Abstract
Satellite remote sensing is very important to obtain a variety of cloud properties. However, the data quality from satellites varies with different satellite characteristics. From December 2015 to January 2016, ground-based air quality index (AQI) data showed severe haze events occurred successively in eastern China, particularly in the Beijing–Tianjin–Hebei region. During those days, a red alert (the most serious level), orange alert (the second-highest level), and yellow alert (the third-highest level) for haze, were issued in Beijing. Cloud detection from four sensors onboard the ‘A-Train’ satellite constellation were compared for two severe haze episodes, on 21 and 30 December 2015 respectively. Results showed that the MODIS sensor onboard the Aqua satellite misclassified aerosol as cloud, while the other three sensors–AIRS onboard Aqua, the cloud profiling radar onboard CloudSat, and CALIOP onboard CALIPSO–did not observe cloud over the same location. Through the high-AQI haze region in the CALIPSO and CloudSat orbit track, MODIS marked cloud close to the surface, while the MODIS true-color image and CALIOP observed an aerosol layer over the same location, suggesting MODIS falsely observed cloud there. Over the haze region in eastern China, MODIS observed 36% on average greater cloud fraction than AIRS, suggesting haze pollution induces a greater MODIS cloud amount.
摘要
云在天气、气候和地球能量平衡中非常重要。雾霾地区不同卫星云探测精度如何的研究较少。本论文详细比较了中国东部地区2015–2016年冬季2次严重雾霾天气时,“A-Train”星群上四个遥感器的云探测。结果显示,在雾霾区域,MODIS/Aqua真彩色图和CALIOP/CALIPSO清楚地观测到气溶胶层时,AIRS/Aqua、CALIOP和CPR/CloudSat没有观测到云,而MODIS则观测到云顶高度接近地面的云,表明MODIS将气溶胶误判为云。更多雾霾天气个例结果显示,在雾霾区域,MODIS云量比AIRS云量高13%–49%,平均高36%.
1. Introduction
Clouds play an important role in weather, climate, and the Earth’s energy balance. Clouds have a cooling effect through the enhancement of the planetary albedo and a heating effect through emitting longwave infrared radiation to the surface (Ramanathan et al. Citation1989; Liu, Shi, and Zhao Citation2007). The observed net cloud forcing is about four times the radiative forcing under a doubling of CO2 (Ramanathan et al. Citation1989). Thus, it is very important to observe and study cloud properties.
Satellite remote sensing is an important approach to obtain a variety of cloud properties because of its continuous temporal and spatial resolution. Nevertheless, discrepancies among satellite cloud datasets are apparent and these can be explained by differences in instruments, algorithms, and sampling (Kahn et al. Citation2014). The MODIS instrument onboard the Aqua and Terra satellites, launched respectively in 2002 and 1999, has 36 spectral bands covering a wide spectral range from 0.41 to 14.24 μm. The MODIS cloud mask algorithm uses a series of threshold tests applied to as many as 22 of the 36 bands to identify the presence of clouds in each pixel. Finally, it classifies each 1 × 1 km pixel as either ‘confidently clear’, ‘probably clear’, ‘probably cloudy’, or ‘cloudy’ (Ackerman et al. Citation1998; Platnick et al. Citation2003; King et al. Citation2013). The infrared/microwave sounder suite, AIRS/AMSU, located onboard Aqua, has also been used in cloud detection and climate studies (Aumann et al. Citation2003; Kahn et al. Citation2008, 2014). The AIRS/AMSU suite uses a cloud-clearing methodology to detect cloud (Susskind, Barnet, and Blaisdell Citation2003). The AIRS field-of-view (FOV) spatial resolution is 13.5 km at nadir, whereas that of AMSU is ~45 km at nadir and coaligned to a 3 × 3 array of AIRS FOVs. The cloud top and fraction properties are retrieved after completion of the cloud-clearing steps by comparing calculated and observed AIRS radiances in a set of channels sensitive to cloud amount and height (Kahn et al. Citation2014). A milestone for cloud and aerosol research was the launching of CloudSat (Stephens et al. Citation2002) and CALIPSO in 2006 (Winker et al. Citation2009), both carrying active sensors–a 94-GHz cloud profiling radar (CPR) onboard CloudSat and a two-wavelength (532 and 1064 nm) CALIOP lidar onboard CALIPSO. CALIOP detects aerosol and cloud vertically from laser backscatter intensity profiles. The cloud–aerosol discrimination algorithm is based on statistical differences in the various optical and physical properties of cloud and aerosol layers (Winker et al. Citation2009). The CPR cloud mask algorithm detects cloud vertically from the raw radar-measured return power, which is due primarily to microwave emissions by the radar components (Marchand et al. Citation2008). CloudSat and CALIOP generally provide more direct and easily interpreted vertical cloud detection than passive methods, and a priori assumptions of surface and atmospheric quantities are necessary to infer cloud properties from passive methods (Kahn et al. Citation2008).
Some studies have deduced that aerosol may be one of the reasons for the difference in cloud fraction between satellite and ground-based observations. Comparisons between satellite and surface observations have shown that AVHRR obtains an overly high cloud amount in the Mediterranean region due to urban and aerosol haze effects (Kästner, Bissolli, and Hoppner Citation2004). Several studies have shown that MODIS is weakly correlated with the ground-based cloud amount over the North China Plain and Taklimakan Desert and that this may be attributable to aerosol (Ma et al. Citation2014; Shang et al. Citation2014; Mao et al. Citation2015). In recent years, haze pollution has occurred more frequently in central and eastern China, especially in the North China Plain region, including Beijing–Tianjin–Hebei (Chen and Wang Citation2015; Wang et al. Citation2015; Wu, Ding, and Liu Citation2017). Therefore, it is important for cloud climatology to assess the accuracy of satellite cloud detection in haze pollution regions through combining active (CPR and CALIOP) and passive satellite sensors (like MODIS and AIRS). These sensors are flying onboard the ‘A-train’ constellation, in which only slight observational time differences (<70 s) enable near simultaneous and collocated cloud observations (Kahn et al. Citation2008).
In this paper, we present results from a case study that compared the cloud detection retrieved from MODIS, AIRS, CloudSat, and CALIPSO in eastern China. We assessed the impact of serious haze pollution on cloud detection from different satellites. The specific questions we set out to answer were: (1) Are there any errors in satellite cloud detection for serious haze events? (2) What is the impact of haze pollution on satellite cloud fraction?
2. Data and methods
The ground-based air quality index (AQI) was used to recognize a severe pollution day in eastern China. Hourly AQI and PM10 concentration data were obtained from the surface observations of the China National Environmental Monitoring Center (http://www.mep.gov.cn). The data-set included a total of 837 monitoring stations across the research region (Figure ). The air quality was classified as excellent, good, lightly polluted, moderately polluted, heavily polluted, and severely polluted when the AQI was ≤50, 51–100, 101–150, 151–200, 201–300, and ≥301, respectively. For haze episodes, the data from 12:00 to 14:00 China Standard Time (CST = UTC + 08), covering the overpass times of Aqua, CALIPSO, and CloudSat, were averaged to obtain daily mean data.
Figure 1. (a) Daily averages of AQI and PM10 concentration from 1 November 2015 to 31 January 2016 over the research region. The labeled dates are the two haze cases in this study. (b) Averaged AQI during 19–26 December 2015.
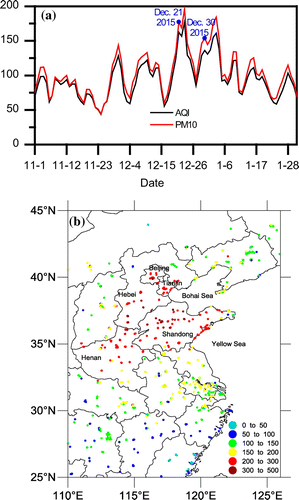
The cloud mask products of MODIS and AIRS onboard the Aqua satellite were used for intercomparison. Specifically, the MODIS collection 6 MYD35 cloud mask products (Ackerman et al. Citation2010) were used. The MODIS cloud mask was recorded at a 1-km (at nadir) spatial resolution. According to the cloud likelihood of a given pixel, it was labeled as ‘cloudy’, ‘uncertain – probably cloudy’, ‘probably clear’, or ‘confidently clear’. When calculating the cloud fraction, the first two conditions were regarded as cloudy, while the latter two were clear (Platnick et al. Citation2003). The cloud top pressure (CTP) and cloud top height (CTH) were also used. AIRS was the first hyperspectral infrared radiometer, with 2378 spectral channels covering 3.7–15.4 μm (Aumann et al. Citation2003). The Version 6 Level 2 AIRS products, including effective cloud fraction (ECF) and CTP, were used in this study. The ECF is the cloud fraction value if the cloud emissivity value is always 1 (Kahn et al. Citation2008, 2014). The spatial resolution of the AIRS FOV is 13.5 km at nadir and is coaligned to a 3 × 3 array of the AIRS FOV in each AMSU FOV. All 1-km MODIS cloud masks in each AIRS FOV were used to calculate one cloud fraction compared with AIRS.
The vertical cloud profiles from CloudSat (Stephens et al. Citation2002) and the cloud and aerosol profiles from CALIPSO (Winker et al. Citation2009) were used to compare with cloud detection from MODIS to AIRS. The Version R04 Level 2B CloudSat 2B-GEOPROF product was used. The confidence mask values in this product ranged from 0 to 40 (Kahn et al. Citation2008). The vertical resolution is 480 m and the horizontal resolution is approximately 1.4 km (cross-track) × 2.5 km (along-track) (Kahn et al. Citation2008). The latest Level 2 Version 4.10 CALIPSO product was used. The data have a 5-km horizontal resolution. The vertical resolution is 30 m below 8.2 km, 60 m within 8.2–20.2 km, and 180 m above 20.2 km (Winker et al. Citation2009). The cloud properties of MODIS and AIRS located in the CALIPSO and CloudSat tracks were then calculated to compare with CALIPSO and CloudSat.
3. Results and discussion
3.1. Haze episodes over eastern China
From 18 December 2015 to 16 January 2016, severe haze pollution occurred successively in eastern China, particularly in the Beijing–Tianjin–Hebei region. A red alert for haze (the most serious level) was issued in Beijing for the period from 07:00 CST 19 December to 24:00 CST 22 December 2015. Then, during 19 December 2015 to 16 January 2016, an orange alert (the second-highest level) and yellow alert (the third-highest level) for haze were published in eastern China. The daily AQI and PM10 (Figure (a)) averaged over the research region (Figure (b)) showed that the highest AQI and PM10 occurred on 23 December 2015, with an AQI value of 177 and PM10 concentration of 196 μg m−3.
Over eastern China, the severest haze episode lasted from 19 to 26 December 2015. The average AQI during this period is shown in Figure (b). AQI values showed that the haze pollution affected the whole of eastern China, particularly in the Beijing–Tianjin city region and southern Hebei, Shandong, and Henan provinces. In Beijing city, the air quality on seven consecutive days from 20 to 26 December 2015 remained at heavily or severely polluted levels, with a daily AQI ≥209. Similarly, the air quality on five consecutive days for Tianjin city (21–25 December 2015) and six consecutive days for Shijiazhuang (21–26 December 2015), the capital of Hebei Province, remained at heavily or severely polluted levels, with a daily AQI ≥259 for Tianjin and ≥228 for Shijiazhuang, respectively. The average AQI during 19–26 December 2015 at 213 monitoring stations was >200 (heavily polluted), and 45 sites were >300 (severely polluted). In Beijing, the average AQI at all 12 monitoring sites was >200.
Two cases, on 21 and 30 December 2015 respectively, when severe haze pollution occurred and, simultaneously, the orbit track of CloudSat and CALIPSO passed over the haze region, were selected to compare the cloud detection of MODIS, AIRS, CloudSat, and CALIPSO. The daily AQI on the two days was 162 and 136, respectively, ranking as the second and eighth highest level of pollution during the period from 1 November 2015 to 31 January 2016 (Figure (a)).
3.2. Cloud detection comparison during haze days
To compare different satellite sensors’ cloud detection on haze days, the cloud fields of four satellite sensors–MODIS, AIRS, CPR, and CALIOP–over the orbit track of CALIPSO and CloudSat on 21 and 30 December 2015 are shown in Figures and , respectively.
Figure 2. (a) MODIS Aqua true-color image on the haze day of 21 December 2015. (b) Air quality index at the satellite overpass time. The black line shows the orbit-track of CloudSat and CALIPSO on the same day. The lines (i) and (ii) show the agreement between the CPR onboard the CloudSat satellite and AIRS, and between CPR and MODIS, respectively. Blue coloring indicates agreement and red indicates disagreement. (c) Vertical cross-sections of the CloudSat cloud mask for the orbit shown in (b), with the AIRS cloud fraction (black circles) and cloud top height (centers of the circles) and MODIS cloud top height (red asterisks) also shown. (d) Vertical cross-sections of the CALIPSO cloud and aerosol mask for the orbit shown in (b).
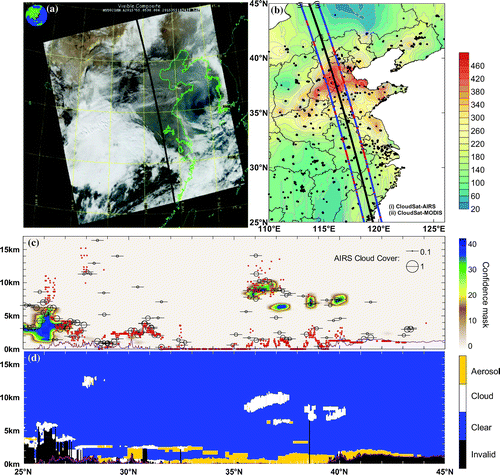
Figure 3. (a) MODIS Aqua true-color image on the haze day of 30 December 2015. (b) Air quality index at the satellite overpass time. The black line shows the orbit-track of CloudSat and CALIPSO on the same day. The lines (i) and (ii) show the agreement between CPR and AIRS, and between CPR and MODIS, respectively. Blue coloring indicates agreement and red indicates disagreement. (c) Vertical cross-sections of the CloudSat cloud mask for the orbit shown in (b), with the AIRS cloud fraction (black circles) and cloud top height (centers of the circles) and MODIS cloud top height (red asterisks) also shown. (d) Vertical cross-sections of the CALIPSO cloud and aerosol mask for the orbit shown in (b).
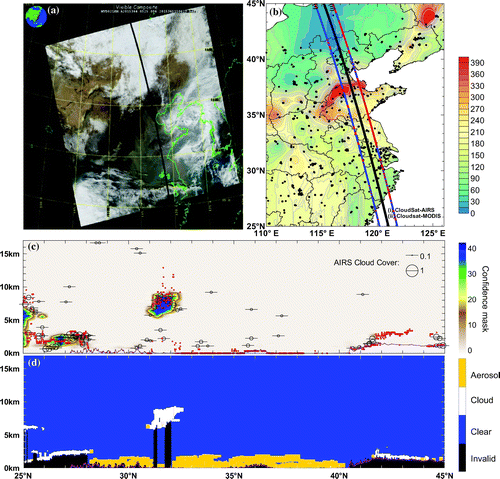
On 21 December 2015, a severe haze event occurred at the overpass time of the three satellites, i.e. Aqua, CALIPSO, and CloudSat. The MODIS true-color image (Figure (a)) showed that an aerosol layer overhung the center of eastern China and the Bohai–Yellow seas. The aerosol layer was consistent with high AQI values observed by surface monitoring sites (Figure (b)). The AQI was >300 over Beijing–Tianjin–Hebei and surrounding regions, meaning it was the region with the severest pollution level. Along the CloudSat and CALIPSO orbit track passing over the haze region, the four satellite sensors observed their cloud fields (Figure (c) and (d)). CPR and CALIOP revealed details on the vertical structure of clouds. The location and height of cloud resolved by CPR were highly consistent with CALIOP. The main difference was that CALIOP observed more cloud, e.g. the 2-km altitude cloud and >11-km-high cloud between 27.5°N and 28.5°N (Figure (d)), while CPR observed a weak confidence mask at about 2 km but not at 11 km.
The color of the lines showing agreement in Figure (b) indicates the level of accordance between the cloud detection of MODIS/AIRS and CPR, with blue for agreement (either cloudy or clear) and red for disagreement, respectively. The lines of agreement show that when compared to CPR and CALIOP cloud masks, the AIRS cloud field agreed better with them than MODIS. Kahn et al. (Citation2008) reported that the AIRS CTH agreed better with CPR when the cloud fraction was relatively large over South America, and similar results were obtained from the case on 21 December. The AIRS CTH was consistent with CPR and/or CALIOP when the cloud fraction was relatively large (Figure (c)). In the severe haze region around 31.6°–35.6°N and 37.6°–38.4°N, MODIS detected cloud with a CTH of 0 m and a CTP of >1015 hPa. That is distinctly false cloud according to the true-color image, ground surface AQI, and CALIOP aerosol vertical structure observations. Mao et al. (Citation2015) also analyzed an intermediate and serious aerosol loading cases in January 2009 and found that heavy aerosol loading during hazy days affected the capability of MODIS cloud detection. Along this track, AIRS did not observe cloud, which was consistent with CPR and CALIOP, suggesting AIRS did a better job than MODIS under high aerosol loading conditions.
The MODIS true-color image also showed high aerosol loading and less cloud over eastern China on 30 December 2015 (Figure (a)). High AQI values indicated a severe haze event occurred in eastern China, particularly in southern Hebei and Shandong provinces, where the AQI was >300–the most severe pollution level (Figure (b)). The cloud detection from AIRS, MODIS, CPR, and CALIOP along the orbit track of CloudSat and CALIPSO are compared in Figure (c) and (d). CPR and CALIOP observed similar vertical cloud structures along-track. Under the condition of a clean environment (south of 28°N and north of 40.5°N) or cloud located above the aerosol layer (31°–32°N), the AIRS and MODIS CTHs and cloud identification were to a certain extent consistent with CPR or CALIOP. Over the severe haze region between around 32.5°–39.5°N, CALIOP observed an aerosol layer at low levels (<3 km), and MODIS mistook aerosol for cloud because the CTH was close to the surface (0 m). Along the same track, AIRS observed no cloud in most locations and a small cloud fraction (<0.2) at several points. This is consistent with the case on 21 December 2015 in that AIRS did a better job in the haze region than MODIS, as clearly shown by the line of agreement in Figure (b).
3.3. Cloud fraction difference between MODIS and AIRS
To compare the impact of aerosol on cloud detection, the difference in cloud fraction between MODIS and AIRS was further investigated.
The AIRS cloud fraction and MODIS cloud mask on 21 and 30 December 2015 are shown in Figure . The spatial distribution of the AIRS cloud fraction (Figure (a) and (c)) was consistent with the MODIS true-color image (Figures (a) and (a)), and no cloud was observed by AIRS over the haze region. MODIS could identify extensive clouds well, e.g. the southwestern and southeastern region on 21 December (Figures (a) and (b)) and the southwestern and eastern region over the Bohai and Yellow seas and Korean peninsula on 30 December (Figures (a) and (d)). However, over many high aerosol regions on the two days (Figures (a) and (a)), the MODIS cloud mask observed ‘cloudy’ or ‘uncertain – probably cloudy’ results (Figure (b) and (d)).
Figure 4. AIRS cloud fraction on (a) 21 December 2015 and (c) 30 December 2015; MODIS cloud mask on (b) 21 December 2015 and (d) 30 December 2015.
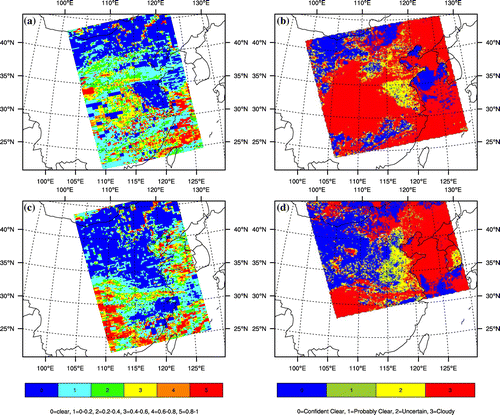
To quantify the difference in cloud fraction between MODIS and AIRS over the haze region of (30°–40°N, 110°–120°E), another six haze cases (26 and 28 December 2015; 6, 8, 15, and 27 January 2016) were also considered. The cloud fractions are shown in Table . The results showed that MODIS observed a 13%–49% (average: 36%) greater cloud fraction than AIRS, suggesting a large difference in cloud fraction between these two instruments.
Table 1. Comparisons of cloud fraction between MODIS and AIRS over the region (30°–40°N, 110°–120°E).
4. Conclusion
In this study, we compared the MODIS and AIRS cloud mask onboard the Aqua satellite and the cloud vertical products from the CALIPSO and CloudSat satellites over eastern China on two severe haze pollution days (21 and 30 December 2015). Compared with MODIS, AIRS, CALIPSO and CloudSat showed better cloud detection over severe haze pollution regions, while MODIS may have led to the misclassification of high aerosol as cloud, suggesting that high pollution may induce a greater MODIS cloud amount. In fact, MODIS observed a 13%–49% (average: 36%) greater cloud fraction than AIRS in eastern China for eight haze cases in December 2015 and January 2016. Although only two cases are reported in detail in this paper, they were representative of very severe haze pollution in eastern China and the results still have implications for satellite cloud detection. In future, more cases will be discussed to compare the differences among these satellites.
Disclosure statement
No potential conflict of interest was reported by the authors.
Funding
This work was supported by the National Natural Science Foundation of China [grant number 41590874] and [grant number 41590875].
Acknowledgments
The MODIS cloud and aerosol properties were provided by the Level 1 and Atmosphere Archive and Distribution System of the NASA Goddard Space Flight Center (http://ladsweb.nascom.nasa.gov/). We thank the NASA Goddard Earth Science Data and Information Services Center for providing the AIRS cloud products (http://daac.gsfc.nasa.gov/), the CloudSat Data Processing Center (http://www.cloudsat.cira.colostate.edu/) for providing the vertical profiles of cloud detection, and the NASA Langley Research Center Atmospheric Science Data Center (http://eosweb.larc.nasa.gov/) for providing the vertical profiles of the CALIPSO cloud and aerosol mask.
References
- Ackerman, S. A., K. I. Strabala, W. P. Menzel, R. A. Frey, C. C. Moeller, and L. E. Gumley. 1998. “Discriminating Clear Sky from Clouds with MODIS.” Journal of Geophysical Research: Atmospheres 103: 32141–32157. doi:10.1029/1998JD200032.
- Ackerman, S. A., R. Frey, K. I. Strabala, Y. Liu, L. Gumley, B. A. Baum, and W. P. Menzel. 2010. Discriminating Clear-sky from Cloud with MODIS: Algorithm Theoretical Basis Document (MOD35), Version 6.1, Madison: Cooperative Institute for Meteorological Satellite Studies, University of Wisconsin-Madison.
- Aumann, H. H., M. T. Chahine, C. Gautier, M. D. Goldberg, E. Kalnay, L. M. McMillin, H. Revercomb, et al. 2003. “AIRS/AMSU/HSB on the Aqua Mission: Design, Science Objectives, Data Products, and Processing Systems.” IEEE Transactions on Geoscience and Remote Sensing 41: 253–264. doi:10.1109/TGRS.2002.808356.
- Chen, H., and H. Wang. 2015. “Haze Days in North China and the Associated Atmospheric Circulations Based on Daily Visibility Data from 1960 to 2012.” Journal of Geophysical Research: Atmospheres 120: 5895–5909. doi:10.1002/2015JD023225.
- Kahn, B. H., M. T. Chahine, G. L. Stephens, G. G. Mace, R. T. Marchand, Z. Wang, C. D. Barnet, et al. 2008. “Cloud Type Comparisons of AIRS, CloudSat, and CALIPSO Cloud Height and Amount.” Atmospheric Chemistry and Physics 8: 1231–1248. doi:10.5194/acp-8-1231-2008.
- Kahn, B. H., F. W. Irion, V. T. Dang, E. M. Manning, S. L. Nasiri, C. M. Naud, J. M. Blaisdell, et al. 2014. “The Atmospheric Infrared Sounder Version 6 Cloud Products.” Atmospheric Chemistry and Physics 14: 399–426. doi:10.5194/acp-14-399-2014.
- Kästner, M., P. Bissolli, and K. Hoppner. 2004. “Comparison of a Satellite Based Alpine Cloud Climatology with Observations of Synoptic Stations.” Meteorologische Zeitschrift 13: 233–243. doi:10.1127/0941-2948/2004/0013-0233.
- King, M. D., S. Platnick, W. P. Menzel, S. A. Ackerman, and P. A. Hubanks. 2013. “Spatial and Temporal Distribution of Clouds Observed by MODIS Onboard the Terra and Aqua Satellites.” IEEE Transactions on Geoscience and Remote Sensing 51: 3826–3852. doi:10.1109/TGRS.2012.2227333.
- Liu, Y.-Z., G.-Y. Shi, and J.-Q. Zhao. 2007. “A Study of the Radiative Forcing of Clouds by Using a One-dimensional Radiative-convective Model.” Chinese Journal of Atmospheric Sciences 31: 486–494. (in Chinese).
- Ma, J., H. Wu, C. Wang, X. Zhang, Z. Li, and X. Wang. 2014. “Multiyear Satellite and Surface Observations of Cloud Fraction over China.” Journal of Geophysical Research: Atmospheres 119: 7655–7666. doi:10.1002/2013JD021413.
- Mao, F., M. Duan, Q. Min, W. Gong, Z. Pan, and G. Liu. 2015. “Investigating the Impact of Haze on MODIS Cloud Detection.” Journal of Geophysical Research: Atmospheres 120: 12237–212247. doi:10.1002/2015JD023555.
- Marchand, R., G. G. Mace, T. Ackerman, and G. Stephens. 2008. “Hydrometeor Detection Using Cloudsat – An Earth-orbiting 94-GHz Cloud Radar.” Journal of Atmospheric and Oceanic Technology 25: 519–533. doi:10.1175/2007jtecha1006.1.
- Platnick, S., M. D. King, S. A. Ackerman, W. P. Menzel, B. A. Baum, J. C. Riedi, and R. A. Frey. 2003. “The MODIS Cloud Products: Algorithms and Examples from Terra.” IEEE Transactions on Geoscience and Remote Sensing 41: 459–473. doi:10.1109/TGRS.2002.808301.
- Ramanathan, V., R. D. Cess, E. F. Harrison, P. Minnis, B. R. Barkstrom, E. Ahmad, and D. Hartmann. 1989. “Cloud-Radiative Forcing and Climate: Results from the Earth Radiation Budget Experiment.” Science 243: 57–63. doi:10.1126/science.243.4887.57.
- Shang, H., L. Chen, J. Tao, L. Su, and S. Jia. 2014. “Synergetic Use of MODIS Cloud Parameters for Distinguishing High Aerosol Loadings from Clouds over the North China Plain.” IEEE Journal of Selected Topics in Applied Earth Observations and Remote Sensing 7: 4879–4886. doi:10.1109/JSTARS.2014.2332427.
- Stephens, G. L., D. G. Vane, R. J. Boain, G. G. Mace, K. Sassen, Z. Wang, A. J. Illingworth, et al. 2002. “The Cloudsat Mission and the A-train.” Bulletin of the American Meteorological Society 83: 1771–1790. doi:10.1175/bams-83-12-1771.
- Susskind, J., C. D. Barnet, and J. M. Blaisdell. 2003. “Retrieval of Atmospheric and Surface Parameters from AIRS/AMSU/HSB Data in the Presence of Clouds.” IEEE Transactions on Geoscience and Remote Sensing 41: 390–409. doi:10.1109/TGRS.2002.808236.
- Wang, H., M. Xue, X. Y. Zhang, H. L. Liu, C. H. Zhou, S.-C. Tan, H. Z. Che, B. Chen, and T. Li. 2015. “Mesoscale Modeling Study of the Interactions between Aerosols and PBL Meteorology during a Haze Episode in Jing–Jin–Ji (China) and Its Nearby Surrounding Region – Part 1: Aerosol Distributions and Meteorological Features.” Atmospheric Chemistry and Physics 15: 3257–3275. doi:10.5194/acp-15-3257-2015.
- Winker, D. M., M. A. Vaughan, A. Omar, Y. Hu, K. A. Powell, Z. Liu, W. H. Hunt, and S. A. Young. 2009. “Overview of the CALIPSO Mission and CALIOP Data Processing Algorithms.” Journal of Atmospheric and Oceanic Technology 26: 2310–2323. doi:10.1175/2009JTECHA1281.1.
- Wu, P., Y. Ding, and Y. Liu. 2017. “Atmospheric Circulation and Dynamic Mechanism for Persistent Haze Events in the Beijing–Tianjin–Hebei Region.” Advances in Atmospheric Sciences 34: 429–440.10.1007/s00376-016-6158-z