Abstract
We developed a coupled soil initialization scheme for the Florida State University/Center for Ocean-Atmosphere Prediction Studies (FSU/COAPS) climate model, which is coupled to the Community Land Model version 2. This method indirectly initializes the land surface component of the climate model by assimilating precipitation in the atmospheric component. A continuous assimilation is performed while the land and atmosphere components exchange fluxes, thus keeping the coupled model in a physically consistent state, obviating the need for any correction. Comparison with in situ observations in Illinois and Oklahoma shows very good agreement with the initialized soil moisture profile. A global comparison of the initialized land surface model with the Global Soil Wetness Project 2 multi-model analysis also shows very good agreement. Short-term climate forecasts (2 weeks to 2 months), performed in conjunction with the Global Land Atmosphere Coupling Experiment, Part 2, show that initialized soil moisture conditions improve surface temperature forecasts.
1. Introduction
It has been hypothesized that the persistence of soil moisture may lead to increased predictability of climate in the short term, 2 weeks to 2 months, in much the same manner that persistence of sea surface temperatures has lead to improvements of seasonal predictability on the timescale of 3 months or more. Some initial studies have shown that significant changes in soil moisture anomalies can have an impact on the short-term climate Citation1–7. A joint multi-model comparison project, the Global Land Atmosphere Coupling Experiment Part 1 (GLACE1), identified ‘hot spots’ or regions where there was large sensitivity to climate due to soil moisture anomalies Citation8. These findings have lead to increased motivation to develop improved land surface conditions, which may enhance predictability. However, initializing land surface models (LSMs) poses a number of challenges. Most significantly, there are very few observations of soil moisture throughout the globe. Moisture that is deep in the soil requires in situ probes to measure. For near surface soil conditions, there have been attempts at using remote-sensing techniques to estimate soil moisture. These remotely sensed estimates, however, have not been fully validated (again due to the lack of sufficient in situ observations), and the length of record is often not long enough to cover historical periods of interest. Thus, the lack of adequate soil moisture data makes it exceedingly difficult to create a set of initial conditions for LSMs. It also makes it difficult to even validate LSMs. As a result, it is difficult to calibrate LSMs and develop proper parameterizations of land surface processes. Different LSMs, therefore, may have large differences in the mean state of the land surface variables. Complicating the situation is that the atmospheric component of climate models have biases themselves, and LSMs have to be tuned to achieve realistic results in a coupled land–atmosphere system. So, even if one has perfect observations for the land surface state, it may still not be adequate to initialize the coupled system. The coupled land–atmosphere modelling system, each component with their own imperfections, tends to develop its own ‘climatology’.
The problem of analysing soil moisture can be characterized as an inverse problem. Therefore, some standard techniques used to compute inverse solutions can be applied to this problem. Data assimilation is a very important part of numerical prediction systems. Data assimilation is indeed an inverse problem. Therefore, some strategies employed for data assimilation can be applied to address the current problem.
Perhaps the most common approach to initialize an LSM is to drive the LSM ‘off-line’ (uncoupled) with observed or analysed atmospheric fluxes. In this approach, the atmospheric fluxes are applied directly to the surface of the LSM, and there is no feedback from the LSM. However, the subsequently initialized land state will, in general, not be in balance with the coupled system. A correction to the mean and variance of the state variables is then often applied
(1)
This correction essentially adjusts the climatology of the off-line model to the climatology of the coupled (online) model. This correction introduces errors due to the nonlinearity of the system.
To overcome some of the prevalent issues in off-line initialization methods, we sought to develop a coupled initialization method. Since precipitation is of overriding importance in initializing soil moisture, we needed a method that ensures that the precipitation flux entering the LSM is as close to observed as possible. Yet, we also require that all of the other fluxes, such as solar radiation, be consistent with the precipitation flux, and that all of the fluxes are physically consistent in general. Our solution, then, was to assimilate observed precipitation into the atmospheric component of the model while the atmospheric and land components are coupled, and hence exchange fluxes. The precipitation assimilation method is a nudging method that alters the vertical humidity profile in order to bring the model rain rate closer to the observed rain rate. The atmospheric prognostic variables are nudged towards observation-based reanalyses so that the atmospheric state remains close to observations while still permitting modifications needed to reflect the assimilation of precipitation (e.g. heating and moistening in the vertical column, local circulation changes).
Nudging methods are often criticized in light of the more sophisticated assimilation systems available today. While much of the criticisms may be valid in general, they may not be as relevant to the problem we are trying to solve here. For example, one criticism is that nudging methods do not take into account the observational error. We assume that the observed precipitation has no error. However, the off-line methods discussed above also make this same assumption, so the nudging method is no worse. On the contrary, the nudging method, when appropriately applied, will only assimilate precipitation that is consistent with the model atmospheric state, thereby reducing possibly erroneous precipitation forcing of the LSM. While the nudging method described herein neglects the relative error characteristics of the atmospheric variables, we have generally found that assimilating precipitation leads to more plausible values of the atmospheric state variables when compared to independent observations. For example, the divergent circulation associated with deep convection appears to be more credible after precipitation assimilation Citation9. In any case, any error that could potentially be introduced in a particular atmospheric state variable is likely to be more than compensated by the overall improvement in the surface fluxes, particularly the moisture and radiative fluxes.
An additional concern about the nudging method is that the resulting solution is not unique. This is typical, in general, for nonlinear inverse problems. There are an infinite number of vertical profiles of atmospheric state variables that can yield the same precipitation. Our assumption is that by incrementally modifying the state variables to achieve the desired precipitation, the solution will tend, in the best case, towards the most likely solution, and in the worst case, towards a reasonable solution. However, a reasonable solution that is incorrect may not be too detrimental to the soil initialization. This is due to the fact that, as stated above, soil moisture is most important, and the LSM responds to the fluxes at the surface, and not the details of the three-dimensional atmospheric state variables. For example, the short-wave radiative flux at the surface will be highly correlated to the precipitation at the surface, even though the clouds could be distributed in a variety of different ways throughout the vertical column.
This article presents some first results from two multi-year assimilation experiments. We compare the soil initialization with in situ observations as well as a global multi-model analysis. The Florida State University (FSU) Center for Ocean–Atmosphere Prediction Studies (COAPS) participated in a joint international multi-model intercomparison project, GLACE2, to assess the impact of initialized LSMs on short-term climate forecasts. Section 2 describes the model, assimilation method and data used. Section 3 describes the results of the assimilation validation and presents forecast results. We conclude this article in Section 4.
2. Methodology
2.1. Model
In this study, we use the FSU/COAPS climate model as described in Cocke and LaRow Citation10. The model is a spectral primitive equation model with an Eulerian semi-implicit time integration scheme. For these experiments, the model is configured with a horizontal resolution of 63 waves with triangular truncation (‘T63’), about 1.875° latitude–longitude at equator (200 km), and 27 vertical terrain-following σ-coordinate levels. The vertical coordinate is defined as σ = P/Ps, where P is the pressure at the vertical level, and Ps is the pressure at the surface. Thus, the vertical coordinate goes from 0 at the top of the atmosphere to 1 at the surface. The sea surface temperatures were specified as lower boundary conditions rather than using the dynamical ocean model. The FSU/COAPS climate model has been enhanced to include options for physical parameterizations adapted from the National Center for Atmospheric Research (NCAR) Community Climate Model (CCM) version 3.6. In the results presented here, the deep cumulus scheme used was the modified Kuo scheme as in Cocke and LaRow Citation10. The shallow convection, cloud parameterization, vertical diffusion and radiation schemes are from the CCM 3.6, and are described in Kiehl et al. Citation11. The shallow convection parameterization is based on Hack Citation12. The cloud parameterization is a generalization of Slingo Citation13 and depends on relative humidity, vertical velocity, stability and convective mass flux. The long-wave radiative transfer is an absorptivity–emissivity method based on Ramanathan and Downey Citation14. Short-wave radiation is based on the delta-Eddington approximation Citation15. The vertical diffusion is based on the nonlocal diffusion method of Holtslag and Boville Citation16. The FSU/COAPS climate model was coupled to the NCAR/Community Land Model version 2 (CLM2, Citation17). Each grid cell in the CLM2 is divided into five primary subgrid land cover fractions (glacier, lake, wetland, urban and vegetated). Each vegetated portion of the grid cell is divided into patches of up to four plant function types. The CLM2 produces prognostic soil moisture fields for 10 soil layers. The FSU/COAPS model coupled to the CLM2 land model is hereafter referred to as the ‘FSUCLM’. Details of the coupling method and validation can be found in Shin et al. Citation18 and Boisserie et al. Citation19.
2.2. Assimilation method
As discussed in Section 1, our objective is to assimilate soil moisture indirectly by assimilating precipitation in the coupled atmosphere–land model. The Precipitation Assimilation Reanalysis (PAR) method used here is similar to the physical initialization scheme used in the study of Krishnamurti et al. Citation20, but modified according to Kim et al. Citation9 and Nunes and Cocke Citation21. The basic concept of PAR is to nudge the model precipitation towards observed estimates by adjusting the vertical moisture profile while simultaneously nudging the other prognostic atmospheric variables (temperature, surface pressure, vorticity and divergence) towards observation-based atmospheric reanalyses using Newtonian relaxation as the model is integrated forward in time. The physical initialization scheme has a similar humidity adjustment procedure and Newtonian relaxation, but includes other adjustments that are not incorporated in the PAR method and is discussed in more detail in Krishnamurti et al. Citation20.
Precipitation nudging is performed by explicitly modifying the vertical profile of humidity in the model based on the model and observed rain rates during the assimilation phase. At each time step of the model (15 min), the specific humidity (q) at each σ-level is modified according to
(2)
where Ro is the observed rain rate (interpolated in time to the time step of the model) and Rm is the model rain rate. The effect of this modification is to alter the moist static energy of the atmosphere to help induce or inhibit moist convection. The amount of modification of the specific humidity at each time step is capped so that unrealistic moisture distributions do not occur and permit a more gradual assimilation of the precipitation. As the humidity profile is incrementally modified, the model physics will generate changes in the tendencies of the vertical heating profile and associated circulation so that the model state will move towards a state that is consistent with the assimilated precipitation.
The Newtonian relaxation is incorporated into the equations of motion via a dynamical nudging term
(3)
where A is a prognostic variable of the model, F(·) is the full model time tendency of A, with H being an observation operator, Ar is the corresponding reanalysis variable, interpolated to the time step of the model and α is a relaxation (nudging) coefficient. The first term on the right-hand side of Equation (3) represents the dynamical and physical tendencies generated by the model for the prognostic variable and the second term is a relaxation term. In other words, a relaxation term is added to each equation of motion for each prognostic variable: surface pressure, temperature, vorticity and divergence. The model solves the time integration of the equations of motion in the spectral domain, using the Fourier–Legendre coefficients, so the reanalysis variables must be transformed to spectral coefficients prior to relaxation. The nudging coefficients, α, are based on empirical studies, such as those in Citation9, and are chosen to be large enough to constrain the model towards the reanalysis fields, which we assume accurately reflect the observed large-scale fields, but weak enough to permit adjustments needed to assimilate precipitation. For the experiments in this study, the coefficients are 10−4 s−1 for each variable.
A simplified analysis can be used to show that the nudging scheme can be employed to address the convergence to the correct initial condition. For a linear dynamical system
(4)
and assuming A = HAtrue, the nudging equation can be rewritten as
(5)
where the evolution equation for the error between the true state Atrue and the nudging state A
is given by
(6)
Equation (6) can be expressed as
(7)
If the eigenvalues λ from the matrix (F − αH) has the property Re{λ} < 0, then EA → 0 (i.e. A → Atrue) when t → ∞. Similar analysis can be applied for nonlinear models, implying in a similar result, if the nonlinear operator F(A) in the evolution equation follows a Lipschitz condition:
(the Lipschitz condition is a contractive mapping). A more sophisticated assimilation method can also be used, or other formulation of the nudging method (see Citation22, in this issue).
As the model is integrated forward in time during the precipitation assimilation phase, the atmospheric and land components of the model exchange fluxes, including precipitation, in order to initialize the LSM variables. Prior to the start of the assimilation, a 50-year ‘spinup’ run of the coupled land–atmosphere model was performed. More details can be found in Boisserie Citation23.
2.3. Data
The sea surface temperatures that are used in the model are from Reynolds et al. Citation24. The reanalysis used for dynamical nudging as well as the initial atmospheric conditions are the National Centers for Environmental Prediction (NCEP) Reanalysis 2 (R2) product Citation25. The NCEP R2 is the product of a comprehensive, coupled data assimilation system. The land component used in producing R2 is the Oregon State University (OSU) LSM with two soil layers: 0–10 and 10–200 cm Citation26,Citation27. R2 does not use precipitation assimilation, thus the R2 precipitation can deviate significantly from observations. To mitigate drift in the OSU land model, a correction of the top 10 cm moisture layer is made using the difference between the running 5-day mean of the model and observed rain Citation28. The NCEP R2 data set includes land surface fields and surface fluxes, including precipitation, which we do not use in the PAR procedure. However, we use these fields for a baseline comparison to evaluate the PAR results.
For precipitation, three different data sets have been used: the Tropical Rainfall Measurement Mission (TRMM) 3-hourly product Citation29, precipitation from the Global Dataset of Meteorological Forcing (GDMF) Citation30 and the Global Precipitation Climatology Center (GPCC) product Citation31. The first two data sets have been used in the assimilation for different experiments due to the higher temporal resolution (3-hourly), whereas the daily GPCC product was used as an independent validation data set.
There are relatively few locations with in situ measurements that we can use for validation. While we have validated the FSUCLM at a limited number of locations globally, in this article we only show results for the states of Oklahoma and Illinois in central US. We use in situ measurements for validation prior to Citation1995 from the Illinois Climate Network, which is available from the Global Soil Moisture Data Bank Citation32. This data set comprises 19 stations in the state of Illinois. Soil moisture is measured at 11 layers to a depth of 2 m. Details on this data set can be found in Hollinger and Isard Citation33. For validation after Citation1997, we use the Oklahoma Mesonet Citation34, which has been operational since 1995 and has 332 sensors at 102 sites placed at four depths: 5, 25, 60 and 75 cm. In order to compare model results with station observations, values at model grid points within the boundaries of each state were averaged and compared to the average of the station observations within the state.
For global evaluation of soil moisture initialization, we compare our results with the Global Soil Wetness Project 2 (GSWP2) multi-model analysis Citation35,Citation36. This multi-model analysis is the mean of 13 state-of-the-art LSMs driven by the same GDMF atmospheric near-surface analysis data set. The multi-model analysis provides a useful benchmark to evaluate the model but we emphasize that the model-derived GSWP2 analysis is not solely based on observations. It cannot be fully validated, due to lack of sufficient observations, and there are known errors in the forcing data set as well as in the models used in producing the analysis. In fact, the GDMF forcing data set itself incorporates model-derived reanalysis data. We may, however, expect the mean of the 13 models to be as good of an estimate as any that currently exists of the land surface state on a global scale. For some variables, such as runoff, there is such a large spread in the GSWP2 model results that there is low confidence in the mean value. The GSWP2 multi-model analysis has fared relatively well, however, for soil moisture when compared to a limited set of in situ data. However, the GSWP2 analysis reports only one soil moisture layer to a depth of 1.5 m. To compare with GSWP2 soil moisture, the R2 and FSUCLM soil layers are combined to give an equivalent 1.5 m layer.
3. Results
3.1. Precipitation assimilation
In order to assess the fidelity of the precipitation assimilation method, we performed an initial experiment of continuous assimilation for the month of November, 2004, using TRMM observations and NCEP R2 reanalyses. shows the average daily precipitation of TRMM observations and the results from PAR. Note that TRMM data are not available outside the 50 S to 50 N global belt. The figure shows that the model assimilated the precipitation very well. shows a snapshot of the 6 hourly precipitation from TRMM, PAR and NCEP R2. Thus, the PAR precipitation matches very closely with TRMM even on short time scales. The NCEP R2 precipitation shows more significant differences over both land and ocean. Note, for example, the large discrepancy of rainfall over the South American continent. Comparisons of PAR and NCEP R2 against GPCC observations (not shown) still reveal that PAR is substantially more correlated with observed precipitation than R2.
Figure 1. Comparison of observed TRMM rain rate (left panel) and assimilated rain rate (right panel) for the month of November 2004. Shown are averaged daily precipitation in mm day−1.
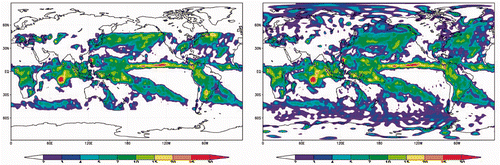
Figure 2. The 6 h accumulation of precipitation for November 2004 (15 UTC) for TRMM (upper left), PAR (upper right) and NCEP R2 (lower panel). Units are in mm/6 h.
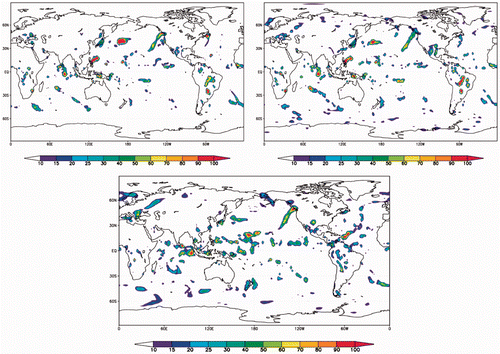
shows a time series of the area-averaged 6-hourly accumulations over part of the Eastern Pacific Inter-Tropical Convergence Zone (ITCZ), from 170 W to 120 W and 5 S to 5 N. We see again that PAR matches very closely with the TRMM observations, and much more so than R2. The R2 precipitation is unable to capture the more intense events.
3.2. Soil moisture initialization – comparison with in situ observations
Two assimilation experiments were performed with different precipitation data sets: one using TRMM precipitation for 1998–2004, and the other using GDMF precipitation for 1986–1995. The latter experiment was done in coordination with the GLACE2 multi-model comparison study in which we participated Citation37. A control run using no assimilation was also performed.
For the first experiment, we show comparisons with observations for Oklahoma, US, using data from the Oklahoma Mesonet as described above. The results are spatially averaged over the domain (100 W to 94 W, 33 N to 37 N). This represents about six grid points for the model and 110 stations for the observed. shows the top-level soil moisture and precipitation anomalies. The PAR soil moisture anomalies (fine dotted lines) follow very closely the observed anomalies (solid black lines) from the mesonet data, with a correlation of 0.65. The control run has much lower correlation, around 0.20, as expected. The lower panel shows the precipitation anomalies of PAR and Control compared with the GPCC data. We see additional confirmation that the PAR method is able to assimilate observed rain very well.
Figure 4. (a) Soil moisture (m3 m−3) and (b) precipitation (mm) for Oklahoma. Black lines are from observations (Oklahoma Mesonet and GPCC), fine dotted lines are from PAR and dash–dotted lines are from the control experiments.
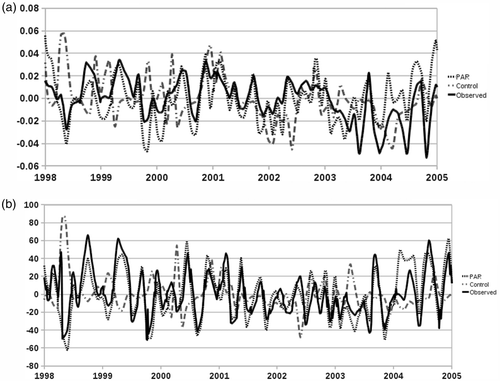
shows the vertical profile of the soil moisture climatology for the years 1998–2004. The PAR profile is drier than observations, similar in magnitude to the control run. However, the pattern of the PAR climatology is much closer to observations. As discussed in Section 1, LSMs can have large biases when compared to observations. We do not expect the coupled assimilation to remove much bias, but rather improve representation of anomalies. Nevertheless, we see some improvement in the seasonal bias with PAR.
Figure 5. Monthly soil moisture climatology profile from observations (top panel), PAR (middle panel) and Control experiment (lower panel). Soil moisture units are m3 m−3 and depth is in cm.
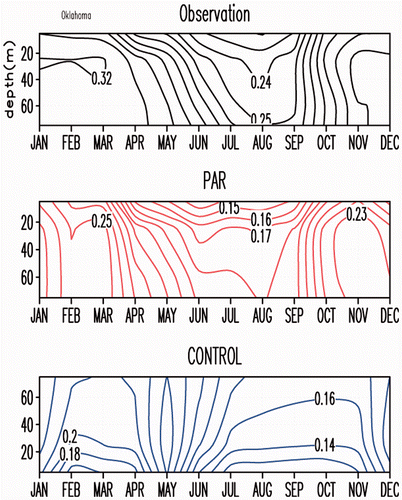
shows the soil moisture anomalies for four depth layers. The PAR method tends to capture the monthly anomalies, including magnitude of the anomaly, rather well for all layers. There appears to be some discrepancies starting around 2003–2004 that need to be further investigated.
Figure 6. Soil moisture anomaly at four layer depths for Oklahoma. Observations are black lines, PAR fine dotted lines and Control dash–dotted lines. Units are in m3 m−3. Vertical grid spacing is 0.1 for the top layer and 0.05 for the bottom three layers. Bold horizontal lines are zero.
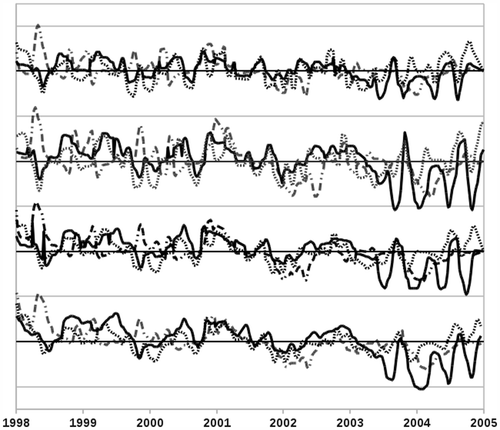
We pointed out in Section 1 that one advantage to using a coupled assimilation is that the surface fluxes, including precipitation, will be physically consistent. One of its verifications is shown in , which shows the net short-wave radiation flux. The correlation of the PAR radiation flux and observed satellite estimate is 0.86. For the control run, the correlation is −0.05. Short-wave radiation is an indicator of cloudiness. and together show that the model captures the proper rainfall and associated cloud impact. An inaccurate radiation flux could lead, for example, to excessive evaporation, drying the top soil layer before the water can penetrate to deeper levels.
Figure 7. Net short-wave radiation flux anomaly (W m−2) for Oklahoma. Observation is the black line, PAR is the fine dotted line and Control is the dash–dotted line.
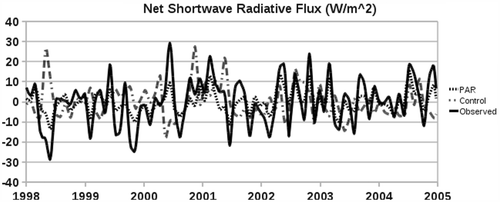
The second assimilation experiment covered the time period of 1986–1995. For this experiment, GDMF precipitation was used since TRMM data are not available prior to 1998. This time period includes two notable extreme events in the Central US: the 1988 drought and 1993 flood. Figures 3.9 and 3.10 of Boisserie Citation23 shows vertical soil moisture profiles for observations in Illinois, NCEP R2, PAR and Control. These results are averaged over the domain (92 W to 87.3 W, 37 N to 43 N). This is six grid points for the model and 19 stations for the observed. Overall, the PAR simulates the observed anomalies very well compared to R2. The 1988 and 1993 events are reasonably captured, though the anomalies are weaker than observed. The PAR has the correct sign of the anomaly for most months during the assimilation, whereas R2 frequently has the wrong sign. Also, for R2, the sign of the anomaly for the top layer is often different from the deep layer. We are not sure why this happens, but may be due to the correction method mentioned earlier to reduce drift in the OSU land model.
3.3. Soil moisture initialization – comparison with global estimates
For a global evaluation of the PAR soil initialization, we compare the results with the multi-model analysis of the GSWP2. shows the boreal summer and winter mean average soil moisture content for GSWP2, NCEP R2, PAR and Control for 1986–1995. The NCEP R2 appears to be notably wetter than GSWP2 for both seasons, especially in the desert regions. The PAR results are much closer to the GSWP2 results, and agree very well in both the magnitude and spatial pattern. The PAR results are perhaps somewhat drier, similar to what we noted in the in situ comparisons described above. The control run also appears to be drier than GSWP2 in nondesert regions, indicating that the possible dry bias of the FSUCLM is not due to the precipitation assimilation method. The dry bias may be due to the CLM2. A study by Lawrence et al. Citation39 found that the CLM2 has incorrect partitioning of evapo-transpiration, and that by increasing transpiration and infiltration and decreasing soil evaporation the bias can greatly reduced. A new version of the land model (CLM3) incorporates these corrections to address this problem.
Figure 8. Average soil moisture content from 1986 to 1995 for: (a) boreal summer and (b) boreal winter months for top 1.5 m depth. Units are in mm.
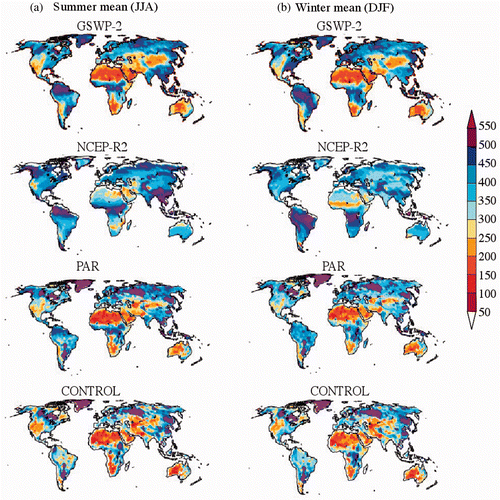
The results for surface runoff were more varied (see Figure 3.14 of Citation24). The NCEP R2 has a number of locations with very high runoff, exceeding 200 mm month−1, as compared to both GSWP2 and PAR. As mentioned earlier, there is a lot of spread of runoff estimates amongst the LSMs that comprise the GSWP2 analysis; therefore, the best that we can conclude is that the PAR results are not inconsistent with the GSWP2.
We examined the average surface evaporation. The PAR results are much closer to GSWP2 than NCEP R2, and in good agreement overall. R2 has generally higher evaporation than GSWP2 and PAR throughout most nondesert regions of the globe, and in some cases the difference is quite substantial, such as in the eastern US in summer. It is not clear why the evaporation in R2 is significantly higher, though we note that, as shown in , R2 has an overall higher soil moisture content, which could be a contributing factor. It is also possible that the drift correction that is applied to the land model in R2, described in Section 2.3, may induce excessive evaporative fluxes.
We computed the temporal monthly anomaly correlations for NCEP R2, PAR and Control against the GSWP2 analysis of soil moisture, surface runoff and evaporation. For all three fields, the PAR results are more highly correlated overall to the GSWP2 analysis than NCEP R2. For soil moisture, PAR is generally well correlated with GSWP2, except at high latitudes. The disagreement at the northern high latitudes may be due to the treatment or diagnosis of snow cover, and needs further investigation. The correlation for runoff between PAR and GSWP2 is moderate to high only in localized regions. While there may be relatively large differences in the magnitude of the runoff between PAR and GSWP2, the temporal anomaly correlations show that the results are generally consistent. There is very good correlation of evaporation between PAR and GSWP2, except in the tropical land regions. The lack of significant correlation in the tropical land regions may be partly due to small variations in evaporation, as a result of the soil being nearly always adequately moist, and thus the anomaly variations may be dominated by noise. The control run shows no significant correlation, as might be expected since there was no assimilation.
3.4. Impact of initialization on forecasts
FSU participated in the GLACE2 model inter-comparison to study the impacts of soil initialization on short-term climate (2 weeks to 2 months) forecasts. This international experiment involved models from major centres including the Center for Ocean-Land-Atmosphere studies, the European Center for Medium-range Weather Forecasts (ECMWF), NCAR, The Geophysical Fluid Dynamics Laboratory, NCEP and the Koninklijk Nederlands Meteorologisch Instituut. In this experiment, two series of runs were performed for each model; one with soil moisture initialization and one without. Forecasts of up to 2 months were made starting at the 1st and 15th of each month between June and August of each year between 1986 and 1995. Thus, there is a total of 60 forecast starting dates. We performed 10 ensemble members for each forecast starting date, for a total of 600 forecast runs. Each ensemble was created by shifting the member start time ahead or behind by multiples of 6 h centred around the forecast start date. Forecast skill and criteria are defined by GLACE2 protocol. The metric used is based on the temporal Pearson correlation between the observed and model anomaly time series. Observed and model anomalies are defined as the deviation from the temporal mean value of the forecast variable over the corresponding target forecast period for all years in the experimental configuration (1986–1995). A comparison of two model forecasts can be evaluated in terms of the difference in the explained variance, the square of the Pearson correlation.
We show in , the temperature anomaly correlations for the ensemble mean of the forecasts using soil initialization via PAR and Control runs in which unassimilated initial conditions were used. The right panel in the figure shows the difference in the explained variance between the initialized and control runs. For all forecast periods, the PAR results show increased skill in the temperature anomaly prediction. This result is reasonable since surface temperature is determined from surface fluxes, and soil initialization improves the surface fluxes. The persistence of soil moisture anomalies leads to increased predictability of temperature.
Figure 9. Forecast skill as measured by anomaly correlation for PAR (left panel), Control (middle panel) and difference in explained variance between PAR and Control (right panel).
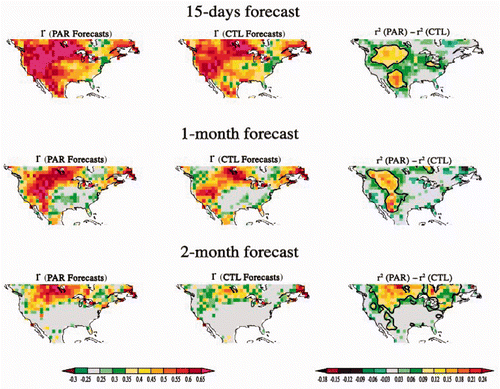
The case for precipitation forecasts is quite different. We did not see any significant increase in skill for precipitation forecasts using the above metrics for any forecast length (not shown). The same result was reported for all GLACE2 individual models. The link between precipitation and soil moisture anomalies is much weaker than with temperature, leading to very little increase in predictability. Whether this is a model deficiency, common to all participant models, or an inherent predictability barrier in nature remains to be determined. We do note, however, that the precipitation forecasts for 1988 and 1993, the two main extreme events over the period, were improved using soil initialization Citation24. Thus, it may turn out that there is only measurable predictability of precipitation in the case of extreme soil moisture anomalies. This would be a positive result, since these situations are of greater societal concern.
4. Conclusion
We have developed a simple but an effective soil initialization scheme for the FSUCLM climate model. The method indirectly initializes soil moisture while assimilating observed precipitation in the atmospheric component of the model while coupled to the land surface component. The coupled initialization ensures that the LSM is in balance with the atmospheric component, eliminating the need for any correction that is typical of off-line methods. The method is computationally efficient, with virtually no overhead compared to running the model in forecast mode. Yet, despite its simplicity, the PAR method assimilates the observed precipitation to a very high degree, as we have shown in this article. It is unlikely that any other assimilation method for precipitation would produce model precipitation that is measurably closer to the observed precipitation. A more sophisticated assimilation scheme could produce a superior representation of the overall atmospheric state, but it is not clear that the resulting fluxes at the surface will be significantly better for land initialization, especially given that precipitation is the predominant flux for determining soil moisture. We have shown a representative example where the short-wave radiative flux, which can indicate cloudiness, is very close to observations, confirming physical consistency of the fluxes driving the LSM during the assimilation phase.
Comparison with in situ data for Illinois and Oklahoma shows that the PAR method reproduces the soil moisture anomalies reasonably well, and better than R2. While biases are still present, the anomaly patterns are well correlated to observations. For global evaluation, we compared our results with the GSWP2 multi-model analysis, and found good correlation with PAR. The PAR results were more consistent overall to GSWP2 than R2.
The use of land surface initialization is becoming more prevalent in longer-term operational forecasting systems, such as the ECMWF-integrated forecast system Citation39, Canadian Centre for Climate Modelling and Analysis CanCCM3 Citation40 and more recently in the NCEP Climate Forecast System version 2 Citation41, as well as in major research models. The GLACE2 experiment was designed as a systematic and comprehensive way to evaluate the impact of land surface initialization on longer-range (subseasonal) forecasts Citation37. The FSUCLM climate model was unique among the model participants of GLACE2 in that a coupled land–atmosphere assimilation method was used.
Coordinated forecasts made as part of our participation in the GLACE2 experiment show that the PAR method leads to measurably improved predictability in the surface temperature anomaly for 2-week and 1 - and 2-month forecasts. The degree of improvement and location of increased skill were comparable among most of the GLACE2 model results. The increase in skill appears to correspond to the so-called ‘hotspots’ identified in the GLACE1 experiment Citation8,Citation42. However, there appears to be some impact in other locations as well, in both our results and the other GLACE2 model experiments (see also Citation37). Thus, further study is need to fully attribute the increase in skill.
There was not much improvement in the precipitation forecasts, as was the case for all participants in the GLACE2 experiment. This was also the case for all forecast lead times. Improving prediction of precipitation remains a major challenge. However, there appears to be some evidence that prediction of extreme events related to large soil moisture anomalies may be improved in the FSUCLM Citation23. This was also noted in Citation38 for the ensemble of GLACE2 models. These results are based on a preliminary analysis of the GLACE2 results. A future paper will evaluate the results in more detail.
Due to the large differences in the participating models, such as in the resolution, physics, it is not possible to determine whether there is any superiority in any particular data assimilation method used. A more controlled experiment is needed to isolate the impact of a particular assimilation method. Nevertheless, these preliminary results show that the PAR method is a viable method that can be readily implemented in other modelling systems.
Additional improvements in the soil moisture initialization may be possible by using higher horizontal resolution. Higher resolution would allow more accurate specification of the soil and other surface characteristics. Precipitation variability would also be more realistic. The PAR method has been shown to work well for precipitation assimilation in regional models Citation21 at around 50 km and higher resolution.
Acknowledgement
This research was partially supported via a USDA/NIFA-funded collaborative EaSM project (grant 2011-0828). This work was also partially supported by the Korea Institute of Atmospeheric Prediction Systems.
References
- Shukla, J, and Mintz, Y, 1982. Influence of land-surface evapotranspiration on the Earth's climate, Science 215 (1982), pp. 1498–1500.
- Rind, D, 1982. The influence of ground moisture conditions in North America on summer climate as modeled in the GISS GCM, Mon. Weather Rev. 110 (1982), pp. 1487–1494.
- Yeh, TC, Wetherald, RT, and Manabe, S, 1984. The effect of soil moisture on the short-term climate and hydrology change: A numerical experiment, Mon. Weather Rev. 112 (1984), pp. 474–490.
- Sud, YC, and Fennessy, M, 1984. Influence of evaporation in semi-arid regions on the July circulation: A numerical study, J. Climatol. 4 (1984), pp. 383–398.
- Fennessy, MJ, and Shukla, J, 1999. Impact of initial soil wetness on seasonal atmospheric predictions, J. Clim. 12 (1999), pp. 3167–3180.
- Koster, RD, Suarez, MJ, and Heiser, M, 2000. Variance and predictability of precipitation at seasonal-to-interannual timescales, J. Hydrometeorol. 1 (2000), pp. 26–46.
- Hong, S-Y, and Kalnay, E, 2000. Role of sea-surface temperature and soil-moisture feedback in the 1998 Oklahoma–Texas drought, Nature 408 (2000), pp. 842–844.
- Koster, RD, Guo, Z, Dirmeyer, PA, Bonan, G, Chan, E, Cox, P, Davies, H, Gordon, CT, Kanae, S, Kowalczyk, E, Lawrence, D, Liu, P, Lu, C-H, Malyshev, S, McAvaney, B, Mitchell, K, Mocko, D, Oki, T, Oleson, KW, Pitman, A, Sud, YC, Taylor, CM, Verseghy, D, Vasic, R, Xue, Y, and Yamada, T, 2006. GLACE: The global land–atmosphere coupling experiment. Part I: Overview, J. Hydrometeorol. 7 (2006), pp. 590–610.
- Kim, B-M, Cocke, S, Lim, G-H, and Oh, J-H, 2007. Assimilation of TRMM rain rate into global analysis and its impact on the summer mean circulation over tropics, J. Korean Meteorol. Soc. 43 (4) (2007), pp. 397–409.
- Cocke, S, and LaRow, TE, 2000. Seasonal Prediction using a Regional Spectral Model embedded within a Coupled Ocean Atmosphere Model, Mon. Weather Rev. 128 (2000), pp. 689–708.
- Kiehl, JT, Hack, JJ, Bonan, GB, Boville, BA, Williamson, DL, and Rasch, PJ, 1998. The National Center for Atmospheric Research Community Climate Model: CCM3, J. Clim. 11 (1998), pp. 1131–1149.
- Hack, JJ, 1994. Parameterization of moist convection in the National Center for Atmospheric Research Community Climate Model (CCM2), J. Geophys. Res. 99 (1994), pp. 5551–5568.
- Slingo, JM, 1987. The development and verification of a cloud prediction scheme for the ECMWF model, Q.J. R. Meteorol. Soc. 113 (1987), pp. 899–927.
- Ramanathan, V, and Downey, P, 1986. A nonisothermal emissivity and absorptivity formulation for water vapor, J. Geophys. Res. 91 (1986), pp. 8649–8666.
- Briegleb, BP, 1992. Delta-Eddington approximation for solar radiation in the NCAR Community Climate Model, J. Geophys. Res. 97 (1992), pp. 7603–7612.
- Holtslag, AAM, and Boville, BA, 1993. Local versus nonlocal boundary-layer diffusion in a global climate model, J. Clim. 6 (1993), pp. 1825–1842.
- Bonan, GB, Oleson, KW, Vertenstein, M, Levis, S, Zeng, X, Dai, Y, Dickinson, RE, and Yang, Z-L, 2002. The land surface climatology of the Community Land Model coupled to the NCAR Community Climate Model, J. Clim. 15 (2002), pp. 3123–3149.
- Shin, DW, Cocke, S, LaRow, TE, and O’Brien, JJ, 2005. Seasonal surface air temperature and precipitation in the FSU climate model coupled to the CLM2, J. Clim. 18 (2005), pp. 3217–3228.
- Boisserie, M, Shin, DW, LaRow, TE, and Cocke, S, 2006. Evaluation of soil moisture in the Florida State University climate model-National Center for Atmospheric Research community land model (FSU-CLM) using two reanalysis (R2 and ERA40) and in situ observation, J. Geophys. Res. 111 (2006), pp. 1–14, D08103.
- Krishnamurti, T, Ingles, K, Cocke, S, Pasch, R, and Kitade, T, 1984. Details of low latitude medium range numerical weather prediction using a global spectral model. Part II: Effect of orography and physical initialization, J. Meteorol. Soc. Jpn 62 (1984), pp. 613–649.
- Nunes, AM, and Cocke, S, 2004. Implementing a physical initialization procedure in a regional spectral model: Impact on the short-range rainfall forecasting over South America, Tellus A, 56 (2) (2004), pp. 125–140.
- Auroux, D, and Bansart, P, 2012. J. Blum, An evolution of the Back and Forth Nudging for geophysical data assimilation: application to Burgers equation and comparisons, Inverse Probl. Sci. Eng. (2012), doi: 10.1080/17415977.2012.712528.
- Boisserie, M, , Generation of a realistic soil moisture initialization and its potential impact on seasonal forecasting of continental precipitation and air temperature, Ph.D. diss., Florida State University, 2010.
- Reynolds, RW, Rayner, NA, Smith, TM, Stokes, DC, and Wang, W, 2002. An improved in situ and satellite SST analysis for climate, J. Clim. 15 (2002), pp. 1609–1625.
- Kanamitsu, M, Ebiszaki, W, Woolen, J, Yang, S-K, Hnilo, JJ, Fiorinim, M, and Potter, GL, 2002. NCEP-DOE AMP-II reanalysis (R2), Bull. Am. Meteorol. Soc. 83 (2002), pp. 1631–1648.
- Pan, H-L, and Mahrt, L, 1987. Interaction between soil hydrology and boundary-layer development, Boundary Layer Meteorol. 38 (1987), pp. 185–202.
- Pan, H-L, 1990. A simple parameterization scheme of evapotranspiration over land for the NMC medium-range forecast model, Mon. Weather Rev. 118 (1990), pp. 2500–2512.
- Kanamitsu, M, Lu, C-H, Schemm, J, and Ebisuzaki, W, 2003. The predictability of soil moisture and near surface temperature in hindcasts of NCEP Seasonal Forecast Model, J. Clim. 16 (2003), pp. 510–521.
- Huffman, GJ, Adler, RF, Bolvin, DT, Gu, G, Nelkin, EJ, Bowman, KP, Stocker, EF, and Wolff, DB, 2006. The TRMM multi-satellite precipitation analysis: Quasi-global, multi-year, combined-sensor precipitation estimates at fine scale, J. Hydrometeorol. 8 (2006), pp. 38–55.
- Sheffield, J, Goteti, G, and Wood, EF, 2006. Development of a 50-year high-resolution global dataset of meteorological forcings for land surface modeling, J. Clim. 19 (13) (2006), pp. 3088–3111.
- Huffman, GJ, Adler, RF, Morrissey, M, Bolvin, DT, Curtis, S, Joyce, R, McGavock, B, and Susskind, J, 2001. Global precipitation at one-degree daily resolution from multi-satellite observations, J. Hydrometeorol. 2 (2001), pp. 36–50.
- Robock, A, Vinnikov, KY, Srinivasan, G, Entin, JK, Hollinger, SE, Speranskaya, NA, Liu, S, and Namkhai, A, 2000. The Global Soil Moisture Data Bank, Bull. Am. Meteorol. Soc. 81 (2000), pp. 1281–1299.
- Hollinger, SE, and Isard, SA, 1994. A soil moisture climatology of Illinois, J. Clim. 7 (1994), pp. 822–833.
- Brock, FV, Crawford, KC, Elliott, RL, Cuperus, GW, Stadler, SJ, Johnson, HL, and Eilts, MD, 1995. The Oklahoma Mesonet: A technical overview, J. Atmos. Oceanic Technol. 12 (1995), pp. 5–19.
- Dirmeyer, PA, Gao, X, and Oki, T, , The Second Global Soil Wetness Project, GSWP-2, IGPO Publication No. 37, International GEWEX Publication Office, Silver Spring, MD, pp. 65, 2002. Available at http://www.iges.org/gswp/publications.html.
- Dirmeyer, PA, Gao, X, Zha, M, Guo, Z, Oki, T, and Hanasaki, N, , The second Global Soil Wetness Project (GSWP-2): Multi-model analysis and implications for our perception of the land surface, COLA Tech. Rep. 185, Center for Ocean-Land-Atmosphere Studies, Calverton, MD, pp. 46, 2005.
- Koster, RD, Mahanama, SP, Yamada, TJ, Balsamo, G, Berg, AA, Boisserie, M, Dirmeyer, PA, Doblas-Reyes, FJ, Drewitt, G, and Gordon, CT, 2010. Contribution of land surface initialization to subseasonal forecast skill: First results from a multi-model experiment, Geophys. Res. Lett. 37 (2) (2010), p. L02402.
- Lawrence, DM, Thornton, PE, Oleson, KW, and Bonan, GB, 2007. The partitioning of evapotranspiration into transpiration, soil evaporation, and canopy evaporation in a GCM: Impacts on land-atmosphere interaction, J. Hydrometeorol. 8 (2007), pp. 862–880.
- Vitart, F, Buizza, R, Balmaseda, MA, Balsamo, G, Bidlot, J-R, Bonet, A, Fuentes, M, Hofstadler, A, Molteni, F, and Palmer, TN, 2008. The new VAREPS-monthly forecasting system: a first step towards seamless prediction, Q.J. R. Meteorol. Soc. 134 (2008), pp. 1789–1799.
- Scinocca, JF, McFarlane, NA, Lazare, M, Li, J, and Plummer, D, 2008. The CCCma third generation AGCM and its extension into the middle atmosphere, Atmos. Chem. Phys. 8 (2008), pp. 7055–7074.
- Saha, S, Moorthi, S, Pan, H-L, Wu, X, Wang, J, Nadiga, S, Tripp, P, Kistler, R, Woollen, J, Behringer, D, Liu, H, Stokes, D, Grumbine, R, Gayno, G, Wang, J, Hou, Y-T, Chuang, H-Y, Juang, HMH, Sela, J, Iredell, M, Treadon, R, Kleist, D, van Delst, P, Keyser, D, Derber, J, Ek, M, Meng, J, Wei, H, Yang, R, Lord, S, van Den Dool, H, Kumar, A, Wang, W, Long, C, Chelliah, M, Xue, Y, Huang, B, Schemm, J-K, Ebisuzaki, W, Lin, R, Xie, P, Chen, M, Zhou, S, Higgins, W, Zou, C-Z, Liu, Q, Chen, Y, Han, Y, Cucurull, L, Reynolds, RW, Rutledge, G, and Goldberg, M, 2010. The NCEP climate forecast system reanalysis, Bull. Am. Meteorol. Soc. 91 (2010), pp. 1015–1057.
- Koster, RD, Dirmeyer, PA, Guo, Z, Bonan, G, Chan, E, Cox, P, Davies, H, Gordon, T, Kanae, S, Kowalczyk, E, Lawrence, D, Liu, P, Lu, S, Malyshev, S, McAvaney, B, Mitchell, K, Oki, T, Oleson, K, Pitman, A, Sud, Y, Taylor, C, Verseghy, D, Vasic, R, Xue, Y, and Yamada, T, 2004. Regions of strong coupling between soil moisture and precipitation, Science 305 (2004), pp. 1138–1140.