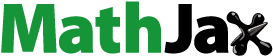
ABSTRACT
This editorial summarises the papers published in issue 14(4) so as to raise the bar in applied spatial economic research and highlight new trends. The first paper analyses the impact of re-exports on bilateral trade data. The second paper proposes a new measure for the popular smart specialisation index (S3). The third paper proposes a new solution for the so-called reflection problem in spatial or social interaction models. The fourth paper analyses bankruptcy spillovers in the state of Maryland. The final paper estimates a system of equations to analyse the mutual relationship between the domestic migration of highly-skilled graduates and innovation and productivity in U.S. states.
Spatial Economic Analysis is a pioneering journal dedicated to the development of theory and methods in spatial economic analysis. This issue contains five papers contributing to the journal's mission.
The first, by Lankhuizen and Thissen (Citation2019, this issue), analyses the impact of re-exports on bilateral trade data. Re-exports occur when imported goods in one country are exported to another country without having received any significant industrial processing. In a trading country such as the Netherlands, re-exports constitute 54% of total exports. By means of non-linear optimization techniques, the authors introduce an improvement based on the World Input–Output Database (WIOD) that properly ascribes re-exports to origin and destination countries. Next, they estimate and compare gravity equations using data over the period 1995–2011 both corrected and uncorrected for re-exports. The results show that the effects of geographical distance, cultural distance and proximity tend to be underestimated. This challenges the results of previous methodological studies, which in turn questioned earlier gravity studies using international trade data, such as Krisztin and Fischer (Citation2015), Burger, Van Oort, and Linders (Citation2009), and Lankhuizen, De Graaff, and De Groot (Citation2015). The observed trade data changes in this paper also affect recent assessments of the North America trade agreement, especially the role of Mexico as an exporter to the United States and the potential economic consequences of Brexit. The authors illustrate that there may be additional losses to the UK economy from Brexit that are not taken into account in existing studies.
The second paper by Santoalha (Citation2019, this issue) also proposes a methodological development, this time in relation to the regional innovation policy concept known as the regional Smart Specialisation (S3) potential. It tests and illustrates this newly developed measure using patent data of regions (NUTS-2) across 28 European Union countries and four European Free Trade Association (EFTA) countries (Iceland, Lichtenstein, Norway and Switzerland) over the period 1980–2013. The author also makes a distinction between the calculation of this measure at a particular point in time and over a particular period. One of the reviewers of this paper praised the clear step-wise construction of the measure, the realistic portrayal of its possibilities and caveats, as well as the elaborate checks on the internal consistency of the measure. Interestingly, using the dynamic measure over the period 2000–13, the regions in the sample can be classified as being persistent leaders, recent leaders, persistent followers and recent followers. Unsurprisingly, the author also finds significant evidence in favour of the hypothesis that the S3 index is spatially autocorrelated, that is, the leading regions are not randomly distributed across space. This finding demonstrates the importance of recognizing that regions should not be treated as independent entities when studying smart specialisation.
To account for correlation among different units, researchers should follow developments not only in the spatial econometrics literature but also in the social interactions literature. These literatures are closely related to each other, even though they use completely different terminology. Whereas the spatial econometrics literature talks about a spatial econometric model with one spatially lagged dependent variable (WY), K spatially lagged independent variables (WX) and one spatial error term (Wε), the social interaction literature talks about a linear-in-means model with peer effects, contextual effects and correlated or group (unobservable) effects. The link between these two literatures is also discussed by Gibbons, Overman, and Patacchini (Citation2015). To become more acquainted with this literature, the editorial board particularly welcomes the two following papers: Corrado, Distante, and Joxhe (Citation2019, this issue) and Grana and Eschelbach Hansen (Citation2019, this issue). The first provides a generalization of the standard linear-in-means model containing individual and group specific unobservable (time invariant) effects with a time-lagged dependent variable, that is, a dynamic spatial panel data model with spatial and time period fixed effects. The authors propose a solution to the so-called reflection problem (Manski, Citation1993); the difficulty of disentangling peer effects and contextual effects from each other (WY and WX), since only K + 1 of the K + 2 parameters are identified. Using the expected average rather than the observed behaviour of the individuals belonging to one group, the authors show that the reflection problem can be solved. Corrado et al. (Citation2019) also set out how the parameters of their model can be estimated by generalized methods-of-moments (GMM). Next, they apply their model to investigate the impact of social ties for the body mass index (BMI) of US youth.
The paper is of interest since it repeats – in a different context – the study of Burridge, Elhorst, and Zigova (Citation2017), who claim that the reflection problem is based on a misconception, even though it is still mentioned in many studies as a serious identification problem. Lee, Liu, and Lin (Citation2010) are among the first to provide formal proofs and conditions under which the parameters of a linear spatial econometric model or a linear-in-means model with K + 2 spatial lags or social interactions are identified. Importantly, their proofs are limited to a spatial weight matrix that, just as in Corrado et al. (Citation2019), is specified as a block diagonal matrix where each block represents a group of units that interact with each other but not with members of other groups. In that case the value of all off-diagonal elements within a block equals , where
denotes the number of units in group r. Despite the fact that such a group interaction matrix lacks popularity in spatial econometric research, these proofs make clear that Manski's reflection problem does not carry over when the endogenous peer effect operates via the mean of each individual's peers, since this mean is different for each individual.
This issue is also mentioned by Gibbons et al. (Citation2015) as the main of three solutions to the reflection problem. It is at the moment that the spatial weight matrix W is not equal to its square () that the parameters of a spatial econometric model or linear-in-means model with K + 2 spatial lags or social interactions can be identified. To understand this mathematical interpretation better, Gibbons et al. make a distinction between complete and incomplete networks, that is, identification problems or not. In the first situation every unit is connected to every other unit within a group, say all regions located within one country; in the second situation each unit has its own group of units. Most spatial econometric researchers presume the second situation and so do not face identification problems. Gibbons et al. argue that determining the correct network structure (read: W matrix) in this case is difficult and requires detailed and accurate data to know who interacts with whom. However, labelling every structure as an ad hoc approach (p. 143) may go too far, all the more so since presupposing a complete network is also an ad hoc decision set by the researcher. Readers interested in more details should consult their handbook chapter, the paper by Corrado et al., as well as one of our previous editorials (Elhorst et al., Citation2017).
Grana and Eschelbach Hansen (Citation2019, this issue) offer an interesting application of a mixed spatial econometric and social interaction model, although not all K + 2 potential spatial lags or social interactions are considered as in the previous study. The paper analyses bankruptcy spillovers using a data set of all debtors who filed in the state of Maryland from 1949 to 1973. Bankruptcy spillovers are defined at the household level as the decision to file for bankruptcy in relation to time lags and space–time lags of the bankruptcy of surrounding households. An alternative description provided in the paper is that closer neighbours are more likely to share an attorney. The novel geocoded data set used here is well described and the assumptions regarding its construction are plausible. In the empirical model, supported by an economic–theoretical derivation, the authors aggregate the data at both the county and neighbourhood levels, and presume that the potential number of time or space–time lags at the first higher level is smaller than at the second lower level. Grana and Eschelbach Hansen find that the time lags have more effect than space–time lags and that these effects at the neighbourhood level exceed those at the county level. According to them, these results point to interpersonal exchange of information as the dominant mechanism of transmission since the alternative, stigma effects, would remain intact rather than die out.
This paper fits within a series of earlier papers in this journal based on financial economic or banking data. In issue 14(2) we published a paper by Gross (Citation2019) who explains that a spatial weight matrix W can be estimated using a global vector autoregressive (GVAR) approach, which is relevant since it offers a solution to the issue raised by Gibbons et al. (Citation2015) and the GVAR approach is a popular tool to explain country data and (financial) links among countries. In issue 13(4) we published a paper by Zhu (Citation2018), who used a GVAR model to analyse the multi-country transmission of sovereign and banking risk. Finally, we recently accepted a paper by Capasso, D’Uva, Fiorelli, and Napolitano (Citation2019), who also estimate a GVAR model, this time focusing on spatial asymmetries in monetary policy effectiveness in Italian regions. Related work appeared by Ramajo, Márquez, and Hewings (Citation2017) in the International Regional Science Review. This is evidently a new trend in the spatial economics literature.
The fifth paper, by Kazakis (Citation2019, this issue), investigates the mutual relationships between the domestic migration of highly skilled graduates in the United States and the innovation and productivity in the states of destination. Using an economic–theoretical model, the author demonstrates that not only wages (read: innovation or productivity), but also housing prices and amenities affect people's decisions about where to migrate. Patents are used as a proxy for innovation; and total factor productivity and labour productivity per hour as proxies for productivity. Amenities are measured by density, income inequality, air quality and the tax rate. The author extensively discusses the chicken and egg problem. If graduates move to other states, they increase the level of innovation or productivity in that state. Conversely, if a state is more innovative or productive, this state may act as a magnet to new graduates. For this reason, the author estimates a two-equation system in which both the flow of highly skilled immigration and the level of innovation or productivity (measured by a range of different variables) are not only left-hand-side variables but also appear as right-hand-side variables in each other's equation. This system is estimated both by two-stage least squares (2SLS) and three-stage least squares (3SLS). 3SLS is more efficient than 2SLS since it also accounts for correlation among the error terms within the system. The instrumental variables used to run these estimators are the level of education of entrepreneurs, research and development (R&D) expenditures, and a product term between the ratio of population with a university degree and the share of wealth of the top 1% of the income distribution, say, the number of Bill Gates’s. All these variables turn out to play an important role, either positive or negative, in the explanation of regional innovation. This paper fits within in a series of previous papers in Spatial Economic Analysis dealing with graduate migration in the United States (e.g., Faggian & Franklin, Citation2014), or graduate migration in combination with the use of instrumental variables (e.g., Haapanen & Böckerman, Citation2017).
Turning to other matters, last year co-editor Harry Garretsen (University of Groningen) finished his term on the journal. This summer we welcomed Coro Chasco (Autonomous University of Madrid) and Daniel Felsenstein (Hebrew University of Jerusalem) as new co-editors. We thank the departing co-editor for all the work he has done for the journal, and we wish the two new co-editors all the best with their new positions. Finally, we would like to congratulate the winners of the Spatial Economic Analysis Regional Studies Association (RSA) awards for 2019: Konstantin Gluschenko (Novosibirsk State University), who has been awarded best paper for his study entitled ‘Measuring regional inequality: to weight or not to weight?’ (Gluschenko, Citation2018) and Harry Kelejian (University of Maryland), who has been awarded best referee.
REFERENCES
- Burger, M., Van Oort, F., & Linders, G.-J. (2009). On the specification of the gravity model of trade: Zeros, excess zeros and zero-inflated estimation. Spatial Economic Analysis, 4(2), 167–190.
- Burridge, P., Elhorst, J. P., & Zigova, K. (2017). Group interaction in research and the use of general nesting spatial models. In K. Pace, J. P. LeSage, & B. Baltagi (Eds.), Advances in econometrics, volume 37, spatial and spatiotemporal econometrics (pp. 223–258). Amsterdam: Elsevier.
- Capasso, S., D’Uva, M., Fiorelli, C., & Napolitano, O. (2019). Spatial asymmetries in monetary policy effectiveness in Italian regions. Spatial Economic Analysis. doi: 10.1080/17421772.2020.1673899
- Corrado, L., Distante, R., & Joxhe, M. (2019). Body mass index and social interactions from adolescence to adulthood. Spatial Economic Analysis, 1–21. doi: 10.1080/17421772.2019.1626020
- Elhorst, P., Abreu, M., Amaral, P., Bhattacharjee, A., Corrado, L., Doran, J., … Yu, J. (2017). Raising the bar (5). Spatial Economic Analysis, 12(1), 1–7.
- Faggian, A., & Franklin, R. S. (2014). Human capital redistribution in the USA: The migration of the college-bound. Spatial Economic Analysis, 9(4), 376–395.
- Gibbons, S., Overman, H. G., & Patacchini, E. (2015). Spatial methods. In G. Duranton, J. Vernon Henderson, & W. C. Strange (Eds.), Handbook of regional and urban economics, volume 5 (pp. 115–168). Amsterdam: Elsevier.
- Gluschenko, K. (2018). Measuring regional inequality: To weight or not to weight? Spatial Economic Analysis, 13(1), 36–59.
- Grana, J., & Eschelbach Hansen, M. (2019). New evidence of spillovers in personal bankruptcy using point-coded data. Spatial Economic Analysis, 1–19. doi: 10.1080/17421772.2019.1636128
- Gross, M. (2019). Estimating GVAR weight matrices. Spatial Economic Analysis, 14(2), 219–240.
- Haapanen, M., & Böckerman, P. (2017). More educated, more mobile? Evidence from post-secondary education reform. Spatial Economic Analysis, 12(1), 8–26.
- Kazakis, P. (2019). On the nexus between innovation, productivity and migration of US university graduates. Spatial Economic Analysis, 1–21. doi: 10.1080/17421772.2019.1636127
- Krisztin, T., & Fischer, M. M. (2015). The gravity model for international trade: Specification and estimation issues. Spatial Economic Analysis, 10(4), 451–470.
- Lankhuizen, M. B. M., De Graaff, T., & De Groot, H. L. F. (2015). Product heterogeneity, intangible barriers and distance decay: The effect of multiple dimensions of distance on trade across different product categories. Spatial Economic Analysis, 10(2), 137–159.
- Lankhuizen, M., & Thissen, M. (2019). The implications of re-exports for gravity equation estimation, NAFTA and Brexit. Spatial Economic Analysis, 1–20. doi: 10.1080/17421772.2019.1623419
- Lee, L. F., Liu, X., & Lin, X. (2010). Specification and estimation of social interaction models with network structures. Econometrics Journal, 13(2), 145–176.
- Manski, C. F. (1993). Identification of endogenous social effects: The reflection problem. Review of Economic Studies, 60(3), 531–542.
- Ramajo, J., Márquez, M. A., & Hewings, G. J. D. (2017). Spatiotemporal analysis of regional systems: A multiregional spatial vector autoregressive model for Spain. International Regional Science Review, 40(1), 75–96.
- Santoalha, A. (2019). New indicators of related diversification applied to smart specialization in European regions. Spatial Economic Analysis, 1–21. doi: 10.1080/17421772.2019.1584328
- Zhu, B. (2018). The multi-country transmission of sovereign and banking risk: A spatial vector autoregressive approach. Spatial Economic Analysis, 13(4), 422–441.