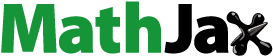
ABSTRACT
This paper relies on a regional knowledge production function to examine the heterogeneous determinants of knowledge creation across five US manufacturing sectors and 853 metropolitan counties over the period 2001–08. Using a Tobit model with state fixed effects, the results indicate that local intra- and inter-sectoral research and development (R&D) investments by the private sector as well as university R&D play a key role in knowledge creation across all sectors under study. We also find that the role of short- versus long-distance interregional spillovers on knowledge creation varies greatly across sectors. These key features improve the design of future local and national innovation policies.
INTRODUCTION
As knowledge accumulation and its spillovers are recognized as important determinants of economic growth (Jaffe, Citation1989; Romer, Citation1986) and firm productivity (Audretsch & Belitski, Citation2020), the knowledge production function literature has paid an increasing amount of attention to the role and the geography of knowledge spillovers (Acs et al., Citation2002; Audretsch & Feldman, Citation2004; Autant-Bernard, Citation2012; Buzard et al., Citation2020; Ganguli et al., Citation2020; Kwon et al., Citation2020; Thompson, Citation2006). While geographical proximity facilitates the flow of knowledge (Buzard et al., Citation2020; Ganguli et al., Citation2020; Jaffe et al., Citation1993), other mechanisms such as non-market interactions (Glaeser & Scheinkman, Citation2000), technological proximity (Maggioni et al., Citation2011), labour mobility (Almeida & Kogut, Citation1999), social networks (Crescenzi et al., Citation2016) and patent citations (Kang & Dall’erba, Citation2016a, Citation2016b; Peri, Citation2005) also promote the diffusion of knowledge across space. As such, a large amount of the more recent research in this area has challenged the traditional view that local knowledge flows are the main determinants of local innovation (Caragliu & Nijkamp, Citation2015; Malerba et al., Citation2013; Peri, Citation2005; Ponds et al., Citation2010; Qiu et al., Citation2017). At the same time, a growing number of studies follows Glaeser et al. (Citation1992) in debating the relative importance of intra- and inter-sectoral knowledge spillovers on the creation of innovation. In spite of an extensive literature on Marshallian versus Jacobian spillovers, there is still no consensus on which type matters more (Groot et al., Citation2016). Rather, the existence of sectoral heterogeneity has been recognized in the literature as summarized in a recent meta-analysis of the literature on knowledge creation and spillovers (Neves & Sequeira, Citation2018).
To the best of our knowledge, comprehensive examinations on the sectoral heterogeneity of various types of interregional and inter-sectoral knowledge spillovers are limited. Jaffe (Citation1989) and Anselin et al. (Citation2000) differentiate the localized knowledge spillovers by sector but only capture intra-sectoral spillovers. Autant-Bernard and LeSage (Citation2011) demonstrate the significant impact of inter-sectoral spillovers of private research and development (R&D) among French metropolitan areas. However, their panel model is averaged across all sectors. Acemoglu et al. (Citation2016) highlight the importance of inter-sectoral spillovers of knowledge in the United States as captured through a matrix of patent creation–patent citations. Yet, their work is performed at the national level, hence provides no guidance on how the geographical distance between the origin and destination locations of these spillovers may affect knowledge creation across sectors. Malerba et al. (Citation2013) also study sectoral heterogeneity and knowledge spillovers for three large industries in Europe. They examine inter- and intra-sectoral spillovers and distinguish between national and international spillovers, but they do not analyse the importance of geography within a country. On the other hand, Autant-Bernard and LeSage (Citation2019) focus on the effect of geographical distance when they study the knowledge creation and diffusion process of four industries across French regions. However, their panel data approach does not distinguish the marginal effects by sector. Finally, Baum et al. (Citation2017) argue about sectoral heterogeneity in knowledge creation, but they do not focus on any type of spillovers for innovation.
This paper contributes to this literature by identifying the singular role of intra- and inter-sectoral knowledge spillovers on knowledge creation and by reporting results by sector. In order to decompose sectoral knowledge spillovers geographically, we classify these spillovers into three categories: (1) local spillovers (within the county), (2) short-distanced interregional spillover (from neighbouring counties located on a 50-mile radius), and (3) spillovers from the rest of the United States (beyond 50 miles). More specifically, we focus on the five most innovative manufacturing sectors in the United States: (1) chemical, (2) drugs and medical, (3) mechanical, (4) computer and communication, and (5) electrical and electronic. They represent about 82% of our patent data drawn from the US Patent and Trade Office (USPTO) (Citation2010). We study how the existing stock of research inputs impacts new knowledge creation by relying on an interregional innovation network of patent creation–patent citation. This approach and our reliance on the actual patent network allow us to recognize the complex and spatially dependent nature of innovation and to improve our understanding of industrial innovation dynamics. To our knowledge, this is the first study to examine the role of interregional and inter-sectoral knowledge spillovers simultaneously and reports results by sector.
Our sample covers 853 metropolitan counties over the period 2001–08, which allows us to obtain detailed results on the regional and sectoral knowledge production process compared with the existing literature where the data are aggregated at larger geographical scales (Acemoglu et al., Citation2016; Anselin et al., Citation2000; Peri, Citation2005). We use a panel Tobit model with time and state fixed effects to control for the case where no knowledge output is recorded and for cross-sectional unobservable heterogeneity (Wooldridge, Citation2010). Knowledge spillovers are based on the data collected by Lai et al. (Citation2013). This dataset tracks the actual flows of knowledge from the place where they are created to the place(s) where they are cited and it has been previously used in the literature in different contexts (Autor et al., Citation2016; Galasso & Schankerman, Citation2018; Moretti & Wilson, Citation2017). The major advantage of capturing the directionality of the knowledge flows is to allow us to explicitly identify the role of externalities on knowledge output.
Our results support the importance of geographical proximity for knowledge creation and indicate that both local intra-sectoral as well as local inter-sectoral spillovers are important determinants of knowledge production. Regarding the importance of interregional private and university spillovers, we observe large sectoral heterogeneity as the various sectors benefit differently from the knowledge created in other regions whether they are close by or remote. These results suggest that the cumulative process of scientific discovery is heterogenous and complex.
The remainder of the paper is organized as follows: The next section reviews the previous literature. We then describe our knowledge production function, the strategy of modelling knowledge spillovers and the data. The estimation results, their interpretation and a robustness test are afterwards reported. The last section concludes.
LITERATURE REVIEW
The geography of knowledge spillovers
Any discussion about the importance of knowledge spillovers for innovation and growth goes back to Marshall (Citation1890) and industrial clusters. These externalities are referred to as the Marshall–Arrow–Romer (MAR) externalities in the literature, which emphasize that industrial specialization leads to enhanced knowledge spillovers. A well-known example is Silicon Valley where knowledge flows across high-technology firms are galvanized through non-market interactions and inter-firm mobility of skilled workers (Fallick et al., Citation2006; Saxenian, Citation1994). The second type of local externalities, based on Jacobs (Citation1969), derives from industrial diversity, where diverse knowledge coming from different sectors can complement a specific sector’s knowledge and thus facilitate innovation. Since the work of Glaeser et al. (Citation1992), a large literature has been devoted to examine the importance of local spillovers for knowledge creation and innovation (see Beaudry & Schiffauerova, Citation2009; and Groot et al., Citation2016, for literature reviews).
One common finding in this literature is that knowledge spillovers are sensitive to geographical distance (Andersson et al., Citation2016; Carlino & Kerr, Citation2015). Thus, a large share of the literature has primarily focused on the importance of local knowledge spillovers for innovation (Anselin et al., Citation1997; Buzard et al., Citation2020; Feldman, Citation1994; Jaffe, Citation1986; Jaffe et al., Citation1993; Lin, Citation2014; Murata et al., Citation2014). The general conclusion is that local knowledge flows are crucial for innovation.
Nevertheless, too much reliance on local knowledge without external ties can cause territorial lock-in effects which are harmful for innovation (Boschma, Citation2005). Thus, a branch of the literature has paid attention to knowledge emanating from geographically distant sources (Ascani et al., Citation2020; Balland & Boschma, Citation2021; Bathelt et al., Citation2004; Caragliu & Nijkamp, Citation2015; Owen-Smith & Powell, Citation2004; Trippl et al., Citation2009). These studies treat the access to external knowledge as a way to avoid regional lock-ins. The literature has demonstrated that firms with limited access to distant knowledge pools tend to be less innovative and generate less output than their peers (Feldman & Kogler, Citation2010; Moreno & Miguélez, Citation2012). For Maskell et al. (Citation2006), it is the complementarity between the local knowledge pool and distant sources of knowledge that will promote regional innovation growth. Because each region has its own industry mix and exploits local and distant knowledge pools differently (Feldman & Kogler, Citation2010), one should expect the relative role of distant intra- and inter-sectoral knowledge spillovers on local innovation to vary across sectors.
Sectoral heterogeneity and knowledge spillovers
A handful of studies focus on the differences in regional knowledge production across sectors. Using US state-level data, the seminal work of Jaffe (Citation1989) investigates the influence of university research on corporate patents across four different sectors. He finds that the drugs, chemical and mechanical sectors benefit from intra-sectoral university research that is taking place locally. Based on metropolitan statistical areas (MSAs) data, Anselin et al. (Citation2000) also investigate how local (within the MSA) university research spills over to four industrial sectors and, unlike the previous study, highlight that interregional (beyond the MSA boundaries) spillovers of university research play a key role for some sectors. The authors find that the local effects from university research for the drugs and chemical sector are not significant, but the interregional externalities have a strong impact in innovation. Their findings indicate also that while the Electronic and Instruments sectors enjoy significant local and interregional spillovers, the Machinery sector is the only one that benefits from long-distance research spillovers.
One element that is missing from the aforementioned studies is the presence of spillovers of private R&D, which have been found to promote innovation as well (Mukim, Citation2012; Orlando, Citation2004; Wallsten, Citation2001). Autant-Bernard and LeSage (Citation2011) account for the capacity of both private and public intra- and interregional R&D spillovers to promote knowledge across 11 sectors of 94 French regions. They conclude that Jacobian externalities dominate MAR externalities when they emanate from private R&D efforts. Local Jacobs and MAR externalities have roughly a similar role on innovation when they come from public R&D efforts, while at the interregional level only the MAR externalities matter. However, they do not account for heterogeneity across sectors. In addition, the spatial extent of the knowledge spillovers is modelled on geographical contiguity. Thus, neither the geographical extent nor the directionality of the knowledge flows is captured. In a follow-up study, Autant-Bernard and LeSage (Citation2019) estimate region-specific knowledge production functions in French regions for four different sectors. They reveal the existence of large sectoral heterogeneity, but they do not focus on the role of intra- and inter-sectoral knowledge spillovers. Malerba et al. (Citation2013) study and compare the importance of intra-sectoral, inter-sectoral combined with national and international knowledge spillovers for knowledge creation for three industries in six European countries (chemicals, electronics and machinery). Their results confirm that there are large differences across industries. For instance, the chemical sector benefits the most from national inter-sectoral spillovers while in the electronics industry intra-sectoral and international spillovers are the most important. They also conclude that, in general, intra-sectoral spillovers are less sensitive to geographical distance than intersectoral ones. Nevertheless, no analysis is provided on the importance of within-country geography.
A recent study focusing on the innovation network within and across sectors by Acemoglu et al. (Citation2016) examines the 1975–84 patent citation network in the United States. The authors find that most of the patents are cited within the sector to which they belong. Intersectoral spillovers take place mostly within the parent sector subsectors belong to (e.g., computer peripherals citing from computer communication) and in a few cases across parent sectors (e.g., chemical sector citing from the drug and medical sector). However, their contribution does not provide a sense of the geographical extent of these spillovers as the results are exclusively at the national level. Finally, based on a matrix of patent creation–patent citation, Cai and Li (Citation2018) argue for the role of inter-sectoral spillovers in knowledge creation. But they also find that there is a strong sectoral heterogeneity in the marginal effect of the inter-sectoral spillovers, where some sectors are heavily cited while others are not. As such, this paper contributes to this literature by tracking the various types (intra- versus interregional, intra- versus intersectoral, private versus public R&D) of knowledge flows that exist.
EMPIRICAL MODEL AND DATA
Regional knowledge production function and Tobit model
Our empirical model relies on a regional approach of Griliches’ (Citation1979) knowledge production function using US county-level panel data. The knowledge production function is assumed to follow a Cobb–Douglas functional form as depicted in equation (1), where is the knowledge output of sector h in county i at time t;
is the kth knowledge input; and
is the elasticity of the output with respect to the corresponding input. In addition,
represent state and time fixed effects as well as an error term, respectively.
(1)
(1) The logarithm transformation of equation (1) leads to a log-linear model that is widely used in empirical studies of the knowledge production function (Acs et al., Citation2002; Anselin et al., Citation1997; Bode, Citation2004; Fischer & Varga, Citation2003).
As usual in the literature, we use patent data as a proxy for knowledge output (Autant-Bernard & LeSage, Citation2011; Parent & LeSage, Citation2008) and work with patent applications (Cincera, Citation1997; Ramani et al., Citation2008) instead of granted patents because the former are closer in time to knowledge creation. Patent application data are retrieved from the database constructed by Lai et al. (Citation2013).Footnote1 To allocate the patent data across counties, we use the fractional counting method suggested by Jaffe et al. (Citation1993). When a patent is created by N inventors, 1/N fraction of the patent is attributed to each inventor. Each 1/N fractional patent is geocoded to its associated county based on the address of the inventor. As a result, the patent data are a rational number.Footnote2 Besides location, we also focus on the knowledge created across five manufacturing sectors: (1) chemical, (2) drugs and medical, (3) mechanical, (4) computer and communication, and (5) electrical and electronic. Thus, we classify the patent applications into these five sectors based on the North American Industry Classification System (NAICS) defined in 2002 ().Footnote3
Table 1. Classification of industrial sectors.
According to Kang and Dall’erba (Citation2016a, Citation2016b), the metropolitan regions have a greater propensity to innovate and their knowledge production mechanism is different from that of the non-metropolitan regions. Thus, we only focus on the metropolitan counties. There were 853 of them across the 3109 continental US counties in 2000.
Since the minimum value of observed patent data is 0,Footnote4 we rely on a Tobit model for our empirical estimation (Cameron & Trivedi, Citation2009), where is the unobservable latent value of patent application; and
is the observed patent application, so that:
The second reason for the choice of a Tobit model comes from Autant-Bernard and LeSage (Citation2011), who argue that patenting is an uncertain process. Even if R&D investments and innovation take place, patenting is a strategic decision that may or may not happen as it depends on other factors such as the cost and benefits of filing. Because we expect unobserved spatial heterogeneity to be present in our panel dataset that covers the period 2001–08, we specify our panel data Tobit model with state and time fixed effects as follows:
Following Wooldridge (Citation2010, p. 709), we use a conditional Tobit model with time and state fixed effects. We justify the use of the time fixed effects through the surge in innovative activities that three of the five sectors have experienced over the study period. Including state fixed effects allows us to control for differences in state-level policies and innovative milieus. One example is enterprise zones (Ham et al., Citation2011), but many states also have R&D tax credit incentives (Wilson, Citation2009), state-specific corporate tax rates and non-compete laws (Greenstone & Looney, Citation2011).
Among the independent variables, the stock of knowledge is a major factor of the knowledge production function (Griliches, Citation1979). Here, the county-level knowledge stock is modelled through lagged expenditures in R&D using the perpetual inventory method (equation 2) as in Mancusi (Citation2008). In equation (2), and
represent the stock of knowledge and the R&D expenditure in county i, sector h at time t. All R&D expenditures are converted in constant 2008 US dollars using each sector’s producer price index (PPI) from the US Bureau of Labor Statistics.Footnote5 We assume a 15% depreciation rate (
) following Okubo et al. (Citation2006) and Mancusi (Citation2008). To calculate the knowledge stock of the initial year, we approximate the industry specific growth rate of R&D expenditures (
) by the average of the annual growth rate over the period 1990–99 across the US continental counties. This approach is used for each individual sector as in Mancusi (Citation2008).
(2)
(2) We model two types of regional knowledge stocks: private and academic R&D. The private knowledge stock in sector h (
) is approximated by the R&D expenditure of private companies collected from Standard & Poor’s Compustat (Standard & Poor’s, Citation2011). The dataset from Lai et al. (Citation2013) links the raw assignee from patent records with the name and address of the assignee. Here we use the address of these companies and their NAICS codes to allocate the R&D expenditures across counties and sectors.
captures R&D expenditures in the four sectors q which are not the sector of interest h. Thus, if significant, the coefficients associated with
and
measure the importance of intra- and inter-industry externalities on knowledge creation, respectively.
The regional academic knowledge stock () is measured by the total amount of R&D spent across universities and colleges according to the National Science Foundation’s (NSF) Survey of R&D expenditures (National Center for Science and Engineering Statistics, Citation2013). In order to match this type of expenditure to a specific county, we use the address of the institutions. Since one academic field can contribute to several of the five economic sectors under study (e.g., electrical engineering is relevant to mechanical, computer and communication, and electrical and electronic), it is difficult to distinguish between intra- and inter-sectoral externalities emanating from the academia and therefore we sum all academic R&D expenditures.
Besides R&D, it is well known that human capital plays an important role in knowledge creation (Audretsch & Feldman, Citation2004). To measure the level of human capital available by county and industrial sector, we use the share of graduate (master’s and doctoral) or professional degree holders who are 25 years and over relative to the total employees (). Following the previous literature, we allow for the time needed for the innovation inputs to translate into output by including a one-year lag between the dependent variable and all independent variables (Fritsch & Slavtchev, Citation2011; Wang et al., Citation2016). The data come from the 2000–07 Integrated Public Use Microdata Series (IPUMS) developed by Ruggles et al. (Citation2010). IPUMS is surveyed based on the public use microdata area (PUMA), thus we match the location of the PUMA with that of the counties based on their 2000 US Census boundaries.Footnote6
In addition, we control for several county-specific conditions. Regional differences in the size of each sector are captured by the total number of employees in each sector () as in Bode (Citation2004). This variable is constructed based on the same data as the human capital variable. We also include the share of establishments with at least 500 employees (
) in order to account for the role of firm size on innovation (Acs et al., Citation1994; Acs & Armington, Citation2004). This cut-off is used by the 2000 County Business Patterns to define small businesses and it has been used for similar purposes by Acs and Audretsch (Citation1988) and Anselin et al. (Citation1997).
The degree of industrial diversity (Glaeser et al., Citation1992) is also included to control for the economic structure of each locality (). It is measured through the inverse of the Herfindahl–Hirschman index (Ruffner & Spescha, Citation2018).Footnote7 This variable is necessary to capture the net effect of MAR versus Jacobs externalities on innovation. Its calculation is reported in equation (3), where
is the share of industry h in county i’s employment at time t – 1. The number of employees is measured across 13 industries.Footnote8 Note that both variables
and
measure the structure of the region. They therefore change over time and space but not by sector.
(3)
(3) In addition, we account for (1) intra-regional and inter-sectoral spillovers, (2) interregional and intra-sectoral spillovers, and (3) interregional and inter-sectoral spillovers. Intra-regional and intra-sectoral spillovers are already accounted for in
since they capture expenses within the same county and sector as the dependent variable. We decide to have the intersectoral knowledge spillovers emanate from private R&D expenses only because, as noted above, university R&D spending in one academic field can contribute to innovation across several sectors.
The three types of spillovers above are modelled based on Lai et al. (Citation2013). Since these data allow us to track the patent creation–citation flows between all 3109 US continental counties as well as the industrial sector of both the cited and citing patents, we first construct 25 (5 × 5 sectors) technological network matrices across the 3109 × 3109 counties and then use the 853 × 3109 submatrices to capture the knowledge flowing to the 853 metropolitan counties only. The fractional counting method is used here too so that we capture all 1/(O × D) knowledge flows between the number of inventors at the place origin O and their peers at the destination D for any pair of origin–destination sectors. This patent creation–patent citation matrix is noted . It represents the citation flows from sector q county j to sector h in the MSA county i. This matrix is the basis for the intraregional spillovers as well as the interregional spillovers (below 50 and above 50 miles, respectively) that will be described further below. Here we use the sum of the patent citation flows over the period 1997–2000 to model the spillovers of knowledge stocks every single year over 2001–03 and the sum of the patent citation flows over 2001–03 for the spillovers measured each year over 2004–08. It is important that the two windows regarding the patent citation flows are neither too short nor too long. The reason is that a short window leads to a low number of citations because there is not enough time to allow it to be cited. On the other hand, if the period is too long, we cannot ensure that the patents from too far back are still relevant in today’s knowledge creation. This split also ensures that there is no overlap between the two creation–citation matrices and that their time lag compared to the dependent variable avoids any bias coming from reverse causality. We do not rely on past data for the measurement of the stock of knowledge created outside of j (
) because the same 15% depreciation rate used in equation (2) applies to R&D expenditure in j and obsolete R&D is not expected to have any impact on knowledge creation.
We make use of matrix above to model first the intra-regional and inter-sectoral spillovers from private knowledge stock (intra-regional and inter-sectoral externalities are noted
in our tables of results for simplicity) as follows:
(4)
(4) Following Kang and Dall’erba (Citation2016a), we normalize the column sums of this matrix to represent the frequency of the citation flows from sector q to h within the MSA county i.
The interregional and intra-sectoral spillovers are modelled as in equations (5) and (6). We distinguish the singular role of short- versus long-distance interregional knowledge spillovers. The former (equation 5) have a spatial extent limited to 50 miles as in Anselin et al. (Citation1997) and Mukherji and Silberman (Citation2013) since it corresponds to the average daily US commuting distance (Smallen, Citation2004). Distant interregional spillovers (equation 6) correspond to externalities taking place from 50 miles to any farther counties. Distances are based on the great circle distance between the centroids of counties i and j.
(5)
(5)
(6)
(6) where
is also column standardized so it captures the share of patents created in i that cite and rely on patents previously created in j.
Interregional Jacobian externalities below that threshold or
and above it (
) are built on the same model as equations (5) and (6), but they are captured through the normalized patent creation–citation flows
from the other four sectors to sector h
The same holds true for the definition of the local spillovers (noted
), short-distance (
or
) and long-distance (
) spillovers of academic knowledge although, as mentioned above, they are not disaggregated by sector. shows the descriptive statistics for all the variables.
Table 2. Descriptive statistics: five manufacturing sectors.
In summary, the full model we estimate can be written as:
(7)
(7) where
;
and
and
are state and time fixed effects, respectively.
ESTIMATION RESULTS
shows the maximum likelihood estimation results of the fixed-effects Tobit models with a 50-mile distance cut-off. The Hausman test is significant across all specifications, indicating that the state and time fixed effect model is preferred over the random effect model. It confirms our expectations that the covariates are not uncorrelated with the fixed effects. We also report , the estimate of the standard deviation of
.
Table 3. Fixed effects Tobit model with 50-mile distance cut-off spillovers.
All specifications indicate that the local stock of private R&D leads to significant and positive intra-regional intra-sectoral () and inter-sectoral (
) externalities on regional knowledge creation. Moreover, the latter displays a greater elasticity than the former at the 5% significance level (one-tailed test) for all sectors but drugs and medical. These results confirm the importance of geographical proximity and associated face-to-face interactions to facilitate knowledge creation and knowledge spillovers (Anselin et al., Citation1997; Buzard et al., Citation2020; Feldman, Citation1994; Jaffe, Citation1986; Jaffe et al., Citation1993; Lin, Citation2014; Murata et al., Citation2014). In addition, our results confirm our expectations by reporting that spending in academic R&D promotes knowledge creation (Anselin et al., Citation2000; Jaffe, Citation1989; Kang & Dall’erba, Citation2016a).
We also find a significant positive role of graduates and employees in a sector on the patenting activity of the same sector. Finally, we find that the greater is the presence of large establishments the more knowledge is created across all sectors but particularly in computer and communication. Acs and Armington (Citation2004) indicate that large firms lead to a greater local labour pool which contributes to agglomeration economies and, as seen above, innovation. The industrial diversity within the county also shows a positive effect on the patenting activity for all sectors, in line with the existing literature on agglomeration economies (Groot et al., Citation2016; Mukim, Citation2012).
The results related to all types of interregional spillovers appear in the middle part of . Unlike the marginal effect of the local variables, spillovers display different magnitudes and significance levels across sectors. For instance, the chemical industry is the only one that benefits from short-distance intra-sectoral spillovers. Long-distance spillovers, however, do not display a significant role in that industry (column 1). This result reveals the dominance of localized face-to-face contacts in the chemical industry as highlighted by Mariani (Citation2000), Autant-Bernard and LeSage (Citation2019) and Malerba et al. (Citation2013). Ernst et al. (Citation2000) justify it by the fact that, in that sector, few key inventors are responsible for a large part of the firm’s technological progress. On the other hand, both short- and long-distance intersectoral spillovers have a significant impact on patenting in the drugs and medical industry. This result indicates that close geographical proximity is not a requirement to transfer basic research knowledge in this sector. This result is in tune with the findings of Gittelman (Citation2007) and Cardinal and Hatfield (Citation2000) who indicate that the collaboration network of the US biotechnology industry and the US pharmaceutical industry, respectively, is spread geographically. Brown and Duguid (Citation1998) highlight the reason for it; new drugs rely on numerous groups of researchers highly specialized in specific aspects of basic or fundamental research. In addition, our results indicate that this sector’s innovation process relies heavily on knowledge from other sectors. Henderson (Citation1994) and Spilker (Citation1989) indicate that, as drug research becomes more complex, it relies increasingly on a broader array of scientific fields such as chemistry, toxicology, pharmacology and biology; hence, the intersectoral nature (mostly with the chemical industry) of the spillovers we find significant. For instance, Spilker (Citation1989) finds that for every 10,000 chemical compounds synthesized, only one will end up approved for commercial introduction.
The results for the mechanical sector show a slightly different picture as they benefit from long-distance intra-sectoral spillovers and short-distance university knowledge externalities. In the United States, the link between academic research and this field has a long history that started with the 1862 Morrill Act, which granted land for the establishment of one college focusing on agriculture and mechanical engineering per state. This link carries on today through initiatives such as the Industry–University Cooperative Research Centers or other funding from the NSF and has been fostered through the 1980 Bayh–Dole Act that let universities retain ownership of the inventions that result from federal funding.
At the same time, the significance of long-distance intra-sectoral spillovers reflects the highly organized input–output supply chain systems typical of this industry (Markusen, Citation1996). A well-known example is the highly fragmented and cross-border production sharing process in the automobile industry (Türkcan & Ates, Citation2011).
Finally, our results indicate that the two remaining industries, computer and communications, and electrical and electronic, benefit from short-distance university spillovers as well as from short-distance intersectoral spillovers emanating from the private sector. The first finding corroborates with Mansfield (Citation1995) who highlights that geographical proximity of academic research plays a greater role in these industries as they require both basic and applied R&D. Both fields are supported by government foundations such as the NSF to promote public–private partnerships and both fields are major contributors of spin-out companies from the university (Shah & Pahnke, Citation2014; Smilor et al., Citation1990). We also find that, for both industries, the magnitude of short-distance interregional spillovers is relatively similar to the local ones, which reflects that companies rely on a network that extends to the counties nearby. These results are in line with those of Kang and Dall’erba (Citation2016b) and Anselin et al. (Citation2000). Finally, long-distance intersectoral spillovers also play a significant role in the computer and communications industry and we suspect that most of them come from the electrical and electronic industry.
Before we conclude, we perform one robustness check on the fixed effects Tobit model by pooling the interregional spillovers above and below 50 miles. The assumption is that, for some sectors, the 50-mile cut-off may not be relevant and could mask the significant role of spillovers covering a greater distance. The summary estimation results of pooling interregional spillovers are displayed in .Footnote9 The marginal effects estimated for the variables are similar to those found in . For instance, the intra-sectoral spillovers in the mechanical sectors and, to a lower degree, in the chemical sector are still significant. We also find that intersectoral spillovers
have a significant role in patenting in drugs and medical, computer and communication, and electrical and electronic. Based on the results of , long- but mostly short-distance spillovers were driving the finding for these industries. When it comes to academic interregional spillovers, the results indicate they positively affect patenting in the electrical and electronic sector, which corroborates with the findings in where short-distance spillovers had a somewhat more significant and greater role on patenting than long-distance university spillovers.Footnote10
Table 4. Summary estimation results of pooled interregional spillovers.
CONCLUSIONS
The regional knowledge production literature has given an increasing amount of attention to the role of spatial spillovers on knowledge creation. However, the bulk of empirical studies relies on an aggregated approach that masks the differences in the marginal effect of intra-sectoral and intersectoral R&D investments on knowledge creation across sectors. The few exceptions (e.g., Jaffe, Citation1989; Anselin et al., Citation2000) have highlighted the presence of sectoral heterogeneity in the size of the localized knowledge spillovers emanating from university research. However, they have not investigated how intersectoral and distant interregional knowledge spillovers matter. Autant-Bernard and LeSage (Citation2011) have remedied this problem but at the cost of providing estimates averaged across all sectors. This paper contributes to this literature by examining the heterogeneous role of intra- and interregional as well as intra- and inter-sectoral knowledge spillovers across 5 US manufacturing sectors that cover 82% of the patents recorded in the USPTO. In addition, interregional spillovers are measured through a matrix of patent creation – patent citation as in Peri (Citation2005) and Kang and Dall’erba (Citation2016a) that allows us to explicitly account for the directionality of the flows of knowledge.
Measured over 853 US metropolitan counties and in the frame of a Tobit model with state fixed effects, our results show that both intra- and inter-sectoral spillovers taking place within a county are important determinants of knowledge production. It implies that frequent face-to-face contacts are still an important factor for the creation of new knowledge. When it comes to the interregional spillovers of private and university knowledge, the relative role of each type depends on the industry under study suggesting that there is strong heterogeneity across sectors on the mechanisms of how new knowledge is created. These differences are not only visible in terms of different sensitivity to geographical proximity, but also depending on whether spillovers arise from the same industry, from others and/or from academic research.
Our estimation results suggest two important implications for policymakers. First, because technology developed in the academia matters a great deal for the innovative capacity of each industry, policy makers need to facilitate local and nationwide spillovers of academic R&D through, for instance, grants, tax breaks (Ponds et al., Citation2010), university–industry cooperative research (Fukugawa, Citation2013), and science and technology parks (Díez-Vial & Fernández-Olmos, Citation2015). Second, our work indicates that both intra- and inter-sectoral externalities matter for innovation. While the bulk of the innovation literature started with a focus on intra-sectoral externalities, evidence that technology diffuses across industry is now well accepted but seldom measured. Among our results, it is surprising to see how much the stock of intersectoral knowledge, whether local or from other regions, affects patenting in some industries (drugs and medical, computer and communication, and electrical and electronic). Furthermore, the spatial extent of the interregional spillovers that affect patenting differ by industry. For instance, innovation in the chemical and electrical and electronic industries is not affected by long-distance private R&D spillovers while it is in other industries. Therefore, recommendations based on the traditional, aggregated, approach mask this underlying heterogeneity and can lead to inappropriate conclusions. As a result, future policies should identify the broad array of scientific fields and the spatial extent of the network linkages these industries rely on for their innovation process before implementing new programmes and instruments.
While these results contribute to the existing literature on knowledge spillovers and innovation, this study has several limitations that, in turn, create promising avenues for future work. First, our database does not include the service sector even though it can also be a patent-producing sector. Understanding the dynamics of that sector is therefore highly important for urban and regional growth and productivity (Moyart, Citation2005; Zhang, Citation2016). Second, the degree of (dis)aggregation of our sectors follows the availability of the data. More disaggregated information would lead to more accurate results and policy recommendations. Third, our results rely on US data and the innovation dynamics within that country. Data at the international level would allow us to compare our findings with those of other countries and to include flows of patent citation/creation between US and foreign firms. Last, while patent creation/citation flows leave a paper trail of knowledge spillovers that is easy to quantify, other mechanisms might also be at play. For example, knowledge spillovers can happen due to labour mobility of the skilled workers (Almeida & Kogut, Citation1999; Kekezi & Klaesson, Citation2020), non-market interactions (Glaeser & Scheinkman, Citation2000), technological proximity (Maggioni et al., Citation2011), or social networks (Crescenzi et al., Citation2016). While some of these mechanisms are embedded in the short-distance spillovers we define in our study, quantifying their individual characteristics would clarify their role in the innovation process.
Supplemental Material
Download MS Word (28.6 KB)ACKNOWLEDGEMENT
The authors thank the participants at the 2017 Agricultural and Applied Economics Annual Meeting, the 2017 North American Regional Science Association meeting, and the 55th Annual Meeting of Western Regional Science Association for their feedback to a previous version of this manuscript.
DISCLOSURE STATEMENT
No potential conflict of interest was reported by the authors.
Additional information
Funding
Notes
1 For the files, see https://github.com/funginstitute/downloads/.
2 We rely on the USPCS-NAICS 2002 concordance file developed by the USPTO to transfer the data in NAICS format.
3 The geographical allocation of the patent data could also be based on the address of the assignee(s). However, large companies use the address of their headquarters to file patents, which is not always the place where the research took place. Using the inventor’s address to geocode the creation and citation of patent data can lead to a similar problem (Autant-Bernard & LeSage, Citation2011), but the size of the error is smaller as we assume that inventors live close to their workplace.
4 If the variables have a minimum value of 0, we add a 1 before log transformation.
5 The average of the PPI of the NAICS sectors 1 is used to calculate the annual PPI of each of our five sectors.
6 If a PUMA area consists of more than one county, we allocate the number of degree holders of each sector proportionally to the counties’ total number of degree holders (for all sectors) of which data come from the 2000 US Census. This approach is used for 2000, 2005, 2006 and 2007. The IPUMS provides personal information at the state level only during the period 2001–04. Thus, we first calculate the total sum of degree holders by state and by sector and then distribute it across counties proportionally to their average number of degree holders between 2000 and 2005.
7 The results are robust to other diversity measures, such as those presented by Duranton and Puga (Citation2000).
8 The 13 industries are based on the 2000 US Census classification: (1) Agriculture, forestry, fishing and hunting, and mining; (2) Construction; (3) Manufacturing; (4) Wholesale trade; (5) Retail trade; (6) Transportation and warehousing, and utilities; (7) Information; (8) Finance, insurance, real estate, and rental and leasing; (9) Professional, scientific, management, administrative, and waste management services; (10) Educational, health and social services; (11) Arts, entertainment, recreation, accommodation and food services; (12) Other services (except public administration); and (13) Public administration or industries not classified. We use the 2000 US Census for the Herfindahl and Hirschman index of 2000 and the County Business Patterns for the index over the period 2003–07. For 2002, the Census Bureau does not provide the number of employees for several sectors. We fill these data with the corresponding values from the 2003 County Business Patterns data and use an average of 2000 and 2002 as a proxy for 2001.
9 Table A1 in the supplemental data online shows the full estimation results.
10 We tested another robustness check whereby the distance cut-off moves from 50 to 75 miles to account for ‘stretch commuters’ (Smallen, Citation2004). All the results are very consistent with those displayed in ; hence, they are not reported but available from the authors upon request.
REFERENCES
- Acemoglu, D., Akcigit, U., & Kerr, W. R. (2016). Innovation network. Proceedings of the National Academy of Sciences, 113(41), 11483–11488. https://doi.org/https://doi.org/10.1073/pnas.1613559113
- Acs, Z., Anselin, L., & Varga, A. (2002). Patents and innovation counts as measures of regional production of new knowledge. Research Policy, 31(7), 1069–1085. https://doi.org/https://doi.org/10.1016/S0048-7333(01)00184-6
- Acs, Z., & Armington, C. (2004). Employment growth and entrepreneurial activity in cities. Regional Studies, 38(8), 911–927. https://doi.org/https://doi.org/10.1080/0034340042000280938
- Acs, Z. J., & Audretsch, D. B. (1988). Innovation in large and small firms: An empirical analysis. The American Economic Review, 78(4), 678–690. https://doi.org/https://doi.org/10.2307/1811167
- Acs, Z. J., Audretsch, D. B., & Feldman, M. P. (1994). R&D spillovers and recipient firm size. The Review of Economics and Statistics, 76(2), 336–340. https://doi.org/https://doi.org/10.2307/2109888
- Almeida, P., & Kogut, B. (1999). Localization of knowledge and the mobility of engineers in regional networks. Management Science, 45(7), 905–917. https://doi.org/https://doi.org/10.1287/mnsc.45.7.905
- Andersson, M., Klaesson, J., & Larsson, J. P. (2016). How local are spatial density externalities? Neighbourhood effects in agglomeration economies. Regional Studies, 50(6), 1082–1095. https://doi.org/https://doi.org/10.1080/00343404.2014.968119
- Anselin, L., Varga, A., & Acs, Z. (1997). Local geographic spillovers between university research and high technology innovations. Journal of Urban Economics, 42(3), 422–448. https://doi.org/https://doi.org/10.1006/juec.1997.2032
- Anselin, L., Varga, A., & Acs, Z. (2000). Geographical spillovers and university research: A spatial econometric perspective. Growth and Change, 31(4), 501–515. https://doi.org/https://doi.org/10.1111/0017-4815.00142
- Ascani, A., Bettarelli, L., Resmini, L., & Balland, P.-A. (2020). Global networks, local specialisation and regional patterns of innovation. Research Policy, 49(8), 104031. https://doi.org/https://doi.org/10.1016/j.respol.2020.104031
- Audretsch, D., & Feldman, M. (2004). Knowledge spillovers and the geography of innovation. In J. V. Henderson & J.-F. Thisse (Eds.), Handbook of regional and urban economics (pp. 2713–2739). Elsevier.
- Audretsch, D. B., & Belitski, M. (2020). The role of R&D and knowledge spillovers in innovation and productivity. European Economic Review, 123, 103391. https://doi.org/https://doi.org/10.1016/j.euroecorev.2020.103391
- Autant-Bernard, C. (2012). Spatial econometrics of innovation: Recent contributions and research perspectives. Spatial Economic Analysis, 7(4), 403–419. https://doi.org/https://doi.org/10.1080/17421772.2012.722665
- Autant-Bernard, C., & LeSage, J. (2011). Quantifying knowledge spillovers using spatial econometric models. Journal of Regional Science, 51(3), 471–496. https://doi.org/https://doi.org/10.1111/j.1467-9787.2010.00705.x
- Autant-Bernard, C., & LeSage, J. P. (2019). A heterogeneous coefficient approach to the knowledge production function. Spatial Economic Analysis, 14(2), 196–218. https://doi.org/https://doi.org/10.1080/17421772.2019.1562201
- Autor, D., Dorn, D., Hanson, G. H., Pisano, G., & Shu, P. (2016). Foreign competition and domestic innovation: Evidence from US patents. National Bureau of Economic Research.
- Balland, P.-A., & Boschma, R. (2021). Complementary interregional linkages and smart specialisation: An empirical study on European regions. Regional Studies, 55(6), 1059–1070. https://doi.org/https://doi.org/10.1080/00343404.2020.1861240
- Bathelt, H., Malmberg, A., & Maskell, P. (2004). Clusters and knowledge: Local buzz, global pipelines and the process of knowledge creation. Progress in Human Geography, 28(1), 31–56. https://doi.org/https://doi.org/10.1191/0309132504ph469oa
- Baum, C. F., Lööf, H., Nabavi, P., & Stephan, A. (2017). A new approach to estimation of the R&D–innovation–productivity relationship. Economics of Innovation and New Technology, 26(1–2), 121–133. https://doi.org/https://doi.org/10.1080/10438599.2016.1202515
- Beaudry, C., & Schiffauerova, A. (2009). Who’s right, Marshall or Jacobs? The localization versus urbanization debate. Research Policy, 38(2), 318–337. https://doi.org/https://doi.org/10.1016/j.respol.2008.11.010
- Bode, E. (2004). The spatial pattern of localized R&D spillovers: An empirical investigation for Germany. Journal of Economic Geography, 4(1), 43–64. https://doi.org/https://doi.org/10.1093/jeg/4.1.43
- Boschma, R. (2005). Proximity and innovation: A critical assessment. Regional Studies, 39(1), 61–74. https://doi.org/https://doi.org/10.1080/0034340052000320887
- Brown, J. S., & Duguid, P. (1998). Organizing knowledge. California Management Review, 40(3), 90–111. https://doi.org/https://doi.org/10.2307/41165945
- Buzard, K., Carlino, G. A., Hunt, R. M., Carr, J. K., & Smith, T. E. (2020). Localized knowledge spillovers: Evidence from the spatial clustering of R&D labs and patent citations. Regional Science and Urban Economics, 81, 103490. https://doi.org/https://doi.org/10.1016/j.regsciurbeco.2019.103490
- Cai, J., & Li, N. (2018). Growth through inter-sectoral knowledge linkages. The Review of Economic Studies, 86(5), 1827–1866. https://doi.org/https://doi.org/10.1093/restud/rdy062
- Cameron, A. C., & Trivedi, P. K. (2009). Microeconometrics using Stata (Vol. 5). Stata Press.
- Caragliu, A., & Nijkamp, P. (2015). Space and knowledge spillovers in European regions: The impact of different forms of proximity on spatial knowledge diffusion. Journal of Economic Geography, 16(3), 749–774. https://doi.org/https://doi.org/10.1093/jeg/lbv042
- Cardinal, L. B., & Hatfield, D. E. (2000). Internal knowledge generation: The research laboratory and innovative productivity in the pharmaceutical industry. Journal of Engineering and Technology Management, 17(3), 247–271. https://doi.org/https://doi.org/10.1016/S0923-4748(00)00025-4
- Carlino, G., & Kerr, W. R. (2015). Chapter 6 – Agglomeration and innovation. In G. Duranton, J. V. Henderson, & W. C. Strange (Eds.), Handbook of regional and urban economics (pp. 349–404). Elsevier.
- Cincera, M. (1997). Patents, R&D, and technological spillovers at the firm level: Some evidence from econometric count models for panel data. Journal of Applied Econometrics, 12(3), 265–280. https://doi.org/https://doi.org/10.1002/(SICI)1099-1255(199705)12:33.0.CO;2-J
- Crescenzi, R., Nathan, M., & Rodríguez-Pose, A. (2016). Do inventors talk to strangers? On proximity and collaborative knowledge creation. Research Policy, 45(1), 177–194. https://doi.org/https://doi.org/10.1016/j.respol.2015.07.003
- Díez-Vial, I., & Fernández-Olmos, M. (2015). Knowledge spillovers in science and technology parks: how can firms benefit most. The Journal of Technology Transfer, 40(1), 70–84.
- Duranton, G., & Puga, D. (2000). Diversity and specialisation in cities: Why, where and when does it matter? Urban Studies, 37(3), 533–555. https://doi.org/https://doi.org/10.1080/0042098002104
- Ernst, H., Leptien, C., & Vitt, J. (2000). Inventors are not alike: The distribution of patenting output among industrial R&D personnel. IEEE Transactions on Engineering Management, 47(2), 184–199. https://doi.org/https://doi.org/10.1109/17.846786
- Fallick, B., Fleischman, C. A., & Rebitzer, J. B. (2006). Job-hopping in Silicon Valley: Some evidence concerning the microfoundations of a high-technology cluster. Review of Economics and Statistics, 88(3), 472–481. https://doi.org/https://doi.org/10.1162/rest.88.3.472
- Feldman, M., & Kogler, D. (2010). Chapter 8 – Stylized facts in the geography of innovation. In B. H. Hall & N. Rosenberg (Eds.), Handbook of the economics of innovation (pp. 381–410). North-Holland.
- Feldman, M. P. (1994). The geography of innovation. Kluwer Academic.
- Fischer, M., & Varga, A. (2003). Spatial knowledge spillovers and university research: Evidence from Austria. The Annals of Regional Science, 37(2), 303–322. https://doi.org/https://doi.org/10.1007/s001680200115
- Fritsch, M., & Slavtchev, V. (2011). Determinants of the efficiency of regional innovation systems. Regional Studies, 45(7), 905–918. https://doi.org/https://doi.org/10.1080/00343400802251494
- Fukugawa, N. (2013). University spillovers into small technology-based firms: Channel, mechanism, and geography. The Journal of Technology Transfer, 38(4), 415–431. https://doi.org/https://doi.org/10.1007/s10961-012-9247-x
- Galasso, A., & Schankerman, M. (2018). Patent rights, innovation, and firm exit. The RAND Journal of Economics, 49(1), 64–86. https://doi.org/https://doi.org/10.1111/1756-2171.12219
- Ganguli, I., Lin, J., & Reynolds, N. (2020). The paper trail of knowledge spillovers: Evidence from patent interferences. American Economic Journal: Applied Economics, 12(2), 278–302. https://doi.org/https://doi.org/10.1257/app.20180017
- Gittelman, M. (2007). Does geography matter for science-based firms? Epistemic communities and the geography of research and patenting in biotechnology. Organization Science, 18(4), 724–741. https://doi.org/https://doi.org/10.1287/orsc.1070.0249
- Glaeser, E. L., Kallal, H. D., Scheinkman, J. A., & Shleifer, A. (1992). Growth in cities. Journal of Political Economy, 100(6), 1126–1152. https://doi.org/https://doi.org/10.1086/261856
- Glaeser, E. L., & Scheinkman, J. (2000). Non-market interactions. National Bureau of Economic Research.
- Greenstone, M., & Looney, A. (2011). A dozen economic facts about innovation. The Hamilton Project, 3. https://www.hamiltonproject.org/assets/legacy/files/downloads_and_links/08_innovation_greenstone_looney.pdf
- Griliches, Z. (1979). Issues in assessing the contribution of research and development to productivity growth. The Bell Journal of Economics, 10(1), 92–116. https://doi.org/https://doi.org/10.2307/3003321
- Groot, H. L. F., Poot, J., & Smit, M. J. (2016). Which agglomeration externalities matter most and why? Journal of Economic Surveys, 30(4), 756–782. https://doi.org/https://doi.org/10.1111/joes.12112
- Ham, J. C., Swenson, C., İmrohoroğlu, A., & Song, H. (2011). Government programs can improve local labor markets: Evidence from state enterprise zones, federal empowerment zones and federal enterprise community. Journal of Public Economics, 95(7), 779–797. https://doi.org/https://doi.org/10.1016/j.jpubeco.2010.11.027
- Henderson, R. (1994). The evolution of integrative capability: Innovation in cardiovascular drug discovery. Industrial and Corporate Change, 3(3), 607–630. https://doi.org/https://doi.org/10.1093/icc/3.3.607
- Jacobs, J. (1969). The economy of cities. Random House.
- Jaffe, A. B. (1986). Technological opportunity and spillovers of R&D: Evidence from firms’ patents, profits and market value. American Economic Review, 76, 984–1001. https://doi.org/https://doi.org/10.3386/w1815
- Jaffe, A. B. (1989). Real effects of academic research. The American Economic Review, 79(5), 957–970. http://www.jstor.org/stable/1831431
- Jaffe, A. B., Trajtenberg, M., & Henderson, R. (1993). Geographic localization of knowledge spillovers as evidenced by patent citations. The Quarterly Journal of Economics, 108(3), 577–598. https://doi.org/https://doi.org/10.2307/2118401
- Kang, D., & Dall’erba, S. (2016a). An examination of the role of local and distant knowledge spillovers on the US regional knowledge creation. International Regional Science Review, 39(4), 355–385. https://doi.org/https://doi.org/10.1177/0160017615572888
- Kang, D., & Dall’erba, S. (2016b). Exploring the spatially varying innovation capacity of the US counties in the framework of Griliches’ knowledge production function: A mixed GWR approach. Journal of Geographical Systems, 18(2), 125–157. https://doi.org/https://doi.org/10.1007/s10109-016-0228-8
- Kekezi, O., & Klaesson, J. (2020). Agglomeration and innovation of knowledge intensive business services. Industry and Innovation, 27(5), 538–561. https://doi.org/https://doi.org/10.1080/13662716.2019.1573660
- Kwon, H.-S., Lee, J., Lee, S., & Oh, R. (2020). Knowledge spillovers and patent citations: Trends in geographic localization, 1976–2015. Economics of Innovation and New Technology, 1–25. https://doi.org/https://doi.org/10.1080/10438599.2020.1787001
- Lai, R., D’Amour, A., Yu, A., Sun, Y., & Felming, L. (2013). Disambiguation and co-authorship networks of the U.S. patent inventor database (1975–2010). Harvard Dataverse. https://doi.org/https://doi.org/10.7910/DVN/5F1RRI
- Lin, J. (2014). The paper trail of knowledge transfers. Federal Reserve Bank of Philadelphia Business Review, Second Quarter.
- Maggioni, M. A., Uberti, T. E., & Usai, S. (2011). Treating patents as relational data: Knowledge transfers and spillovers across Italian provinces. Industry and Innovation, 18(1), 39–67. https://doi.org/https://doi.org/10.1080/13662716.2010.528928
- Malerba, F., Mancusi, M. L., & Montobbio, F. (2013). Innovation, international R&D spillovers and the sectoral heterogeneity of knowledge flows. Review of World Economics, 149(4), 697–722. https://doi.org/https://doi.org/10.1007/s10290-013-0167-0
- Mancusi, M. L. (2008). International spillovers and absorptive capacity: A cross-country cross-sector analysis based on patents and citations. Journal of International Economics, 76(2), 155–165. https://doi.org/https://doi.org/10.1016/j.jinteco.2008.06.007
- Mansfield, E. (1995). Academic research underlying industrial innovations: Sources, characteristics, and financing. The Review of Economics and Statistics, 77(1), 55–65. https://doi.org/https://doi.org/10.2307/2109992
- Mariani, M. (2000). Title. WP No. 2/20-019, Economic Research Institute on Innovation and Technology (MERIT).
- Markusen, A. (1996). Sticky places in slippery space: A typology of industrial districts. Economic Geography, 72(3), 293–313. https://doi.org/https://doi.org/10.2307/144402
- Marshall, A. (1890). Principles of economics (Vol. 1). MacMillan.
- Maskell, P., Bathelt, H., & Malmberg, A. (2006). Building global knowledge pipelines: The role of temporary clusters. European Planning Studies, 14(8), 997–1013. https://doi.org/https://doi.org/10.1080/09654310600852332
- Moreno, R., & Miguélez, E. (2012). A relational approach to the geography of innovation: A typology of regions. Journal of Economic Surveys, 26(3), 492–516. https://doi.org/https://doi.org/10.1111/j.1467-6419.2012.00727.x
- Moretti, E., & Wilson, D. J. (2017). The effect of state taxes on the geographical location of top earners: Evidence from star scientists. American Economic Review, 107(7), 1858–1903. https://doi.org/https://doi.org/10.1257/aer.20150508
- Moyart, L. (2005). The role of producer services in regional development: What opportunities for medium-sized cities in Belgium? The Service Industries Journal, 25(2), 213–228. https://doi.org/https://doi.org/10.1080/0264206042000305420
- Mukherji, N., & Silberman, J. (2013). Absorptive capacity, knowledge flows, and innovation in U.S. metropolitan areas. Journal of Regional Science, 53(3), 392–417. https://doi.org/https://doi.org/10.1111/jors.12022
- Mukim, M. (2012). Does agglomeration boost innovation? An econometric evaluation. Spatial Economic Analysis, 7(3), 357–380. https://doi.org/https://doi.org/10.1080/17421772.2012.694142
- Murata, Y., Nakajima, R., Okamoto, R., & Tamura, R. (2014). Localized knowledge spillovers and patent citations: A distance-based approach. Review of Economics and Statistics, 96(5), 967–985. https://doi.org/https://doi.org/10.1162/REST_a_00422
- National Center for Science and Engineering Statistics. (2013). Guide for public use data files national science foundation’s higher education research and development survey: Fiscal year 2011. In Yearly. Virginia NSF National Center for Science and Engineering Statistics. http://www.nsf.gov/statistics/herd/pub_data.cfm
- Neves, P. C., & Sequeira, T. N. (2018). Spillovers in the production of knowledge: A meta-regression analysis. Research Policy, 47(4), 750–767. https://doi.org/https://doi.org/10.1016/j.respol.2018.02.004
- Okubo, S., Robbins, C. A., Moylan, C. E., Sliker, B. K., Schultz, L. I., & Mataloni, L. S. (2006). R&D satellite account: Preliminary estimates. Bureau of Economic Analysis, US Department of Commerce.
- Orlando, M. J. (2004). Measuring spillovers from industrial R&D: On the importance of geographic and technological proximity. The RAND Journal of Economics, 35(4), 777–786. https://doi.org/https://doi.org/10.2307/1593773
- Owen-Smith, J., & Powell, W. W. (2004). Knowledge networks as channels and conduits: The effects of spillovers in the Boston biotechnology community. Organization Science, 15(1), 5–21. https://doi.org/https://doi.org/10.1287/orsc.1030.0054
- Parent, O., & LeSage, J. (2008). Using the variance structure of the conditional autoregressive spatial specification to model knowledge spillovers. Journal of Applied Econometrics, 23(2), 235–256. https://doi.org/https://doi.org/10.1002/jae.981
- Peri, G. (2005). Determinants of knowledge flows and their effect on innovation. Review of Economics and Statistics, 87(2), 308–322. https://doi.org/https://doi.org/10.1162/0034653053970258
- Ponds, R., van Oort, F., & Frenken, K. (2010). Innovation, spillovers and university–industry collaboration: An extended knowledge production function approach. Journal of Economic Geography, 10(2), 231–255. https://doi.org/https://doi.org/10.1093/jeg/lbp036
- Qiu, S., Liu, X., & Gao, T. (2017). Do emerging countries prefer local knowledge or distant knowledge? Spillover effect of university collaborations on local firms. Research Policy, 46(7), 1299–1311. https://doi.org/https://doi.org/10.1016/j.respol.2017.06.001
- Ramani, S. V., El-Aroui, M.-A., & Carrère, M. (2008). On estimating a knowledge production function at the firm and sector level using patent statistics. Research Policy, 37(9), 1568–1578. https://doi.org/https://doi.org/10.1016/j.respol.2008.06.009
- Romer, P. M. (1986). Increasing returns and long-run growth. Journal of Political Economy, 94(5), 1002–1037. https://doi.org/https://doi.org/10.1086/261420
- Ruffner, J., & Spescha, A. (2018). The impact of clustering on firm innovation. CESifo Economic Studies, 64(2), 176–215. https://doi.org/https://doi.org/10.1093/cesifo/ify009
- Ruggles, S., Trent Alexander, J., Genadek, K., Goeken, R., Schroeder, M. B., & Sobek, M. (2010). Integrated public use microdata series (Version 5.0) [Machine-readable database]. University of Minnesota.
- Saxenian, A. (1994). Regional advantage. Harvard University Press.
- Shah, S. K., & Pahnke, E. C. (2014). Parting the ivory curtain: Understanding how universities support a diverse set of startups. The Journal of Technology Transfer, 39(5), 780–792. https://doi.org/https://doi.org/10.1007/s10961-014-9336-0
- Smallen, D. (2004). 3.3 million Americans are stretch commuters traveling at least 50 miles one-way to work. United States Department of Transportation.
- Smilor, R. W., Gibson, D. V., & Dietrich, G. B. (1990). University spin-out companies: Technology start-ups from UT-Austin. Journal of Business Venturing, 5(1), 63–76. https://doi.org/https://doi.org/10.1016/0883-9026(90)90027-Q
- Spilker, B. (1989). Multinational drug companies: Issues in drug discovery and development. Raven press.
- Standard & Poor’s. (2011). Standard & Poor’s Compustat® Xpressfeed understanding the data. The McGraw-Hill Companies.
- Thompson, P. (2006). Patent citations and the geography of knowledge spillovers: Evidence from inventor- and examiner-added citations. Review of Economics and Statistics, 88(2), 383–388. https://doi.org/https://doi.org/10.1162/rest.88.2.383
- Trippl, M., Tödtling, F., & Lengauer, L. (2009). Knowledge sourcing beyond buzz and pipelines: Evidence from the Vienna software sector. Economic Geography, 85(4), 443–462. https://doi.org/https://doi.org/10.1111/j.1944-8287.2009.01047.x
- Türkcan, K., & Ates, A. (2011). Vertical intra-industry trade and fragmentation: An empirical examination of the US auto-parts industry. The World Economy, 34(1), 154–172. https://doi.org/https://doi.org/10.1111/j.1467-9701.2010.01316.x
- US Patent and Trademark Office (USPTO). (2010). Patents BIB: Selected bibliographic information from US patents issued 1969 to present. USPTO.
- Wallsten, S. J. (2001). An empirical test of geographic knowledge spillovers using geographic information systems and firm-level data. Regional Science and Urban Economics, 31(5), 571–599. https://doi.org/https://doi.org/10.1016/S0166-0462(00)00074-0
- Wang, Y., Ning, L., Li, J., & Prevezer, M. (2016). Foreign direct investment spillovers and the geography of innovation in Chinese regions: The role of regional industrial specialization and diversity. Regional Studies, 50(5), 805–822. https://doi.org/https://doi.org/10.1080/00343404.2014.933800
- Wilson, D. J. (2009). Beggar thy neighbor? The in-state, out-of-state, and aggregate effects of R&D tax credits. Review of Economics and Statistics, 91(2), 431–436. https://doi.org/https://doi.org/10.1162/rest.91.2.431
- Wooldridge, J. M. (2010). Econometric analysis of cross section and panel data. MIT Press.
- Zhang, C. (2016). Agglomeration of knowledge intensive business services and urban productivity. Papers in Regional Science, 95(4), 801–818. https://doi.org/https://doi.org/10.1111/pirs.12204