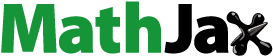
ABSTRACT
Background: The importance of subcutaneous drug delivery using self-injection devices based on common device platforms continues to grow. The resulting broad adoption of potentially look-alike or similar devices, however, raises concerns over limited device distinguishability and ensuing risk of medication errors. The objective of the study is thus to understand whether and how users effectively distinguish between self-injection device variants.
Methods: Seventy-four patients, caregivers, and healthcare professionals were asked to pairwise rate the similarity of eight platform autoinjector variants. Multidimensional scaling was then used to convert individual ratings into spatial configurations and thereby identify the attributes that influence device distinguishability.
Results: Five different device attributes driving distinguishability were identified. Three of the attributes corresponded to single design features (the label color, device size and device shape). Two device attributes (the aspect ratio and chromaticity) combined distinct yet interrelated design features.
Conclusions: The study provides initial empirical evidence that users are able to distinguish between device variants and as to what device attributes drive distinguishability. Furthermore, the results highlight patterns in how various user groups distinguish between device variants. These patterns relate with the user group characteristics (e.g. age, sight or dexterity) and the context of device usage (e.g. healthcare professionals).
1. Introduction
Responding to the soaring number of new biologics and concomitant interest in innovative and easy-to-use devices for subcutaneous drug delivery, self-injection device platforms have recently made fast market inroads and are in the process of fundamentally changing the modus operandi of device development [Citation1,Citation2]. Departing from prior literature [Citation3–Citation6], a self-injection device platform is defined here as a set of sub-systems with a common design pre-tested across users, which facilitates the efficient development and manufacturing of a stream of custom-specific product derivatives. Self-injection device platforms are thus in sharp contrast to specific self-injection devices designed, realized, and industrialized for a single drug product, such as the Humira® Pen, Simponi® Pen, and Genotropin® pen for the administration of Adalimumab, Golimumab, and Somatropin, respectively [Citation7–Citation9]. Recently, the customization of device platforms into commercial device derivatives has emerged as a new dominant logic of device innovation for wearable on-body patch injectors [e.g. Citation10, Citation11, Citation12], dial-and-dose pens [e.g. Citation13, Citation14, Citation15, Citation16, Citation17, Citation18], and syringe-based autoinjectors [e.g. Citation19, Citation20, Citation21, Citation22]. In fact, a large majority of recently launched disposable single-use autoinjectors reflect variants of device platforms (see for a market overview). Industry experts keep re-emphasizing the advantages that such self-injection device platforms offer, such as short time-to-market, attractive cost structures, or proven handling concepts [Citation1,Citation2,Citation23]. However, the high rate of platform adoption raises concerns with respect to the distinguishability between potentially look-alike or similar device variants and the ensuing risk of medication errors. In line with the definition provided by the U. S. Food and Drug Administration, medication errors are defined as preventable events that result in inappropriate medication use or patient harm [Citation24]. Device distinguishability describes the extent to which users are able to effectively perceive differences between self-injection devices and is used in conjunction with the study objective. In contrast, the term similarity refers to the research methodology and the process of data analysis. Device similarity thus inversely relates to device distinguishability (i.e. the more similar, the less distinguishable).
Table 1. Overview of commercial product approvals/launches based on disposable single-use pen and autoinjector device platforms. 24 out of 29 US launches of single-use disposable pre-filled syringe based autoinjectors for subcutaneous drug delivery were based on device platforms to date (as per 24 June 2019). Non-platform-based disposable autoinjector launches were: Humira® (AbbVie), Simponi® (JnJ), Avonex® (Biogen), Sumatriptan (Dr. Reddy’s), and Makena® (AMAG).
There are two scenarios where the distinguishability of platform-based device derivatives is particularly relevant to avoid inappropriate medication use. The first is where a patient is subject to multiple treatments. As the number of chronically ill and multi-morbid patients on continues to grow, and as self-injection devices help shift the point of care from the hospital to the home, lay users become increasingly likely to encounter device variants for different drugs derived from the same basic platform. While medication errors may well occur when healthcare professionals control drug usage [e.g. Citation25, Citation26, Citation27, Citation28], research has shown that the problem is particularly pronounced for therapies where patients themselves identify and administer medication [e.g. Citation29, Citation30, Citation31]. The second scenario is where patients are confronted with different dose strengths of the same drug as part of their treatment. More complex clinical trial protocols as well as approved medication regimens, such as for liraglutide [Citation32] or albiglutide [Citation33], require up-titration to optimize the therapeutic effects while minimizing the potential side effects. Consequently, variants of the same self-injection device platform are often used across dose strengths for the same drug substance. For instance, consider pre-filled autoinjectors containing methotrexate [Citation34,Citation35]. Variants of the same device platform are used for different dose strengths. Differentiation, i.e. modifications made to the devices in order to render the variants distinguishable by users, typically occurs by adjusting the coloring scheme of the label [Citation34,Citation36]. The industry must carefully understand to what extent such limited differentiation strategies may risk erroneous dosing.
Studying the incidence of and approaches to preventing medication errors has become a subject of growing importance in medical and pharmaceutical sciences [Citation37–Citation41]. It has been shown that the occurrence of inappropriate medication use can be linked not only to name confusion [Citation42,Citation43] but also, most notably, to labelling and packaging confusion [Citation44–Citation46]. Specifically, past research offers insights into the mechanisms of how labelling and final packaging influence the ability to accurately recognize medicines [Citation25,Citation47,Citation48]. For instance, the use of coloring schemes to convey drug strength information has been found to positively influence search times and efficacy [Citation30]. Researchers similarly studied the influence of the appearance of the solid oral dosage form on medication errors, confirming that the color of the pill more effectively drives identification than does its shape and size [Citation49]. There is thus good understanding in the literature of which design features of classical oral dosage forms, packaging, and labelling influence the potential for inappropriate drug usage. However, these research efforts have largely overlooked what features determine the distinguishability of advanced drug delivery technologies such as injection devices. The study by Lefkowitz [Citation50] is a notable exception. Here, patients were asked to distinguish between various pen injectors that are based on the same platform and used to administrate both long- and short-acting insulins. The study concluded that differentiation by full pen body coloring, rather than label color only, contributed to reducing patients’ error rates. However, the article did not systematically examine which attributes drive device distinguishability. Furthermore, the article focused solely on injection-experienced diabetics, routinely injecting multiple times a day using multi-dose pen injectors. The results thus show limited applicability to other therapy settings with less frequent injections, to other types of injection devices such as single-use autoinjectors [e.g. Citation51, Citation52, Citation53, Citation54], and to user groups across other disease states.
The present study aims to address these gaps by developing deeper insights into which device attributes drive device distinguishability across user groups. Here, the term user group refers to a set of potential injection device users stratified on the basis of characteristics that may influence their ability to effectively distinguish between self-injection device variants. Such characteristics include professional background, age, dexterity or eye-sight. Furthermore, a device attribute is defined as a single device characteristic or a combination thereof that is empirically identified to drive device distinguishability. The work presented here operationalizes device attributes into three broad categories: shape, size and color scheme. In the study, seventy-four patients, caregivers, and healthcare professionals were asked to rate the perceived similarity between autoinjector platform device variants. As a key insight, the article contributes to the understanding of what device attributes empirically drive device distinguishability. Moreover, the results highlight specific patterns in how various user groups distinguished between self-injection devices. These insights form the basis for more informed approaches to mitigate the risk of inappropriate medication usage.
2. Methods
Multidimensional scaling analysis (MDS) [Citation55] was used as a tool to empirically determine the device attributes that drive the distinguishability between autoinjector device variants. The participants first rated the perceived similarity for each autoinjector device pair presented. MDS then transforms the study participants’ device similarity ratings into multidimensional spatial configurations, thereby determining the underlying dimension of individual similarity perceptions. The greater the perceived similarity between devices, the lower the distance between them in the multidimensional configuration. An analogy to the MDS analysis is drawing a map on the basis of the distances between pairs of villages. The resulting map could be one-dimensional (i.e. all villages arranged on a line), two-dimensional (i.e. a typical map), three-dimensional (i.e. a map illustrated as globe) and so forth. Each dimension of the resulting spatial configuration can then be assigned to a specific device attribute. MDS thus serves as useful tool to identify the drivers of device distinguishability without the need for users to verbalize any criteria used to distinguish between the devices. Instead, participants were asked to directly rate the perceived similarity of platform device variants presented as pairs. Participants were neither asked to generate relevant attributes nor to prioritize between them from a pre-defined list [e.g. Citation47, Citation50, Citation56]. The risk of introducing either participants’ or researchers’ biases is thus lower with MDS compared to other methodologies that may require direct evaluation of device attributes. MDS involves multiple rounds of data analysis, coding, and interpretation as discussed below.
2.1. Materials
Autoinjector platform device variants that differed with respect to four design features were included. The design features for which variation was introduced are presented in and are the color of the label, the color of the needle shield, the overall size, and the device shape. These four design features are typically adapted when customizing an autoinjector platform into a drug-specific device variant.
Figure 1. Illustration of the four design features for which variation was introduced.
Note: For the label color, the label without the device is shown. For the needle shield color, one device example of each color, corresponding to the small square design, is presented.
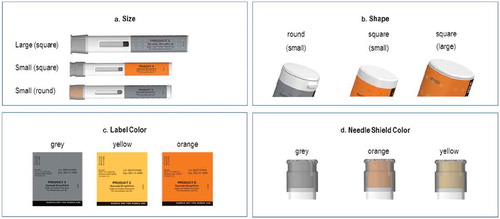
First, the colors used for both the label and the needle shield were grey, orange, and yellow. Orange and yellow were introduced given that signaling colors are commonly used to call user attention, for instance to prevent users from touching the needle with their fingers or to highlight a warning that appears on the drug label. In contrast, grey was included to study the differential impact of a neutral color on the distinguishability of autoinjector platform devices. Second, two device sizes were included. The large variant had a length of 153 mm and a diameter of 21 mm, and the small variant had a length of 145 mm and a diameter of 18 mm. Various sizes of autoinjectors are marketed due to differences in the arrangement and thus space requirements of the platform core technical components or the primary packaging configuration (e.g. 1.0mL Vs. 2.25mL pre-filled syringes). Third, a round profile and a square profile were included as the two shape variants. Round and square shapes correspond to the standard geometrical shapes of most commercial device variants and thus well reflect the autoinjector device market landscape to date. A total of eight different autoinjector variants were included in the study. provides a description of all autoinjector variants evaluated and their respective characteristics.
Table 2. Description of the eight autoinjector platform variants (A.1 - A.8) included in the pair-wise comparison.
2.2. Participants
A total of 74 participants were recruited in Germany. The sample included 40 female (34 male) subjects with (25) and without (49) prior self-injection experience. The sample was selected based on the intended user population for autoinjector devices. The intended user population is reflected by patients with a variety of chronic diseases as well as by people who care for such patients (either professional or non-professional caregivers). The sample was divided into different user groups taking into account characteristics that may affect the users’ ability to effectively distinguish between autoinjector devices. First, three patient age groups (G3, G4 and G5) were defined to study the influence of both decreasing cognitive skills and dexterity on their ability to effectively distinguish between injection devices. Second, visually impaired patients (G6) were included to investigate the importance of non-visual device attributes for device distinguishability. Third, a group of healthcare professionals (HCPs, G1) was included, which was contrasted with a group of non-professional caregivers (G2) to investigate the impact of professional education and use context on device distinguishability.
All study subjects recruited for the three patient age groups (G3, G4 and G6) were diagnosed with at least one of the following chronic conditions where treatment options are offered using autoinjector devices: rheumatoid arthritis, psoriasis, Morbus Crohn’s or inflammatory bowel disease, systemic lupus erythematosus, migraine, chronic pain, cancer, multiple sclerosis, asthma/COPD, diabetes, cardiovascular disease and osteoporosis. provides an overview of the study subjects, including the prevalence of the chronic diseases. The recruitment and compensation of the participants was handled by a specialized and independent agency specialized in recruiting subjects for user testing and medical device research. Participants were selected using a convenience sampling procedure based on the criteria for patient inclusion introduced above. The inclusion criteria were verified using a screening questionnaire at the beginning of the simulated use session. Participants received a small financial compensation.
Table 3. Description of the user population included in the study.
2.3. Research design
The observational non-interventional simulated use study was based on single-site visits. The research design used two sets of independent variables. First, the design features of the autoinjector platform device variants (color, size, and shape) were used in a within-subject variation as described above. Second, a quasi-experimental between-subjects variation was introduced by sampling diverse user groups that differ with respect to characteristics that may affect their ability to effectively distinguish between autoinjector devices (e.g. sight, age or professional experience). The dependent variable was the perceived device similarity ratings obtained on the basis of a complete pairwise comparison procedure of all autoinjector variants.
2.4. Study protocol and procedure
The participants in the simulated use study were seated at a table in a normal office environment on which all autoinjector variants were presented in pairs of two. A moderator provided all instructions during the testing according to a standardized protocol. Each session started with a general explanation of the purpose of the study, a short overview of the procedure and a presentation of an autoinjector device similar to those used in the study. The participants were asked to sign a declaration of consent and to fill out the questionnaires used to verify whether they met the criteria to be included for any of the six user groups. The study subjects were then asked to compare the similarity of eight autoinjector platform device variants in a complete pairwise comparison. Thus, a total of 28 device pairs was presented to each participant. The presentation order of the pairs was randomized. A nine-point Likert-type scale (1 = ‘completely similar’; 9 = ‘completely dissimilar’) was used to capture the subjects’ assessment of the perceived similarity between the device pairs. Four ‘warm-up trials’ with devices similar to those being assessed in the actual study were included to stabilize the subjects´ evaluation criteria and process. For each pairwise comparison, the moderator handed the two autoinjector variants to the subject and asked the participant to closely examine them for their similarity. A tablet was used to capture both the participant’s similarity ratings and the response time.
All participants were informed about the strict confidentiality of the data handling and the possibility to withdraw from participation and from the consent to use their data at any time without any disadvantage. Due to the observational non-clinical nature of the simulated use study no approval by an Ethics Committee was needed. None of the reporting guidelines for the conduct of clinical research (e.g. EQUATOR) were applicable for the study design at hand. Compliance in reporting was ensured as appropriate.
2.5. Data analysis
The statistical software R (version 3.4.3, 2017–11-30) was used for descriptive and inferential statistics. An ANOVA was conducted to study the differences in participants’ similarity ratings between device pairs and user groups. The R package SMACOF was used for the MDS analysis [Citation57]. The INSDSCAL procedure was used with a Euclidean distance model, which is the default method for distance evaluation. The MDS procedure determines the multidimensional configuration and the underlying device attributes on the basis of the participants’ similarity ratings. The Kruskal stress index was then used to evaluate the resulting spatial configurations in terms of goodness-of-fit [Citation58]. The better the fit, the lower the stress index. The study followed Kruskal and Carmone [Citation59] with respect to the acceptability of levels for stress. It should be noted that stress also depends on the number of dimensions used, with a higher number of dimensions leading to lower stress indices for the same level of goodness-of-fit [58.]
Three evaluation criteria were used to decide which of the spatial configurations should be retained for further data analysis and interpretation. First, the elbow criterion – plotting the stress indices per configuration against the number of dimensions – was used to determine the appropriate number of dimensions. The pertinent configurations were selected on the basis of where the stress index values started levelling off, i.e. forming an almost horizontal line in the scree plot [Citation60,Citation61]. The stress values of the spatial configurations were also compared to the stress values of Monte-Carlo type simulation of random data. Second, the data reduction coefficient should be close to or larger than 2. Third, the assignment of a device attribute to the dimension must be ensured.
The stress index was also used to arrive at the final sample size of 74 participants. To continuously monitor the evolution of the spatial configuration and related stress, preliminary MDS analyses were repeatedly conducted while participant recruitment was still ongoing. Recruitment was completed only after the spatial configurations were well-developed and stable, and the corresponding stress indices sustained at acceptable to good levels. The iterative procedure confirmed that further increasing the sample size beyond a total of 74 participants and 12 to 13 representatives per user group was unnecessary.
2.6. Coding procedures
Conscious efforts were made to eliminate any biases in identifying the device attributes that drive device distinguishability. A three-step procedure to assign a device attribute to each dimension of the visual configuration was used for both the overall sample and for each user group. First, two subject matter experts who were unaware of the study purpose established a common coding scheme. Specifically, a round of independent open coding was performed to compile a mutually agreed list of device attributes that accurately described all dimensions emerging from the visual configuration plots. Additional discussion and reconciliation were needed in the case of conflicting coding labels. Second, the two experts independently coded each dimension in each configuration plot based on the agreed coding scheme. The inter-coder reliability was computed to assess the agreement. Whereas Cohen’s Kappa for coding the two-dimensional configurations was 0.606, the value for the three-dimensional configurations was 0.372, thus pointing to moderate to good agreement. The results highlighted a need for final consensus building among all co-authors of the study. Thus, the authors revisited the data considering the individual stress per data point as a variable for agreement on the assignment of device attributes. The three-step process resulted in an unambiguous assignment of device attributes to all dimensions of both the two-dimensional and three-dimensional configurations.
3. Results
The descriptive statistics of the participants’ similarity ratings are presented in . The results illustrate how the distinguishability indeed differed between the self-injection device variants. Mean (median) values of the similarity ratings ranged between 2.26 (2.00) and 7.35 (8.00) for the self-injection device pairs A.6-A.7 and A.1-A.8, respectively.
Table 4. Descriptive statistics of the pairwise similarity ratings (total sample). The similarity assessment was performed based on a nine-point Likert-type scale (1 = ‘completely similar’; 9 = ‘completely dissimilar’). The values for the mean (mea), median (med), and standard deviation (std) are reported.
The analysis of variance (ANOVA) reported statistically significant differences for the overall similarity ratings between the pairs (F(27,1904) = 55.75, p < 0.001). The ANOVA furthermore showed that the differences in the similarity ratings also occurred between the six user groups (F(6,1904) = 4.43, p < 0.001). Given that the ANOVA does not indicate any interaction effects between groups and pairs (p = 0.97), the between-group differences do not depend on the pairs. Although all self-injection device pairs reflected variants of the same platform, users could still distinguish between the 28 pairs of autoinjectors to a moderate to high degree: 14.3% (4) of the mean values for the pairwise perceived similarity ratings were between 1 and 3; 35.7% (10) of all mean values were between 3 and 5; 35.7% (10) of the mean values were between 5 and 7; and 14.7% (4) of the mean values were between 7 and 9.
The descriptive statistics revealed certain patterns around users’ perception of the device similarity. However, it did not explain what device attributes in fact underlie the individual ratings. MDS was therefore used to empirically identify the attributes driving self-injection device distinguishability. presents the two- and three-dimensional visual configurations obtained through MDS for the total sample (N = 74). Similar plots were prepared and analyzed for each user group (data not shown).
Figure 2. Illustration of the two-dimensional (I) and three-dimensional (II) configuration of the self-injection device platform variants (A.1 - A.8) derived from the total sample (N = 74). The three-step coding process resulted in assigning the following device attributes to each dimension: aspect ratio (1-2D), label color (2-2D), device size (1-3D), device shape (2-3D), and device chromaticity (3-3D).
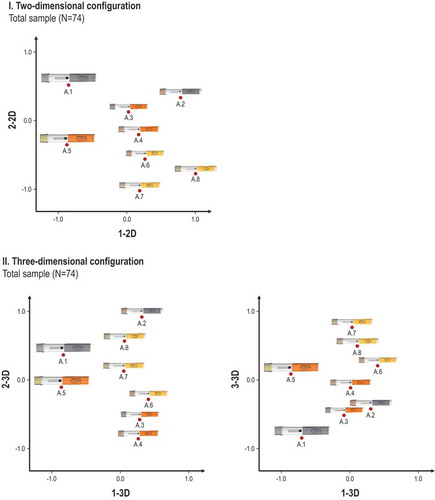
An evaluation of the data and plots according to the three criteria (i.e. the elbow criterion, data reduction coefficient and dimension interpretability) suggested that both the two-dimensional and three-dimensional spatial configurations provided an accurate description of the data. Both solution spaces were thus used as the basis to assign device attributes to the dimensions of the emerging spatial configurations.
The three-step coding process performed for the two- and three-dimensional plots derived from the MDS resulted in assigning a total of five device attributes to the dimensions of all visual configurations. The device attributes were: 1) label color, 2) device size, 3) device shape, 4), device aspect ratio, and 5) chromaticity. defines and illustrates the set of empirically identified device attributes, which the participants thusly used to distinguish between device variants of the same platform.
Table 5. Overview of the device attributes empirically identified as relevant for device distinguishability.
Two of the five device attributes, namely, the aspect ratio and chromaticity, did not directly correspond to a single specific design feature but instead pointed to interaction effects between such features. First, the aspect ratio was interpreted to represent a combination of the self-injection device size and shape and was defined as a continuum with the device presentations [size: small/shape: round] and [size: large/shape: square] as its two extreme ends. Second, the chromaticity was interpreted as the combination of the label and needle shield color. It reflected the overall device hue and brightness and was defined as a continuum with the device presentations [needle shield color: grey/label color: grey] and [needle shield color: yellow/label color: yellow] as its two extreme ends (see ). Three of the five device attributes were related to the geometrical design features of the device variants, and two of the attributes were related to the color, indicating that the geometry took precedence over the color in driving the distinguishability.
presents the assignment of the device attributes to the overall sample as well as to each user group for both the 2-dimensional and 3-dimensional configurations. The device attributes related to the color as well as the geometry were relevant for both the 2-dimensional and 3-dimensional configurations of the total sample. For the individual user groups, certain differences compared to the overall sample emerged. Specifically, health care professionals (HCPs) and visually impaired patients (G1 and G6, respectively) seemed to rely only on the geometrical device attributes to distinguish between devices. In the 3-dimensional configuration, the adolescent patients and elderly patients (G3 and G5, respectively) used the label color instead of the overall chromaticity whereas HCPs (G1), non-professional caregivers (G2) and adult patients (G4) all employed the aspect ratio instead of the shape for distinguishing between the device variants.
Table 6. Device attributes relevant for self-injection device variant distinguishability per user group. The results are presented for the two-dimensional (A) and the three-dimensional (B) configurations. The overall stress values indicate the goodness-of-fit and are reported separately for each user group.
also presents the goodness-of-fit statistics – i.e. Kruskal’s stress index values [Citation58] – of the two- and three-dimensional configurations for each user group. Whereas the stress index values for the two-dimensional configurations ranged between 0.112 for the adult patients (G4) and 0.162 for the HPCs (G1), the stress index values for the three-dimensional configurations ranged between 0.070 for adult patients (G4) and 0.088 for the HCPs (G1). All stress values were in the acceptable to good range, thus pointing to an accurate description of the data [Citation59]. further highlights that the individual stress values per user group were in accordance with the values reported for the overall sample, confirming the robustness of the results.
4. Discussion
This article studied whether and how potential self-injection device users effectively distinguished between autoinjector platform variants. MDS was used to empirically determine the device attributes that drive self-injection device distinguishability. MDS reflects a useful tool to explore the phenomenon at hand without asking participants to articulate the basis for distinguishability ratings [Citation55]. In so doing, the study differed from prior research that typically asked participants to explicitly prioritize from a pre-defined list of design features defined ex ante [e.g. Citation47, Citation50, Citation56]. The results presented here underscore the unique value of the inductive research design. Interestingly, not all design features for which variation was introduced were relevant device attributes that drive device distinguishability. Whereas the size, shape and label color were identified as device attributes that directly determine the distinguishability, the needle shield color did not emerge as an independent device attribute driving the distinguishability. Overall, the geometrical features appeared to take precedence over the color in driving the distinguishability. These findings contrast with what has been previously observed for the packaging, labelling and oral dosage forms [Citation30,Citation49].
The study also provided some unexpected results. Two of the device attributes empirically identified to drive platform distinguishability, namely, the aspect ratio and chromaticity, each reflected a combination of single user interface elements. To the authors’ knowledge, the study, for the first time, identified interaction effects between distinct design features as a basis to distinguish between devices. These findings have methodological implications for the effective conduct of empirical studies. Although it remains valuable to analytically distinguish between user interface elements as separate units of analysis, the results suggest that future work on device distinguishability should also consider multiple levels of analysis, taking into account interaction effects between design features.
The results also advanced our understanding of the user group-specific patterns in what device attributes were relevant for device distinguishability. Whereas some user groups conformed to the overall sample, other user groups relied on specific device attributes to distinguish between platform variants. The results suggest that these differences could be linked to either the user group characteristics (e.g. professional education, dexterity or visual impairments) or the context in which that user group typically administers medication. Prior research had similarly shown that various situational factors, such as the time available to familiarize oneself with the design features or interruptions during medication verification, influenced the incidence of medication errors [Citation62,Citation63]. For instance, the patterns of elderly patients (G5) notably deviated from the patterns of the overall sample. Specifically, the aspect ratio was neither included in the two- nor the three-dimensional solution space for this group. It is worth noting that the aspect ratio, as identified in this study, may correlate well with the ranked ease of holding between device versions. Thus, the aspect ratio can be hypothesized to constitute a haptic rather than a visual device attribute. The elderly patients’ (G5) emphasis on visual rather than haptic features could, in turn, be explained by the general tendency towards decreasing dexterity with increasing age [Citation64]. The user group may compensate for its characteristics by prioritizing visual device attributes as the basis for decision-making. The tendency to countervail group-specific characteristics can also be observed for visually impaired patients (G6). Device attributes related to the color, i.e. label color and chromaticity, did not drive the distinguishability. Instead, the group relied mainly on the geometric device attributes to assess the device distinguishability.
The results further underscored that HCPs (G1) adopt unique tactics to effectively distinguish between self-injection devices. Specifically, the study provided evidence that HCPs (G1) – unlike non-professional caregivers (G2) – primarily used geometrical features, such as the size, shape and aspect ratio, to distinguish between platform devices variants. Interestingly, none of the color-oriented device attributes (i.e. the label color or chromaticity) proved relevant for this group (G1). These findings again correlated well with prior research concluding that healthcare professionals raised concerns about the usefulness of their knowledge on label design and coloring as a basis for effective medication identification [Citation62].
Several limitations apply to the study presented here. First, a non-exhaustive list of device features was introduced as the independent variables of the study. The analysis specifically focused on user interface elements typically adjusted during the customization process of platform devices, such as the size, shape, and coloring schemes. More unique customization strategies were not included in the study. For instance, the label design other than coloring was not varied. Second, the total sample size of 74 participants and 12 to 13 representatives per patient group may not be representative of the entire population. However, the sample size yielded stable and well-developed MDS models with acceptable to good stress values. Third, although a multi-stage coding procedure was implemented to minimize individual biases in interpreting the results – such as including independent experts who were unaware of the study purposes for the most critical stages in the data interpretation – such biases could not be completely eliminated.
5. Conclusion
Despite the increasing interest in subcutaneous drug delivery and the resulting broad adoption of potentially look-alike injection platform device variants, few attempts have been made to study whether and how users were able to effectively distinguish between injection device variants. Although prior literature has repeatedly emphasized the importance of labelling and packaging design to prevent medication errors there is a lack of in-depth analysis of not only what device attributes empirically drive distinguishability but also whether differences between groups occur. The study at hand empirically identifies a set of device attributes that drive injection device distinguishability using multidimensional scaling analysis. Three of the device attributes corresponded to a single design feature: the label color, device size and device shape. Two of the device attributes (the device aspect ratio and chromaticity) each combined two distinct yet interrelated design features. Interestingly, one design feature that was varied, the needle shield color, did not emerge at all as a relevant device attribute. Overall, the geometrical features appeared to take precedence over the color in driving the distinguishability. Moreover, the study unveils user group-specific patterns in what attributes prove relevant to distinguish between devices. These patterns can be directly linked to user-group specific characteristics (e.g. age, sight, dexterity) and situational factors of typical medication administration (e.g. HCPs).
These findings hold important implications for practice. First, the study draws the attention of medical device design toward those device attributes that are found to drive device distinguishability. The study thus starts developing a toolbox that guides the industry toward those device attributes that in fact shape device distinguishability. In so doing, the article provides novel approaches that may well contribute to the effective mitigation of medication errors. Second, the article unveils group-specific patterns in how users effective distinguish between devices. In so doing, the results link device distinguishability with user characteristics. The reduction of medication errors through improved device distinguishability thus must be carefully adjusted to the intended user population (e.g. age, sight or dexterity) and depends on the context of device usage (e.g. in the case of healthcare professionals). The study provides initial guidance to the industry as to which device attributes were relevant for what user groups to effectively distinguish between autoinjector platform device variants. For instance, elderly patients appeared to rely on haptic rather than visual device attributes when distinguishing between devices. Similarly, healthcare professionals seem to predominantly rely on geometrical device attributes. Overall, the research informs approaches to improve the device distinguishability and thereby reduce the occurrence of preventable events of inappropriate medication use.
Author contributions
All authors contributed to the conception and design of the study. H-P Horn performed the study. H Kolrep and H-P Horn did the data preparation and analysis. All authors took part in interpreting the data for the study. All authors commented on and helped to revise drafts of this paper. All authors have approved the final version.
Declaration of interest
A Schneider, C Jordi, P Richard and J Lange work for Ypsomed AG. H Kolrep and H-P Horn work for Human-Factors-Consult (HFC). The authors have no other relevant affiliations or financial involvement with any organization or entity with a financial interest in or financial conflict with the subject matter or materials discussed in the manuscript apart from those disclosed.
Reviewer disclosures
Peer reviewers on this manuscript have no relevant financial or other relationships to disclose.
Additional information
Funding
References
- Lange J, Richard P, Bradley N. Usability of a new disposable autoinjector platform device: results of a formative study conducted with a broad user population. Med Devices (Auckl). 2015;8:255–264. PubMed PMID: 26082667; PubMed Central PMCID: PMC4461121.
- Thompson I, Lange J. Pen and autoinjector drug delivery devices. In: Kolhe P, Shah M, Rathore N, editors. Sterile product development. New York, NY: Springer; 2016. p. 331–356.
- Baldwin CY, Woodard CJ. The architecture of platforms: a unified view. In: Gawer A, editor. Platforms, markets, and innovation. Cheltenham, UK: Edward Elgar; 2009. p.19–44.
- Bresnahan TF, Greenstein S. Technological competition and the structure of the computer industry. J Ind Econ. 1999;47(1):1–40.
- Meyer MH, Lehnerd AP. The power of product platforms: building value and cost leadership. New York: Free Press; 1997.
- Robertson D, Ulrich K. Planning for product platforms. Sloan Manage Rev. 1998;39(4):19.
- Dehoratius RJ, Brent LH, Curtis JR, et al. Satisfaction with subcutaneous golimumab and its auto-injector among rheumatoid arthritis patients with inadequate response to adalimumab or etanercept [journal article]. Patient - Patient-Centered Outcomes Res. 2018 June 01;11(3):361–369.
- Kivitz A, Segurado OG. HUMIRA pen: a novel autoinjection device for subcutaneous injection of the fully human monoclonal antibody adalimumab. Expert Rev Med Devices. 2007 Mar;4(2):109–116. PubMed PMID: 17359217.
- Hey-Hadavi J, Pleil A, Deeb LC, et al. Ease of use and preference for a new disposable self-injection pen compared with a reusable pen for administering recombinant human growth hormone: A multicenter, 2-month, single-arm, open-label clinical trial in patient-caregiver dyads. Clin Ther. 2010;32(12):2036–2047.
- Torjman MC, Machnicki R, Lessin J, et al. Evaluation of an investigational wearable injector in healthy human volunteers. Expert Opin Drug Deliv. 2017 January 02;14(1):7–13.
- Joshi RS, Egbuna OI, Cairns AS, et al. Performance of the pegfilgrastim on-body injector as studied with placebo buffer in healthy volunteers. Curr Med Res Opin. 2016;1–6. DOI:10.1080/03007995.2016.1257980
- Noymer P, Shaked A, Newell K. Human factors and usability engineering in the development of SMT-101 for the treatment of pulmonary arterial hypertension. Expert Opin Drug Deliv. 2018 April 03;15(4):325–333.
- Bode A. Development of the SoloSTAR insulin pen device: design verification and validation. Expert Opin Drug Deliv. 2009 Jan 6;6(1):103–112. PubMed PMID: 19236211.
- Haak T, Edelman S, Walter C, et al. Comparison of usability and patient preference for the new disposable insulin device Solostar versus Flexpen, lilly disposable pen, and a prototype pen: an open-label study. Clin Ther. 2007 Apr;29(4):650–660. PubMed PMID: 17617288.
- Davis EM, Sexson EL, Spangler ML, et al. An evaluation of prefilled insulin pens: a focus on the next generation Flexpen((R)). Med Devices (Auckl). 2010;3:41–50. PubMed PMID: 22915920; PubMed Central PMCID: PMC3417864.
- Bailey T, Campos C. FlexTouch(R) for the delivery of insulin: technical attributes and perception among patients and healthcare professionals. Expert Rev Med Devices. 2012 May;9(3):209–217. PubMed PMID: 22471783.
- Pfutzner A, Forst T, Niemeyer M, et al. Assessment for ease of use and preference of a new prefilled insulin pen (FlexTouch Degludec U100/U200) versus the SoloSTAR insulin pen by patients with diabetes and healthcare professionals. Expert Opin Drug Deliv. 2014 Sep;11(9):1381–1389. PubMed PMID: 24921223.
- Schneider AE, Lange J. Pen devices for self-injection: contrasting measured injection force with users’ perceived ease of injection. Expert Opin Drug Deliv. 2018 February 01;15(2):115–125.
- Callis Duffin K, Bukhalo M, Bobonich MA, et al. Usability of a novel disposable autoinjector device for ixekizumab: results from a qualitative study and an open-label clinical trial, including patient-reported experience. Med Devices (Auckl). 2016;9:361–369. PubMed PMID: 27785115; PubMed Central PMCID: PMC5067052.
- Dashiell-Aje E, Harding G, Pascoe K, et al. Patient evaluation of satisfaction and outcomes with an autoinjector for self-administration of subcutaneous belimumab in patients with systemic lupus erythematosus. Patient. 2018 Feb;11(1):119–129. 10.1007/s40271-017-0276-2. PubMed PMID: 28956281.
- Egeth M, Soosaar J, Nash P, et al. Patient and healthcare professionals preference for brenzys vs. Enbrel autoinjector for rheumatoid arthritis: a randomized crossover simulated-use study. Adv Ther. 2017 Apr 17 [cited Feb 03];34(5):1157–1172.
- Pachon JA, Kivitz AJ, Heuer KU, et al. Assessing usability, label comprehension, pen robustness and pharmacokinetics of a self-administered prefilled autoinjector pen of methotrexate in patients with rheumatoid arthritis. SAGE Open Med. 2014;2:2050312114564241, 10.1177/2050312114564241. PubMed PMID: 26770759; PubMed Central PMCID: PMC4712753.
- Lange J, Richard P, Bradley N. Usability of devices for self-injection: results of a formative study on a new disposable pen injector. Med Devices. 2014 Jun 12;7:195–203.
- U.S. Food and drug administration, medication errors related to CDER-regulated drug products [Internet]. [cited 2019 Jun 18]. Available from: https://www.fda.gov/drugs/drug-safety-and-availability/medication-errors-related-cder-regulated-drug-products
- Estock JL, Murray AW, Mizah MT, et al. Label design affects medication safety in an operating room crisis: a controlled simulation study. J Patient Saf. 2015 Mar 07. PubMed PMID: 25760652. DOI:10.1097/PTS.0000000000000176.
- Fyhr A, Akselsson R. Characteristics of medication errors with parenteral cytotoxic drugs. Eur J Cancer Care (Engl). 2012 Sep;21(5):606–613. . PubMed PMID: 22335508; PubMed Central PMCID: PMC3509217.
- Garcia BH, Elenjord R, Bjornstad C, et al. Safety and efficiency of a new generic package labelling: a before and after study in a simulated setting. BMJ Qual Saf. 2017;26:817–823.
- Garnerin P, Perneger T, Chopard P, et al. Drug selection errors in relation to medication labels: a simulation study. Anaesthesia. 2007 Nov;62(11):1090–1094. PubMed PMID: 17924887.
- Hellebek A, Schytte-Hansen S, Fischer H, et al. Patient safety in drug label design: analysis of reported adverse events before and after introducing a new label design. Eur J Hosp Pharm Sci Pract. 2013;20(4):212–217.
- Hellier E, Tucker M, Kenny N, et al. Merits of using color and shape differentiation to improve the speed and accuracy of drug strength identification on over-the-counter medicines by laypeople. J Patient Saf. 2010;6(3):158–164. PubMed PMID: 01209203-201009000-00006.
- Lenahan JL, McCarthy DM, Davis TC, et al. A drug by any other name: patients’ ability to identify medication regimens and its association with adherence and health outcomes. J Health Commun. 2013 Dec 04;18(sup1):31–39.
- Nauck M, Frid A, Hermansen K, et al. Efficacy and safety comparison of liraglutide, glimepiride, and placebo, all in combination with metformin in type 2 diabetes mellitus (LEAD-2 Met). Diabetes Care. 2008;32:84–90.
- Emery P, Breedveld FC, Hall S, et al. Comparison of methotrexate monotherapy with a combination of methotrexate and etanercept in active, early, moderate to severe rheumatoid arthritis (COMET): a randomised, double-blind, parallel treatment trial. Lancet. 2008;372(9636):375–382.
- Demary W, Schwenke H, Rockwitz K, et al. Subcutaneously administered methotrexate for rheumatoid arthritis, by prefilled syringes versus prefilled pens: patient preference and comparison of the self-injection experience. Patient Prefer Adherence. 2014;8:1061–1071. PubMed PMID: 25125973; PubMed Central PMCID: PMC4130714.
- Freundlich B, Kivitz A, Jaffe JS. Nearly pain-free self-administration of subcutaneous methotrexate with an autoinjector: results of a phase 2 clinical trial in patients with rheumatoid arthritis who have functional limitations. J Clin Rheumatol. 2014 Aug;20(5):256–260. . PubMed PMID: 25036562; PubMed Central PMCID: PMC4165470.
- Hudry C, Lebrun A, Moura B, et al. Evaluation of usability and acceptance of a new autoinjector intended for methotrexate subcutaneous self-administration in the management of rheumatoid arthritis. Rheumatol Ther. 2017 Jun;4(1):183–194. 10.1007/s40744-017-0057-3. PubMed PMID: 28243967; PubMed Central PMCID: PMC5443726.
- Kaushal R, Bates DW, Landrigan C, et al. Medication errors and adverse drug events in pediatric inpatients. Jama. 2001;285(16):2114–2120.
- Phillips DP, Christenfeld N, Glynn LM. Increase in US medication-error deaths between 1983 and 1993. Lancet. 1998;351(9103):643–644.
- Donaldson MS, Corrigan JM, Kohn LT. To err is human: building a safer health system. Vol. 6. Washington DC (USA): National Academies Press; 2000.
- Carayon P, Wetterneck TB, Cartmill R, et al. Characterising the complexity of medication safety using a human factors approach: an observational study in two intensive care units. BMJ Qual Saf. 2014;23(1):56–65.
- Zhong W, Feinstein JA, Patel NS, et al. Tall man lettering and potential prescription errors: a time series analysis of 42 children’s hospitals in the USA over 9 years. BMJ Qual Saf. 2015. DOI:10.1136/bmjqs-2015-004562.
- Seoane-Vazquez E, Rodriguez-Monguio R, Alqahtani S, et al. Exploring the potential for using drug indications to prevent look-alike and sound-alike drug errors. Expert Opin Drug Saf. 2017 Oct 03;16(10):1103–1109.
- Schroeder SR, Salomon MM, Galanter WL, et al. Cognitive tests predict real-world errors: the relationship between drug name confusion rates in laboratory-based memory and perception tests and corresponding error rates in large pharmacy chains. BMJ Qual Saf. 2016;bmjqs-2015–005099. DOI:10.1136/bmjqs-2015-005099
- Berman A. Reducing medication errors through naming, labeling, and packaging. J Med Syst. 2004 Feb;28(1):9–29. PubMed PMID: 15171066; eng.
- Ward J, Buckle P, John CP. Designing packaging to support the safe use of medicines at home. Appl Ergon. 2010 Sep;41(5):682–694. PubMed PMID: 20153457; eng.
- Porat N, Bitan Y, Shefi D, et al. Use of colour-coded labels for intravenous high-risk medications and lines to improve patient safety. Qual Saf Health Care. 2009;18(6):505–509.
- Filik R, Purdy K, Gale A, et al. Labeling of medicines and patient safety: evaluating methods of reducing drug name confusion. Hum Factors. 2006 Spring;48(1):39–47. PubMed PMID: 16696255.
- Gerhart JM, Spriggs H, Hampton TW, et al. Applying human factors to develop an improved package design for (Rx) medication drug labels in a pharmacy setting. J Safety Res. 2015 Dec;55:177–184. PubMed PMID: 26683561.
- Stegemann S, Riedl R, Sourij H. Identification of different shapes, colors and sizes of standard oral dosage forms in diabetes type 2 patients-A pilot study. Int J Pharm. 2017 Jan 30;517(1–2):112–118. PubMed PMID: 27919699.
- Lefkowitz M. Do different body colors and labels of insulin pens enhance a patient’s ability to correctly identify pens for injecting long-acting versus short-acting insulins? J Diabetes Sci Technol. 2011 Jan 01;5(1):136–149. PubMed PMID: 21303636; PubMed Central PMCID: PMCPmc3045242. eng.
- Lim WH, Chan D, Boudville N, et al. Patients’ perceptions of subcutaneous delivery of darbepoetin alfa by autoinjector prefilled pen versus prefilled syringe: a randomized, crossover study. Clin Ther. 2012 Sep;34(9):1948–1953. PubMed PMID: 22902097.
- Phillips JT, Fox E, Grainger W, et al. An open-label, multicenter study to evaluate the safe and effective use of the single-use autoinjector with an Avonex(R) prefilled syringe in multiple sclerosis subjects. BMC Neurol. 2011 Oct 14;11:126. PubMed PMID: 21999176; PubMed Central PMCID: PMC3213083.
- Schiff M, Koo J, Jin E, et al. Usability and acceptability of the abatacept pre-filled autoinjector for the subcutaneous treatment of rheumatoid arthritis. Adv Ther. 2016 Feb;33(2):199–213. 10.1007/s12325-016-0286-9. PubMed PMID: 26833303; PubMed Central PMCID: PMC4769728.
- Schulze-Koops H, Giacomelli R, Samborski W, et al. Factors influencing the patient evaluation of injection experience with the SmartJect autoinjector in rheumatoid arthritis. Clin Exp Rheumatol. 2015 Mar -Apr;33(2):201–208. PubMed PMID: 25664404.
- Kruskal JB, Wish M Multidimensional scaling. Number 07–011 in Sage University Paper series on quantitative applications in the social sciences. Sage Publications, Beverly Hills; 1978.
- Endestad T, Wortinger LA, Madsen S, et al. Package design affects accuracy recognition for medications. Hum Factors. 2016 Dec;58(8):1206–1216. PubMed PMID: 27591209; PubMed Central PMCID: PMC5570154.
- de Leeuw J, Mair P. Multidimensional scaling using majorization: SMACOF in R. Journal of Statistical Software. 2009. Aug 04; 31(3):30. doi: 10.18637/jss.v031.i03.
- Kruskal JB. Multidimensional scaling by optimizing goodness of fit to a nonmetric hypothesis. Psychometrika. 1964;29(1):1–27.
- Kruskal JB, Carmone FJ. How to use MDSCAL, a program to do multidimensional scaling and multidimensional unfolding. Murray Hill, NY: Bell Laboratories; 1973. ( Bell Telephone Laboratories).
- Cattell RB. The scree test for the number of factors. Multivariate Behav Res. 1966;1(2):245–276.
- Borg I, Groenen PJF, MairP. Applied multidimensional scaling. New York: Springer; 2013. p. 81–86.
- Dieckmann P, Clemmensen MH, Sørensen TK, et al. Identifying facilitators and barriers for patient safety in a medicine label design system using patient simulation and interviews. J Patient Saf. 2016;12(4):210–222.
- Prakash V, Koczmara C, Savage P, et al. Mitigating errors caused by interruptions during medication verification and administration: interventions in a simulated ambulatory chemotherapy setting. BMJ Qual Saf. 2014;23(11):884–892.
- Martin JA, Ramsay J, Hughes C, et al. Age and grip strength predict hand dexterity in adults. PloS One. 2015;10(2):e0117598.