Abstract
The availability of experimental nanotoxicity data is in general limited which warrants both the use of in silico methods for data gap filling and exploring novel methods for effective modeling. Read-Across Structure-Activity Relationship (RASAR) is an emerging cheminformatic approach that combines the usefulness of a QSAR model and similarity-based Read-Across predictions. In this work, we have generated simple, interpretable, and transferable quantitative-RASAR (q-RASAR) models which can efficiently predict the cytotoxicity of TiO2-based multi-component nanoparticles. A data set of 29 TiO2-based nanoparticles with specific amounts of noble metal precursors was rationally divided into training and test sets, and the Read-Across-based predictions for the test set were generated. The optimized hyperparameters and the similarity approach, which yield the best predictions, were used to calculate the similarity and error-based RASAR descriptors. A data fusion of the RASAR descriptors with the chemical descriptors was done followed by the best subset feature selection. The final set of selected descriptors was used to develop the q-RASAR models, which were validated using the stringent OECD criteria. Finally, a random forest model was also developed with the selected descriptors, which could efficiently predict the cytotoxicity of TiO2-based multi-component nanoparticles superseding previously reported models in the prediction quality thus showing the merits of the q-RASAR approach. To further evaluate the usefulness of the approach, we have applied the q-RASAR approach also to a second cytotoxicity data set of 34 heterogeneous TiO2-based nanoparticles which further confirmed the enhancement of external prediction quality of QSAR models after incorporation of RASAR descriptors.
Graphical Abstract. Schematic representation of the concept of RASAR
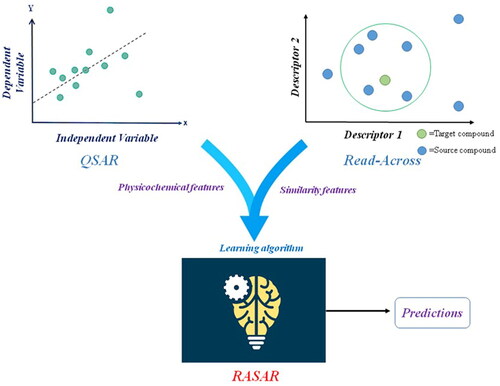
Acknowledgments
The authors thank Dr. Agnieszka Gajewicz-Skretna of the University of Gdansk, Poland for providing descriptor values using the VASP package and helpful discussions. AB thanks the Life Science Research Board, DRDO, New Delhi for a senior research fellowship. SK wants to thank the administration of Dorothy and George Hennings College of Science, Mathematics and Technology (HCSMT) of Kean University for providing research opportunities and resources. SP thanks the All India Council for Technical Education (AICTE), New Delhi for a scholarship.
Author contributions
AB (Computation, model development, initial draft); SK (Computation, editing); SP (Coding); KR (Conceptualization, supervision, funding, editing).
Disclosure statement
No potential conflict of interest was reported by the author(s).
Data availability statement
The original data in part was submitted to a preprint server https://www.preprints.org/manuscript/202210.0402/v1. Any additional data related to this manuscript will be shared on request.