ABSTRACT
Recent hardware advances in the field of 3D imaging are democratizing 3D sensing with tremendous scientific and societal implications. Point cloud processing has traditionally required meshing the point clouds output by 3D cameras followed by surface reconstruction, and geometric feature recognition. However, meshing a point cloud is fundamentally an ill-posed problem, and the definition of a good solution is not general. This, in turn, demands new paradigms for processing point cloud information.
In this paper, we focus on the task of classifying 3D point clouds captured with commercial 3D cameras, and we integrate supervised machine learning algorithms with three different yet underexplored shape descriptors, namely Light-Field Descriptors, Angular Radial Transform (ART), and Zernike Descriptors (ZD). We evaluate the classification performance of different machine learning algorithms combined with these different shape descriptors on point cloud models obtained from the Google 3D Warehouse, and we demonstrate good classification performance for 3D point clouds. Furthermore, we show that Zernike Descriptors are practically insensitive to noise levels typically found in point cloud models captured via 3D sensing.
GRAPHICAL ABSTRACT
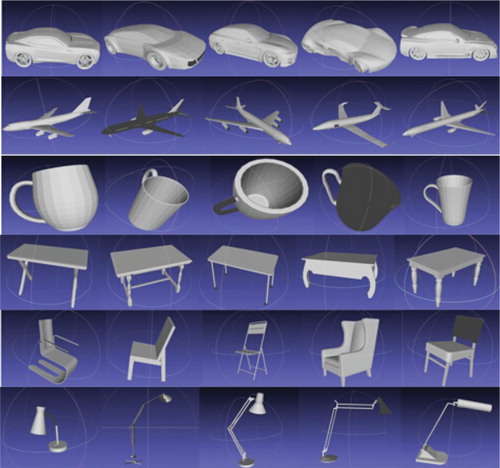
Acknowledgements
This work was supported in part by the National Science Foundation grants CMMI-1200089, CMMI 1462759, and CHS 1526249.
ORCID
Xiaojun Zhao http://orcid.org/0000-0002-7769-5272