ABSTRACT
Hydrological processes are hard to accurately simulate and predict because of various natural and human influences. In order to improve the simulation and prediction accuracy of the hydrological process, the firefly algorithm with deep learning (DLFA) was used in this study to optimise the parameters of support vector for regression (SVR) automatically, and a prediction model was established based on DLFA and SVR. The hydrological process of Huangfuchuan in Fugu County, Shanxi Province was taken as the research object to verify the performance of the prediction model, and the results were compared with those by the other six prediction models. The experimental results showed that the proposed prediction model achieved improved prediction performance compared with the other six models.
GRAPHICAL ABSTRACT
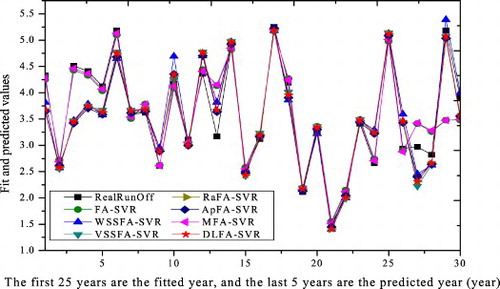