defined as the ENS of drought parameters from 40 members. In this study, the three-month SPI was used to represent meteorological drought. Results from other SPI lengths were similar (figures not shown).
3. Results
3.1. Annual precipitation from OBS and CESM_LE
The observed annual mean precipitation averaged over China is approximately 1.71 mm d−1, varying from below 0.07 mm d−1 to over 5.76 mm d−1 over different regions for the reference period of 1971–2000 (Figure (a)), and the values from the CESM_LE 40-member set vary from 2.48 mm d−1 to 2.58 mm d−1 for the whole-China domain. Compared with OBS, the CESM_LE ENS (2.52 mm d−1) broadly captures the spatial pattern of annual mean precipitation (Figure (b)), but it substantially overestimates the average over China. The most substantial overestimation appears in the middle region between the Yellow River and Yangtze River valley basins, and over SW where ENS fails to reproduce the observed nocturnal precipitation in summer (Yu, Wang, and Zhou Citation2004). The OBS contains both ICV and EF effects, while the CESM_LE ENS only represents the effects of EF. Therefore, besides model deficiencies, the ICV may be responsible for part of above differences (Figure (b)).
The annual precipitation change of the CESM_LE 40-member ENS between 2071–2100 and 1971–2000 (Figure (c)) shows a clear regional pattern, with the largest differences over both SE and SW, and smallest differences over NW (where OBS precipitation is light). The magnitude differences roughly follow the spatial patterns of the annual mean (Figure (a)), with an exception over SW, where the differences are quite substantial in spite of the annual mean value being relatively small. To demonstrate the spread change of simulated precipitation among the CESM_LE 40 members between RCP8.5 and historical periods, we computed the percentage ratio of the standard deviation from 40-member annual precipitation differences between two periods to their ENS differences (Figure (d)). This ratio displays substantial regional variations over China, varying from 7.03% to 68.17%. This indicates the relative importance of ICV and EF on annual precipitation changes due to anthropogenic forcing. The greater the ratio, the more important the ICV.
3.2. Regional drought changes
Based on the three-month SPI derived from both CESM-LE and OBS precipitation, Figure , as an example, shows the mean drought parameters from OBS, CESM_LE, and its ENS for the period 1971–2000. Note that drought parameters for ENS are derived from the average of drought parameters from 40 ensemble members. The drought frequency varies from 3.5% to 10.9% from CESM_LE 40 members over different regions, while it only changes from 6.7% to 8.2% in ENS. Similarly, the drought duration from CESM_LE varies from 1.5 to 3.4 months over different regions, while its change is much smaller in ENS (Figure (c)). Figure also shows that the drought parameters from OBS are all located within the spread of the 40 CESM-LE members for different regions, indicating the representativeness of the CESM_LE simulations on observational drought characteristics in China. That is, the drought parameters derived from the 40 CESM_LE members capture the variability induced by both ICV and EF. It should be noted here that the drought is defined based on events, which is different from the ones with the month-based drought indices (e.g. Li, Zhou, and Chen Citation2015; Zhao and Dai Citation2016). Hence, occurrence of drought here is not as frequent as in the month-based ones.
Figure 2. Drought characteristics based on SPI-03 (<−1.0) derived from OBS (red triangles) CESM-LE members (CESM_LE; black dots) and the ensemble mean (ENS; blue circles) for 1971–2000: (a) frequency; (b) mean severity; (c) mean drought duration; and (d) maximum duration.
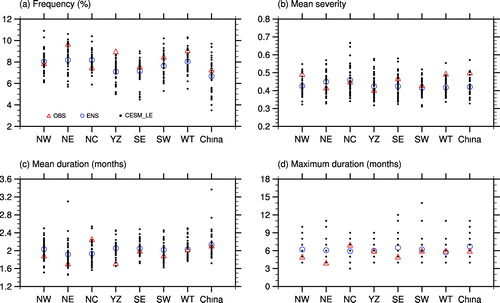
As discussed in Section 2, changes in drought parameters from ENS between two periods are primarily induced by EF, while the standard deviations of these changes across all CESM_LE members are mainly due to ICV. To qualify the relative role of EF and ICV in the temporal variation of drought, we define a signal-to-noise ratio (SNR) index as the absolute magnitude difference of the drought parameters from ENS between two periods divided by the standard deviation of their differences across 40 ensemble members of CESM_LE. A smaller SNR (less than 1) indicates a more dominant role of ICV, while a larger SNR (more than 1) indicates the greater importance of EF. A similar definition was used in Deser et al. (Citation2014) to quantify the effects of ICV and EF on precipitation and temperature trends due to global warming.
To illustrate the drought changes due to EF and ICV effects under global warming, we compare mean drought parameters for two periods, i.e. 1971–2000 and 2071–2100, representing the historical period and future period under the RCP8.5 scenario, respectively. To help understand these results, we also divide the historical period into two sub-periods: 1951–1980 and 1971–2000. Other periods are also analyzed, and the results are similar.
For the two historical periods (Figure (a)), the magnitude of the SNR varies slightly with regions and drought parameters, and the magnitudes of all 32 SNR values are less than 1, indicating the predominant effects of ICV on meteorological drought changes from one historical period to another. To gain a better understanding of these SNR values, Figure (a) shows the differences in ENS between the two periods and the standard derivation of differences across all ensemble members, corresponding to the numerator and denominator of the SNR, respectively. Note that the absolute value of the ENS differences is used in the numerator for the SNR computation.
Figure 3. Signal-to-noise ratio (SNR) for drought parameters derived from SPI-03 for (a) 1971–2000 vs. 1951–1980, and (b) 2071–2100 vs. 1971–2000.
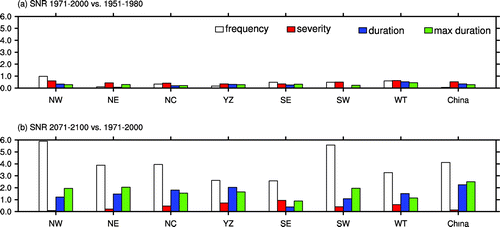
Figure 4. Mean drought parameter differences from the ensemble mean (ENS) and standard deviation (STD) of the differences from CESM_LE between 1971–2000 and 1951–1980, and between 2071–2100 and 1971–2000.
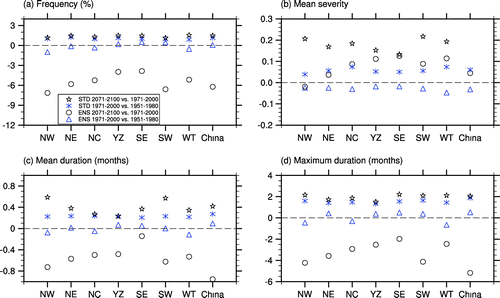
For a specific drought parameter, the ENS differences (i.e. EF effects) show regional variations and sign changes, while the standard derivations (i.e. ICV effects) change only slightly with regions, indicating the regional coherence of the ICV effect on drought characteristics. The sign and magnitude of ENS differences in Figure (a) also quantify the changes in drought parameters between two periods. The negative ENS differences denote a wetting tendency, while a positive value means a drying tendency between two periods. For instance, over NW, the drought threat was less for 1971–2000 with respect to 1951–1980, with all negative values in ENS differences. Over SE, three of the four parameters (except for drought severity) were enhanced, with positive values of ENS differences, while the mean severity was lessened slightly.
With these results for two historical periods, the question arises as to how the ICV, EF, and SNR will change in the future under the RCP8.5 scenario (atmospheric radiative forcing of 8.5 W m−2 in 2100 relative to the pre-industrial level), as induced by greenhouse gases, land cover and land use change, and air pollution emissions (van Vuuren et al. Citation2011). Over the seven regions in China, the increase in ENS temperature is approximately 4.48–5.95°C from 1971–2000 to 2071–2100, which is much larger than the temperature change from 1951–1980 to 1971–2000 (−0.01 to 0.31°C) (figure not shown).
Compared to the SNR between the two historical periods (Figure (a)), the magnitude of SNR values derived from 1971–2000 to 2071–2100 are much larger for all parameters over all regions (Figure (b)). Of the 32 SNR values in total, the magnitudes of 22 are greater than 1, and SE is the only region with an SNR magnitude less than 1 for three drought parameters. Over all eight regions, EF has dominant effects on the changes of three drought parameters (i.e. except for severity; Figure (a) and (b)), with most SNR values being greater than 1.0 between the historical and future periods.
Figure also shows that 25 of the 32 ENS differences of the four drought parameters between 1971–2000 and 2071–2100 are negative, and some have remarkably large magnitudes. The magnitude of ENS differences is also much larger than those between the two historical periods. The increase of EF in magnitude with the global warming (Figure ) leads to greater SNR values in magnitude (Figure ) in a warming world. This suggests that the meteorological drought (except for drought severity) based on precipitation only in China will be somehow lessened in the future under RCP8.5. The increase in precipitation in CMIP5 simulations over China has been reported under both the RCP4.5 and RCP8.5 scenarios (e.g. Chen Citation2013). However, studies have also shown that drought will be enhanced under the global warming in both China and global land areas when other variables (e.g. temperature, radiation) are accounted for in drought analyses (e.g. Wang, Lettenmaier, and Sheffield Citation2011; Dai et al. Citation2015).
For the standard deviations of the drought parameter differences between 1971–2000 and 2071–2100, the frequency and maximum duration are also of regional coherence, which are similar to those for the two historical periods (Figure ). In contrast, the standard deviation of differences of mean severity and mean duration are larger than those in the two historical periods over most regions, indicating a greater spread of the CEMS_LE members under the RCP8.5 scenario.
4. Conclusion and discussion
This paper presents meteorological drought analyses based on precipitation simulated by 40 CESM_LE members in both historical periods and future periods under the RCP8.5 scenario. The relative importance of the ICV and EF on SPI-03 (<−1.0) drought and its variations under global warming is investigated. In historical periods, the ICV plays a dominant role in meteorological drought change, while under the RCP8.5 scenario the EF is the more prominent factor for drought characteristics. Comparing the results in future and historical periods, the effect of ICV on drought varies with parameters, showing regional coherency (i.e. with similar ICV values over different regions and between historical and future periods) for frequency and maximum duration, but ICV increases for severity and mean duration with the global warming signal. Therefore, we suggest that ICV should be accounted for when climate model simulations are used to study drought—in particular, for historical periods.
There are some limitations in the current study. First, we use only the SPI to characterize regional meteorological drought, which does not consider other variables (e.g. air temperature, evapotranspiration) related to drought changes. The conclusions here might be affected when other drought indices (e.g. soil moisture, Palmer Drought Severity Index, etc.) are used. Since drought does not have a unified definition, the above analyses will be extended in the future using different drought indices and over different continents.
Furthermore, according to the SPI definition and classifications, SPI <−1.0 accounts for moderate, severe, and extreme drought events, and at a specific region for an individual month the accumulated occurrence probability is about 15.9% (McKee, Doesken, and Kleist Citation1993). This somehow restricts the maximum drought occurrence. Second, the CESM_LE project might not accurately account for all EF factors, and the 40-member ensemble might not be large enough to cover all possible ICVs. Deser et al. (Citation2012) indicated that the contributions of ICV to future climate uncertainties vary with climate variables, time period, season, and location. Hawkins and Suttond (Citation2009) also indicated that model uncertainty has greater importance than ICV for decadal scales at regional spatial scales (~2000 km). Third, the mechanisms underlying meteorological drought are not explored here. Previous studies have demonstrated that drought in China is mainly related to the phase change of atmosphere–ocean teleconnection through its impacts on the East Asian monsoon (Yu, Wang, and Zhou Citation2004; Ma Citation2007; Zhou et al. Citation2013). For example, Ma (Citation2007) found a strong connection between the annual dry/wet condition in North China and the Pacific Decadal Oscillation. Despite the above limitations, the method and analysis in this study provide some potential applications for using ensemble simulations to investigate drought variations.
Funding
The work of AW was supported by the Key Project of the Ministry of Science and Technology of China [grant number 2016YFA0602401]; the National Natural Science Foundation of China [grant number 41275110]; the work of X. Zeng was supported by the National Science Foundation [grant number AGS-0944101].
Disclosure statement
No potential conflict of interest was reported by the authors.
Acknowledgments
We thank the CESM Large Ensemble Community Project for the datasets, obtained from https://www.earthsystemgrid.org/. Dr. Pu SHAO is also thanked for helping to download the CESM_LE data.
References
- Chen, H. 2013. “Projected Change in Extreme Rainfall Events in China by the End of the 21st Century Using CMIP5 Models.” Chinese Science Bulletin 58: 1462–1472. doi:10.1007/s11434-012-5612-2.
- Dai, A. 2013. “Increasing Drought Under Global Warming in Observations and Models.” Nature Climate Change 3: 52–58. doi:10.1038/nclimate1633.
- Dai, A., and T. Zhao. 2016. “Uncertainties in Historical Changes and Future Projections of Drought. Estimates of Historical Drought Changes.”Climatic Change. doi:10.1007/s10584-016-1705-2.
- Dai, A., J. C. Fyfe, S.-P. Xie, and X. Dai. 2015. “Decadal Modulation of Global Surface Temperature by Internal Climate Variability.” Nature Climate Change 5: 555–559. doi:10.1038/NCLIMATE2605.
- Deser, C., A. S. Phillips, V. Bourdette, and H. Teng. 2012. “Uncertainty in Climate Change Projections: The Role of Internal Variability.” Climate Dynamics 38: 527–546. doi:10.1007/s00382-010-0977-x.
- Deser, C., A. S. Phillips, M. A. Alexander, and B. V. Smoliak. 2014. “Projecting North American Climate over the Next 50 Years: Uncertainty Due to Internal Variability.” Journal of Climate 27: 2271–2296. doi:10.1175/JCLI-D-13-00451.1.
- Dong, B., and A. Dai. 2015. “The Influence of the Inter-Decadal Pacific Oscillation on Temperature and Precipitation over the Globe.” Climate Dynamics 45: 2667–2681.10.1007/s00382-015-2500-x
- Feng, S., and Q. Fu. 2013. “Expansion of Global Drylands in a Warming World.” Atmospheric Chemistry and Physics 13: 10081–10094.10.5194/acp-13-10081-2013
- Guttman, N. B. 1999. “Accepting the Standardized Precipitation Index: A Calculation Algorithm.” JAWRA Journal of the American Water Resources Association 35 (2): 311–322.10.1111/jawr.1999.35.issue-2
- Hawkins, E., and R. Suttond. 2009. “The Potential to Narrow Uncertainty in Regional Climate Predictions.” Bulletin of the American Meteorological Society 90: 1095–2209.10.1175/2009BAMS2607.1
- Hurrell, J., et al. 2013. “The Community Earth System Model: A Framework for Collaborative Research.” Bulletin of the American Meteorological Society 94: 1339–1360. doi:10.1175/BAMSWDW12W00121.1.
- Kay, J. E., et al. 2015. “The Community Earth System Model (CESM) Large Ensemble Project: A Community Resource for Studying Climate Change in the Presence of Internal Climate Variability.” Bulletin of the American Meteorological Society 96: 1333–1349. doi:10.1175/BAMS-D-13-00255.1.
- Li, X., W. Zhou, and Y. Chen. 2015. “Assessment of Regional Drought Trend and Risk over China: A Drought Climate Division Perspective.” Journal of Climate 28: 7025–7037. doi:10.1175/JCLI-D-14-00403.1.
- Lorenz, E. N. 1963. “Deterministic Nonperiodic Flow.” Journal of the Atmospheric Sciences 20: 130–141.10.1175/1520-0469(1963)020<0130:DNF>2.0.CO;2
- Ma, Z. 2007. “The Interdecadal Trend and Shift of Dry/Wet over the Central Part of North China and Their Relationship to the Pacific Decadal Oscillation (PDO).” Chinese Science Bulletin 52: 2130–2139.10.1007/s11434-007-0284-z
- Ma, S., T. Zhou, A. Dai, and Z. Han. 2015. “Observed Changes in the Distributions of Daily Precipitation Frequency and Amount over China from 1960 to 2013.” Journal of Climate 28: 6960–6978. doi:10.1175/JCLI-D-15-0011.1.
- McKee, T. B., N. J. Doeskin, and J. Kleist. 1993. “The Relationship of Drought Frequency and Duration to Time Scales.” Preprint, Eighth Conf. on Applied Climatology, 179–184. Anaheim, CA: Amer. Meteor. Soc.
- Song, J., H. Yang, and C. Y. Li. 2011. “A Further Study of Causes of the Severe Drought in Yunnan Province during the 2009/2010 Winter (in Chinese).” Chinese Journal of Atmospheric Sciences 35: 1009–1019. doi:10.3878/j.issn.1006-9895.2011.06.02.
- Taylor, K. E., R. J. Stouffer, and G. A. Meehl. 2012. “An Overview of CMIP5 and the Experiment Design.” Bulletin of the American Meteorological Society 93: 485–498.10.1175/BAMS-D-11-00094.1
- van Vuuren, D., et al. 2011. “The Representative Concentration Pathways: An Overview.” Climatic Change 109: 5–31.10.1007/s10584-011-0148-z
- Wang, A., T. Bohn, P. Mahanama, R. Koster, and D. Lettenmaier. 2009. “Multimodel Ensemble Reconstruction of Drought over the Continental United States.” Journal of Climate 22: 2694–2712. doi:10.1175/2008JCLI2586.1.
- Wang, A., D. Lettenmaier, and J. Sheffield. 2011. “Soil Moisture Drought in China, 1950–2006.” Journal of Climate 24: 3257–3270. doi:10.1175/2011JCLI3733.1.
- Wu, J., and X. J. Gao. 2013. “A Gridded Daily Observation Dataset Over China Region and Comparison with the Other Datasets.” Chinese Journal of Geophysics 56 (4): 1102–1111. doi:10.6038/cjg20130406. (in Chinese)
- Wu, H., M. J. Hayes, D. A. Wilhite, and M. D. Svoboda. 2005. “The Effect of the Length of Record on the Standardized Precipitation Index Calculation.” International Journal of Climatology 25: 505–520.10.1002/(ISSN)1097-0088
- Yu, R., B. Wang, and T. Zhou. 2004. “Tropospheric Cooling and Summer Monsoon Weakening Trend Over East Asia.” Geophysical Research Letters 31: L22212. doi:10.1029/2004GL021270.
- Zeng, X., and K. Geil. 2016. “Global Warming Projection in the 21st Century Based on an Observational Data-Driven Model.” Geophysical Research Letters 43: 10947–10954. doi:10.1002/2016GL071035.
- Zhao, T., and A. Dai. 2016. “Uncertainties in Historical Changes and Future Projections of Drought. Part II: Model-Simulated Historical and Future Drought Changes.”Climatic Change. doi:10.1007/s10584-016-4281742-x.
- Zhou, T., F. Song, R. Lin, X. Chen, and X. Chen. 2013. “The 2012 North China Floods: Explaining an Extreme Rainfall Event in the Context of a Long-term Drying Tendency.” Bulletin of the American Meteorological Society 94 (9): S49–S51.