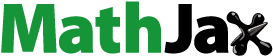
ABSTRACT
This editorial summarizes the papers published in issue 15(2) so as to raise the bar in applied spatial economic research and highlight new trends. The first paper combines a conditionally autoregressive process from the spatial statistics literature with a spatial Durbin error model from the spatial econometrics literature. The second paper feeds a multistage and multilevel data envelopment analysis with a microeconomic foundation. The third paper provides empirical evidence that Flegg’s location quotient combined with a gravity model produces the most accurate interregional input–output multipliers. The fourth paper investigates the impact of inventor networks on the number of patents per capita in Brazil.
Spatial Economic Analysis is a pioneering journal dedicated to the development of theory and methods in spatial economic analysis. This issue contains four papers contributing to these developments.
The first paper, by Epifani et al. (Citation2020, this issue), is an interesting, consistent and well-documented piece of research. It models spatial dependence while controlling for both local and global heterogeneity within the applied empirical strategy of explaining population distribution across space. It is interesting for a series of reasons. First, it adopts a conditionally autoregressive (CAR) rather than a spatial autoregressive (SAR) process. Although Spatial Economic Analysis has published not many papers estimating CAR processes (e.g., Beręsewicz & Nikulin, Citation2018; Panzera et al., Citation2016), the spatial demography literature has largely focused on the CAR model. This points to a dichotomy between the spatial econometric and the spatial statistics literatures that apparently is difficult to bridge. Spatial statistics focuses on CAR models that offer simple interpretation in terms of conditional distributions and good predictions, while spatial econometrics focuses on structural models such as SAR that enable counterfactual spatial analysis. Spatial econometricians may read the Handbook of Spatial Statistics, edited by Gelfand et al. (Citation2010), to appreciate that CAR models are quite different from SAR models. Fortunately, there are also researchers trying to bridge the gap, such as Wall (Citation2004) and Ver Hoef et al. (Citation2018), who explain the differences and similarities between CAR and SAR processes. Readers may also be interested in other recent demographic work attempting to draw structural interpretations from CAR models with unknown spatial weights matrix (e.g., Castro et al., Citation2015; Zhang et al., Citation2020).
Second, the paper by Epifani et al. (Citation2020, in this issue) is quite unique because of its focus on the spatial Durbin error model (SDEM), which is a model containing spatially lagged explanatory variables, a spatially correlated error term (CAR process), but not a spatially lagged dependent variable. Elhorst (Citation2017) has extensively discussed the differences. A SAR model or spatial Durbin model (SDM) causes global spillover effects and a SDEM model local spillover effects. Spatial Economic Analysis receives a striking number of submissions only focusing on the SDM model, probably because of the popular textbook by LeSage and Pace (Citation2009), which strongly motivates this model. However, not many researchers ask themselves whether global spillovers really make sense for the problem at hand. In many cases, local spillovers are much more likely, requiring the SDEM model. In later work, LeSage (Citation2014) fixes this by developing a Bayesian comparison test that helps to test the SDM with global spillovers and the SDEM with local spillovers against each other. Successful applications of this approach are Rios et al. (Citation2017) and Credit (Citation2019). We hope this new contribution on CAR will further strengthen this tendency and make inroads into the spatial statistics literature.
Third, this submission caused a fascinating discussion between the authors and a reviewer about the existence of a spatial unit root problem in the outcomes. Yu et al. (Citation2012) outline the conditions for a spatial unit root from an econometric–theoretical viewpoint, and Bhattacharjee et al. (Citation2016) discuss the connection with spatial granularity (Pesaran & Tosetti, Citation2011), while Elhorst et al. (Citation2013) extensively discuss its empirical implications. Epifani et al. (Citation2020, in this issue) follow a different approach. They change the prior density function used to generate the spatial autocorrelation coefficient of the error term in their Bayesian estimator, such that this parameter is less close to unity. Nevertheless, they still find values ranging from 0.763 to 0.842 for this spatial parameter ( in their Table 6). To justify this outcome, they refer to several other studies that also found high values, almost all of which appeared in the journal Spatial Statistics. We leave the judgement of this alternative approach to readers, though not without the warning that one potential reason for high values of spatial dependence thwarting many empirical studies is the lack of controls for time period fixed effects. Lee and Yu (Citation2010) show, using Monte Carlo simulation experiments, that ignoring time-period fixed effects may lead to large upward biases (up to 0.45) of the spatial dependence parameter.
The second paper in this issue, by Ribeiro et al. (Citation2020, in this issue), deals with multistage and multilevel data envelopment analysis (DEA). DEA is a widely used non-parametric or deterministic technique to measure the efficiency of decision-making units (DMUs). According to Nijkamp and Suzuki (Citation2009), it has become an established benchmark tool to develop efficiency strategies in both the public and private sectors. A multistage extension of DEA offers the opportunity to divide the process to be analysed into different stages. In their empirical illustration, they consider the production and commercialization stages of regional innovation systems in Portugal. Similarly, a multilevel approach offers the opportunity to distinguish DMUs at different levels of scale. An alternate popular framework for analyses of efficiency is stochastic factor analysis, and readers may refer to Carvalho (Citation2018) for a recent paper on spatial stochastic frontier analysis.
In their empirical application, Ribeiro et al. (Citation2020, in this issue) distinguish municipalities (sub-DMUs) within the 25 NUTS-3 regions in Portugal. In addition, they consider cases with constant and increasing returns to scale. A mathematical overview of these different models and cases are provided in the first part of paper, while an appendix with supplementary material (data, supporting files, mathematical details and proofs of the claims made) is available online. The objective of the paper is to feed DEA with a microeconomic foundation by introducing the Spence distortion principle, perhaps better known as the average-based pricing principle, as the pillar for the proper evaluation of efficiency scores in multilevel and multistage systems. According to a reviewer, this set-up is ground-breaking, while the empirical application illustrates the most important ideas. Spatial Economic Analysis has been making special efforts to encourage authors to make their data and supplemental material available for replication and further research (Elhorst et al., Citation2016, Citation2019a; Rey, Citation2019).
The third paper, by Jahn et al. (Citation2020, in this issue) fits within a series of papers on input–output analysis (e.g., see past editorials in Spatial Economic Analysis for more details; Elhorst et al., Citation2019b, Citation2020). As also highlighted recently by Fujimoto (Citation2019), the regionalization of national input–output tables is a major issue in regional science as corresponding regional data are often unavailable. Recently, Jahn (Citation2017) developed a framework to estimate intra- and interregional input–output tables. The intraregional estimates are based on Flegg’s location quotient (FLQ), while the interregional estimates are constructed using gravity modelling. Jahn illustrated this methodology for German regions; in the present paper it is illustrated for South Korean regions. However, the authors take several additional steps forward. First, as Jahn did not have the necessary survey-based regional data for the 16 federal states in Germany to test the proposed methodology, his previous empirical work was entirely illustrative. Second, the gravity model employed to produce the estimates for Germany was estimated using Eurostat data on international trade, while the Korean data set in this study also contains data on interregional trade. Because of this lack of data, Jahn was previously unable to generate estimates of the optimal values of the δ parameter in the FLQ formula, which is a major research topic in this interesting methodological contribution to the input–output literature. Finally, for the same reason, he could not assess the relative merits of gravity and simple trade models. All the above are key aspects of this study, which makes it an important contribution.
The fourth paper, by Gonçalves et al. (Citation2020, in this issue), investigates the impact of inventor networks on the output of economically useful technological knowledge measured by the number of patents per capita. As highlighted by Zhao and van Dijk (Citation2020, passim), knowledge and innovation spillovers has been an important topic in Spatial Economic Analysis. Gonçalves et al. (Citation2020, in this issue) consider four network-related explanatory variables: intra- and interregional collaborative inventor network characteristics, network density, and international network characteristics. Their discussion of theoretical background results in four testable hypotheses, while their empirical model is estimated using data of 558 microregions in Brazil over a period of 12 years, 2000–11. This econometric model takes the form of a SAR model with regional fixed effects and quadratic terms in some of the explanatory variables to test for non-linearity. The authors find that intraregional collaboration is beneficial and interregional collaboration is advantageous only above a certain threshold. In backward regions, international collaborative links sometimes compensate this lack of benefits. Finally, network density turns out to have a negative effect. The study enhances our understanding of the spread of innovation and its economic impacts.
REFERENCES
- Beręsewicz, M., & Nikulin, D. (2018). Informal employment in Poland: an empirical spatial analysis. Spatial Economic Analysis, 13(3), 338–355. https://doi.org/10.1080/17421772.2018.1438648
- Bhattacharjee, A., Castro, E. A., Maiti, T., & Marques, J. L. (2016). Endogenous spatial regression and delineation of submarkets: A new framework with application to housing markets. Journal of Applied Econometrics, 31(1), 32–57. https://doi.org/10.1002/jae.2478
- Carvalho, A. (2018). Efficiency spillovers in Bayesian stochastic frontier models: Application to electricity distribution in New Zealand. Spatial Economic Analysis, 13(2), 171–190. https://doi.org/10.1080/17421772.2018.1444280
- Castro, E. A., Zhang, Z., Bhattacharjee, A., Martins, J. M., & Maiti, T. (2015). Regional Fertility Data Analysis: A Small Area Bayesian Approach. In D. K. Dey, A. Loganathan, U. Singh, & S. K. Upadhyay (Eds.), Current Trends in Bayesian Methodology with Applications, Chapter 10 (pp. 203–224). CRC Press.
- Credit, K. (2019). Transitive properties: a spatial econometric analysis of new business creation around transit. Spatial Economic Analysis, 14(1), 26–52. https://doi.org/10.1080/17421772.2019.1523548
- Elhorst, J. P. (2017). Spatial Panel Data Analysis. In S. Shekhar, H. Xiong, & X. Zhou (Eds.), Encyclopedia of GIS (2nd ed., pp. 2050–2058). Springer.
- Elhorst, P., Abreu, M., Amaral, P., Bhattacharjee, A., Chasco, C., Corrado, L., Doran, J., Felsenstein, D., Fuerst, F., Le Gallo, J., McCann, P., Monastiriotis, V., Quatraro, F., Temursho, U., & Yu, J. (2020). Raising the bar (14). Spatial Economic Analysis, 15(1), 60–61. https://doi.org/10.1080/17421772.2020.1708574
- Elhorst, P., Abreu, M., Amaral, P., Bhattacharjee, A., Corrado, L., Doran, J., Fuerst, F., Le Gallo, J., McCann, P., Monastiriotis, V., Quatraro, F., & Yu, J. (2019a). Raising the bar (12). Spatial Economic Analysis, 14(3), 269–272. https://doi.org/10.1080/17421772.2019.1639035
- Elhorst, P., Abreu, M., Amaral, P., Bhattacharjee, A., Corrado, L., Doran, J., Fuerst, F., Le Gallo, J., McCann, P., Monastiriotis, V., Quatraro, F., & Yu, J. (2019b). Raising the bar (10). Spatial Economic Analysis, 14(1), 1–4. https://doi.org/10.1080/17421772.2019.1553658
- Elhorst, P., Abreu, M., Amaral, P., Bhattacharjee, A., Corrado, L., Fingleton, B., Fuerst, F., Garretsen, H., Igliori, D., Le Gallo, J., McCann, P., Monastiriotis, V., Pryce, G., & Yu, J. (2016). Raising the bar (2). Spatial Economic Analysis, 11(2), 123–127. https://doi.org/10.1080/17421772.2016.1153838.
- Elhorst, P., Zandberg, E., & de Haan, J. (2013). The Impact of interaction effects among neighbouring countries on financial liberalization and reform: A dynamic spatial panel data approach. Spatial Economic Analysis, 8(3), 293–313. https://doi.org/10.1080/17421772.2012.760136
- Epifani, I., Ghiringhelli, C., & Nicolini, R. (2020). Population distribution over time: modelling local spatial dependence with a CAR process. Spatial Economic Analysis. https://doi.org/10.1080/17421772.2020.1708442
- Fujimoto, T. (2019). Appropriate assumption on cross-hauling national input–output table regionalization. Spatial Economic Analysis, 14(1), 106–128. https://doi.org/10.1080/17421772.2018.1506151
- Gelfand, A. E., Diggle, P. J., Fuentes, M., & Guttorp, F. (2010). Handbook of Spatial Statistics. CRC Press.
- Gonçalves, E., Medeiros de Oliveira, P., & Almeida, E. (2020). Spatial determinants of inventive capacity in Brazil: the role of inventor networks. Spatial Economic Analysis. https://doi.org/10.1080/17421772.2019.1637532
- Jahn, M. (2017). Extending the FLQ formula: a location quotient-based interregional input−output framework. Regional Studies, 51(10), 1518–1529. https://doi.org/10.1080/00343404.2016.1198471
- Jahn, M., Flegg, A. T., & Tohmo, T. (2020). Testing and implementing a new approach to estimating interregional output multipliers using input–output data for South Korean regions. Spatial Economic Analysis. https://doi.org/10.1080/17421772.2020.1720918
- Lee, L. F., & Yu, J. (2010). Some recent developments in spatial panel data models. Regional Science and Urban Economics, 40(5), 255–271. https://doi.org/10.1016/j.regsciurbeco.2009.09.002
- LeSage, J. P. (2014). Spatial econometric panel data model specification: A Bayesian approach. Spatial Statistics, 9, 122–145. https://doi.org/10.1016/j.spasta.2014.02.002
- LeSage, J. P., & Pace, R. K. (2009). Introduction to Spatial Econometrics. Chapman & Hall/CRC Press.
- Nijkamp, P., & Suzuki, S. (2009). A generalized goals-achievement model in data envelopment analysis: an application to efficiency improvement in local government finance in Japan. Spatial Economic Analysis, 4(3), 249–274. https://doi.org/10.1080/17421770903114687
- Panzera, D., Benedetti, R., & Postiglione, P. (2016). A Bayesian Approach to Parameter Estimation in the Presence of Spatial Missing Data. Spatial Economic Analysis, 11(2), 201–218. https://doi.org/10.1080/17421772.2016.1102962
- Pesaran, M. H., & Tosetti, E. (2011). Large panels with common factors and spatial correlation. Journal of Econometrics, 161(2), 182–202. https://doi.org/10.1016/j.jeconom.2010.12.003
- Rey, S. J. (2019). PySAL: the first 10 years. Spatial Economic Analysis, 14(3), 273–282. https://doi.org/10.1080/17421772.2019.1593495
- Ribeiro, V. M., Varum, C., & Daniel, A. D. (2020). Introducing microeconomic foundation in DEA: the average-based approach. Spatial Economic Analysis. https://doi.org/10.1080/17421772.2020.1701701
- Rios, V., Pascual, P., & Cabases, F. (2017). What drives local government spending in Spain? A dynamic spatial panel approach Spatial Economic Analysis, 12(2–3), 230–250. https://doi.org/10.1080/17421772.2017.1282166
- Ver Hoef, J. M., Hanks, E. M., & Hooten, M. B. (2018). On the relationship between conditional (CAR) and simultaneous (SAR) autoregressive models. Spatial Statistics, 25, 68–85. https://doi.org/10.1016/j.spasta.2018.04.006
- Wall, M. M. (2004). A close look at the spatial structure implied by the CAR and SAR models. Journal of Statistical Planning and Inference, 121(2), 311–324. https://doi.org/10.1016/S0378-3758(03)00111-3
- Yu, J., de Jong, R., & Lee, L. F. (2012). Estimation for spatial dynamic panel data with fixed effects: The case of spatial cointegration. Journal of Econometrics, 167(1), 16–37. https://doi.org/10.1016/j.jeconom.2011.05.014
- Zhang, Z., Bhattacharjee, A., Maiti, T., & Marques, J. L. (2020). Spatio-temporal patterns in Portuguese regional fertility rates: A Bayesian approach for spatial clustering of curves. Journal of Official Statistics( Forthcoming).
- Zhao, M. Q., & van Dijk, J. (2020). Modelling knowledge and innovation spillovers in China. Spatial Economic Analysis, 15(1), 1–4. https://doi.org/10.1080/17421772.2020.1700088