Abstract
The main problem of the quantitative texture analysis is discussed. The sharp texture of nanocrystalline magnesium is under investigation. The well-known harmonic (Roe–Bunge) method of texture determination does not give good results for the materials with low sample symmetry. The robust component fit method for the determination of the orientation distribution function from the set of incomplete X-ray pole figures is used in this work. A unique and reliable solution is achieved.
1. Introduction
The orientation distribution function (ODF) f(g) defined on the rotation group SO(3) gives full texture description, but it is not measured directly. The experimental pole figures (PF) denoted as are the projections of the ODF to the unit sphere S2 Citation1:
The problem of the ODF determination from the set of PFs is ill-posed mainly because of the solution non-uniqueness. This is caused by experimental undistinguishness of the crystallographic directions
and
. Another source of ill-posedness is the presence of experimental errors. This evokes the solution non-robustness.
The component fit method also referred to as the method of ODF approximation by standard functions is well-known in the quantitative texture analysis (QTA) Citation2–5. The method is served for the texture investigation in polycrystalline materials. Complete or incomplete PFs obtained by X-ray or the neutron scattering method are used for ODF determination. Various analogues of the Gaussian distribution on the rotation group SO(3) are proposed for mathematical modelling of an ODF and PFs Citation2,Citation3,Citation6. We used the normal distribution obeying the central limit theorem on the group SO(3) Citation4,Citation5.
2. Peculiar properties of the experimental PFs
In this study, the specimen of nanocrystalline (submicroscopic) Mg is investigated. This is obtained from initial coarse-grained bar by repeated pushing through an equal-channel angular die at temperature T = 300°C (so–called ECAP procedure). There were six incomplete (i.e. determinated not on the whole sphere) non-normalized X-ray PFs {0002}, ,
,
,
and
measured in the Institute of Metallurgy (IMET, Moscow, Russia). Distinctive features of the investigated material are the lattice hexagonal symmetry and the lack of specimen orthorhombic symmetry in the presence of fairly sharp texture. The main aim of this study is to determine ODF using the set of PFs. The ODF Fourier–coefficients are used in researching the superplasticity phenomenon of magnesium nanomaterials.
3. Difficulties connected with the material features
The Roe–Bunge Citation1 method associated with ODF and PFs expansion into Fourier series on corresponding manifolds, does not yield satisfactory results. Firstly, in classic scheme this method reconstructs only the even part of ODF, further the method is extremely sensible to measurement errors, which may reach a degree of 40% in X-ray diffraction experiment (this is the case in our investigation). Lastly, the method does not allow the control of the consistency of values at different PFs. Numerous modifications of this method Citation7–10 which allow these problems to be more or less overcome, do not work in the case of fairly sharp peaks on the PFs, combined with the specimen low symmetry. In this case, the sharp texture may be approximated only with long fragment of Fourier series, whose number of coefficients is too large.
4. The component fit method. Central normal distributions
The component fit method, which we employ, allows the modelling of the main texture features using a few parameters. Let be the orientation distance between rotations g and g0k (here
signifies the trace of the matrix product). The ODF is supposed to be a superposition of K central normal distributions both peak
and axial
with unknown weights ck (c0–background weight), dispersion parameters ϵk, maxima positions g0k (for axial components with axes
also), k = 1, …, K. Here
denotes the Legendre polynomial of l–th order whose argument is the product of vectors
and
. Such kind of ODF generates the PF with the same parameters
These are the PFs which are usually measured in the experiment, so we can estimate unknown parameters mostly by fitting the set of measured PFs. Letting and
be directions in the sample and in the space correspondingly, then
In this equation, vector
is a set of linear parameters (component weights),
is a vector of nonlinear (remaining) parameters and
are the measurement errors. The last ones depend on many factors, such as number of reflecting grains, non-ideal geometry, defocussing effects, etc. In our work, the measurement errors are assumed to be independent and to have identical accuracy.
The maximum likelihood estimators for unknown parameters of the model are the values which minimize the sum of weighted squared residuals
Here the statistical weight
reflects the degree of the significance of the measurement at point
on
. The parameters Nλ should be chosen to normalize the experimental PFs.
Consider first the linear problem. Suppose the texture to be formed of (K − 1) components with almost exactly known maxima positions (the class of correctness for the problem). Then the model ODF is
so it generates the model PFs
The number of linear parameters to be fitted is K. Let A = (Ask) be the design matrix Citation11 of this problem and let vector
represent the experimental PF data. Then the minimization of r2 yields the normal equations of the least squares problem:
In many cases, the normal equations are very close to singularity (the condition number is too large), so a regularization for this linear system is necessary. The regularization is realized by application of singular value decomposition of the design matrix A Citation12. The condition number of the system is decreased due to thresholding and zeroing small singular values.
In the case of nonlinear parameters the minimization has to be implemented iteratively. Starting from
we update the values
in each step of the iterations. The initial values
are usually solutions of a simpler problem. Consider the quadratic approximation of the common residual
where D = (Dpq) is the Hessian matrix
In the Levenberg–Marquard method Citation13, the Hessian D is replaced by some approximation
with some heuristic parameter μ, and the updating procedure is given by
If
, then
assigns the new values
and μ decreases by some factor, if it is not the case, then
retains the old values and μ increases by some factor. Iterations stop when common residual value becomes stable. It should be noted that matrix
may become degenerate if the dependence on some particular parameter (tp) is rather weak, and in some other cases when μ is very small. That is why the regularization of the same type is desirable in the nonlinear case too.
5. Results
Only 2–3 PFs from the set are used for searching the parameters. All the set of model PFs is recalculated after definition of the parameters. The reliability of the model is checked by the response probability (RP)-criterion Citation1. The RP values for PFs used in the least squares approximation and for unused ones are the same.
The texture of initial coarse-grained specimen is found to be axial. The complete ODF is equal to the even ODF part (so the odd ODF part is null). In some PFs before equal channel angular pressing are represented, the experimental data are to the left and model data to the right. In the sections of corresponding complete ODF are displayed.
Figure 1. Initial axial texture of coarse-grained specimen: experimental PFs (left) and model PFs (right).
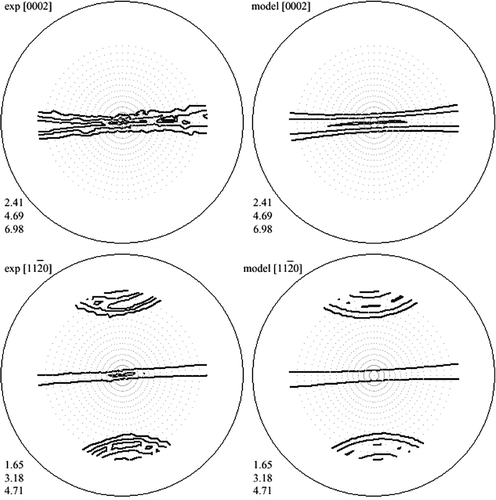
After ECAP procedure, the texture became very sharp and the ODF got significant odd part. In , experimental and model PFs after ECAP are shown. Complete ODF and its even part are presented in and . The fit component parameters are given in .
Figure 3. Texture after equal channel angular pressing: experimental PFs (left) and model PFs (right).
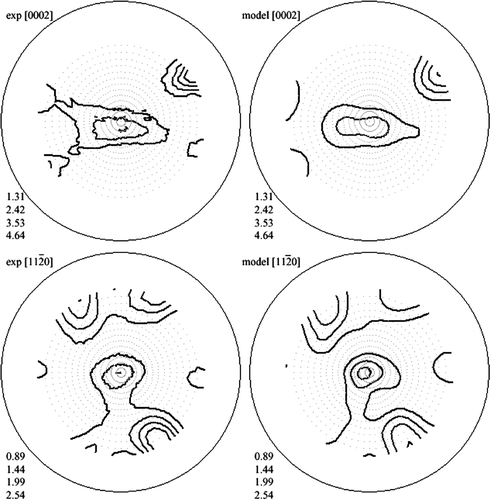
Figure 5. Texture after ECAP: the sections of the even ODF part. The regions of negative values are marked.
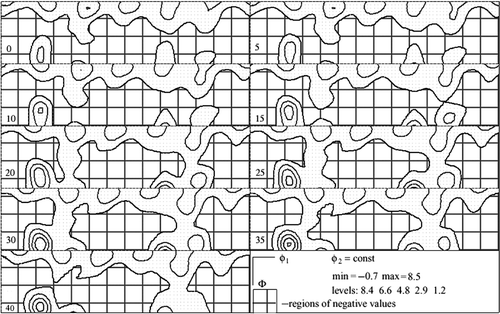
Table 1. The texture parameters after severe deformation.
6. Conclusions
The initial texture of the material is axial. Arising after severe deformation, the nanocrystalline texture is formed by eight peak components.
Due to material features the application of harmonic methods for ODF definition requires an enormous quantity of experimental data, which is inaccessible.
Experimental PFs contain large errors that makes the problem of ODF definition very unstable. The robustness is achieved by regularization. Zeroing the small singular numbers in the problem improves the statistic properties of the solution, by making it more smooth and stable.
The even part of ODF contains domains with vastly negative values and the maxima of the even ODF part are 30% less than the maxima of the complete ODF.
The robust component method using central normal distributions is the method of first choice, since it allows matched values of different PFs (to control consistency of different PFs–both used and unused in the approximation procedure), reconstructs full non-negative ODF and operates with a small number of parameters. This method results in an explicit form for the ODF coefficients.
Acknowledgements
This work is supported by the State contract ‘Investigation of textures and plastic anisotropy magnesium nanomaterials’ No. 02.513.11.3340. The authors thank research worker of IMET, V.I. Serebryany, for consultation about experimental part of the work and given PFs.
References
- Bunge, HJ, 1982. Texture Analysis in Material Science. London: Butterworths; 1982.
- Matthies, S, 1982. Form effect in the description of the orientation distribution function of texturized materials by model components, Phys. Status Solidi (b) 112 (1982), pp. 705–716.
- Helming, K, and Eschner, T, 1990. A new approach to texture analysis of multiphase materials using a texture component model, Cryst. Res. Technol. 25 (1990), pp. K203–K208.
- Savyolova, TI, 1985. Introduction in New Methods of Polycrystyalline Materials Study. Moscow: Metallurgy; 1985. pp. 10–30.
- Ivanova, TM, and Savyolova, TI, 2006. Robust method of approximating the orientation distribution function by canonical normal distribution, P. Metals Metall. 101 (N2) (2006), pp. 129–133.
- Schaeben, H, 1996. A unified view of methods to resolve the inverse problem of texture goniometry, Text. Microstruct. 25 (1996), pp. 171–181.
- Bunge, HJ, and Esling, C, 1981. Determination of the texture function by anomalous scattering, J. Appl. Cryst. 14 (1981), pp. 253–255.
- Dahms, M, and Bunge, HJ, 1989. The iterative series-expansion method for quatitative texture analysis. I. General outline: II Applications, J. Appl. Cryst. 22 (1989), pp. 439–447, J. Appl. Cryst. 25(1992), pp. 259–267.
- Wagner, F, 1996. The use of positivity method to approximate the complete ODF, Text. Microstruct. 25 (1996), pp. 171–204.
- Serebryany, VN, Kourtasov, SF, and Savyolova, TI, 1996. Presented at Proceedings of the 14th International Conference on Textures of Materials. Leuven, Belgium, Switzerland, 2005.
- Kahaner, D, Moler, C, and Nash, S, 1989. Numerical Methods and Software. Englewood Cliffs, NJ: Prentice Hall; 1989.
- Golub, G, and Van Loan, Ch, 1996. Matrix Computations. Baltimore: Johns Hopkins University Press; 1996.
- Dennis, JE, and Schnabel, RB, 1983. Numerical Methods for Unconstrained Optimization and Nonlinear Equations. Englewood Cliffs, NJ: Prentice Hall; 1983.