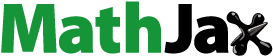
ABSTRACT
Historical patterns of land-use development across many countries in the Global North have generally been characterised by land-use segregation, low-density settlements, and limited transport accessibility. This can lead to inefficient regional developmental patterns and can increase the environmental degradation attributable to regional expansion. Numerous metrics and datasets have been employed to infer the sustainability and efficiency of these developments. Here, we quantify and visualise the relative entropy of land-use configurations across the Republic of Ireland using a novel dataset. Specifically, we combine the discipline-standard CORINE Land Cover 2018 dataset and OpenStreetMap data, which provides more thorough land-use classifications in urban areas. Spatial differences in relative entropy are visualised using a spatial typology distinguishing between areas exhibiting low and high levels of relative entropy. Our Main Map visualises the coverage of both CORINE and OpenStreetMap datasets, compares relative entropy estimates for both datasets, and illustrates the disparities in cross-dataset estimates.
1. Introduction
Land-use distributions determine the locations and intensities of human activities, rendering them fundamental to human geography and regional development (Gao et al., Citation2020). Because land is a finite resource, perpetual expansion is impossible, highlighting the importance of using these resources efficiently (Nechyba & Walsh, Citation2004). Here, we assess the sustainability and efficiency of land-use patterns across the Republic of Ireland by quantifying relative entropy levels across Ireland's Electoral Divisions (ED). Calculating the sustainability and efficiency of land-use patterns is usually approached from the perspective of efficient resource allocation, whereby traits such as segregation and low-population densities are typically classed as inefficient, and subsequently negatively contribute to sustainability efforts (Ewing, Citation1997; OECD, Citation2018). Accordingly, the datasets needed to measure land-use mixing must contain spatial maps of land pockets defined by their purpose of use (Song et al., Citation2013).
Relative entropy is a metric which can infer sustainability and efficiency in land and infrastructure use. Relative entropy measures the mix of land-use types within specified areas by measuring the relative proportion of space allocated to different land types (Eldeeb et al., Citation2021; Song et al., Citation2013). This enables inferences to be made about the relative segregation of land and subsequently facilitates investigations into the sustainability and efficiency of developmental patterns (Mavoa et al., Citation2018; O’Driscoll et al., Citation2023). Measures such as relative entropy are important because they offer objective measures of the relative sustainability and efficiency of land-use policies (Song et al., Citation2013). Ireland offers an important case study for this area of research as unsustainable developmental patterns are prominent, with CitationAhrens and Lyons (Citation2019) observing that ‘urban land expansion in Ireland is among the highest in Europe’ (Ahrens & Lyons, Citation2019, p. 1).
Primarily catalysed by the commercialisation of private cars (Paterson, Citation2000; Pooley & Turnbull, Citation2005), regional development in Ireland is characterised by urban sprawl and suburbanisation (Faccini et al., Citation2021; Qiao et al., Citation2019; Strollo et al., Citation2020). Generally characterised by low settlement densities and segregated land-use, suburbs exacerbate environmental degradation through excessive habitat destruction (Ewing, Citation1997; Nechyba & Walsh, Citation2004; OECD, Citation2018). Land-use policies which focus on increasing density and multifunctionality have been touted as mechanisms to abate the environmental degradation attributable to sprawl (Badland & Schofield, Citation2005; Dieleman & Wegener, Citation2004; Newman & Kenworthy, Citation1996). By focusing developments on compactness and mixed-use, policymakers can reduce the environmental burden attributable to sprawl by increasing infrastructure efficiencies (Carpio-Pinedo et al., Citation2021; Huang et al., Citation2020; Millward et al., Citation2013; Newman & Kenworthy, Citation1996). Accordingly, land-use developments characterised by single-use settlements and segregation have been touted as unsustainable (Nechyba & Walsh, Citation2004). Relative entropy explicitly measures the mixing of unique land-use categories across geographic spaces, therefore providing a direct mechanism to infer the sustainability and efficiency of land and infrastructure use.
Studies measuring the sustainability and efficiency of land-use patterns typically utilise the CORINE Land Cover dataset due to its universal European coverage, and many use relative entropy to measure the sustainability and efficiency of land-use configurations (Eldeeb et al., Citation2021; Ton et al., Citation2019). We contribute to this literature by analysing the relative sustainability and efficiency of national land-use patterns through the lens of small geographic spaces (Ahrens & Lyons, Citation2019; Calka, Citation2021; Druga & Minár, Citation2018; Gao et al., Citation2020; Strollo et al., Citation2020). We explicitly build upon the work of CitationO’Driscoll et al. (Citation2023) by combining the CORINE Land Cover dataset with more refined land-use data from OpenStreetMap. However, we go further by using a nationally comprehensive study scope. This allows us to compare the robustness of relative entropy estimates across datasets, thereby enhancing the quality of metrics which infer the sustainability and efficiency of land-use configurations. This also allows us to answer calls for greater research into the sustainability of regional developmental forms across diverse geographies (Carpio-Pinedo et al., Citation2021; Gan et al., Citation2021; Ton et al., Citation2019).
When measuring the sustainability and efficiency of development forms comparisons between, and the combination of, CORINE and OpenStreetMap are important. This is because while CORINE is recognised for its comprehensiveness,Footnote1 it lacks thoroughness in urban environments, thoroughness which OpenStreetMap provides. OpenStreetMap, while less comprehensive in coverage (particularly in rural areas), provides greater detail in the areas it does cover (urban areas). Combining these datasets enables the creation of a more comprehensive and thorough land-use dataset. Specifically, we exploit OpenStreetMap's thoroughness and rectify it with nationally comprehensive CORINE data. We build on existing literature by visualising the multifunctionality of land across small geographical spaces using data at a unique disaggregated spatial scale of analysis (Carpio-Pinedo et al., Citation2021; Druga & Minár, Citation2018; O’Driscoll et al., Citation2023). Specifically, we use the administrative unit level of Electoral Divisions (EDs) from the 2016 Irish Census as our spatial scale, which are Ireland's Local Administrative Units 2 (LAU2) that account for 3409 localities.
The remaining sections of this paper detail our data and methodology utilised in the study and illustrate our results and their implications. We conclude the analysis by highlighting the policy implications of this work, while also highlighting the study's limitations and potential avenues for future research.
2. Data and methods
2.1. Data
The foundation of our Main Map comes from shapefiles provided by the Central Statistics Office (CSO) and Ordnance Survey Ireland (OSI) (CitationOrdnance Survey Ireland [OSI], 2016). The CSO shapefile features ungeneralised ED boundaries for the 2016 Irish Census, which are the smallest legally defined administrative areas in the State, and capture 3409 localities (CitationCentral Statistics Office [CSO], 2016). This shapefile is then clipped using the Ordnance Survey Ireland Sea boundary shapefiles to mitigate the base Census shapefile's omission of important facets of Ireland's land mass, such as the Shannon Estuary (CitationOrdnance Survey Ireland [OSI], 2016). Northern Ireland is not analysed in this study and is therefore only included in outline format to increase cartographic accuracy.
Our adoption of an Electoral Division spatial scale is appropriate because regional heterogeneities in land-use patterns may be hidden at larger scales, like the NUTS3 regions, of which there are only eight in Ireland (Boarnet & Sarmiento, Citation1998; O’Driscoll et al., Citation2022). Similarly, meaningful results may not be extractable from smaller spatial units. For example, the smallest spatial scale available in an Irish context are referred to as Small Areas, (of which there are 18,641) and these provide greater spatial disaggregation at sub-neighbourhood levels, but may compromise policymaking recommendations due to potentially conflicting insights emerging within administrative localities (Boarnet & Sarmiento, Citation1998; O’Driscoll et al., Citation2022).
Our land-use dataset allows us to quantify land-use mixing in Ireland. Specifically, we combine the CORINE Land Cover dataset with OpenStreetMap data which allows us to exploit OpenStreetMap's thoroughness in classifying urban land-uses and supplement it with CORINE's comprehensive land-use classification, a combination which creates a nationally comprehensive, fine-grain land-use classification catalogue. We use OpenStreetMap as our principal dataset and use CORINE to fill any remaining blanks in the data, creating a best-of-both dataset. Alternative datasets to CORINE exist in other countries, and are usually centrally administered (Carpio-Pinedo et al., Citation2021). But, in Ireland the CORINE Land Cover is the best available land-use dataset due to its comprehensiveness, hence its use here. However, in cases where additional datasets are available which can enhance our understanding of land-use configurations, they should be employed (Fleischmann & Arribas-Bel, Citation2021).
2.2. Dataset construction
CORINE Land Cover data is imported into Quantum Geographic Information Systems (QGIS) where it is clipped to our adjusted ED boundary file to only include observations within the Republic of Ireland. This database identified 18,872 unique land-use polygons. Each polygon is then dissolved according to aggregated land-use categories, and then intersected with our ED boundary file, creating a database with 10,544 polygons. We group land-use types into seven broad umbrella groups in line with existing literature (Calka, Citation2021; Gao et al., Citation2020). below illustrates these land-use categories for our CORINE land-cover dataset.
Table 1. CORINE land cover categories as per (Calka, Citation2021; Gao et al., Citation2020).
To acquire a comprehensive catalogue of OpenStreetMap land-use polygons, we collect every polygon across the Republic of Ireland identified by OpenStreetMap's QuickOSM plugin within QGIS as characterising land-use. This query identified 395,835 unique land-use polygons. Again, polygons are then aggregated into umbrella categories of land-use. To account for potential human/identification errors present in this data, we allow scope for overlap in these categories, particularly in those dedicated to public space. Due to the novelty of using OpenStreetMap data within this research context, no precedent exists to guide our aggregation process, with the exception of CitationO’Driscoll et al. (Citation2023). Consequently, we roughly follow previous aggregations of the CORINE dataset (Calka, Citation2021) by basing our aggregation on the official CORINE class 2 and class 3 nomenclature.Footnote2 below illustrates these aggregated land-use categories for our OpenStreetMap dataset.
Table 2. OpenStreetMap land cover categories.
Upon completing this process, we collect all non-overlapping features from both shapefiles. These differences are then merged with our OpenStreetMap data. This creates a comprehensive land-use dataset, combining all information from both CORINE and OpenStreetMap, for the Republic of Ireland.Footnote3 The merge results in a dataset containing 411,889 land-use pockets, where aggregated land-use categories are adjusted to construct a consistent metric which appropriately incorporates elements from each shapefile. Thereafter, this shapefile is dissolved according to newly aggregated land-use categories, creating a shapefile containing 36,337 unique land-use polygons. Finally, this dataset is intersected with our ED boundary file, enabling us to calculate the proportion of each aggregated land-use category present within every ED. below details our aggregated land-use categories when we combine our CORINE and OpenStreetMap dataset.
Table 3. Combined OSM and CORINE land cover categories.
2.3. Measuring entropy
Conceptually, there are two approaches to analysing the spatial distribution of land. Firstly, integral measures, which primarily measure area-wide proportional shares of land type categories, and secondly divisional measures, which mainly quantify the relative similarity of neighbouring land pockets (Song et al., Citation2013). Because the results produced by individual measures within each category are highly correlated, the choice of indicator is usually dependent on the geographic scope of the analysis.Footnote4 Integral measures are usually preferred when analysing small geographical areas whereas divisional measures are preferred in larger geographies (Song et al., Citation2013). Consequently, both categories are inherently susceptible to the Modifiable Area Unit Problem due to their dependency on geographical boundary specification (Druga & Minár, Citation2018; Song et al., Citation2013). Because our analysis adopts local administrative units (EDs) as our spatial boundaries, and because our focus principally lay in analysing area-wide land-use shares as opposed to inter-regional similarities in land-use distributions, using an integral measure is appropriate (Bhatta et al., Citation2010; Eldeeb et al., Citation2021; Jeong et al., Citation2022; Mavoa et al., Citation2018).
Land-use Mixing (i.e. relative entropy) is a cornerstone concept across regional development literature to analyse the sustainability and efficiency of developmental forms (Cao, Citation2016; Eldeeb et al., Citation2021; Luan & Fuller, Citation2022; Mavoa et al., Citation2018). Relative entropy measures the relative mixing of land types across pre-defined spatial boundaries, whereby higher levels of relative entropy (measured on a 0–1 scale) indicate greater mixing, something linked to greater levels of sustainability and efficiency in developmental forms (Eldeeb et al., Citation2021; Song et al., Citation2013; Zhang & Zhao, Citation2017). Relative entropy for a given spatial unit can be calculated as
(1)
(1) whereby Ej reflects the relative entropy score of area j. Pkj reflects the summed proportions of land types k within area j.
2.4. Cartographic visualisation
Placed atop a light blue–magenta background, our Main Map depicts three distinct sections. Northern Ireland is only included in outline format, rendering the Republic of Ireland land mass our primary focus. The Republic of Ireland land mass is presented according to Electoral Division boundaries. The coordinate system used to visualise this data is the Irish Transverse Mercator (EPSG: 2157).
The first section of our Main Map, on the top-left, illustrates the choropleth of land-use polygons collected from the CORINE Land Cover dataset and that from the OpenStreetMap dataset as described in Section 2.1. Here, OpenStreetMap's thoroughness in urban areas relative to that of CORINE is evident while CORINE's national comprehensiveness is displayed.
The second section, the bottom left, contrasts the relative entropy scores attributable to every Irish ED when using the CORINE dataset alone with those computed using the combined CORINE and OpenStreetMap dataset.Footnote5 It is evident that discrepancies in the datasets are most pronounced in urban areas and in rural areas, particularly rural areas in the West of Ireland.
The principal component of our Main Map is presented on the right-hand half of the Main Map. Specifically, it denotes our principal legend, which is constructed according to equal interval measures, and provides regional contextual points and boundaries by illustrating where city centres are located, and the scale of Electoral Divisions. Additionally, this map visualises the differences in relative entropy calculations between our two datasets. This is complimented by inset shapefiles which highlight these differences in urban core areas, whereby ‘core’ is defined as per OECD definitions (Dijkstra et al., Citation2019). Positive values in our Main Map indicate that relative entropy estimates are higher when using our CORINE-OpenStreetMap dataset while negative values indicate that relative entropy estimates are lower when using our CORINE-OpenStreetMap dataset, highlighting imprecisions in measurement.
We chose our colour schemes to accommodate natural inclinations that associate darker colours with larger values and lighter colours with smaller values (Calka, Citation2021; Schiewe, Citation2019). Conscious of not isolating colour-blind readers, we avoid red-green combinations. Subsequently, we implement a viridis colour ramp on maps displaying relative entropy scores. We adopt a three-dimensional colour scheme to illustrate the results of our Main Map because these span positive and negative values. Specifically, we adopt a three-dimensional dark magenta, grey, green-cyan colour hue, which complements our alternative colour scheme by showing darker colours for larger values.
3. Results and discussion
3.1. CORINE
Lighter colours indicate lower levels of relative entropy and by implication, greater segregation in land-use developments. Supporting existing evidence, the CORINE database indicates that relative entropy is minimised in regional peripheries (Eldeeb et al., Citation2021), demonstrating land-use developments in these areas to be inherently unsustainable as a result of low land-use mixing (Nechyba & Walsh, Citation2004). However, rural areas generally exhibit higher relative entropy scores, indicating a greater mixing of land-use, while central areas also exhibit low relative entropy scores. This appears contradictory to existing evidence which shows that human settlements characterised by mixed-use developments will have higher relative entropy estimates due to greater efficiencies in land and infrastructure use, something indicative of greater levels of developmental sustainability (Mavoa et al., Citation2018; OECD, Citation2018).
3.2. CORINE and OSM
Tables 1, 2, and 3, illustrate the comprehensiveness of the CORINE dataset and its potential to investigate land-use sustainability and efficiency across large geographies. However, we observe that CORINE data lacks thoroughness in distinguishing land-uses within urban built environments, hence producing low relative entropy scores. OpenStreetMap provides a remedy for this potential issue by providing a thorough built environment catalogue, something further illustrated across and . Subsequently, we combine the CORINE dataset with our OpenStreetMap dataset and calculate relative entropy using arguably enhanced land-use measurements.
Our relative entropy calculations from this combined dataset depict a more theoretically consistent picture. By refining our built environment categories through OpenStreetMap, and by re-enforcing our peripheral/rural database with CORINE, we observe that relative entropy levels are generally at their highest in urban centres, where land-use is characterised by compactness and mixed-use (Ewing, Citation1997; OECD, Citation2018). High levels of relative entropy in our combined map outside of major urban centres illustrate the limitations associated with OpenStreetMap. Rural/coastal land-use pockets are only comprehensively incorporated through CORINE alone due to a lack of thoroughness in OSM, thereby artificially identifying greater quantities of land-use per area, and thus giving the illusion of mixed-use developmental forms.
3.3. Implications for policymakers
Policymakers’ interests lay principally in our Main Map, whereby the discrepancies in relative entropy scores between our two datasets are illustrated. We see that across urban centres relative entropy scores are systematically underestimated by the CORINE dataset, the most comprehensive land-use dataset for Ireland. This finding is attributable to a lack of refined categories for urban built environments. Somewhat surprisingly, the Main Map indicates that CORINE generally overestimates relative entropy scores in rural areas. However, this finding may be principally attributable to the fact that the CORINE dataset is more heavily weighted towards documenting rural land-use categories, thereby creating a bias in land-type counts.
Our Main Map illustrates systematic disparities in the relative entropy of urban areas, thereby directly impacting the interpretation of land-use sustainability studies. We see that relative entropy in central areas is generally higher than typically accounted for whereas relative entropy in fringe areas is generally lower. This suggests that existing peripheral expansion patterns in Ireland are inherently less sustainable and efficient in their use of land and space than those adopted in urban centres (CitationO'Driscoll et al., 2023a), and that the data typically overestimate the sustainability and efficiency of these peripheral developments.
4. Conclusions
This paper accompanies our Main Map which visualises the relative entropy of land-use configurations across the Republic of Ireland, whereby relative entropy is used to measure the sustainability and efficiency of land-use patterns. By comparing the measurements of discipline-standard datasets with those of novel open-source data, we gain inferences into the relative accuracy of these data and enhance existing knowledge on the sustainability of land-use across small geographical spaces.
Our principal finding is that relative entropy in urban areas is systematically underestimated by standard land-use datasets and that the incorporation of opensource built environment data enhances existing methodologies by clearly distinguishing between urban centres, regional peripheries, and rural areas. We find that relative entropy is generally at its highest in urban centres, which are areas characterised by mixed-use developments, whereas relative entropy decreases in peripheries, implying land-use policies in these areas may be unsustainable.
This research has some limitations and proposes numerous future research avenues. Specifically, while we sought to enhance land-use sustainability metrics, future research could expand this by explicitly incorporating transport networks and population dynamics into land-use datasets. Additionally, extensions could be made whereby relative entropy is regionalised and compared to base geographies, thereby acknowledging regional heterogeneities in developmental patterns. Finally, comparisons between CORINE and OpenStreetMap data should be applied to more geographies to test the robustness of these findings.
Software
QGIS 3.16 was used to collate the OpenStreetMap and CORINE data alongside construct the maps. Relative entropy was calculated using a STATA script.
Supplemental Material
Download MS Word (19.9 KB)Supplemental Material
Download PDF (57.9 MB)Acknowledgements
We would like to thank Dr. Paul Holloway and Mr. Josh O’Driscoll for their comments and suggestions on early drafts of our Main Map and manuscript.
Disclosure statement
No potential conflict of interest was reported by the author(s).
Data availability statement
The data that support our findings are available in the public domain via OpenStreetMap through the QGIS plugin QuickOSM and via The Copernicus Land Monitoring Service which is accessible via https://land.copernicus.eu/pan-european/corine-land-cover/clc2018. The rectified dataset forms part of a PhD project and is currently not ready to share.
Notes
1 We interpret comprehensiveness as a dataset's total coverage. We interpret thoroughness as the depth of detail provided by a dataset.
2 An alternative guide for land-use classification is CitationClark and Scott (Citation2013), who create relative entropy measures using self-aggregated land-use classes.
3 Some of these data are straddled along the Republic of Ireland and Northern Ireland border, whereby the majority concentrate around the Dundalk and Lifford-Stribane areas (major towns in the border region). In this context, land-use data are clipped according to boundary layers. The most affected classes include Residential Land, Agricultural Land, and Public Green Land.
4 For an in-depth discussion on this topic, see: CitationSong et al. (Citation2013).
5 OpenStreetMap is unable to calculate entropy on its own because its underlying data does not comprehensively cover the national land mass, which is a mathematical requirement of the entropy measure.
References
- Ahrens, A., & Lyons, S. (2019). Changes in land cover and urban sprawl in Ireland from a comparative perspective over 1990-2012. Land, 8(1), 1–14. https://doi.org/10.3390/land8010016
- Badland, H., & Schofield, G. (2005). Transport, urban design, and physical activity: An evidence-based update. Transportation Research Part D: Transport and Environment, 10(3), 177–196. https://doi.org/10.1016/j.trd.2004.12.001
- Bhatta, B., Saraswati, S., & Bandyopadhyay, D. (2010). Urban sprawl measurement from remote sensing data. Applied Geography, 30(4), 731–740. https://doi.org/10.1016/j.apgeog.2010.02.002
- Boarnet, G., & Sarmiento, S. (1998). Can land-use policy really affect travel behaviour? A study of the link between non-work travel and land-use characteristics. Urban Studies, 35(7), 1155–1169. https://www.jstor.org/stable/43083936
- Calka, B. (2021). Bivariate choropleth map documenting land cover intensity and population growth in Poland 2006–2018. Journal of Maps, 17(1), 163–169. https://doi.org/10.1080/17445647.2021.2009925
- Cao, X. (2016). How does neighborhood design affect life satisfaction? Evidence from Twin Cities. Travel Behaviour and Society, 5, 68–76. https://doi.org/10.1016/j.tbs.2015.07.001
- Carpio-Pinedo, J., Benito-Moreno, M., & Lamíquiz-Daudén, P. J. (2021). Beyond land use mix, walkable trips. An approach based on parcel-level land use data and network analysis. Journal of Maps, 17(1), 23–30. https://doi.org/10.1080/17445647.2021.1875063
- Central Statistics Office (CSO). (2016). Census 2016.
- Clark, A. F., & Scott, D. M. (2013). Does the social environment influence active travel? An investigation of walking in Hamilton, Canada. Journal of Transport Geography, 31, 278–285. https://doi.org/10.1016/j.jtrangeo.2013.06.005
- Dieleman, F., & Wegener, M. (2004). Compact city and urban sprawl. Built Environment, 30(4), 308–323. https://www.jstor.org/stable/24026084
- Dijkstra, L., Poelman, H., & Veneri, P. (2019). The EU-OECD definition of a functional urban area. OECD Regional Development Working Papers. 2019/11. https://doi.org/10.1787/d58cb34d-en
- Druga, M., & Minár, J. (2018). Exposure to human influence – A geographical field approximating intensity of human influence on landscape structure. Journal of Maps, 14(2), 486–493. https://doi.org/10.1080/17445647.2018.1493408
- Eldeeb, G., Mohamed, M., & Páez, A. (2021). Built for active travel? Investigating the contextual effects of the built environment on transportation mode choice. Journal of Transport Geography, 96, 1–14. https://doi.org/10.1016/j.jtrangeo.2021.103158
- Ewing, R. (1997). Is Los Angeles-style sprawl desirable? Journal of the American Planning Association, 63(1), 107–126. https://doi.org/10.1080/01944369708975728
- Faccini, F., Giardino, M., Paliaga, G., Perotti, L., & Brandolini, P. (2021). Urban geomorphology of Genoa old city (Italy). Journal of Maps, 17(4), 51–64. https://doi.org/10.1080/17445647.2020.1777214
- Fleischmann, M., & Arribas-Bel, D. (2021, June 29–July 3). XXVIII International seminar on urban form (ISUF2021): Urban form and the sustainable and prosperous cities. Classifying urban form at national scale: The British morphosignatures, Glasgow, Scotland.
- Gan, Z., Yang, M., Zeng, Q., & Timmermans, H. J. P. (2021). Associations between built environment, perceived walkability/bikeability and metro transfer patterns. Transportation Research Part A: Policy and Practice, 153, 171–187. https://doi.org/10.1016/j.tra.2021.09.007
- Gao, Y., Li, M., & Jiang, P. (2020). Land-type mapping and its application to land-use zoning: A case study of Jiangsu province, eastern China. Journal of Maps, 16(2), 847–854. https://doi.org/10.1080/17445647.2020.1838959
- Huang, H.-J., Xia, T., Tian, Q., Liu, T.-L., Wang, C., & Li, D. (2020). Transportation issues in developing China’s urban agglomerations. Transport Policy, 85, A1–A22. https://doi.org/10.1016/j.tranpol.2019.09.007
- Jeong, J., Lee, J., & Gim, T. T. (2022). Travel mode choice as a representation of travel utility: A multilevel approach reflecting the hierarchical structure of trip, individual, and neighborhood characteristics. Papers in Regional Science, 101(3), 745–765. https://doi.org/10.1111/pirs.12665
- Luan, H., & Fuller, D. (2022). Urban form in Canada at a small-area level: Quantifying “compactness” and “sprawl” with Bayesian multivariate spatial factor analysis. Environment and Planning B: Urban Analytics and City Science, 49(4), 1300–1313. https://doi.org/10.1177/23998083211062901
- Mavoa, S., Eagleson, S., Badland, H. M., Gunn, L., Boulange, C., Stewart, J., & Giles-Corti, B. (2018). Identifying appropriate land-use mix measures for use in a national walkability index. Journal of Transport and Land Use, 11(1), 681–700. https://doi.org/10.5198/jtlu.2018.1132
- Millward, H., Spinney, J., & Scott, D. (2013). Active-transport walking behavior: Destinations, durations, distances. Journal of Transport Geography, 28, 101–110. https://doi.org/10.1016/j.jtrangeo.2012.11.012
- Nechyba, T. J., & Walsh, R. P. (2004). Urban sprawl. Journal of Economic Perspectives, 18(4), 177–200. https://doi.org/10.1257/0895330042632681
- Newman, P. W. G., & Kenworthy, J. R. (1996). The land use—Transport connection: An overview. Land Use Policy, 13(1), 1–22. https://doi.org/10.1016/0264-8377(95)00027-5
- O’Driscoll, C., Crowley, F., Doran, J., & McCarthy, N. (2023a). How the relationship between socio-demographics, residential environments and travel influence commuter choices. Regional Studies, 30, 1–18. http://doi.org/10.1080/00343404.2023.2199779
- O’Driscoll, C., Crowley, F., Doran, J., & McCarthy, N. (2023). Land-use mixing in Irish cities: Implications for sustainable development. Land Use Policy, 128, 1–7. https://doi.org/10.1016/j.landusepol.2023.106615
- O’Driscoll, J., Meredith, D., Crowley, F., Doran, J., O’Shaughnessy, M., & Zimmermann, J. (2022). The spatiotemporal dimension of population change in Ireland: Visualisation of growth and shrinkage in Irish Electoral Divisions (1986–2016). Journal of Maps, 18(3), 551–557. https://doi.org/10.1080/17445647.2022.2052766
- OECD. (2018). Rethinking urban sprawl: Moving towards sustainable cities (policy highlights). https://doi.org/10.1787/9789264189881-en
- Ordnance Survey Ireland (OSI). (2016). Sea area - OSi National 250k Map of Ireland. Retrieved March 27, 2022, from https://data.gov.ie/dataset/sea-area-osi-national-250k-map-of-ireland2?package_type=dataset
- Paterson, M. (2000). Car culture and global environmental politics. Review of International Studies, 26(2), 253–270. https://doi.org/10.1017/S0260210500002539
- Pooley, C. G., & Turnbull, J. (2005). Coping with congestion: Responses to urban traffic problems in British cities c.1920–1960. Journal of Historical Geography, 31(1), 78–93. https://doi.org/10.1016/j.jhg.2003.08.019
- Qiao, W., Wang, Y., Ji, Q., Hu, Y., Ge, D., & Cao, M. (2019). Analysis of the evolution of urban three-dimensional morphology: The case of Nanjing city, China. Journal of Maps, 15(1), 30–38. https://doi.org/10.1080/17445647.2019.1568922
- Schiewe, J. (2019). Empirical studies on the visual perception of spatial patterns in choropleth maps. KN - Journal of Cartography and Geographic Information, 69(3), 217–228. https://doi.org/10.1007/s42489-019-00026-y
- Song, Y., Merlin, L., & Rodriguez, D. (2013). Comparing measures of urban land use mix. Computers, Environment and Urban Systems, 42, 1–13. https://doi.org/10.1016/j.compenvurbsys.2013.08.001
- Strollo, A., Smiraglia, D., Bruno, R., Assennato, F., Congedo, L., De Fioravante, P., Giuliani, C., Marinosci, I., Riitano, N., & Munafò, M. (2020). Land consumption in Italy. Journal of Maps, 16(1), 113–123. https://doi.org/10.1080/17445647.2020.1758808
- Ton, D., Duives, D. C., Cats, O., Hoogendoorn-Lanser, S., & Hoogendoorn, S. P. (2019). Cycling or walking? Determinants of mode choice in the Netherlands. Transportation Research Part A: Policy and Practice, 123, 7–23. https://doi.org/10.1016/j.tra.2018.08.023
- Zhang, M., & Zhao, P. (2017). The impact of land-use mix on residents’ travel energy consumption: New evidence from Beijing. Transportation Research Part D: Transport and Environment, 57, 224–236. https://doi.org/10.1016/j.trd.2017.09.020