Abstract
The authors examine the spatial and temporal characteristics of the simulated Pacific Decadal Oscillation (PDO) in 109 historical (i.e. all forcings) simulations derived from 25 coupled models within CMIP5. Compared with observations, most simulations successfully simulate the observed PDO pattern and its teleconnections to the SSTs in the tropical and southern Pacific. BNU-ESM, CanESM2, CCSM4, CESM1-FASTCHEM, FGOALS-g2, GFDL CM3, MIROC5, and NorESM1-M show better performance. Compared with the temporal phases of the observed PDO in the twentieth century, only five simulations — from CNRM-CM5, CSIRO Mk3.6.0, HadCM3, and IPSL-CM5A-LR — simulate an evolution of the PDO similar to that derived from observation, which suggests that current coupled models can barely reproduce the observed phase shifting of the PDO. To capture characteristics of the observed PDO in the twentieth century, a requirement is that all the relevant external forcings are included in the models. How to add realistic oceanic initial states into the model may be another key point.
摘要
太平洋年代际振荡(PDO)是影响亚洲—太平洋气候,乃至全球气候变化非常重要的海洋模态之一。目前为止,有关PDO形成的动力根源及机制仍不清楚。本文聚焦20世纪PDO的空间模态和时间序列演变,系统分析了CMIP5框架下25个耦合模式开展的109个历史时期模拟试验的结果。结果显示,大多数耦合模式可以成功模拟出20世纪PDO“马蹄形”海表温度距平,其中BNU-ESM,CanESM2,CCSM4,CESM1-FASTCHEM,GFDL CM3,MIROC5,NorESM1-M等模式和中国科学院大气物理研究所的FGOALS-g2模拟的PDO太平洋海表温度遥相关型空间分布与观测相似度较高;耦合模式模拟的PDO时间序列与观测相差较大,表明现有的耦合模式几乎不能模拟出20世纪PDO的位相演变特征。
1. Introduction
The Pacific Decadal Oscillation (PDO) is one of the most important modes of decadal climate variability (Mantua et al. Citation1997; Mantua and Hare Citation2002), whose index is defined as the leading principal component of SST anomalies in the Pacific basin, poleward of 20°N. Based on the last 100 years of observations, the spatial pattern of the PDO shows a characteristic ‘horseshoe’ shape in the North Pacific. During a positive PDO phase, anomalously cool SSTs are observed in the Kuroshio–Oyashio extension and central North Pacific, surrounded by anomalously warm SSTs along the west coast of the Americas that extend towards the tropics (Figure , observation). The spatial pattern is reversed during a negative PDO phase.
Figure 1. Observed and simulated regression distributions of SST on the PDO index (units: °C).
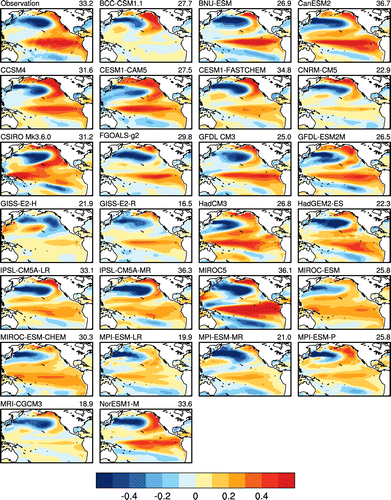
Previous studies indicate that the PDO has significant influences on regional, or even global climate. Fox example, its regime shifts have been associated with long-term fluctuations of the East Asian summer monsoon (e.g. Yu et al. Citation2015; Dong and Xue Citation2016), dry/wet conditions in North China (e.g. Wang et al. Citation2013; Qian and Zhou Citation2014; Zhu et al. Citation2015), Australian rainfall (Arblaster, Meehl, and Moore Citation2002; Cai and van Rensch Citation2012), and climate changes over the North Pacific and its coasts (Mantua and Hare Citation2002; Deser, Phillips, and Hurrell Citation2004). Additionally, the recent global-warming hiatus has also been partly attributed to the negative PDO-like SSTs in the early years of the twenty-first century (Kosaka and Xie Citation2013).
Despite its importance, the root cause of the PDO is still unclear. Local air–sea interactions in the North Pacific are suggested as a crucial process for the PDO. An anomalous Aleutian low and anomalous advection in the Kuroshio–Oyashio extension and the subtropical-gyre have also been invoked to explain the PDO (Latif and Barnett Citation1994; Schneider and Cornuelle Citation2005; Sun and Wang Citation2006). However, Deser, Phillips, and Hurrell (Citation2004) suggested that the tropics should play a key role in North Pacific interdecadal climate variability. At the same time, the remote influence from the Atlantic Multidecadal Oscillation cannot be excluded (Zhang and Delworth Citation2007). Therefore, this issue is so complicated that the mechanisms responsible for the PDO are open disputed.
In the twentieth century, the PDO experienced four regime shifts, in roughly 1925, 1946, 1977, and 1999 (http://research.jisao.washington.edu/pdo/). Although, as an internal variability, the PDO and its regime shifts are most likely influenced by external forcings (e.g. solar irradiance, volcanoes, anthropogenic greenhouse gases, and aerosols) (Wang et al. Citation2012; Dong, Zhou, and Chen Citation2014). Therefore, all relevant external forcings are needed for a model to obtain the correct timing of the simulated PDO in the twentieth century. There is a large amount of historical simulations within CMIP5. Both the natural and anthropogenic forcings covering the period 1850–2005 have been added into the models in these simulations. A more recent study indicated that most coupled models in CMIP5 have good capacity to simulate the climate mean state of the Asian-Pacific climate (Zhou Citation2016). Nevertheless, the skill of these coupled models in modelling the PDO remains unclear. In the present study, therefore, we use CMIP5 historical simulations to examine the performance of multiple models for the simulation of the spatial pattern and evolution of the PDO during the entire twentieth century.
The rest of this paper is organized as follows: Section 2 describes the models, experimental design, and the method employed. In Section 3, the simulated PDO in the CMIP5 coupled models is compared with observations. Finally, conclusions and a discussion are given in Section 4.
2. Model experiments, data, and method
A total of 109 historical simulations from 25 coupled models in CMIP5 (Taylor, Stouffer, and Meehl Citation2012) are analyzed in this study. The coupled models in the historical simulations (also called all-forcing simulations) are forced by both natural (total solar irradiance and volcanoes) and anthropogenic (well-mixed greenhouse gases and anthropogenic aerosols) forcings, which cover the period from 1850 to 2005. The details of the CMIP5 coupled models, including the model acronyms and affiliations, are listed in Table . The observed SST used in this study is from the Hadley Center’s monthly SST data-set (Rayner et al. Citation2003).
Table 1. Details of the 25 CMIP5 coupled models in this study.
In this study, we calculated the PDO index as the leading principal component of SST anomalies in the North Pacific (poleward of 20°N). Before the EOF analysis, the global warming signal is removed from the data by subtracting the mean global SST anomaly. For the entire twentieth century, we focus on the spatiotemporal characteristics of the PDO in the Northern Hemisphere winter (i.e. November–March), because the PDO behavior is substantially intensified in winter (Mantua et al. Citation1997; Deser and Phillips Citation2006). We use the regular-grid surface temperature (i.e. the CMIP5 output variable ‘ts’) over the ocean instead of the irregular-grid SST fields in the analysis of modeled data. In addition, we set the surface temperature in regions covered by sea ice to −1.8 °C according to the observed SST data-set (Rayner et al. Citation2003).
To evaluate the effectiveness of the models for the simulation of the PDO, the spatial correlation coefficient (SCC) of the PDO teleconnection patterns and the correlation coefficient of the PDO indices between the observations and CMIP5 coupled models are computed in this study. The multi-model ensemble simulation is calculated as the arithmetic mean of the ensemble simulation for the multi-member 22 coupled models with multi-member simulations (i.e. greater than one member). The Pearson’s linear correlation coefficient is used to describe the significance of the correlation coefficients. To highlight the decadal signals, the 9-yr running mean is used on all of the PDO indices before calculating the correlation coefficients of the time series. The formula of Quenouille (Citation1952) is used to estimate the effective degrees of freedom (Ne) of the 9-yr running mean indices:
where N is the number of data points, and ai and bi are the ith order autocorrelations for time series a and b, respectively.
3. Results
As shown in the regression map (Figure ), the observed SSTs are anomalously cool in the western-central region of the North Pacific during the positive PDO phase, and are surrounded by anomalously warm SSTs along the west coast of the Americas. At the same time, El Niño–like SST anomalies are evident in the central-eastern tropical Pacific, but negative SST anomalies are observed in the subtropical South Pacific. The explained variance for the PDO pattern is 33.2% in the observations. Compared with the observations, most coupled models in CMIP5 reproduce the PDO pattern well, particularly in the North Pacific, which is also the case for the CMIP5 last millennium simulations (Fleming and Anchukaitis Citation2016). BNU-ESM, CanESM2, CCSM4, CESM1-CAM5, CSIRO Mk3.6.0, GFDL CM3, GFDL-ESM2M, HadCM3, HadGEM2-ES, MIROC5, and NorESM1-M reproduce the observed teleconnections of the PDO to SSTs in the tropical and Southern Pacific well. These simulated teleconnections in other models are relatively weak, particularly for the r1i1p1 simulations of BCC_CSM1.1, CNRM-CM5, GISS-E2-H, and MRI-CGCM3. Based on the statistical results, BNU-ESM, CanESM2, CCSM4, CESM1-FASTCHEM, FGOALS-g2, GFDL CM3, MIROC5, and NorESM1-M better depict the PDO pattern (Table ). The SCCs of the PDO regression maps between the observations and these models are greater than 0.8. Additionally, the CanESM2, CCSM4, CESM1-FASTCHEM, FGOALS-g2, MIROC5, and NorESM1-M simulate a higher explained variance for the PDO pattern (~30%), which is comparable to the observations. In the r1i1p1 simulations of GISS-E2-R, MPI-ESM-LR, and MRI CGCM3, the explained variance for the PDO pattern is small, at less than 20%.
Table 2. Spatial correlation coefficients (SCCs) of the PDO regression maps between the observation and simulations.
The ensemble results for the 22 coupled models (i.e. multi-model ensemble) are shown in Figure . MIROC5 reproduces the PDO pattern most similar to the observations. Its SCC is 0.88, which is greater than most single-model ensemble simulations. The SCC of the CCSM4 ensemble simulation is 0.89, and is the highest SCC. However, the intensity of its PDO pattern is weaker than in the observations, as is the case for the other single-model ensemble simulations. This suggests that the internal variability of the PDO is weakened to some extent by the ensemble average, particularly for simulations by the larger members (e.g. CNRM-CM5 and CSIRO Mk3.6.0; Figure ). In the multi-model ensemble simulation, the simulated PDO pattern explains 38.1% of the total variance of the SST anomalies. The SCC between the PDO pattern and the observations is as high as 0.81 (Figure ). However, the teleconnections to the SST anomalies are very weak, as also seen in Figure .
Figure 2. Observed and simulated regression distributions of SST on the PDO index (units: °C).
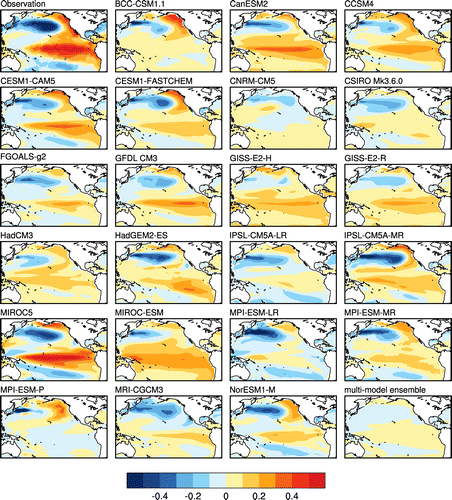
Figure 3. Simulated regression distribution of SST on the PDO index (units: °C) in the multi-model ensemble simulation.
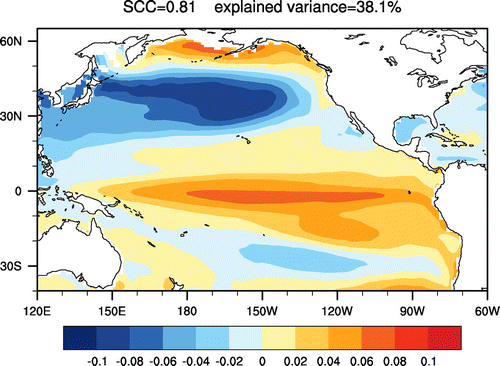
Many climate shifts are closely tied to the phase transition of the PDO (e.g. Hartmann and Wendler Citation2005; Zhu et al. Citation2011; Lyon, Barnston, and DeWitt Citation2014). Therefore, more attention has been paid to the evolution of the PDO and its phase shifts. In the twentieth century, the observed PDO enters a positive phase in the early 1920s, and then a negative phase in the early 1940s (Figure ). Finally, it returns to a positive phase again in the late 1970s. The simulated PDO evolutions in the twentieth century are very different from one another. Different external forcings (e.g. volcanic forcing and anthropogenic aerosols) used in these models can contribute to the differences in simulated PDO evolutions. Even for individual models and forced by the same external forcings, the simulated PDO evolutions are also different among members. This means that the initial states probably play an important role in shaping subsequent PDO evolution. Compared to the observations, therefore, most simulations do not reproduce the observed temporal phases of the PDO (Table ). However, eight simulations produce a similar PDO evolution as observed (Figure ). These are the r5i1p1 simulation of CanESM2, the r3i1p1 and r8i1p1 simulations of CNRM-CM5, the r6i1p1 simulation of CSIRO Mk3.6.0, the r3i1p1 simulation of GISS-E2-R, the r8i1p1 simulation of HadCM3, the r4i1p1 simulation of IPSL-CM5A-LR, and the r1i1p1 simulation of MPI-ESM-LR. The simulated PDO time series in five simulations are significantly associated with the observed PDO index. Their correlation coefficients are greater than 0.36 (p < 0.05; Table ), which suggests that some of the coupled models, forced by all relevant external forcings, could by chance reproduce the observed PDO evolution to some extent. Additionally, the r6i1p1 simulation of CSIRO Mk3.6.0 reproduces the PDO evolution in the second half of the twentieth century well (Figure ).
Figure 4. Observed and simulated PDO indices.
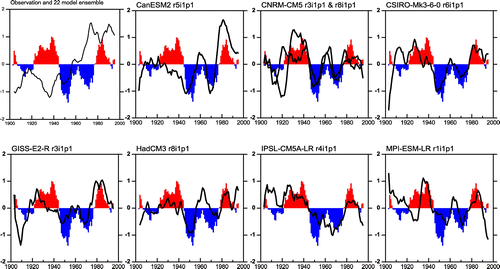
Table 3. Correlation coefficients between the observed and simulated PDO indices, and between the observation and ensemble simulation for each model.
No ensemble simulations reproduce the observed PDO evolution (Table ). In CanESM2, GFDL CM3, GISS-E2-H, GISS-E2-R, HadGEM2-ES, IPSL-CM5A-MR, and MIROC-ESM ensemble simulations, and the multi-model ensemble, the simulated PDO indices show a significant long-term tendency to the positive phase in the twentieth century. One reason for large discrepancies between the observed and simulated PDO indices may be that the internal variability of the PDO has been averaged out in the ensemble simulations, as noted by Dong, Zhou, and Chen (Citation2014). Particularly for the multi-model ensemble, the positive trend of simulated PDO index probably reflects the response of the PDO to increasing levels of the greenhouse gases in the twentieth century (Dong, Zhou, and Chen Citation2014), which are the same for all the coupled models in this study.
4. Summary and discussion
We examine the spatial and temporal characteristics of the PDO in the 109 historical simulations from 25 coupled models in CMIP5. Most of the simulations successfully reproduce the ‘horseshoe’ shape SST anomalies in the North Pacific and observed PDO teleconnections to SSTs in the tropical and South Pacific. Additionally, BNU-ESM, CanESM2, CCSM4, CESM1-FASTCHEM, FGOALS-g2, GFDL CM3, MIROC5, and NorESM1-M better simulate the PDO-associated SST pattern in the Pacific. The SCCs between the observed and simulated PDO regression maps are greater than 0.8.
Compared with the observed PDO evolution in the twentieth century, only five simulations of a total of 109 historical members simulate similar temporal phases of the PDO. The correlation coefficients between the simulated and observed PDO indices are greater than 0.36 for the r3i1p1 and r8i1p1 simulations of CNRM-CM5, the r6i1p1 simulation of CSIRO Mk3.6.0, the r8i1p1 simulation of HadCM3, and the r4i1p1 simulation of IPSL-CM5A-LR. Their similarity is significant at the 0.05 significance level. It suggests that coupled models, forced by all the relevant external forcings, can by chance reproduce the observed PDO evolution. In other words, the current coupled models barely simulate the temporal evolution of the observed PDO in the twentieth century. Perhaps realistic initial states of the oceanic field are necessary for the models to capture the temporal characteristics of the PDO.
We also analyze the simulated PDO in the ensemble simulation for each coupled model and in the multi-model ensemble. After the ensemble average, different internal variabilities from multi-member simulations interfere with one another. Particularly for the multi-model ensemble, some external forcings (e.g. volcanic forcing and anthropogenic aerosol forcing) used in these coupled models are different. Therefore, it is not appropriate to investigate the impacts of the internal modes (e.g. the PDO and the Atlantic Multidecadal Oscillation) on the regional and global climate using ensemble simulations, because the internal variabilities from different members are not consistent and interfere with one another.
Funding
This work was supported by the National Key R&D Program of China [grant number 2017YFA0603802]; the National Natural Science Foundation of China [grant numbers 41661144005, 41320104007, and 41575086]; and the CAS–PKU (Chinese Academy of Sciences–Peking University) Joint Research Program.
Disclosure statement
No potential conflict of interest was reported by the authors.
References
- Arblaster, J. M., G. A. Meehl, and A. M. Moore. 2002. “Interdecadal Modulation of Australian Rainfall.” Climate Dynamics 18 (6): 519–531.
- Cai, W. J., and P. van Rensch. 2012. “The 2011 Southeast Queensland Extreme Summer Rainfall: A Confirmation of a Negative Pacific Decadal Oscillation Phase?” Geophysical Research Letters 39: L08702.
- Deser, C., and A. S. Phillips. 2006. “Simulation of the 1976/77 Climate Transition Over the North Pacific: Sensitivity to Tropical Forcing.” Journal of Climate 19 (23): 6170–6180.10.1175/JCLI3963.1
- Deser, C., A. S. Phillips, and J. W. Hurrell. 2004. “Pacific Interdecadal Climate Variability: Linkages between the Tropics and the North Pacific during Boreal Winter Since 1900.” Journal of Climate 17 (16): 3109–3124.10.1175/1520-0442(2004)017<3109:PICVLB>2.0.CO;2
- Dong, X., and F. Xue. 2016. “Phase Transition of the Pacific Decadal Oscillation and Decadal Variation of the East Asian Summer Monsoon in the 20th Century.” Advances in Atmospheric Sciences 33 (3): 330–338.10.1007/s00376-015-5130-7
- Dong, L., T. J. Zhou, and X. L. Chen. 2014. “Changes of Pacific Decadal Variability in the Twentieth Century Driven by Internal Variability, Greenhouse Gases, and Aerosols.” Geophysical Research Letters 41 (23): 8570–8577.10.1002/2014GL062269
- Fleming, L. E., and K. J. Anchukaitis. 2016. “North Pacific Decadal Variability in the CMIP5 Last Millennium Simulations.” Climate Dynamics 47: 3783–3801.
- Hartmann, B., and G. Wendler. 2005. “The Significance of the 1976 Pacific Climate Shift in the Climatology of Alaska.” Journal of Climate 18 (22): 4824–4839.10.1175/JCLI3532.1
- Kosaka, Y., and S. P. Xie. 2013. “Recent Global-warming Hiatus Tied to Equatorial Pacific Surface Cooling.” Nature 501 (7467): 403–407.10.1038/nature12534
- Latif, M., and T. P. Barnett. 1994. “Causes of Decadal Climate Variability Over the North Pacific and North-America.” Science 266 (5185): 634–637.10.1126/science.266.5185.634
- Lyon, B., A. G. Barnston, and D. G. DeWitt. 2014. “Tropical Pacific Forcing of a 1998-1999 Climate Shift: Observational Analysis and Climate Model Results for the Boreal Spring Season.” Climate Dynamics 43 (3–4): 893–909.10.1007/s00382-013-1891-9
- Mantua, N. J., and S. R. Hare. 2002. “The Pacific Decadal Oscillation.” Journal of Oceanography 58 (1): 35–44.10.1023/A:1015820616384
- Mantua, N. J., S. R. Hare, Y. Zhang, et al. 1997. “A Pacific Interdecadal Climate Oscillation with Impacts on Salmon Production.” Bulletin of the American Meteorological Society 78 (6): 1069–1079.10.1175/1520-0477(1997)078<1069:APICOW>2.0.CO;2
- Qian, C., and T. J. Zhou. 2014. “Multidecadal Variability of North China Aridity and Its Relationship to PDO during 1900–2010.” Journal of Climate 27 (3): 1210–1222.10.1175/JCLI-D-13-00235.1
- Quenouille, M. H. 1952. Associated Measurements, 242. New York: Butterworths Scientific Publications, London and Academic Press.
- Rayner, N. A., D. E. Parker, E. B. Horton, et al. 2003. “Global Analyses of Sea Surface Temperature, Sea Ice, and Night Marine Air Temperature Since the Late Nineteenth Century.” Journal of Geophysical Research-Atmospheres 108 (D14): 4407.
- Schneider, N., and B. D. Cornuelle. 2005. “The Forcing of the Pacific Decadal Oscillation.” Journal of Climate 18 (21): 4355–4373.10.1175/JCLI3527.1
- Sun, J. Q., and H. J. Wang. 2006. “Relationship between Arctic Oscillation and Pacific Decadal Oscillation on Decadal Timescale.” Chinese Science Bulletin 51 (1): 75–79.10.1007/s11434-004-0221-3
- Taylor, K. E., R. J. Stouffer, and G. A. Meehl. 2012. “An Overview of CMIP5 and the Experiment Design.” Bulletin of the American Meteorological Society 93 (4): 485–498.10.1175/BAMS-D-11-00094.1
- Wang, T., O. H. Ottera, Y. Q. Gao, et al. 2012. “The Response of the North Pacific Decadal Variability to Strong Tropical Volcanic Eruptions.” Climate Dynamics 39 (12): 2917–2936.10.1007/s00382-012-1373-5
- Wang, T., H. J. Wang, O. H. Ottera, et al. 2013. “Anthropogenic Agent Implicated as a Prime Driver of Shift in Precipitation in Eastern China in the Late 1970s.” Atmospheric Chemistry and Physics 13 (24): 12433–12450.10.5194/acp-13-12433-2013
- Yu, L., T. Furevik, O. H. Ottera, et al. 2015. “Modulation of the Pacific Decadal Oscillation on the Summer Precipitation Over East China: a Comparison of Observations to 600-years Control Run of Bergen Climate Model.” Climate Dynamics 44 (1–2): 475–494.10.1007/s00382-014-2141-5
- Zhang, R., and T. L. Delworth. 2007. “Impact of the Atlantic Multidecadal Oscillation on North Pacific Climate Variability.” Geophysical Research Letters 34: L23708.
- Zhou, B. T. 2016. “The Asian-Pacific Oscillation Pattern in CMIP5 Simulations of Historical and Future Climate.” International Journal of Climatology 36: 4778–4789.10.1002/joc.2016.36.issue-15
- Zhu, Y. L., H. J. Wang, W. Zhou, et al. 2011. “Recent Changes in the Summer Precipitation Pattern in East China and the Background Circulation.” Climate Dynamics 36 (7–8): 1463–1473.10.1007/s00382-010-0852-9
- Zhu, Y. L., H. J. Wang, J. H. Ma, et al. 2015. “Contribution of the Phase Transition of Pacific Decadal Oscillation to the Late 1990s’ Shift in East China summer rainfall.” Journal of Geophysical Research-Atmospheres 120 (17): 8817–8827.10.1002/2015JD023545