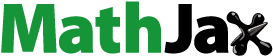
Abstract
An inverse spectral problem is studied for the matrix Sturm–Liouville operator on a finite interval with the general self-adjoint boundary condition. We obtain a constructive solution based on the method of spectral mappings for the considered inverse problem. The nonlinear inverse problem is reduced to a linear equation in a special Banach space of infinite matrix sequences. In addition, we apply our results to the Sturm–Liouville operator on a star-shaped graph.
1. Introduction
The main aim of this paper is to provide a constructive solution of the inverse spectral problem for the matrix Sturm–Liouville operator with the general self-adjoint boundary condition. The operator under consideration corresponds to the following eigenvalue problem :
(1)
(1)
(2)
(2)
Here,
is an m-element vector function;
is an
matrix function, called the potential,
; and λ is the spectral parameter. Denote by
and
the spaces of complex-valued m-element column vectors and
matrices, respectively. It is supposed that
is an orthogonal projector in
,
, where
is the unit matrix, and H = THT. The matrices
and H are assumed to be Hermitian, i.e.
a.e. on
and
, where the symbol † denotes the conjugate transpose. Under these restrictions, the boundary value problem L is self-adjoint.
We have imposed the boundary conditions (Equation2(2)
(2) ), since the problem (Equation1
(1)
(1) )–(Equation2
(2)
(2) ) generalizes eigenvalue problems for Sturm–Liouville operators on a star-shaped graph (see, e.g. [Citation1,Citation2]). Differential operators on geometrical graphs, also called quantum graphs, have applications in mechanics, organic chemistry, mesoscopic physics, nanotechnology, theory of waveguides and other branches of science and engineering (see Refs. [Citation3–7] and references therein).
Inverse problems of spectral analysis consist in reconstruction of operators, by using their spectral information. The most complete results in inverse problem theory are obtained for scalar Sturm–Liouville operators (see the monographs [Citation8–11]). Analysis of an inverse spectral problem usually includes the following steps:
Uniqueness theorem.
Constructive solution.
Necessary and sufficient conditions of solvability.
Local solvability and stability.
Numerical methods.
Uniqueness of solution in most cases is the simplest issue in inverse problem theory. Constructive solution usually means the reduction of a nonlinear inverse problem to a linear equation in a Banach space. In particular, the Gelfand–Levitan method [Citation9] reduces inverse problems to Fredholm integral equations of the second kind. In the method of spectral mappings [Citation11,Citation12], the main role is played by a linear equation in a space of infinite bounded sequences. By relying on these constructive methods, necessary and sufficient conditions of solvability have been obtained; local solvability and stability have been proved and also numerical techniques for solution [Citation13,Citation14] have been developed for various classes of inverse spectral problems. We also have to mention the historically first method of Borg [Citation11,Citation15]. Initially, this method was local. Furthermore, it was developed by Pöschel and Trubowitz [Citation10] and was used for investigating global solvability of inverse problems.
Matrix Sturm–Liouville operators in the form , where
is a matrix function, appeared to be more difficult for investigation. The main difficulties are caused by a complicated structure of spectral characteristics. Uniqueness issues of inverse problem theory for matrix Sturm–Liouville operators on finite intervals have been studied in Refs. [Citation16–21]. In Refs. [Citation22,Citation23], a constructive solution, based on the method of spectral mappings, has been developed for the inverse problem for Equation (Equation1
(1)
(1) ) under the Robin boundary conditions
(3)
(3) where
,
, and
. Chelkak and Korotyaev [Citation24] have given characterization of the spectral data for the problem (Equation1
(1)
(1) ), (Equation3
(3)
(3) ) (in other words, necessary and sufficient conditions for the inverse problem solvability) in the case of asymptotically simple spectrum. In Refs. [Citation23,Citation25], spectral data characterization has been obtained in the general case, with no restrictions on the spectrum, for self-adjoint and non-self-adjoint eigenvalue problems in the form (Equation1
(1)
(1) ), (Equation3
(3)
(3) ). Analogous results for the Dirichlet boundary conditions
are provided in Ref. [Citation26]. Mykytyuk and Trush [Citation27] have given spectral data characterization for the matrix Sturm–Liouville operator with a singular potential from the class
.
There are significantly less results on inverse matrix Sturm–Liouville problems with the general self-adjoint boundary conditions in the form (Equation2(2)
(2) ). In Ref. [Citation21], several uniqueness theorems have been proved for such inverse problems on a finite interval. Harmer [Citation28,Citation29] has studied inverse scattering on the half-line for the matrix Sturm–Liouville operator with the general self-adjoint boundary condition at the origin. However, we find inverse problems for matrix Sturm–Liouville operators on the half-line [Citation28–32] and on the line [Citation33–37] to be in some sense easier for investigation than inverse problems on a finite interval. Namely, the spectrum of the matrix Sturm–Liouville operators on a finite interval can contain infinitely many groups of multiple or asymptotically multiple eigenvalues, while operators on infinite domains usually have a bounded set of eigenvalues.
The main goal of this paper is to develop a constructive algorithm for solving an inverse spectral problem for the matrix Sturm–Liouville operator (Equation1(1)
(1) )–(Equation2
(2)
(2) ). In the future research, we plan to use this algorithm to obtain characterization of the spectral data to investigate local solvability and stability of the considered inverse problem. As far as we know, all these issues have not been studied before for the operator in the form (Equation1
(1)
(1) )–(Equation2
(2)
(2) ). One can also develop numerical methods based on our algorithm. In addition, we intend to apply our results to inverse problems for differential operators on graphs [Citation38,Citation39].
Let us define the spectral data used for reconstruction of the considered operator. Denote and assume that
. Then,
. In Ref. [Citation2], asymptotic properties of the spectral characteristics of the problem (Equation1
(1)
(1) )–(Equation2
(2)
(2) ) have been studied. In particular, it has been proved that the spectrum of L is a countable set of real eigenvalues
, such that
The more detailed eigenvalue asymptotics are provided in Section 2 of our paper. Note that multiple eigenvalues are possible, and they occur in the sequence
multiple times according to their multiplicities. One can also assume that
, if
, i.e.
or
.
Let be the
matrix solution of Equation (Equation1
(1)
(1) ), satisfying the boundary conditions
,
. Define
. The matrix functions
and
are called the Weyl solution and the Weyl matrix of L, respectively. The notion of the Weyl matrix generalizes the notion of the Weyl function for scalar Sturm–Liouville operators. Weyl functions and their generalizations play an important role in inverse problem theory for various classes of differential operators (see Refs. [Citation8,Citation11,Citation22,Citation25]). It can easily be shown that
is a meromorphic matrix function. All the singularities of
are simple poles, which coincide with the eigenvalues
. Define the weight matrices
(4)
(4) The collection
is called the spectral data of L. This paper is devoted to the following inverse problem.
Inverse Problem 1.1
Inverse Problem 1.1:Given the spectral data , recover the problem L, i.e. find the potential
and the matrices T, H.
The statement of Inverse Problem 1.1 generalizes the classical statement by Marchenko (see Refs. [Citation8,Citation11]).
Denote by the eigenvalues of the boundary value problem
,
, and by
the corresponding eigenfunctions, normalized by the condition
. The inverse problem by Marchenko consists in recovering the potential
from the spectral data
, where
are the so-called weight numbers or norming constants. Spectral data similar to
has been used for reconstruction of matrix Sturm–Liouville operators in Refs. [Citation22–25,Citation27] and other papers.
In order to solve Inverse Problem 1.1, we develop the ideas of the method of spectral mappings [Citation11,Citation12], in particular, of its modification for matrix inverse Sturm–Liouville problems [Citation22,Citation23,Citation25,Citation40]. This method is based on the contour integration in the complex plane of the spectral parameter, so it is convenient for working with multiple and asymptotically multiple eigenvalues. Our key idea is to group the eigenvalues by asymptotics and to use the sums of the weight matrices for each group. That allows us to construct a special Banach space of infinite bounded matrix sequences and to reduce Inverse Problem 1.1 to a linear equation in this Banach space.
The paper is organized as follows. In Section 2, we provide preliminaries. In Section 3, the main equation of Inverse Problem 1.1 is derived, its unique solvability is proved, and a constructive algorithm for solving the inverse problems is developed. In Section 3, we only outline the main idea of our method, while the technical details of the proofs are provided in Section 4. In Section 5, we apply our results for the matrix Sturm–Liouville problem in the form (Equation1(1)
(1) )–(Equation2
(2)
(2) ) to the Sturm–Liouville operators on a graph. In Section 6, our algorithm for solving Inverse Problem 1.1 is illustrated by a numerical example.
2. Preliminaries
In this section, we provide asymptotic formulas for the eigenvalues and the weight matrices, obtained in Ref. [Citation2]. We also state the uniqueness theorem for solution of Inverse Problem 1.1.
First, we need some notations. Define the matrix
(5)
(5) and the polynomials
One can easily show that
and
are polynomials of the degrees p and
, respectively. Note that the matrices
and
are Hermitian. Consequently, they have real eigenvalues, part of which coincide with the roots of
, j = 1, 2. Denote the roots of
by
and the roots of
by
, counting with the multiplicities and in the nondecreasing order:
for
and
.
Denote ,
,
. Without loss of generality, we assume that
. This condition can be achieved by a shift of the spectrum. Then, all the numbers
are real.
Below we use the matrix norm in , induced by the Euclidean norm in
, i.e.
, where
is the maximal eigenvalue of a matrix. The symbol C denotes various constants. The notation
is used for various sequences from
. The notation
is used for various matrix sequences, such that
.
The assertion of Ref. [Citation2, Theorem 2.1] implies the following asymptotics for the eigenvalues.
Proposition 2.1
The eigenvalues satisfy the asymptotic relations
(6)
(6) (the sequence
may be different for different k).
In order to provide asymptotics for the weight matrices , we need additional notations. In view of the definition (Equation4
(4)
(4) ), if
, then
. Further we do not need to count such equal weight matrices multiple times. Therefore for every group of multiple eigenvalues
,
, we define
,
,
. It is supposed that there are exactly l eigenvalues among
equal to
. Define the sums
The following proposition combines the results of Theorems 3.1 and 3.4 from Ref. [Citation2].
Proposition 2.2
The following asymptotic relations are valid:
(7)
(7)
(8)
(8)
where
the matrices
are uniquely specified by T, Ω and H.
In Proposition 2.2, the certain formulas for the matrices are not provided, since they are unnecessary for the purposes of this paper. However, by virtue of Bondarenko [Citation2, Corollary 3.3], the following relation holds:
(9)
(9) where
By virtue of Xu [Citation21, Theorem 3.1], the weight matrices are Hermitian, non-negative definite:
,
,
. This fact together with Proposition 2.2 yields the estimate
(10)
(10) Along with the problem L, we consider the problem
of the same form (Equation1
(1)
(1) )–(Equation2
(2)
(2) ) as L, but with different coefficients
,
and
. We agree that if a symbol γ denotes an object related to L, the symbol
with tilde denotes the similar object related to
.
Proposition 2.3
Let two problems L and be such that
and
. Then,
The next proposition is the uniqueness theorem for Inverse Problem 1.1.
Proposition 2.4
Suppose that and
for all
,
. Then,
for a.a.
,
,
.
Proposition 2.4 can be derived from the uniqueness results of Xu [Citation21] for the matrix Sturm–Liouville operator with the two boundary conditions in the general self-adjoint form. Another way to prove Proposition 2.4 is presented in Section 3. There we show that every solution of Inverse Problem 1.1 corresponds to a solution of the main equation (Equation22(22)
(22) ). Furthermore, the unique solvability of the main equation is proved, which implies Proposition 2.4.
3. Inverse problem solution
In this section, a constructive solution of Inverse Problem 1.1 is obtained. We start with a choice of a model problem of the same form as L, but with different coefficients. Then, Inverse Problem 1.1 is reduced to the linear equation (Equation12
(12)
(12) ) by using contour integration in the λ-plane (see Lemma 3.2). Furthermore, we group the eigenvalues by asymptotics and introduce a special Banach space B. It is shown that the linear equation (Equation12
(12)
(12) ) can be represented as Equation (Equation22
(22)
(22) ) in B. Later on, we prove the unique solvability of the main equation (Equation22
(22)
(22) ) (see Theorem 3.4). Furthermore, in this section, the solution of the main equation is used for constructing
and H (see Theorem 3.6). Finally, we arrive at Algorithm 3.7 for solving Inverse Problem 1.1. The results in this section are presented schematically. We provide auxiliary lemmas and the proofs in the next section.
Let the spectral data of some unknown boundary value problem
be given. Our goal is to construct the solution of Inverse Problem 1.1, i.e. to find
, T and H.
Furthermore, we need a model problem , satisfying the conditions
and
. One can construct such a model problem using the following algorithm.
Algorithm 3.1
Let the spectral data be given. We have to construct the model problem
.
Find p, relying on the eigenvalue asymptotics (Equation6
(6)
(6) ).
Construct the matrices
,
and
, by their definitions in Section 2.
Construct the matrices
,
.
Find the numbers
Construct the matrices
Calculate
Define
,
,
,
,
.
The relation (Equation9(9)
(9) ) guarantees that
. Consequently, the asymptotic relations of Proposition 2.3 hold for L and
.
Let us proceed to the derivation of the main equation. Denote by the matrix solution of Equation (Equation1
(1)
(1) ) under the initial conditions
,
. The following notation will be used for the matrix Wronskian:
. Define
(11)
(11) Introduce the notations
Using the method of spectral mappings [Citation11,Citation12], we prove the following lemma:
Lemma 3.2
The following relations hold for
,
(12)
(12)
(13)
(13)
Proof.
Repeating the standard arguments of the proofs of Refs. [Citation11, Lemma 1.6.3] and [Citation22, Lemma 1], we derive the relation
(14)
(14) where γ is the boundary of the region
with the counter-clockwise circuit,
is a fixed number. Clearly, under our assumptions, all the eigenvalues
and
lie in X. The integral in (Equation14
(14)
(14) ) converges for
in the sense
,
. Calculating the integral by the residue theorem, we obtain the relation
where the series converges uniformly with respect to
and λ on compact sets. Substituting
, we arrive at (Equation12
(12)
(12) ).
Similarly, following the proofs of Refs. [Citation11, Lemma 1.6.3] and [Citation22, Theorem 4], we derive the relation
which implies (Equation13
(13)
(13) ).
For each fixed , the relation (Equation12
(12)
(12) ) can be considered as a system of linear equations with respect to
,
,
, s = 0, 1. But the series in (Equation12
(12)
(12) ) converge conditionally in the following sense:
, so it is inconvenient to use (Equation12
(12)
(12) ) as a system of main equations of the inverse problem. Below we transform (Equation12
(12)
(12) ) into an equation in a specially constructed Banach space of bounded infinite sequences.
We divide the square roots of the eigenvalues into collections by asymptotics. Put
(15)
(15) where
, and an integer
is chosen so that
for
. Such
exists because of the asymptotic relations (Equation6
(6)
(6) ). Each collection
may contain multiple elements.
Consider a collection of (possibly multiple) complex numbers. Denote by
the finite-dimensional space of all the matrix functions
, such that
implies
, with the norm
(16)
(16) Introduce the Banach space B of infinite sequences:
(17)
(17) For
, we define the sequence
(18)
(18) where
for all
. Note that
as
. Using Schwarz's lemma similarly to Ref. [Citation11, Section 1.6.1], we get the estimates
(19)
(19) Hence,
for
, so
for each
.
For each fixed , we define the linear operator
, acting on any element
in the following way:
(20)
(20)
(21)
(21)
Thus, the action of the operator is a multiplication of an infinite row vector f of
matrices by the infinite matrix. In (Equation20
(20)
(20) ) and (Equation21
(21)
(21) ), we put operators to the right of their operands, in order to keep the correct order of matrix multiplication.
Theorem 3.3
The series in (Equation20(20)
(20) ) converges in
-norm. For each fixed
, the operator
is bounded and, moreover, compact on B.
The proof of Theorem 3.3 is provided in Section 4.
Define the element and the operator
similarly to
and
, respectively, with
,
instead of S, D. Obviously, the relation (Equation12
(12)
(12) ) can be rewritten in the form
(22)
(22) where
is the identity operator in B. Clearly, the assertion of Theorem 3.3 is valid for
, i.e. for each fixed
, the linear operator
is compact on B. We call Equation (Equation22
(22)
(22) ) in the Banach space B the main equation of Inverse Problem 1.1.
Theorem 3.4
For each fixed the main equation (Equation22
(22)
(22) ) is uniquely solvable in the Banach space B.
Proof.
Using (Equation13(13)
(13) ), (Equation20
(20)
(20) ) and (Equation21
(21)
(21) ), we derive the relation
Symmetrically, one can obtain that
Therefore, the operator
exists and equals
. By virtue of Theorem 3.3, the latter operator is bounded, so Equation (Equation22
(22)
(22) ) is uniquely solvable.
By using the solution of the main equation (Equation22
(22)
(22) ), one can construct the solution of Inverse Problem 1.1. Indeed, recall the definition (Equation18
(18)
(18) ) of
. The known
, in fact, gives us the sequence of the matrix functions
. These matrix functions satisfy Equation (Equation1
(1)
(1) ) for
, so one can construct the potential matrix by the formula
Then, one can find Ω by (Equation5
(5)
(5) ) and determine H from (Equation9
(9)
(9) )
since the matrices Θ and T are already known (see Algorithm 3.1).
Below we describe another way to find and H. The following method is more convenient for further investigation of Inverse Problem 1.1, in particular, for characterization of the spectral data for the problem L.
Define the matrix functions
(23)
(23)
Lemma 3.5
The series (Equation23(23)
(23) ) converges uniformly with respect to
. Moreover, the matrix function
is absolutely continuous on
and the elements of
belong to
.
Theorem 3.6
The following relations hold:
(24)
(24)
The proofs of Lemma 3.5 and Theorem 3.6 are provided in Section 4. Finally, we arrive at the following algorithm for solving Inverse Problem 1.1.
Algorithm 3.7
Let the spectral data be given. We have to construct
and H.
Construct the model problem
, using Algorithm 3.1. At this step, we also determine the matrices T and Θ.
Find the matrix functions
as the solutions of the initial value problems for Equation (Equation1
(1)
(1) ) with the potential
and
.
Using (Equation11
(11)
(11) ), construct the functions
for
,
,
.
Form the collections
by (Equation15
(15)
(15) ).
Using the matrix functions
and
, form the element
and the operator
(see (Equation18
(18)
(18) ), (Equation20
(20)
(20) ) and (Equation21
(21)
(21) )).
Solve the main equation (Equation22
(22)
(22) ) and find
, i.e. obtain
.
Construct
and
by (Equation23
(23)
(23) ).
Find
and H by the formulas (Equation24
(24)
(24) ).
Algorithm 3.7 is theoretical. Relying on this algorithm, one can develop a numerical technique for solving Inverse Problem 1.1. For the scalar Sturm–Liouville equation (m = 1), the numerical algorithm, based on the method of spectral mappings, is provided in Ref. [Citation14]. Similarly, one can obtain a numerical method for the matrix case, but this issue requires an additional investigation. In this paper, we illustrate the work of Algorithm 3.7 by a simple finite-dimensional example in Section 6.
4. Proofs
In this section, the proofs of the assertions from Section 3 are provided. Our methods develop the approach of Bondarenko [Citation25,Citation40] and are based on the grouping (Equation15(15)
(15) ) of the eigenvalues by their asymptotics.
Lemma 4.1
For collections
there are partitions into smaller collections
(25)
(25) such that
(26)
(26)
(27)
(27)
Here,
for any collection
of the form described in Section 3.
Proof.
The assertion of the lemma immediately follows from Propositions 2.1 and 2.3. For k = 1, the partition is trivial: ,
. For k>1, each collection
is composed of the values with equal coefficients
in the asymptotics (Equation6
(6)
(6) ).
Lemma 4.2
For
the following estimates hold:
where the constant C does not depend on n, k, ρ, θ, χ and x.
Proof.
This lemma is proved by the standard approach based on Schwarz's lemma (see Ref. [Citation11, Section 1.6.1]).
Lemma 4.3
For
the following estimate holds:
(28)
(28) where the constant C does not depend on n, k and x.
Proof.
Fix and
. Let h be an arbitrary element of
. Put
. Clearly,
. Let us prove that
(29)
(29) where the constant C does not depend on n, k, x and h. Obviously, the estimate (Equation29
(29)
(29) ) implies (Equation28
(28)
(28) ).
According to (Equation16(16)
(16) ), we have
(30)
(30) First, we prove the estimate
(31)
(31) In view of the definition (Equation21
(21)
(21) ) of
, we have
We derive that
Using (Equation16
(16)
(16) ) for
together with (Equation27
(27)
(27) ), we obtain the estimates
(32)
(32) Lemma 4.2 implies
(33)
(33) for all
,
. It follows from (Equation10
(10)
(10) ) that
(34)
(34) Using (Equation32
(32)
(32) ), (Equation33
(33)
(33) ) and (Equation34
(34)
(34) ), we obtain the estimate
(35)
(35) for s = 1, 2. It remains to prove (Equation35
(35)
(35) ) for
.
Consider the partition (Equation25(25)
(25) ) of the collection
. For every
, denote by
a fixed value
. We represent
in the following form:
Using (Equation16
(16)
(16) ) and (Equation27
(27)
(27) ), we get
(36)
(36) Lemma 4.2 implies
(37)
(37)
(38)
(38)
for all
,
,
. Combining (Equation34
(34)
(34) )–(Equation38
(38)
(38) ), we conclude that (Equation35
(35)
(35) ) holds for s = 4, 5.
In order to prove (Equation35(35)
(35) ) for
, we use the representation
Using (Equation6
(6)
(6) ) and Lemma 4.2, we obtain
(39)
(39)
(40)
(40)
for
,
. Furthermore, it follows from (Equation27
(27)
(27) ) that
(41)
(41) The estimates (Equation36
(36)
(36) ), (Equation37
(37)
(37) ), (Equation39
(39)
(39) )–(Equation41
(41)
(41) ) together yield (Equation35
(35)
(35) ) for s = 7, 8, 9. Consequently, the estimate (Equation31
(31)
(31) ) is valid.
Proof
Proof of Theorem 3.3.
Fix and suppose that
is an arbitrary element of B. By virtue of (Equation17
(17)
(17) ), we have
(42)
(42) The estimates (Equation28
(28)
(28) ) and (Equation42
(42)
(42) ) imply
Using the latter estimate together with the definition (Equation20
(20)
(20) ), we conclude that the series in (Equation20
(20)
(20) ) converges in
and
(43)
(43) In view of (Equation26
(26)
(26) ), we obtain
According to (Equation17
(17)
(17) ), we get
, i.e. the operator
is bounded on B.
Let us show that the operator can be approximated by a sequence of finite-dimensional operators. For
, define the operator
as follows:
Using (Equation43
(43)
(43) ), one can easily show that
Thus, the operator
is compact.
Remark 4.4
Note that all the constants C in the proof of Theorem 3.3 do not depend on x.
Corollary 4.5
Define
and fix
. If
the estimate
holds, where the constant C depends only on
and
.
Proof
Proof of Lemma 3.5.
The definition (Equation23(23)
(23) ) of
can be rewritten in the following form:
(44)
(44) The further arguments resemble the proof of Lemma 4.3. Recall that every collection
is divided into smaller collections
(see Lemma 4.1). For every collection
, we have chosen an arbitrary element
and denoted it as
. For brevity, denote the corresponding matrix function
by
. Then, we derive the relation
(45)
(45)
The relations (Equation19
(19)
(19) ) and (Equation27
(27)
(27) ) yield
(46)
(46) where the constant C does not depend on
and on
. The similar estimates are also valid for
.
Using (Equation34(34)
(34) ), (Equation41
(41)
(41) ), (Equation45
(45)
(45) ) and (Equation46
(46)
(46) ), we conclude that
,
,
. Consequently, the series (Equation44
(44)
(44) ) of continuous functions converges absolutely and uniformly with respect to
, and
Next, we show that the series
converges in
-norm. For definiteness, consider the first sum in (Equation45
(45)
(45) ):
Differentiation yields
Furthermore, we use the asymptotic expressions
for
. Here, the O-estimates are uniform with respect to
. Taking the grouping (Equation15
(15)
(15) ) into account, we define
Clearly,
i.e.
is the main part in the asymptotics (Equation6
(6)
(6) ) of the values from the collection
. Finally, we get
Consequently, the elements of the matrix series
converge in
. The similar technique can be applied to all the other terms in (Equation45
(45)
(45) ). Thus, the elements of the matrix function
belong to
.
Proof
Proof of Theorem 3.6
Step 1. First, we derive the relation for . Using (Equation1
(1)
(1) ) and (Equation11
(11)
(11) ), we obtain
. Then, formally differentiating (Equation14
(14)
(14) ) twice with respect to x, we get
Furthermore, we express the second derivatives from (Equation1
(1)
(1) ) and use (Equation11
(11)
(11) ), so we obtain
By residue theorem, the integral in the square brackets
equals
, defined by (Equation23
(23)
(23) ). Consequently, taking (Equation14
(14)
(14) ) into account, we get the relation
which implies
.
Step 2. Let us derive the relation for H in (Equation24(24)
(24) ). Similarly to (Equation14
(14)
(14) ), one can obtain the relation for the Weyl solution
(47)
(47) where
(48)
(48) Using (Equation47
(47)
(47) ) and (Equation48
(48)
(48) ), we derive
(49)
(49) Recall that
. Furthermore, it is shown that the first integral in (Equation49
(49)
(49) ) also equals zero. Indeed, the definition (Equation48
(48)
(48) ) yields
(50)
(50) The projectors T and
are mutually orthogonal, so it follows from the condition
that
Consequently, the relation (Equation50
(50)
(50) ) implies
(51)
(51) Consider the linear form
One can show that the matrix functions
and
are entire. Consequently, the matrix functions
and
are also entire. Therefore, in view of (Equation51
(51)
(51) ), the first integral in (Equation49
(49)
(49) ) vanishes, so we get
.
Obviously,
Since
, we obtain that
Using the asymptotic formula for the Weyl solution:
we conclude that
.
5. Inverse problem on the star-shaped graph
In this section, we apply our results to the Sturm–Liouville eigenvalue problem on the star-shaped graph [Citation1,Citation2] in the form
(52)
(52) with the standard matching conditions
(53)
(53) where
are real-valued functions from
. Clearly, the boundary value problem (Equation52
(52)
(52) )–(Equation53
(53)
(53) ) can be rewritten in the form (Equation1
(1)
(1) )–(Equation2
(2)
(2) ) with the diagonal matrix potential
, H = 0 and
,
,
.
In this section, we use the notation ,
for diagonal elements of a matrix
. Yurko [Citation38] has proved the uniqueness theorem and suggested an approach to solution of the following inverse problem.
Proof
Inverse Problem 5.1
Given the eigenvalues and the elements
of the weight matrices, construct
.
Thus, since the potential is diagonal, it is sufficient to use only the diagonal elements of the weight matrices, excluding the last elements
. The method of Yurko consists of two steps.
Algorithm 5.2
Let the data and
be given. We have to construct
.
Solving local inverse problems. For each
, find
, by using the data
.
Returning procedure. Using the given data and the already constructed potentials
, find
.
For the first step of Algorithm 5.2, Yurko suggested to derive the main equations in appropriate Banach spaces using the method of spectral mappings. Now, we can easily obtain such main equations and prove their unique solvability, relying on the results of Section 3.
Diagonality of the matrix potential implies that the matrix functions
,
and
are also diagonal. Consequently, taking only the main diagonal in the system (Equation12
(12)
(12) ), we arrive at the scalar equations
(54)
(54) where
,
, s = 0, 1. Equations (Equation54
(54)
(54) ) can be considered separately for each
.
Denote the Banach space B for m = 1 by , i.e.
is a space of scalar infinite sequences. For any element
and each
, we can choose in every matrix component of f the diagonal element at the position
and obtain the element
, by combining these diagonal elements. Taking equation (Equation54
(54)
(54) ) into account, one can construct the compact linear operators
,
, analogous to
and such that
(55)
(55) where
is the identity operator in
. Analogously to Theorem 3.4, we obtain the following result.
Theorem 5.3
For every and each
, Equation (Equation55
(55)
(55) ) is uniquely solvable in the Banach space
.
The main equation (Equation55(55)
(55) ) can be used at step 1 of Algorithm 5.2 for solving the local inverse problems for
. Theorem 5.3 justifies this step. Step 2 of Algorithm 5.2 is described in Ref. [Citation38], and we do not elaborate into that issue in the present paper.
6. Example
In this section, we solve Inverse Problem 1.1 for the following example. Put m = 3. Consider the model problem with
,
and
(56)
(56)
This matrix Sturm–Liouville problem is equivalent to the Sturm–Liouville eigenvalue problem on the star-shaped graph (Equation52
(52)
(52) )–(Equation53
(53)
(53) ) with m = 3 and
,
. It is easy to check that this problem has the eigenvalues
and the weight matrices
Suppose that the spectral data
of the problem L differ from
only by the first eigenvalue,
(57)
(57)
Let us recover the potential matrix and the coefficient H of the problem L from its spectral data
. Since the spectral data of the problems L and
coincide for
, their asymptotics coincide, and therefore we can use the problem
as the model problem for reconstruction of L by the methods of Section 3. Moreover, we have
,
.
Consider the relation (Equation12(12)
(12) ). Note that
, if
. Consequently, we get that
for all
, s = 0, 1. Hence, we obtain from (Equation12
(12)
(12) ) the system of two equations with respect to
and
:
(58)
(58) For our example, we have
Consequently, the system (Equation58
(58)
(58) ) takes the form
(59)
(59) where
Solving the system (Equation59
(59)
(59) ), we obtain
It follows from (Equation23(23)
(23) ), (Equation57
(57)
(57) ) and the above calculations that
(60)
(60) Then it is easy to find
and H by the formula (Equation24
(24)
(24) ).
In particular, for a = 0.3, we have , H = hT, where h approximately equals
. The plot of
is presented in Figure .
Let us check our calculations, by finding the eigenvalues of the problem L. The solution has the form
, where
is the solution of the following scalar initial value problem with the constructed potential
:
The eigenvalues of the problem L coincide with the zeros of its characteristic function
We have calculated the zeros of
numerically, using the forth-order Runge–Kutta method with the step
. The zeros in the interval
are presented in the following table.
Table
Clearly, ,
,
. The multiplier
has the zeros
,
. Thus, the eigenvalues of the constructed problem L coincide with the initially given values, and our method works correctly for this example.
It is clear from (Equation60(60)
(60) ) that for
the matrices
and
are nondiagonal, so the problem L is not the Sturm–Liouville problem on the star-shaped graph. This example shows that a simple perturbation of an eigenvalue can withdraw an operator out of the class of differential operators on graphs. In order to remain in this class, perturbations of the spectral data have to be connected with each other by additional conditions. Obtaining such conditions is a challenging topic for future research.
Disclosure statement
No potential conflict of interest was reported by the author(s).
Additional information
Funding
References
- Pivovarchik VN. Inverse problem for the Sturm–Liouville equation on a star-shaped graph. Math Nachr. 2007;280:1595–1619. doi: 10.1002/mana.200410567
- Bondarenko NP. Spectral analysis of the matrix Sturm–Liouville operator. Bound Value Probl. 2019;2019:178. doi: 10.1186/s13661-019-1292-z
- Nicaise S. Some results on spectral theory over networks, applied to nerve impulse transmission. Berlin: Springer; 1985. p. 532–541 (Lecture notes in mathematics; 1771).
- Langese J, Leugering G, Schmidt J. Modelling analysis and control of dynamic elastic multi-link structures. Boston, MA: Birkhäuser; 1994.
- Kuchment P. Graph models for waves in thin structures. Waves Random Media. 2002;12(4):R1–R24. doi: 10.1088/0959-7174/12/4/201
- Pokornyi YuV, Pryadiev VL. Some problems of the qualitative Sturm–Liouville theory on a spatial network. Russ Math Surv. 2004;59(3):515–552. doi: 10.1070/RM2004v059n03ABEH000738
- Berkolaiko G, Carlson R, Fulling S, et al. Quantum graphs and their applications. Providence, RI: American Mathematical Society; 2006 (Contemp. Math; 415).
- Marchenko VA. Sturm–Liouville operators and their applications. Kiev: Naukova Dumka; 1977; Russian; English transl., Birkhauser; 1986.
- Levitan BM. Inverse Sturm–Liouville problems. Moscow: Nauka; 1984; Russian; English transl., Utrecht: VNU Sci. Press; 1987.
- Pöschel J, Trubowitz E. Inverse spectral theory. New York: Academic Press; 1987.
- Freiling G, Yurko V. Inverse Sturm–Liouville problems and their applications. Huntington, NY: Nova Science Publishers; 2001.
- Yurko VA. Method of spectral mappings in the inverse problem theory. Utrecht: VNU Science; 2002 (Inverse and ill-posed problems series).
- Rundell W, Sacks PE. Reconstruction techniques for classical inverse Sturm–Liouville problems. Math Comput. 1992;58(197):161–183. doi: 10.1090/S0025-5718-1992-1106979-0
- Ignatiev M, Yurko V. Numerical methods for solving inverse Sturm–Liouville problems. Results Math. 2008;52:63–74. doi: 10.1007/s00025-007-0276-y
- Borg G. Eine umkehrung der Sturm-Liouvilleschen eigenwertaufgabe. Acta Math. 1946;78:1–96 (in German). doi: 10.1007/BF02421600
- Carlson R. An inverse problem for the matrix Schrödinger equation. J Math Anal Appl. 2002;267:564–575. doi: 10.1006/jmaa.2001.7792
- Malamud MM. Uniqueness of the matrix Sturm–Liouville equation given a part of the monodromy matrix and Borg type results. Basel: Birkhäuser; 2005. p. 237–270 (Sturm–Liouville theory).
- Chabanov VM. Recovering the M-channel Sturm–Liouville operator from M+1 spectra. J Math Phys. 2004;45(11):4255–4260. doi: 10.1063/1.1794844
- Yurko VA. Inverse problems for matrix Sturm–Liouville operators. Russ J Math Phys. 2006;13(1):111–118. doi: 10.1134/S1061920806010110
- Shieh C-T. Isospectral sets and inverse problems for vector-valued Sturm–Liouville equations. Inverse Probl. 2007;23:2457–2468. doi: 10.1088/0266-5611/23/6/011
- Xu X-C. Inverse spectral problem for the matrix Sturm–Liouville operator with the general separated self-adjoint boundary conditions. Tamkang J Math. 2019;50(3):321–336. doi: 10.5556/j.tkjm.50.2019.3360
- Yurko V. Inverse problems for the matrix Sturm–Liouville equation on a finite interval. Inverse Probl. 2006;22:1139–1149. doi: 10.1088/0266-5611/22/4/002
- Bondarenko N. Spectral analysis for the matrix Sturm–Liouville operator on a finite interval. Tamkang J Math. 2011;42(3):305–327. doi: 10.5556/j.tkjm.42.2011.756
- Chelkak D, Korotyaev E. Weyl–Titchmarsh functions of vector-valued Sturm–Liouville operators on the unit interval. J Func Anal. 2009;257:1546–1588. doi: 10.1016/j.jfa.2009.05.010
- Bondarenko NP. An inverse problem for the non-self-adjoint matrix Sturm–Liouville operator. Tamkang J Math. 2019;50(1):71–102. doi: 10.5556/j.tkjm.50.2019.2735
- Bondarenko NP. Necessary and sufficient conditions for the solvability of the inverse problem for the matrix Sturm–Liouville operator. Funct Anal Appl. 2012;46(1):53–57. doi: 10.1007/s10688-012-0006-4
- Mykytyuk YaV, Trush NS. Inverse spectral problems for Sturm–Liouville operators with matrix-valued potentials. Inverse Probl. 2010;26:015009. doi: 10.1088/0266-5611/26/1/015009
- Harmer M. Inverse scattering for the matrix Schrödinger operator and Schrödinger operator on graphs with general self-adjoint boundary conditions. ANZIAM J. 2002;43:1–8.
- Harmer M. Inverse scattering on matrices with boundary conditions. J Phys A. 2005;38(22):4875–4885. doi: 10.1088/0305-4470/38/22/012
- Agranovich ZS, Marchenko VA. The inverse problem of scattering theory. New York: Gordon and Breach; 1963.
- Freiling G, Yurko V. An inverse problem for the non-selfadjoint matrix Sturm–Liouville equation on the half-line. J Inv Ill-Posed Probl. 2007;15:785–798.
- Bondarenko N. An inverse spectral problem for the matrix Sturm–Liouville operator on the half-line. Bound Value Probl. 2015;2015:6688. doi: 10.1186/s13661-014-0275-3
- Calogero F, Degasperis A. Nonlinear evolution equations solvable by the inverse spectral transform II. Nouvo Cimento B. 1977;39(1):1–54. doi: 10.1007/BF02738174
- Wadati M. Generalized matrix form of the inverse scattering method. In: Bullough RK, Caudry PJ, editors. Solitons, topics in current physics. Vol. 17. Berlin: Springer; 1980. p. 287–299.
- Olmedilla E. Inverse scattering transform for general matrix Schrödinger operators and the related symplectic structure. Inverse Probl. 1985;1:219–236. doi: 10.1088/0266-5611/1/3/007
- Alpay D, Gohberg I. Inverse problem for Sturm–Liouville operators with rational reflection coefficient. Integr Equ Oper Theory. 1998;30:317–325. doi: 10.1007/BF01195586
- Bondarenko N. Inverse scattering on the line for the matrix Sturm–Liouville equation. J Diff Equ. 2017;262(3):2073–2105. doi: 10.1016/j.jde.2016.10.040
- Yurko VA. Inverse spectral problems for Sturm–Liouville operators on graphs. Inverse Probl. 2005;21:1075–1086. doi: 10.1088/0266-5611/21/3/017
- Yurko VA. Inverse spectral problems for differential operators on spatial networks. Russ Math Surv. 2016;71(3):539–584. doi: 10.1070/RM9709
- Bondarenko N. Recovery of the matrix quadratic differential pencil from the spectral data. J Inv Ill-Posed Probl. 2016;24(3):245–263.