1. Introduction
Since the first introduction of Artificial Intelligence (AI) in 1956, its aim was to tackle problems with human intelligence, but with greater speed and accuracy. AI has been inspired a lot by natural swarm intelligences, such as those observed in groupings of animals and insects. Swarm intelligences not only are observed in our surroundings, but also exist within the human body. For instance, vertebrae immune system can be considered as a swarm intelligence in that it consists of independent, self-organized agents that interact with one another to form a higher intelligence [Citation1]. AI has profited from immune system and artificial immune system has been of use in overcoming challenges such as intrusion detection, self-healing of robots, optimization and anomaly detection. As much as immunology has served the AI, recently AI is being used in many immunological fields. Beside remarkable analytic abilities, antigen and phenotype detection, predicting prognosis and treatment outcomes, etc., are examples where AI is serving the immunologists in the field.
2. AI at molecular levels
Machine learning (ML) is an AI tool that can process huge imported data, process them and generate models for those data and their alikes to fit in. This allows AI to classify inputs and predict outcomes. Many algorithms have been developed to predict the outcome of interactions and classification at molecular levels (). For instance, ML can predict genotypes associated with poor prognosis [Citation2]. Phenotype detection is another example of the classification abilities of AI. Cellular phenotype detection and classification can determine the presence of a particular disease or its outcome. In case of certain diseases, the importance of phenotype classification at molecular level is more prominent since it can directly determine prognosis and treatment plan. For instance, HIV-1 response to therapy can be predicted based on viral phenotype, as certain phenotypes are associated with drug resistance. Determining the genotype leading to resistant phenotypes can be easy in some cases as these viruses are subject to point mutations. However, determining mechanisms leading to resistance in other viruses is complicated [Citation3]. Many factors influence resistance and determining the impact of each factor is complicated. ML can determine the resistance-associated phenotypic features and classify virus strains upon them. It can also determine the weight of each factor precisely, a complicated and time-consuming task to be done by conventional statistical analyses [Citation3].
Figure 1. Machine learning methodologies frequently used in immunology: In order for data to be processed by machine learning algorithms, they should be first preprocessed. There are different preprocessing methods such as denoising, feature extraction, etc., based on the type of data used. Then these data are processed using different algorithms in machine learning.
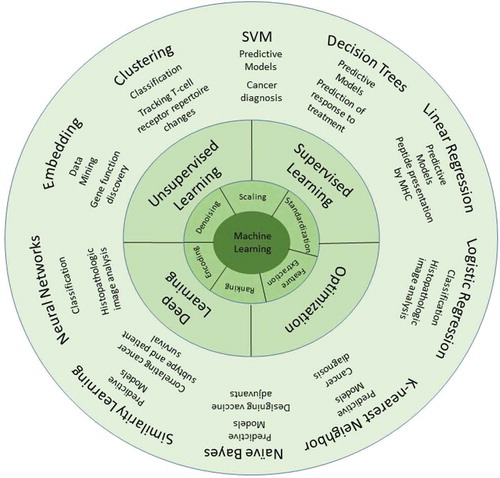
Another way of phenotype detection is image-based phenotype detection. The image-based techniques have remarkable accuracy at both cellular and molecular levels. The classical methods of microscopic visual inspection of tissues are time-consuming and prone to subjectivity. Furthermore, they cannot keep up with the data collected from high-throughput studies. Machines not only can keep up with the pace that the data are generated in modern medicine, but can also detect phenotypic changes that could slip through human screening [Citation4]. The scope of machine screening can be adjusted to detect only a phenotypic alteration of interest or screen for a wider variety of phenotypes. These image-based techniques have been recruited to determine immune responses such as macrophage activation [Citation5] and lymphocyte infiltration in breast cancer and other solid tumors [Citation6], hence assist in determining prognosis and response to treatment.
One of the fields in immunology for which many accurate algorithms exist is predicting the immunopeptidome. Determining whether a peptide can be presented by the MHC molecules is of utmost importance and can be a step forward towards personalized vaccine development. The most accurate way to determine immunopeptidome is mass spectrometry (MS). However, it is an expensive and time-consuming technique. ML not only can determine the immunopeptidome, but it can also be trained by the current data on human immunopeptidome and predict whether a given peptide can be presented by MHC-I molecules. Data acquired from in vitro studies provide information on peptide-MHC affinity. These data can be used to generate models that can predict presentability of neoantigens discovered through whole exome sequencing of tumors by MHC molecules. Predicting epitopes for MHC-II molecules is even further challenging due to different peptide length. AI-based algorithms have shown promising efficacy in predicting MHC-II epitopes based on their amino acid sequence and designing vaccines targeting MHC-II immunopeptidome [Citation7,Citation8]. However, since these models are based on in vitro data, they may not predict in vivo interactions with the same level of accuracy.
Apart from determining the human immunopeptidome, ML has served vaccine development in other ways. ML can help design optimum adjuvants for vaccines by determining epitopes in antigens. For this purpose, machines are trained based on epitope properties retrieved from existing data on epitope of antigens [Citation6]. A scoring system based on the driven epitope properties predicts how likely a given structure (3D) or sequence (2D) is to play the role of an epitope. ML can even go further with predicting the tertiary structure of a protein from scratch based on its amino acid sequence and predict its binding to MHC molecules [Citation7]. A recently proposed support vector machine-based classifier can distinguish epitope peptides from non-epitope peptides [Citation9] and determine the immunogenicity of a given peptide. Similar efforts have been made at the level of DNA and RNA to determine oligonucleotides that have immunomodulatory properties [Citation10]. Many of these in silico cell models have shown promising predictive properties in designing vaccines and computationally evaluate their effectiveness [Citation11]. Furthermore, ML can accelerate finding new candidate antigens in reverse vaccinology [Citation12]. However, the accuracy of these classifiers depends on the set of data and retrieved features they are trained upon. Therefore, it is important to constantly enrich the dataset training these machines.
3. AI in the field
Application of AI is not limited to molecular levels. Apart from detecting potential targets for developing novel therapeutic approaches and predicting their efficacy at in vitro level, AI can be applied at clinical levels and their application can go beyond their analytic power. Recruiting AIs can change the face of clinical trials. AI can check patients for their eligibility to participate in the trials by going through their electronic medical records. It can analyze both structured and non-structured clinical records and compares patients’ information and the eligibility criteria for a given study. This way, AI-based software can propose clinical trials suitable for patients which will increase the probability of patients’ enrolment in a suitable clinical trial [Citation13]. After the enrolment of the patients, AI can increase their adherence to clinical trials by providing constant emotional support by answering their queries. Another example of benefiting from AI in clinical trials and clinics is supervision on patients’ ingestion of medication. Video-based applications use AI algorithms to identify the patient’s face and the drug and make sure that the drug is swallowed (e.g. NCT02243670). Same technology can be adapted for drugs administered other than oral route; however, no algorithm has been provided for this purpose yet.
4. Future perspective
Many studies have recruited AI in biological fields, and their algorithms are mostly publicly accessible online. The fact that these algorithms are designed for public databases allows their comparison in the future and this way algorithms with higher accuracy are developed. summarizes some of these databases which have been used for the development of many of these algorithms.
Table 1. Some of the public databases used to develop algorithms in the field of immunology using machine learning methodologies.
Using AI-based methods apart from saving time and economic resources, has the potential to model entirely artificial studies. AI’s ability to predict interactions such as peptide-MHC affinity is one of its major strength points which can be used to develop new prophylactic/therapeutic agents such as vaccines using neoantigens. Furthermore, AI can simulate non-human and human subjects in preclinical and clinical studies. With the right data set to be trained upon, there is a possibility that AI replace human subjects in clinical trials, at least in early phases. However, such implementation of AI may be ethically a subject of debate as there may remain hidden aspects to in vivo interactions that are not predicted in silico. In order to move towards in silico clinical trials, consensus must be reached between stakeholders and researchers. However, amidst the rapid development of computational medicine, many questions arise: How far can AI go in medical fields? Should replacing humans in clinical trials be considered as the ultimate goal of AI in medicine? How far are we from attaining this probably ultimate goal?
5. Conclusions
The pre-computational era of medicine has provided enough data regarding disease status, prognostic factors and therapeutic approaches to pave the road for AI to use these data to come up with better solutions. The past few decades have witnessed tremendous evolutions in computational medicine. Today, machines are able to go through databases, retrieve desired information and recruit this information to predict outcome and design therapeutic interventions for a given individual. We believe that more studies have the potential to recruit AI. This can be specifically the case for immunology, where there is a tight relationship between the molecular and clinical levels.
Declaration of interest
The authors have no relevant affiliations or financial involvement with any organization or entity with a financial interest in or financial conflict with the subject matter or materials discussed in the manuscript. This includes employment, consultancies, honoraria, stock ownership or options, expert testimony, grants or patents received or pending, or royalties.
Reviewer disclosures
Peer reviewers on this manuscript have no relevant financial or other relationships to disclose.
Additional information
Funding
References
- Timmis J, Andrews P, Hart E. On artificial immune systems and swarm intelligence. Swarm Intell. 2010;4(4):247–273.
- Yu J, Hu Y, Xu Y, et al. LUADpp: an effective prediction model on prognosis of lung adenocarcinomas based on somatic mutational features. BMC Cancer. 2019 March 22;19(1):263.
- Hepler NL, Scheffler K, Weaver S, et al. IDEPI: rapid prediction of HIV-1 antibody epitopes and other phenotypic features from sequence data using a flexible machine learning platform. PLoS Comput Biol. 2014 Sep;10(9):e1003842.
- Vandenberghe ME, Scott MLJ, Scorer PW, et al. Relevance of deep learning to facilitate the diagnosis of HER2 status in breast cancer. Sci Rep. 2017 April 05;7:45938. online.
- Pavillon N, Hobro AJ, Akira S, et al. Noninvasive detection of macrophage activation with single-cell resolution through machine learning. Proc Nat Acad Sci. 2018;115(12):E2676–E2685.
- Sun R, Limkin EJ, Vakalopoulou M, et al. A radiomics approach to assess tumour-infiltrating CD8 cells and response to anti-PD-1 or anti-PD-L1 immunotherapy: an imaging biomarker, retrospective multicohort study. Lancet Oncol. 2018;19(9):1180–1191.
- Moghram BA, Nabil E, Badr A. Ab-initio conformational epitope structure prediction using genetic algorithm and SVM for vaccine design. Comput Methods Programs Biomed. 2018 January 01;153:161–170.
- Degoot AM, Chirove F, Ndifon W. Trans-Allelic Model for Prediction of Peptide: MHC-IIInteractions [Methods]. Front Immunol. 2018 June 20;9(1410).
- Nagpal G, Chaudhary K, Agrawal P, et al. Computer-aided prediction of antigen presenting cell modulators for designing peptide-based vaccine adjuvants. J Transl Med. 2018 July 03;16(1):181.
- Nagpal G, Gupta S, Chaudhary K, et al. VaccineDA: prediction, design and genome-wide screening of oligodeoxynucleotide-based vaccine adjuvants [Article]. Sci Rep. 2015 July 27;5:12478. online.
- Dash R, Das R, Junaid M, et al. In silico-based vaccine design against Ebola virus glycoprotein. Adv Appl Bioinf Chem AABC. 2017;10:11–28.
- Heinson AI, Gunawardana Y, Moesker B, et al. Enhancing the biological relevance of machine learning classifiers for reverse vaccinology. Int J Mol Sci. 2017 Feb 1;18(2).
- Helgeson J, Rammage M, Urman A, et al. Clinical performance pilot using cognitive computing for clinical trial matching at Mayo Clinic. J Clin Oncol. 2018;36(15_suppl):e18598–e18598.
- Li Y, Niu M, Zou Q. ELM-MHC: an Improved MHC identification method with extreme learning machine algorithm. J Proteome Res. 2019 Mar 1;18(3):1392–1401.
- Hoof I, Peters B, Sidney J, et al. NetMHCpan, a method for MHC class I binding prediction beyond humans. Immunogenetics. 2009;61(1):1–13.