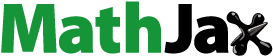
Abstract
This article deals with an inverse problem of determining the space-dependent diffusion coefficient and the source coefficient simultaneously in the multi-term time fractional diffusion equation (TFDE in short) using measurements at one inner point. From a view point of optimality, solving the inverse problem is transformed to minimize an error functional with the help of the solution operator from the unknown to the additional observation. The solution operator is nonlinear but it is of Lipschitz continuity by which existence of a minimum to the error functional is obtained using Sobolev embedding theorems. The homotopy regularization algorithm is introduced to solve the simultaneous inversion problem based on the minimization problem, and numerical examples are presented. The inversion solutions give good approximations to the exact solutions demonstrating that the homotopy regularization algorithm is efficient for the simultaneous inversion problem arising in the multi-term TFDE.
1. Introduction
The fractional diffusion equations have played an important role in modelling of the anomalous diffusion phenomena and in the theory of the complex systems (see e.g. [Citation1–Citation6], and references therein) during the last few decades. The so-called time fractional diffusion equation arises when replacing the first-order time derivative by a fractional derivative of order with
. On the attempting to describe some real processes with the equations of the fractional derivative, several researches confronted with the situation that the time fractional derivative from the corresponding model equations did not remain constant and changed, say, in the interval from 0 to 1, from 1 to 2 or even from 0 to 2. To manage these phenomena, several approaches were suggested. One of them introduces the fractional derivatives of the variable order, i.e. the derivatives with the order that can change with the time or/and depending on the spatial coordinates (see e.g. [Citation7,Citation8]), and the other way is to employ the multi-term time fractional diffusion equations. Let
where
, and denote
for
, the 1D multi-term time fractional diffusion equation with space-dependent diffusion coefficient is given as
(1.1)
(1.1)
where denotes the state variable at space point x and time t,
is the space-dependent diffusion coefficient, s(x, t) is a source term, and
denotes the principal fractional order, and
are the multi-term fractional orders of the derivatives, which satisfy the condition of order:
(1.2)
(1.2)
and are positive constants, and all of the above time-fractional derivatives are defined in the meaning of Caputo from the left-hand side, which for example for
the fractional derivative of a function
at
is given as
(1.3)
(1.3)
where is the usual Gamma function. See, e.g. Podlubny [Citation9] and Kilbas et al. [Citation10] for the definition and properties of the Caputo’s derivative.
From the past few years, there are quite a few researches on the forward problem of multi-term time fractional diffusion equations like Equation (Equation1.1(1.1)
(1.1) ), for instance, see [Citation11,Citation12] for the maximum principles, see [Citation13–Citation15] for the uniqueness and existence results, see [Citation16–Citation19] for the analytic solutions, and see [Citation20,Citation21] for numerical solutions of finite difference method, and recently see [Citation22] for the finite element solution, etc.
However, for real problems, the part of the boundary, or the initial data, or the diffusion coefficient, or the source term cannot be obtained directly and we have to determine them by some additional measurements, which yields to inverse problems arising in the fractional diffusion models. There are still some researches on inverse problems in the single-term time fractional diffusion equation, see, e.g. Murio [Citation23], Liu and Yamamoto [Citation24], Sakamoto and Yamamoto [Citation25], Tuan [Citation26], Jin [Citation27], Yamamoto [Citation28], Luchko et al. [Citation29], Miller and Yamamoto [Citation30] and Wei et al. [Citation31]. Recently, see Jin et al. [Citation32] for a tutorial review on inverse problems for anomalous diffusion processes, and see Liu et al. [Citation33] for the research on recovering a time-dependent boundary source using nonlocal measurement data, and see Zhang [Citation34] for an inverse problem of determining a time-dependent diffusion coefficient in the time fractional diffusion equation using monotonicity of the input–output operator and fixed point method, and see Wei et al. [Citation35] for the research on uniqueness and stability for determining the time-dependent source term also in the one-term time fractional diffusion equation.
To our knowledge, there are few literatures concerned with simultaneous inversion problems, especially for the inverse problems in the multi-term time fractional differential equations. Cheng et al. [Citation36] proved the uniqueness result for a simultaneous inverse problem of identifying the diffusion coefficient and the fractional order in the one-term TFDE, which is the first work on simultaneous inverse problems for fractional diffusion equations, and Li et al. [Citation37] considered with the same inverse problem but with more general conditions and numerical inversions. Li and Yamamoto [Citation38] studied an inverse problem of identifying the multiple fractional orders in the multi-term TFDE, and they gave uniqueness of the solution to the inverse problem using Laplace transform and analytical method, and Li et al. [Citation39] considered the similar model and they proved the uniqueness of determining the fractional orders, the number of the fractional terms and the spatially varying coefficient simultaneously. On the other hand, Rodrigues, Orlande and Dulikravich [Citation40] ever considered a simultaneous inversion problem but in the integer-order diffusion equation by the conjugate gradient method, Zhang et al. [Citation41] considered a simultaneous inversion problem for the space-dependent diffusion coefficient and the source coefficient in the one-term TFDE, and they gave quite a few numerical inversions using the homotopy regularization algorithm, and recently Jia et al. [Citation42] studied a simultaneous inversion problem of determining the diffusion coefficient and the source term in 1D space fractional advection diffusion equation by the optimal perturbation regularization algorithm.
In this paper, by setting in Equation (Equation1.1
(1.1)
(1.1) ), where the time-decay factor
is known, we also deal with the simultaneous inversion problem of determining the space-dependent diffusion coefficient D(x) and the space-dependent source magnitude f(x) but for the multi-term TFDE. It is noticeable that the simultaneous inversion problem here for identifying the space-dependent diffusion coefficient and the source function becomes severely ill-posed as compared with the previous problems since the model is difficult to simulate and the two unknowns are both variable. From a view point of optimality, solving the inverse problem is transformed to minimize an error functional based on the solution operator. Since the solution operator from the unknowns to the additional observations is nonlinear, it is not convex for the error functional, however, we can prove its the Lipschitz continuity by which existence of a minimum to the error functional is obtained using Sobolev embedding theorems. Furthermore, the homotopy regularization algorithm is introduced based on the error functional, and numerical inversions with random noisy data are performed. It is noted that the inversion algorithm can also be employed to determine the space-dependent diffusion coefficient and time-dependent decay factor simultaneously in the case that the spacewise-dependent source magnitude is known.
The rest of the paper is organized as follows. In Section 2, the forward problem for the multi-term TFDE is discussed, and the Lipschitz continuity of the solution operator is proved, and a finite difference scheme to the forward problem is given. In Section 3, the simultaneous inversion problem of determining the diffusion coefficient and the source magnitude by the additional data at one inner point is set forth, and existence of an minimum to the error functional is obtained by the Sobolev embedding theorems. In Section 4, the homotopy regularization algorithm is introduced based on the minimization problem, and numerical inversions are presented, and conclusions are given in Section 5.
2. The forward problem
By we denote the closure of the space domain
. Consider the initial boundary value problem composed by Equation (Equation1.1
(1.1)
(1.1) ) with the initial condition
(2.1)
(2.1)
and the homogeneous Neumann boundary condition(2.2)
(2.2)
For convenience, we give some general settings and notations used in this paper.
Let be a usual
-space with the inner product
and the norm
, and
and
(
) denote the Sobolev spaces (see, e.g. Adams [Citation43]). For
and a Banach space X, we say that an abstract function
provided
For example:
and
2.1. Lipschitz continuity of the solution operator
An inverse problem is always related with a forward problem, and property of the solution to the forward problem is essential to the research of the corresponding inverse problem. On regularity of the solution to the forward problem of TFDEs, we refer to [Citation13,Citation25] for the case of Dirichelet boundary conditions and refer to [Citation35] for the case of Neumann boundary condition in the one-term TFDE. Here for the forward problem (Equation1.1(1.1)
(1.1) ), (Equation2.1
(2.1)
(2.1) )–(Equation2.2
(2.2)
(2.2) ), we give the following lemma to show well-posedness of the solution.
Lemma 2.1:
Under the assumptions in Section 1 for Equation (Equation1.1(1.1)
(1.1) ), also assume that the initial function
and the source term
. Then there is a unique solution
to the forward problem (Equation1.1
(1.1)
(1.1) ), (Equation2.1
(2.1)
(2.1) ) and (Equation2.2
(2.2)
(2.2) ), and there exists a constant
depending on
such that
(2.3)
(2.3)
Noting the properties of the multinomial Mittag–Leffler function, the proof can be completed with a similar method as used in [Citation13,Citation35].
Furthermore, let , and denote
as the solution to the forward problem (Equation1.1
(1.1)
(1.1) ), (Equation2.1
(2.1)
(2.1) ) and (Equation2.2
(2.2)
(2.2) ) for given
and suitable D(x) and f(x). It is obvious that the solution operator
is nonlinear, however, the Lipschitz continuity of the solution operator can be proved by which existence of a minimum to the minimization problem of the error functional is obtained.
Theorem 2.2:
Under the assumptions in Lemma 2.1, and assume that the source term where
is given and satisfies
, and
are the two solutions to the forward problem corresponding to the diffusion coefficients
,
on
and the source functions
. Then it follows that
(2.4)
(2.4)
where is a constant depending only on
, and
.
Let , and U satisfies the equation
(2.5)
(2.5)
where
Denote , and rewrite
as
(2.6)
(2.6)
Noting that , there hold
and
, and there exists a constant
independent of choices of
and
such that
(2.7)
(2.7)
Meantime there hold and
due to
. Thus we have
, and there holds by Lemma 2.1
(2.8)
(2.8)
Hence orth, we get(2.9)
(2.9)
Together with (Equation2.8(2.8)
(2.8) ) and (Equation2.6
(2.6)
(2.6) ) we have
(2.10)
(2.10)
which can end the proof of this theorem.
If the time-dependent decay factor is unknown and the source magnitude
is given, we can get the following assertion with the same method as used in the above.
Corollary 2.3:
Under the assumptions in Lemma 2.1, and assume that the source term where
is given and satisfies
, and
are the two solutions to the forward problem corresponding to the diffusion coefficients
,
on
and the time-dependent decay factor
. Then it follows that
(2.11)
(2.11)
where is a constant depending only on
, and
.
2.2. The difference scheme to the forward problem
In this subsection, an implicit finite difference scheme is set forth to solve the forward problem (Equation1.1(1.1)
(1.1) ), (Equation2.1
(2.1)
(2.1) )–(Equation2.2
(2.2)
(2.2) ) numerically. Although the similar difference scheme has been discussed in the previous works, see e.g. [Citation20,Citation21], we still give it here for completeness.
Firstly, discretizing the space domain by and the time domain by
, and the fractional derivatives by the difference discretization, we have
(2.12)
(2.12)
and(2.13)
(2.13)
where is the space mesh step and
is the time mesh step.
Next, we discretize the derivative in Equation (Equation1.1
(1.1)
(1.1) ) by the general two-order centre difference scheme, which is given as
(2.14)
(2.14)
Denoting ,
,
,
, and substituting (Equation2.12
(2.12)
(2.12) )–(Equation2.14
(2.14)
(2.14) ) into Equation (Equation1.1
(1.1)
(1.1) ), and multiplying by
, we get
(2.15)
(2.15)
for .
Denote
and(2.16)
(2.16)
for , and
(2.17)
(2.17)
for . Thus we have by ignoring the remainder terms
and the initial boundary value conditions are discretized as
For , let
and
and , where
,
, are defined by
(2.18)
(2.18)
and(2.19)
(2.19)
and(2.20)
(2.20)
for ,
. Then we get the implicit finite difference scheme in matrix form
(2.21)
(2.21)
Lemma 2.4:
The coefficient matrix B defined by (Equation2.18(2.18)
(2.18) )–(Equation2.20
(2.20)
(2.20) ) is strictly diagonally dominant, and the difference scheme (Equation2.21
(2.21)
(2.21) ) has only one solution.
Noting that and
for
, there are
(2.22)
(2.22)
and(2.23)
(2.23)
Similarly, for we have
(2.24)
(2.24)
Combining (Equation2.22(2.22)
(2.22) ), (Equation2.23
(2.23)
(2.23) ) with (Equation2.24
(2.24)
(2.24) ) there holds
(2.25)
(2.25)
which implies that the matrix B is strictly diagonally dominant, and the difference scheme (Equation2.21(2.21)
(2.21) ) has unique solution.
Furthermore, we can prove the implicit difference scheme (Equation2.21(2.21)
(2.21) ) is of unconditional stability and convergence with a similar method as used in [Citation20,Citation21,Citation44].
Lemma 2.5:
The difference scheme (Equation2.21(2.21)
(2.21) ) is unconditional stable and convergent to the exact solution of the forward problem for any finite time
.
By Lemma 2.4 there hold and
for
. With a completely similar method as used in [Citation20], there holds
(2.26)
(2.26)
where is the spectral radius of the coefficient matrix B, and
. Thus the unconditional stability and convergence of the finite difference scheme (Equation2.21
(2.21)
(2.21) ) can be proved based on the estimation (Equation2.26
(2.26)
(2.26) ).
In the follows we discuss a simultaneous inversion problem and its numerical inversion based on the difference solution given by (Equation2.21(2.21)
(2.21) ).
3. The inverse problem and inversion algorithm
3.1. The inverse problem
As stated in Section 2.1, we assume that the source term is separable in variable, i.e. there is , where
is known which denotes the time-dependent decay factor in the transport process, and f(x) is the space-dependent source magnitude which is unknown. Moreover, the diffusion coefficient D(x) is also unknown. Thus, a simultaneous inversion problem is encountered with if some additional measurements on the solution can be obtained. Let
be the measured point, and the additional measurement at
be given as
(3.1)
(3.1)
The simultaneous inversion problem is formulated that is to determine the diffusion coefficient D(x) and the source term f(x) based on the forward problem together with the overposed condition (Equation3.1(3.1)
(3.1) ).
We denote as the admissible space for the unknowns D(x) and f(x), and define
(3.2)
(3.2)
as the admissible set for the pairs of solutions of the inverse problem, where E is any positive constant.
From the view point of optimality, solving an inverse problem can be transformed to minimizing an error functional between the output and the additional measurement. Also denote u(D, f)(x, t) as the unique solution to the forward problem corresponding to any given input , we get the input–output solution’s operator
. Then an operator from the unknowns
to the overposed measurements, which is called the observation operator, can be defined by
(3.3)
(3.3)
and the simultaneous inversion problem is transformed to solve the operator equation (Equation3.3(3.3)
(3.3) ). For solving the inverse problem, we define an error functional based on the input–output mapping by
(3.4)
(3.4)
and solving the simultaneous inversion problem is transformed to the minimization problem(3.5)
(3.5)
For the above minimization problem, we set
and the existence of a minimum to the minimization problem (Equation3.5(3.5)
(3.5) ) can be obtained by Theorem 2.2 and Sobolev embedding theorems. Firstly, we have by Theorem 2.2
Corollary 3.1:
The operator is Lipschitz continuous, and there exists a constant
such that
(3.6)
(3.6)
for all .
Next by utilizing the Sobolev embedding theorems (see [Citation43] for instance), there holds
Theorem 3.2:
For the minimization problem (Equation3.5(3.5)
(3.5) ), there exists
such that
(3.7)
(3.7)
for all .
Suppose that there exist a series such that
(3.8)
(3.8)
Since ,
, there exists subseries, also denoted as
, which is weakly convergent in S. That is, there is
such that
(3.9)
(3.9)
On the other hand, the embedding relations and
are compact for the bounded domain
in 1D case, then the embedding
is compact by which the strong convergence is valid
(3.10)
(3.10)
Together with the continuity of the functional J on D and f, there holds(3.11)
(3.11)
which implies that(3.12)
(3.12)
The proof is over.
3.2. The inversion algorithm
Suppose that and
both have Fourier expansions
(3.13)
(3.13)
and(3.14)
(3.14)
where (
) and
(
) are the expansion coefficients of D(x) and f(x), and
and
are sets of basis functions in
and
, respectively. In numerical approximations, by setting
and
we have(3.15)
(3.15)
and(3.16)
(3.16)
respectively, where is the S-dimensional approximate solution to D(x) in
, and
is the K-dimensional approximate solution to f(x) in
, and
and
are dimensions of the approximate spaces, respectively. On concrete computations, it is convenient to set a S-dimensional vector
corresponding to
, and a K-dimensional vector
corresponding to
, respectively. Therefore, to get an approximate solution
is equivalent to finding a vector
, in which meaning we can say that
if there is no possibility of confusion. In what follows, we set
, and its norm is denoted by
(3.17)
(3.17)
For any given , denote
as the unique solution to the forward problem. Combining with the additional information (Equation3.1
(3.1)
(3.1) ), solving the inverse problem is equivalent to a minimization problem
(3.18)
(3.18)
where .
By Theorem 3.2, there at least exists one minimum to the minimization problem (Equation3.18(3.18)
(3.18) ). Following the stabilizing method as used in [Citation41], we utilize the homotopy regularization algorithm to solve (Equation3.18
(3.18)
(3.18) ). Hence orth, it is turned out to solve the following minimization problem instead of (Equation3.18
(3.18)
(3.18) )
(3.19)
(3.19)
where is the homotopy parameter. We refer to [Citation41] for the detailed procedures of the homotopy regularization algorithm, however, we give a sketch for solving the nonlinear minimization problem (Equation3.19
(3.19)
(3.19) ) for completeness of the paper.
By denoting , (Equation3.19
(3.19)
(3.19) ) is transformed to
(3.20)
(3.20)
where is regarded as the regularization parameter, which leads to the optimal perturbation regularization algorithm as studied in the previous works, see e.g. [Citation37,Citation42]. In fact, by linerization and numerical differentiation approximations, solving (Equation3.20
(3.20)
(3.20) ) is transformed to solve a normal equation combining with an iteration process:
(3.21)
(3.21)
where is a perturbation vector for any given
, n denotes the iterative number and
is the initial iteration, and
(3.22)
(3.22)
here is the numerical differential step, and
(
) is the unit basis vector in
, and
(3.23)
(3.23)
The algorithm can be terminated as long as an optimal perturbation satisfying the condition: , here
is the given convergent precision. Noting that
, we have by (Equation3.21
(3.21)
(3.21) )
(3.24)
(3.24)
where is just the homotopy parameter which taking values from near 1 decreasingly approximating to 0. Like done in [Citation41] we employee the homotopy parameter given as
(3.25)
(3.25)
where n is the number of iterations, is the pre-estimated number, and
is the adjust parameter. In the follows, we utilize the above inversion algorithm to perform numerical inversions for the simultaneous inverse problem.
4. Numerical inversion
In all of the computations in this section, we take , and
,
in the difference scheme (Equation2.21
(2.21)
(2.21) ) for solving the forward problem, and we choose
as the preestimated number,
as the adjust parameter, and
as the convergent precision, and we set initial iteration be zero, and numerical differential step be
in the implementation of the inversion algorithm if there is no specification. Moreover, we deal with the two-term TFDE given as
(4.1)
(4.1)
4.1. Ex. 1 – Inversion with accurate data
Let the exact diffusion coefficient be(4.2)
(4.2)
and the exact source magnitude function be(4.3)
(4.3)
and let the initial function be , and the time-dependent decay factor be
. Using the above data we can obtain the solution of the forward problem and get the additional data at one inner point of
, and further we utilize the homotopy regularization algorithm to reconstruct the diffusion coefficient and the source function in suitable approximate spaces. We here choose polynomial spaces as the approximate spaces for D(x) and f(x), and then the exact solution to the simultaneous inversion problem is represented by
(4.4)
(4.4)
due to the expansion properties of the exponential and the trigonometric functions.
Without loss of generality, we choose the fractional orders and
, and the coefficient
, and give the additional data at the central point of
to perform the inversion. Since we perform the inversions in the approximate spaces
and
, the dimensions S and K have important influences on the inversion algorithm especially for nonlinear unknowns. The inversion results with different dimensions are listed in Table , where Err1, Err2 and Err3 denote the relative errors in the inversion solutions defined by
and
and
respectively, where is the inversion diffusion coefficient and
is the inversion source function, and the norms
and
are defined as
and
and the same for and
, respectively. Moreover, for
, the exact and the inversion diffusion coefficients are plotted in Figure (a)–(d), and the exact and the inversion source functions are plotted in Figure (a)–(d), respectively.
Table 1. The inversion results with dimensions of the approximate spaces in Ex. 1.
From Table , Figures and , it can be seen that the relative errors in the solutions become small when the dimensions S and K go to large, and the inversion solutions give good approximations to the exact solutions when , which shows that the inversion algorithm is convergent in the meaning of finite-dimensional approximation.
4.2. Ex. 2 – Inversion with noisy data
In this section, we perform the inversion in the case of using noisy data to testify numerical stability of the algorithm. Suppose that the additional information is polluted with noise which is described by(4.5)
(4.5)
where denotes the noise level and
is a random number ranged in
.
Let the initial function be and the time-dependent decay factor be
, and the exact diffusion coefficient be
, and the exact source magnitude function be
. In the approximate spaces
and
, the exact solution for the inversion problem here is given by
(4.6)
(4.6)
We take the same inversion parameters as used in Ex. 1 but perform the algorithm with random noisy data. Noting the randomness of the data, we carry out the algorithm with continuous 10-time inversions for each given noise level and take an average of the inversions. It is noted that the inversion could become very difficult when coping with large noises. One approach which can overcome the ill-posedness is to utilize more additional measurements. We choose the observations and
as the additional data instead of
in the following computations. The average inversion results are listed in Table , where
is the noise level,
is the average inversion solution of the ten-time inversions, and
denotes the average relative error in the solutions defined by
(4.7)
(4.7)
where is the average inversion diffusion coefficient in
and
is the average inversion source function in
. Moreover, for
, the exact and the average inversion diffusion coefficients are plotted in Figure (a), (b), and the exact and the average inversion source functions are plotted in Figure (c), (d), respectively.
Table 2. The average inversion results with noisy data in Ex. 2.
From Table and Figure , it can be observed that the inversion algorithm is of numerical stability against the noise in the additional data. It is also noted that the inversion becomes difficult if the noise is larger than showing that the simultaneous inversion problem is of severe ill-posedness.
Remark 1:
If the source magnitude is known, and the time-dependent decay factor
and the space-dependent diffusion coefficient
are unknown, we can obtain similar results as stated in the above. For example for Equation (Equation4.1
(4.1)
(4.1) ), let the exact time-dependent decay factor be
, and the exact space-dependent diffusion coefficient be
. In addition, choosing the fractional orders
and
, and the source magnitude
, and we are to reconstruct the time-decay factor
and the spatially diffusion coefficient D(x) simultaneously also utilizing the additional measurements at the inner point of
. Noting the form of the unknowns, we perform the inversion in the approximate space
where
. The exact and the inversion solutions are plotted in Figures and , respectively.
From Figure , it can be seen that the relative errors in the solutions become small when the dimension K goes to large, and the inversion solutions give good approximations to the exact source when , which shows again that the inversion algorithm is convergent in the meaning of finite-dimensional approximation as observed in Ex. 1.
5. Conclusions
The simultaneous inversion problem of determining the space-dependent diffusion coefficient and the source term in the multi-term TFDE is investigated using measurements at the inner point. We prove the Lipschitz continuity of the solution operator, and then we get the existence of the minimum to the error functional between the additional measurements and the input–output data which gives the basis and possible approaches to solving the inverse problems.
Generally speaking, simultaneous inversion problems of determining multiple types of coefficients in a PDE are more difficult than that of determining a single type of coefficient, especially on the theoretical analysis. However, numerical inversions for such kinds of inverse problems can be performed by suitable inversion algorithms. The numerical results show that the homotopy regularization algorithm is efficient for the simultaneous inversion problem in this paper, and the inversion can also be realized successfully even for the determination of the space-dependent diffusion coefficient and the time-dependent decay factor utilizing the time-series measurements. We will focus our attention on conditional well-posedness analysis for the simultaneous inversion problems in the near future.
Additional information
Funding
Notes
No potential conflict of interest was reported by the authors.
References
- Adams EE, Gelhar LW. Field study of dispersion in a heterogeneous aquifer 2: spatial moments analysis. Water Res Res. 1992;28:3293–3307.
- Benson DA. The fractional advection-dispersion equation: development and application. Reno: University of Nevada; 1998.
- Berkowitz B, Scher H, Silliman SE. Anomalous transport in laboratory-scale heterogeneous porous media. Water Res Res. 2000;36:149–158.
- Caponetto R, Dongola G, Fortuna L, et al. Fractional order systems: modeling and control applications. Singapore: World Scientific; 2010.
- Hatano Y, Hatano N. Dispersive transport of ions in column experiments: an explanation of long-tailed profiles. Water Res Res. 1998;34:1027–1033.
- Mainardi F. Fractional calculus and waves in linear viscoelasticity: an introduction to mathematical models. London: Imperial College Press; 2010.
- Chen S, Liu F, Burrage K. Numerical simulation of a new two-dimensional variable-order fractional percolation equation in non-homogeneous porous media. Comput Math Appl. 2014;67:1673–1681.
- Coimbra CFM. Mechanics with variable-order differential operators. Ann Phys. 2003;12:692–703.
- Podlubny I. Fractional differential equations. San Diego: Academic; 1999.
- Kilbas AA, Srivastava HM, Trujillo JJ. Theory and applications of fractional differential equations. Amsterdam: Elsevier; 2006.
- Liu YK, Rundell W, Yamamoto M. Strong maximum principle for fractional diffusion equations and an application to an inverse source problem. Fract Calculus Appl Anal. 2016;19:888–906.
- Luchko Y. Maximum principle for the generalized time-fractional diffusion equation. J Math Anal Appl. 2009;351:218–223.
- Li ZY, Liu YK, Yamamoto M. Initial-boundary value problems for multi-term time-fractional diffusion equations with positive constant coefficients. Appl Math Comput. 2015;257:381–397.
- Luchko Y. Some uniqueness and existence results for the initial-boundary-value problems for the generalized time-fractional diffusion equation. Comput Math Appl. 2010;59:1766–1772.
- Luchko Y. Initial-boundary-value problems for the generalized multi-term time-fractional diffusion equation. J Math Anal Appl. 2011;374:538–548.
- Daftardar VG, Bhalekar S. Boundary value problems for multi-term fractional differential equations. J Math Anal Appl. 2008;345:754–765.
- Ding XL, Jiang YL. Analytical solutions for the multi-term time-space fractional advection-diffusion equations with mixed boundary conditions. Nonlinear Anal: Real Worlds Appl. 2013;14:1026–1033.
- Ding XL, Nieto JJ. Analytical solutions for the multi-term time-space fractional reaction-diffusion on equations on an infinite domain. Fract Calculas Appl Anal. 2015;18:697–716.
- Jiang H, Liu F, Turner I, et al. Analytical solutions for the multi-term time-fractional diffusion-wave/diffusion equations in a finite domain. Comput Math Appl. 2012;64:3377–3388.
- Li GS, Sun CL, Jia XZ, et al. Numerical solution to the multi-term time fractional diffusion equation in a finite domain. Numer Math: Theory Method Appl. 2016;9:337–357.
- Liu F, Meerschaert MM, McGough RJ, et al. Numerical methods for solving the multi-term time-fractional wave-diffusion equations. Fract Calculus Appl. Anal. 2013;16:9–25.
- Jin BT, Lazarov R, Liu YK, et al. The Galerkin finite element method for a multi-term time-fractional diffusion equation. J Comput Phys. 2015;281:825–843.
- Murio DA. Stable numerical solution of fractional-diffusion inverse heat conduction problem. Comput Math Appl. 2007;53:1492–501.
- Liu JJ, Yamamoto M. A backward problem for the time-fractional diffusion equation. Appl Anal. 2010;89:1769–1788.
- Sakamoto K, Yamamoto M. Initial value/boundary value problems for fractional diffusion-wave equations and applications to some inverse problems. J Math Anal Appl. 2011;382:426–447.
- Tuan VK. Inverse problem for fractional diffusion equation. Fract Calculus Appl Anal. 2011;14:31–55.
- Jin BT, Rundell W. An inverse problem for a one-dimensional time-fractional diffusion problem. Inverse Prob. 2012;28:075010.
- Yamamoto M, Zhang Y. Conditional stability in determining a zeroth-order coefficient in a half-order fractional diffusion equation by a Carleman estimate. Inverse Prob. 2012;28:105010.
- Luchko Y, Rundell W, Yamamoto M, et al. Uniqueness and reconstruction of an unknown semilinear term in a time-fractional reaction-diffusion equation. Inverse Prob. 2013;29:065019.
- Miller L, Yamamoto M. Coefficient inverse problem for a fractional diffusion equation. Inverse Prob. 2013;29:075013.
- Wei T, Wang JG. A modified quasi-boundary value method for an inverse source problem of the time-fractional diffusion equation. Appl Numer Math. 2014;78:95–111.
- Jin BT, Rundell W. A tutorial on inverse problems for anomalous diffusion processes. Inverse Prob. 2015;31:035003.
- Liu JJ, Yamamoto M, Yan LL. On the reconstruction of unknown time dependent boundary sources for time fractional diffusion process by distributing measurement. Inverse Prob. 2016;32:015009.
- Zhang ZD. An undetermined coefficient problem for a fractional diffusion equation. Inverse Prob. 2016;32:015011.
- Wei T, Li XL, Li YS. An inverse time-dependent source problem for a time-fractional diffusion equation. Inverse Prob. 2016;32:085003.
- Cheng J, Nakagawa J, Yamamoto M, et al. Uniqueness in an inverse problem for a one-dimensional fractional diffusion equation. Inverse Prob. 2009;25:115002.
- Li GS, Zhang DL, Jia XZ, et al. Simultaneous inversion for the space-dependent diffusion coefficient and the fractional order in the time-fractional diffusion equation. Inverse Prob. 2013;29:065014.
- Li ZY, Yamamoto M. Uniqueness for inverse problems of determining orders of multi-term time-fractional derivatives of diffusion equation. Appl Anal. 2015;94:570–579.
- Li ZY, Imanuvilov OY, Yamamoto M. Uniqueness in inverse boundary value problems for fractional diffusion equations. Inverse Prob. 2016;32:015004.
- Rodrigues FA, Orlande HRB, Dulikravich GS. Simultaneous estimation of spatially dependent diffusion coefficient and source term in a nonlinear 1D diffusion problem. Math Comput Simul. 2004;66:409–424.
- Zhang DL, Li GS, Jia XZ, et al. Simultaneous inversion for space-dependent diffusion coefficient and source magnitude in the time fractional diffusion equation. J Math Res. 2013;5:65–78.
- Jia XZ, Li GS, Sun CL, et al. Simultaneous inversion for a diffusion coefficient and a spatially dependent source term in the SFADE. Inverse Prob Sci Eng. 2016;24:832–859.
- Adams RA. Sobolev spaces. New York (NY): Academic Press; 1975.
- Liu F, Zhuang P, Burrage K. Numerical methods and analysis for a class of fractional advection-dispersion models. Comput Math Appl. 2012;64:2990–3007.