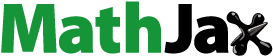
Abstract
This study examines the association between sales order backlog and credit ratings. We posit that credit rating agencies consider order backlog as a positive signal about strong future demand and incorporate that into their rating decisions and provide higher ratings to firms with substantial order backlogs. However, being a non-GAAP, unaudited metric, order backlog could also reduce financial reporting quality and hence, credit ratings of firms. Using a sample of US firms from 1980 to 2017, we find a positive and significant association between order backlog and credit ratings, suggesting that order backlog serves as a valuable measure in credit rating assessment by providing positive signals about future earnings to rating agencies.
1. Introduction
We examine the association between sales order backlog (hereafter order backlog) and credit ratings. Order backlog denotes contractual orders that are not fulfilled but are scheduled to be completed in subsequent accounting periods. Item 101(c) (VIII) of SEC regulations S-K requires disclosure prior to 2020, to the extent material, of the dollar amount of the order backlog believed to be firm, as of a recent date and as of a comparable date in the preceding fiscal year, together with an indication of the portion thereof not reasonably expected to be filled within the current fiscal year, and seasonal or other material aspects of backlog (Rajgopal et al., Citation2003, p. 466). Order backlog is a non-GAAP lead indicator disclosed in the financial statements containing value-relevant information related to future earnings and, thus, perceived as a positive news by the capital markets (Baik & Ahn, Citation2007; Behn, Citation1996; Rajgopal et al., Citation2003). In a 2022 interview at the Qatar Economic Forum conducted by the Bloomberg, Tesla’s Chief Executive Officer emphasized the strength of the firm’s sales order backlog.Footnote1 Subsequently, Tesla’s shares experienced a significant surge of 9%, surpassing the Nasdaq’s 2.5% rebound from the previous week’s decline. Industry analysts attributed Tesla’s notable outperformance to the positive sentiment surrounding the firm’s sales order backlog.
Prior research has established that order backlog is value-relevant (Lev & Thiagarajan, Citation1993), predicts future earnings (Behn, Citation1996; Rajgopal et al., Citation2003) and thus is associated with market returns (Feldman et al., Citation2021; Rajgopal et al., Citation2003). Despite the availability of research demonstrating the value-relevance and predictive power of order backlog for market returns and future earnings, examining the association between order backlog and credit ratings is important for several reasons. First, credit rating agencies focus primarily on a firm’s ability to meet its debt obligations and assess its default risk, which may not align directly with the interest of equity investors seeking growth and profitability (Chu, Citation2017; Jensen & Meckling, Citation1976; Smith & Warner, Citation1979). Second, although several studies examine various determinants of credit ratings (e.g. Akins, Citation2018; Alissa et al., Citation2013; Ashbaugh-Skaife et al., Citation2006; Attig et al., Citation2013; Bonsall & Miller, Citation2017; Habib & Ranasinghe, Citation2022a; Citation2022b), there is no evidence whether rating agencies consider non-GAAP information, such as order backlog, in determining credit ratings. Managers can use non-GAAP tools at their disposal either to mislead investors (Black & Christensen, Citation2009) or to increase the quality of information environment (Marques, Citation2006). We fill this void in the literature by examining whether and how rating agencies use order backlog information in their rating decisions.
As discussed above, order backlog is a useful leading indicator in predicting future earnings and is embedded in stock prices (Behn, Citation1996; Rajgopal et al., Citation2003), but Lev and Thiagarajan (Citation1993) find that changes in order backlog relative to sales have a negative effect on earnings persistence, suggesting revenue manipulation through order backlog. Barber and Hollie (Citation2021) find that revenue recognition restatements are common when changes in order backlog are high. This paper adds to this stream of the literature by examining whether rating agencies consider order backlogs in rating decisions.
We argue that order backlog can be associated with firm’s credit ratings in the following ways. A significant order backlog indicates strong customer demand and potential future revenue (Baik & Ahn, Citation2007; Behn, Citation1996; Rajgopal et al., Citation2003), which can be viewed positively by credit rating agencies. Since rating agencies incorporate both quantitative and qualitative information in their rating decisions (Cornaggia et al., Citation2017), we argue that rating agencies consider order backlog as a positive indicator and rate firms accordingly. However, order backlog is a non-GAAP metric, and the frequency, consistency and comparability of non-GAAP disclosures has been a concern regarding the credibility of financial statements because order backlog is an unaudited metric (Feldman et al., Citation2021). Managers have incentives to engage in revenue manipulation by changing order backlog (Lev & Thiagarajan, Citation1993). Since, rating agencies use both private and publicly available information, among other sources of information, to determine ratings, such disclosure could reduce financial reporting quality (Barber & Hollie, Citation2021) and hence, credit ratings of firms. Although prior research suggests that earnings manipulation is factored into rating decisions (Alissa et al., Citation2013; Brown et al., Citation2015), there is no evidence on whether rating agencies are able to recognize managerial revenue manipulation through order backlog.
Using a US sample from 1987 to 2017, we find a significant and positive relation between order backlog proxied by log of order backlog and order backlog scaled by average total assets and credit ratings. The coefficient on the third proxy for order backlog, change in sales minus changes in order backlog (Lev & Thiagarajan, Citation1993), is insignificant. We then decompose this measure into positive and negative values to assess the possibility of managerial manipulation. A positive value indicates an upward manipulation of sales, or a decline in the order backlog, both likely act as negative indicators. Consistent with this, we find the coefficient on order backlog for this group negative and significant. Conversely, a negative value indicates conservative reporting of sales or increase of order backlog, both likely act as positive indicators. We find the coefficient on order backlog for this group positive and significant, suggesting that credit rating agencies understand the implications of conservative reporting, or increase in orders, and provide higher ratings to more conservative reporting. Our findings hold even after addressing endogeneity concerns which we address by performing entropy-balanced regression, and a two-stage regression analysis.
This paper contributes to the literature on order backlog. Prior research has found that order backlog is an important leading non-GAAP metric that contains information about future earnings (Baik & Ahn, Citation2007; Behn, Citation1996; Penman, Citation2007). Building upon this body of knowledge, our research goes a step further by investigating whether rating agencies consider order backlog when making their rating decisions. In doing so, we not only expand the existing literature on credit ratings but also provide insights into the role of order backlog in this context. While our study focuses on the US data, its implications for European stakeholders remain relevant. Understanding the relationship between order backlog and credit ratings highlights the importance of leading indicators in evaluating firms’ creditworthiness. With the adoption of the International Financial Reporting Standards (IFRS) 15 ‘Revenue from Contracts with Customers’ in Europe in 2018, firms must disclose both the estimated transaction price for remaining performance obligations (i.e. order backlog) and the associated timing. This regulatory requirement offers European regulators insights into the value of integrating such non-GAAP metric into their frameworks, potentially influencing disclosure standards. Investors in European markets stand to gain from recognizing order backlog’s value as a forward-looking indicator of firms’ future earnings and creditworthiness, thereby improving their decision-making processes. Furthermore, our findings underscore credit rating agencies’ awareness of managerial incentives related to order backlog manipulation, emphasizing the necessity for vigilance and transparency in financial reporting practices among European firms.
The paper is organized as follows. In Section 2, we present the related literature and develop the hypotheses. In Section 3, we present our sample selection and research design. In Section 4, we report the results. Section 5 concludes our study.
2. Literature and Hypotheses
2.1. Literature on Order Backlog
A stream of research investigates the value-relevance of non-GAAP measures which are leading indicators such as order backlog, but the empirical findings remain mixed (Behn, Citation1996; Francis et al., Citation2003; Rajgopal et al., Citation2003). Behn (Citation1996) provides some evidence that order backlog provides useful information about future earnings for a small sample of 90 firms, but Francis et al. (Citation2003) fail to find so, after controlling for earnings disclosures for the homebuilding industry. Rajgopal et al. (Citation2003) conclude that investors overweigh information in sales order backlog with respect to future earnings despite equity analysts correctly impounding information in order backlog in their forecasts.Footnote2 In contrast, Baik and Ahn (Citation2007) conclude that investors under-weigh the effects of sales order backlog for future earnings. Chang et al. (Citation2018) find sales order backlog turns out to be a noisier indication for predicting future earnings for the upstream suppliers due to the so-called ‘bullwhip’ effect. Also, financial analysts fail to fully incorporate the predictability of order backlog in their forecasting decisions in the presence of the bullwhip effect. Building upon the notion of change in inventory backlog, Lev and Thiagarajan (Citation1993) argue that an increase in sales order backlog may not necessarily indicate a genuine increase in demand, but rather a managerial earnings management technique. According to Penman (Citation2007), a decrease in order backlog serves as a warning signal for investors when they are forecasting a firm’s future operating performance and market value. Feldman et al. (Citation2021) demonstrate the usefulness of growth in order backlog as a predictive indicator of future sales. Further, the authors find that both quarterly quantitative order backlog increases and a positive qualitative tone in the order backlog disclosures are associated with drift returns. Gu and Huang (Citation2010) evidence that stocks of firms experiencing an increase in order backlogs exhibit stronger momentum compared to stocks of firms without such increases. The authors also find current order backlog has a positive association with future sales growth as well as investment growth.
Although a significant amount of research has investigated the stock market implications of order backlog, a limited number of recent studies have examined its influence on other operational outcomes such as financial reporting quality, cash holdings, and CSR activities. Deng et al. (Citation2020) document a negative association between order backlog and cash holdings, supporting the notion that high order backlog may be indicative of future firm performance and relatively low levels of future business risks. Sun et al. (Citation2019) suggest that firms with high order backlogs ought to prioritize the fulfillment of these orders, which may leave them with limited resources to devote to CSR initiatives and, therefore, have poor CSR performance. Barber and Hollie (Citation2021) contend that order backlog reduces financial reporting quality due to the potential for revenue restatements stemming from the complexity of revenue recognition.
2.2. Literature on Credit Rating
Credit ratings provide an assessment of the creditworthiness and credit risk of an organization that measures the extent to which the borrower can meet the financial commitments (Cheng & Subramanyam, Citation2008). Credit rating agencies are financial intermediaries that assign credit ratings for the borrowing organizations which reflects overall credit quality. Accordingly, prior research finds that credit ratings have important implications for corporate outcomes and capital market consequences (see for example, Basu et al., Citation2022; Even-Tov & Ozel, Citation2021; Gounopoulos & Pham, Citation2017; Kisgen, Citation2006; Norden & Weber, Citation2004).
Credit rating agencies utilize various quantitative and qualitative characteristics of firms to determine credit quality (Cornaggia et al., Citation2017). Evidence suggests that rating agencies factor financial reporting quality in making their rating decisions. For example, Bonsall and Miller (Citation2017) find that financial reporting readability affects credit rating while Jung et al. (Citation2013) find income smoothing is a determinant of credit ratings. Brown et al. (Citation2015) and Alissa et al. (Citation2013) find that real earnings management affects credit ratings. Drawing insights from an informational role, risk mitigating role and monitoring role of brand capital, Hasan and Taylor (Citation2023) argue that credit rating agencies factor brand capital in determining borrowers’ credit risk and credit worthiness. Drawing insights from legitimacy theory, social contract theory, and stakeholder theory, a stream of research documents a positive association between firms’ CSR activities and credit ratings (Attig et al., Citation2013; Chintrakarn et al., Citation2020; Jiraporn et al., Citation2014; Oikonomou et al., Citation2014; Safiullah et al., Citation2021). Fama and Jensen (Citation1983) suggest that various corporate governance mechanisms constrain managerial opportunistic behavior and protect shareholder and debtholder interests. A stream of research examines how various governance mechanisms and characteristics of the top executive team affect rating decisions (Ashbaugh-Skaife et al., Citation2006; Benson et al., Citation2018; Datta et al., Citation2021; Khatami et al., Citation2016; Lee et al., Citation2021; Park et al., Citation2022). From the perspectives of risk and information asymmetry, studies find that analysts (Cheng & Subramanyam, Citation2008), managerial ability (Cornaggia et al., Citation2017), firm life cycle (Blomkvist et al., Citation2021), labor investment efficiency (Habib & Ranasinghe, Citation2022a), asset redeployability (Habib & Ranasinghe, Citation2022b), business strategy (Dang et al., Citation2022) and innovation efficiency (Griffin et al., Citation2018) are some of the factors that rating agencies look at when assigning credit ratings.
2.3. Hypothesis Development
In the context of order backlog, Chang et al. (Citation2018) underscore the significance of this tool in predicting future sales and earnings, particularly in firms with extended operating cycles. Financial analysts face challenges in fully grasping order backlog predictability on average, but those overseeing entire vertical supply chains demonstrate a superior understanding. Furthermore, Feldman et al. (Citation2021) contribute to this disclosure by emphasizing the value of quarterly order backlog disclosures. They emphasize the forecasting benefits of order backlog growth and positive signals from qualitative disclosures, aligning with the use of signals to convey positive prospects for the future of firms. Toynbee (Citation2017) complements this by revealing improved performance metrics for firms disclosing order backlog, particularly those with make-to-order business models, suggesting that order backlog serves as a meaningful indicator of demand.
We posit that higher order backlog levels are associated with good credit ratings due to its ability to convey positive indications about future earnings. As rating agencies factor quantitative and qualitative information in their rating decisions (Cornaggia et al., Citation2017), we argue that leading indicators such as order backlog are significant factors that credit rating agencies consider in rating firms. Order backlog provides positive signals of future sales and stock returns (Behn, Citation1996; Feldman et al., Citation2021; Rajgopal et al., Citation2003). In addition, Lev and Thiagarajan (Citation1993) suggest that order backlog disclosures are one of the main lead indicator variables that analysts consider as signals of future earnings. Therefore, we argue that order backlog provides positive signals about future earnings to rating agencies. As a result, rating agencies expect firms with high order backlogs to enjoy increased future earnings and are more likely to pay their debt and interest in a timely manner: predictors of good credit ratings.
However, a negative association between order backlog and credit rating is also plausible. Order backlog is an unaudited, non-GAAP, metric. The frequency, consistency and comparability of these disclosures raised concerns regarding the credibility of financial statements (Feldman et al., Citation2021). Managers can use the timing and quantity of the order backlog information to manipulate earnings, which increases information asymmetry between debtholders and insiders. For example, Barber and Hollie (Citation2021) find that managers with opportunistic intentions manipulate the timing difference in order backlog reporting, which results in revenue restatements. Rating agencies derive information from private and publicly available information to determine ratings. But, the information asymmetry triggered by the high order backlog can reduce rating quality. Given the competing arguments presented above, we develop the following hypothesis.
H1: There is no association between order backlog and credit ratings.
3. Research Design
3.1. Sample
Our initial sample consists of non-missing Standard & Poor’s (S&P) domestic long-term issuer credit ratings (RATING) data, encompassing the period from 1980 to 2017. The starting period of 1980 aligns with the availability of RATING data in Compustat. We limit our sample to 2017, as S&P discontinued provision of rating data via Capital IQ beyond that year. We exclude financials (SIC codes 60–69) and utility firms (SIC code 49) from our sample and begin with 76,966 firm-year observations. Our sample size reduces to 45,064 firm-year observations because of missing asset data. We further lose 11,743 firm-year observations for calculating discretionary accruals (DAC), a proxy for financial reporting quality included in the baseline regression model. We then drop 18,715 firm-year observations with missing order backlog (OB) data.Footnote3 Missing values for other control variables reduce the baseline sample to 10,181 firm-year observations. To reduce the impact of outliers, we winsorize all the continuous variables at the top and bottom one percentiles. The observations differ in any given model due to the data requirements of each model. presents the industry distribution based on the two-digit SIC industry classification. About 34% of sample observations are from machinery, electrical, and computer equipment followed by 22% from the store merchandise, auto dealers, and home furniture stores. This is consistent with prior research which suggests that OB is generally concentrated in durable manufacturing industries (Rajgopal et al., Citation2003). Business services account for only 9.2% of the sample observations. We source the required data from BoardEx, Compustat, and Thomson Reuters Institutional Holdings Database (13F).
Table 1. Industry distribution.
3.2. Empirical Model
To test the association between order backlog and credit ratings, we use the following OLS regression model.
(1)
(1) Our dependent variable RATING ranges from AAA (extremely strong capacity to meet financial obligations) to CC (highly vulnerable) and D (in general default). To conduct our empirical analysis, we convert the S&P credit rating to numeric values varying from 1 (rating D) to 21 (AAA). A higher value of ratings indicates better credit quality. Our variable of primary interest is OB. We use three specifications of OB, namely, the natural logarithm of OB (LN_OB), OB scaled by average total assets (AVG_OB) (Rajgopal et al., Citation2003; Sun et al., Citation2019) and changes in sales minus changes in order backlog (ΔSALE-ΔOB) (Lev & Thiagarajan, Citation1993). We decompose the ΔSALE-ΔOB into positive values (ΔSALE-ΔOBPOS) and negative values (ΔSALE-ΔOBNEG) to gauge the possibility of manipulation. A positive value indicates an upward manipulation of sales, or a decline in the OB. We take the view that this is a managerial attempt to manipulate revenue and/or OB and if the rating agencies correctly incorporate this information into their rating decision, then the coefficient on ΔSALE-ΔOBPOS should be negative.Footnote4 Conversely, a negative value of ΔSALE-ΔOB indicates conservative reporting of sales or an increase of OB and rating agencies view this conservative reporting behavior positively when awarding their rating decisions and we should expect a positive coefficient on ΔSALE-ΔOBNEG.
We include a set of control variables that affect firm’s credit ratings following prior literature (Attig et al., Citation2013; Cornaggia et al., Citation2017; Jiang et al., Citation2018). These variables include absolute discretionary accruals (DAC), intangible assets scaled by total assets (INTAN), interest coverage ratio (INTCOV), total debt scaled by total assets (LEV), a dummy variable for negative net income (LOSS), market value of equity to book value of equity (MTB), operating cash flows scaled by total assets (OCF), research and development expenses divided by total assets (R&D), income before extraordinary item scaled by shareholders’ equity (ROE), the natural logarithm of total assets (SIZE), a dummy variable if the firm has subordinated debt (SUB), gross property, plant and equipment scaled by total assets (TANG), and financial distress value of Altman (Z_DUM). We include dummy variables in our OLS regressions to account for year and industry (2-digit SIC code) effects. See the Appendix for the variable definitions.
4. Sample and Results
4.1. Descriptive Statistics
We present descriptive statistics of the variables used in the study in . RATING has an average value of 10.49 which corresponds to the S&P rating category of BB. The mean (median) values of LN_OB and AVG_OB are 3.428 (3.85) and 0.335 (0.12), respectively. Sun et al. (Citation2019) report a mean (median) AVG_OB of 0.34 (0.12) for their sample period spanning from 1992 to 2016. The mean (median) ΔSALE-ΔOB is 0.016 (0.041). The mean (median) ΔSALE-ΔOBPOS is 0.185 (0.122), while it is −0.247 (−0.128) for ΔSALE-ΔOBNEG. The average firm size (SIZE) in our sample is 6.675 and has a leverage (LEV) of 25.8%. MTB takes a mean value of 2.509. The sample firms, on average, are profit-making firms (a mean ROE of 12.20%). Intangible assets (INTAN) have an average of 17.10%. About 78.30% of the firm-year observations report an Altman z-score (Z_DUM) higher than 1.81: a score considered to represent firm solvency.
Table 2. Descriptive statistics.
4.2. Correlation Analysis
presents the correlation analysis. The correlation between RATING and LN_OB is positive and significant (correlation 0.225, p < 0.01), and so is the correlation between RATING and AVG_OB (correlation 0.045, p < 0.01), thereby, suggesting that rating agencies favorably view firm’s order backlog. The correlation between ΔSALE-ΔOB and RATING is insignificant. RATING is positively correlated with INTCOV, MTB, OCF, ROE, SIZE, Z_DUM, and (correlations 0.054, 0.061, 0.095, 0.087, 0.554, 0.161; p < 0.01 respectively), but negatively correlated with DAC, LEV, LOSS, and SUB (correlations −0.146, −0.194, −0.168, and −0.177; p < 0.01 respectively). These correlations are consistent with prior research (Cheng & Subramanyam, Citation2008; Hasan & Taylor, Citation2023). Multicollinearity is not a concern, as the highest correlation is −0.524 between TANG and INTAN, which is well within the acceptable limit.
Table 3. Correlation analysis.
4.3. Regression Results
In , Panel A, we present the OLS regression results for the association between order backlog and credit ratings while controlling for the determinants of credit ratings as in Equation (1). Column (1) shows the coefficient on LN_OB positive and significant (β = 0.221, p < 0.01), suggesting that firms with high order backlogs have better credit ratings. Economically, the estimated coefficient implies a 0.246 increase in rating (). The coefficient of the alternative measure of order backlog, AVG_OB, is also positive and significant (β = 0.441, p < 0.05) (Column 2). An interquartile change in AVG_OB is associated with (0.441*0.373) = 0.164 increase in credit rating. The third measure, ΔSALE-ΔOB is not significantly associated with credit rating (Column 3). Since this measure consists of both positive and negative values, we also regress RATING on ΔSALE-ΔOBPOS and ΔSALE-ΔOBNEG in columns (4) and (5). The coefficient on ΔSALE-ΔOBPOS is negative and significant (β = −1.685, p < 0.01) (Column 4). A shift from 25th (less aggressive) to the 75th (more aggressive) percentile of ΔSALE-ΔOBPOS is associated with −1.685*(0.057–0.241) = 0.31 increase in rating. The coefficient on ΔSALE-ΔOBNEG is positive and significant (β = 0.923, p < 0.01) (Column 5), suggesting that conservative reporting of revenue is accurately incorporated into the rating decisions by the rating agencies. With respect to ΔSALE-ΔOBNEG, the 75th (25th) percentile is less (more) conservative. So, a shift towards more conservative reporting is associated with 0.923*(−0.049 – (−0.299)) = 0.23 increase in rating. It should, however, be noted that at the mean, the effect on rating is −0.247*0.923 = −0.23 for ΔSALE-ΔOBNEG but 0.185*(−1.685) = −0.31 for ΔSALE-ΔOBPOS. Our ΔSALE-ΔOBNEG group, i.e. the conservative reporting group, exhibits a higher mean (−0.245) as opposed to the aggressive reporting group (0.185). But, it appears that rating agencies overreact to the information embedded in OB for the ΔSALE-ΔOBPOS group (coefficient −1.68) as opposed to their ΔSALE-ΔOBNEG group counterpart (coefficient 0.923). Therefore, ratings are higher for the ΔSALE-ΔOBNEG group (−0.23) than the ΔSALE-ΔOBPOS group (−0.31). We, therefore, conclude that rating agencies regard differences between changes in sales and the OB suspiciously and hence react negatively to both, albeit at a small magnitude, but more so for the aggressive reporting group. The sign and significance of the control variables are generally consistent with prior credit ratings research. The coefficients on DAC, INTCOV, MTB, OCF, R&D, and SUB are negative and highly significant, whilst those on LOSS, SIZE, and TANG are positive and significant. The highest variance inflation factor (VIF) is 2.31 for TANG, which is below the maximum expected level of 10.00 (Marquardt, Citation1970; Studenmund, Citation2016). Accordingly, multicollinearity is not a concern.
Table 4. Regression results.
In Columns (1) to (5) in Panel B, we perform logit models with RATING as an indicator variable coded 1 if the RATING score is above 11 (where RATING is BBB- and above), i.e. investment-grade rating, and zero otherwise. The coefficients on LN_OB (Column 1) and AVG_OB (Column 2) are positive and significant at p < 0.01 and p < 0.05, respectively. As shown in Column (3), our third measure of order backlog, ΔSALE-ΔOB is not significant. The coefficient on ΔSALE-ΔOBPOS is negative and significant (coefficient −1.649, p < 0.01) (Column 4) while the coefficient on ΔSALE-ΔOBNEG is positive and significant (coefficient 0.694, p < 0.01) (Column 5). In Column (6), we present an ordered probit model as per Ashbaugh-Skaife et al. (Citation2006) and find a positive and significant coefficient on LN_OB (coefficient 0.158, p < 0.01). The corresponding coefficient on AVG_OB is 0.332 (p < 0.05) (Column 7). Again, we do not find significant results for ΔSALE-ΔOB (Column 8). The coefficient on ΔSALE-ΔOBPOS is negative and significant (coefficient −1.149, p < 0.01) (Column 9), while the coefficient on ΔSALE-ΔOBNEG is positive and significant (coefficient 0.557, p < 0.01) (Column 10).
The positive and significant association between order backlog level measures (LN_OB and AVG_OB) and RATING suggest that rating agencies consider order backlog information as leading indicators of future earnings information and rate the firms with high order backlog with high ratings. As opposed to the level measures of order backlog, our third measure of order backlog, ΔSALE-ΔOB reflects the managerial attempt to manipulate revenue (Lev & Thiagarajan, Citation1993; Rajgopal et al., Citation2003). To assess this manipulative behavior, we present separate regression estimates for the negative and positive values of ΔSALE-ΔOB. Our findings support the assertion that managerial attempt to manipulate OB relative to sales (i.e. the positive values of ΔSALE-ΔOB) are reflected in lower ratings. On the other hand, managerial conservative reporting behavior (i.e. the negative values of ΔSALE-ΔOB) is taken into consideration as manifested in higher rating for this group of firms. These results, therefore, reject H1.
4.4. Addressing Endogeneity
The reported results in Section 4.3 could be biased due to endogeneity concerns despite including various firm characteristics that may explain the association between order backlog and credit ratings. Endogeneity issues may arise due to design choices, reverse causation problem and omitted variable bias. shows the results of endogeneity tests performed to address the above concerns. First, we address omitted variable bias by including five firm-level governance variables which have been shown to be important determinants of credit ratings in Columns (1) to (5) of , Panel A. Although our sample size drops from 10,181 to 2,849, we still find positive and significant coefficients for both LN_OB (coefficient 0.203, p < 0.05) and AVG_OB (coefficient 0.474, p < 0.10). As shown in Column (3), we do not find significant results for ΔSALE-ΔOB measure. In Column (4), we find the coefficient on ΔSALE-ΔOBPOS negative and significant (coefficient −1.423, p < 0.01). This finding is similar to the result presented in . However, the coefficient is insignificant for the ΔSALE-ΔOBNEG group (Column 5). In Columns (6) to (10), we include real earnings management (REM) (Alissa et al., Citation2013; Brown et al., Citation2015); analyst following (FOLLOW) (Cheng & Subramanyam, Citation2008), asset redeployability (REDEPLOY) (Habib & Ranasinghe, Citation2022b), annual report readability (BOG) (Bonsall & Miller, Citation2017), managerial ability (ABILITY) (Cornaggia et al., Citation2017), and labor investment efficiency (LABOR_EFF) (Habib & Ranasinghe, Citation2022a) to further mitigate omitted variable concerns. Our conclusion remains unchanged as we still find the coefficients on LN_OB and AVG_OV positive and significant, while the coefficients on ΔSALE-ΔOB remain insignificant. We also find the coefficient on ΔSALE-ΔOBPOS negative and significant while the coefficient on ΔSALE-ΔOBNEG positive and significant. We conclude that our original results are robust even after controlling for omitted variables bias.
Table 5. Endogeneity tests.
Second, we address bias around design choices using entropy balancing method. McMullin and Schonberger (Citation2020) suggest that entropy balancing method improves covariate balance. Further, entropy balancing increases the balancing quality whilst preserving all observations in both the control and treatment groups (Hainmueller, Citation2012). Based on the median OB value, we divide our sample into two groups. Specifically, the treated group includes the observations that have an OB value above the sample median while the control group contains the observations with OB values below the sample median. We present the covariate matching result in Panel B. The outcome is in line with the contention that covariates are balanced between treated and control groups. Then we perform our baseline regression model using the entropy-balanced sample. As shown in Column (1), the coefficient on LN_OB is positive and significant for RATING (β = 0.217, p < 0.01) and so is for AVG_OB (β = 0.426, p < 0.10) (Column 2). The coefficient on ΔSALE-ΔOB is insignificant (Column 3).
Finally, we apply the two-stage least square (2SLS) regression estimations and present the results in Panel C of . We mainly address the reverse causation-related endogeneity problem using the 2SLS model. Specifically, while the initial assertion was that order backlog information affects credit rating decisions, reverse causality suggests that the relationship can also flow in the opposite direction, with credit ratings significantly impacting managers’ intention to adjust order backlog. To mitigate the endogeneity concern, instrumental variable(s) are employed in the first stage to estimate the endogenous variables, which are correlated with the explanatory variables but not directly with the error term of the dependent variable. Subsequently, in the second stage, the predicted values obtained from the first stage are included in the main regression equation: a technique referred to as 2SLS (Wooldridge, Citation2010).
We use LN_OB_INDMEAN, the mean of LN_OB based on the two-digit SIC industry in each year excluding the focal firm, as our instrument. We expect this to be highly correlated with LN_OB because prior research suggests that firms within specific industries tend to adopt the industry norms (Pool et al., Citation2015), but it is not likely to directly affect RATING. , Panel C, Column 1, shows that the coefficient on LN_OB_INDMEAN is positive and highly significant (β = 0.880, p < 0.01), thereby confirming the validity of the chosen instrument. As shown in Column (2), we find a statistically significant positive association between RATING_INTSRUMENTED and RATING (β = 0.288, p < 0.01). The LM statistic suggests that the instrument is ‘relevant.’ The Stock and Yogo (Citation2005) critical value of 16.38 is lower than the Cragg–Donald F-statistic of 1169.91. This suggests that our instrument does not suffer from weak identification. We find similar results when AVG_OB_INDMEAN is used as the instrument. As shown in Column (3), the coefficient on AVG_OB_INDMEAN is positive and highly significant (β = 0.938, p < 0.01), confirming the validity of the instrument. Column (4) shows the coefficient on RATING_INTSRUMENTED is positive and significant (β = 0.830, p < 0.10). The LM statistic suggests that the instrument is ‘relevant.’ A much greater Cragg-Donald F-statistic suggests again that the instrument does not suffer from weak identification. In Column (5), we present the first stage regression estimates when the industry-year mean of ΔSALE-ΔOB is used as the instrument (ΔSALE-ΔOB_INDMEAN). We find that the coefficient is positive and significant suggesting the validity of our instrument (β = 0.991, p < 0.01). As shown in Column (6), we find the coefficient on RATING_INTSRUMENTED insignificant consistent with the baseline result. The endogeneity tests conducted above confirm that our empirical results are not affected by endogeneity problems.
4.5. Additional Tests
(1) | We also test whether there is any industry difference between the relationship between OB and RATING. Our industry distribution clearly reveals that durable manufacturing constitutes a sizable proportion of our sample observations as these industries have a significant amount of OB (Rajgopal et al., Citation2003). We partition our sample into consumer durables, manufacturing, and business equipment and code them as 1 (IND_1) while other firms-year observations as zero and run the baseline regressions for these two industry groups separately. We find the coefficient for LN_OB positive and significant (coefficient 0.31, t-stat 2.68) for IND_1 group. The corresponding coefficient for IND_0 group is positive but insignificant. We find consistent evidence for the AVG_OB measure. | ||||
(2) | Sun et al. (Citation2019) suggest that the level of sales order backlog could be influenced by macroeconomic conditions. One such macroeconomic condition could be economic policy uncertainty (EPU). EPU can be defined as the extent of unpredictability of the government's economic policy changes regarding fiscal, monetary and regulatory policies (Baker et al., Citation2016; Gulen & Ion, Citation2016). During these uncertain times, firms encounter more challenges, and their operational risks are escalated. Consequently, default risks are higher during EPU (Boumparis et al., Citation2017). Therefore, rating agencies are likely to award lower ratings to firms during EPU due to lower levels of credit worthiness and credit risks involved (Orden-Cruz et al., Citation2023). When we include EPU in Equation (1) we indeed find a significant and negative coefficient on EPU (coefficient −0.006, p < 0.01) (untabulated). The coefficient on the interactive variable OB*EPU is positive (coefficient 0.0009, p < 0.01) (untabulated). This suggests that although EPU adversely affects credit ratings, order backlogs act as a positive signaling mechanism during periods of heightened EPU. |
5. Conclusion
We investigate the association between order backlog and credit rating. While there is a plethora of research that examines various determinants of credit ratings, no evidence exists on whether lead indicators such as order backlog is associated with credit ratings. Lead indicators are important non-GAAP metric that provide important information to the capital markets. Credit rating agencies as financial intermediaries use public and private information in reaching their credit rating decisions. Therefore, it is important to understand whether leading indicators reported in financial statements are considered by rating agencies. We argue that order backlog provides information on sales and unfulfilled orders to the market regarding future earnings, which is a positive indicator for rating agencies in terms of firms’ creditworthiness. Using a sample of US data, we find that firms with higher order backlogs tend to receive higher credit ratings, suggesting that order backlog serve as a valuable non-GAAP measure in credit rating assessment. To capture the nuances of rating agencies’ roles in gathering information, we analyze managerial incentives and actions related to changes of order backlog relative to sales and find that credit rating agencies understand the implications of conservative reporting, or increase in orders, and provide higher ratings to more conservative reporting firms for this group.
Acknowledgement
We thank the Associate Editor, Gilad Livne for providing many thoughtful and constructive comments for improving the contents of the manuscript. We also appreciate helpful comments from an anonymous reviewer.
Disclosure Statement
No potential conflict of interest was reported by the author(s).
Notes
1 Why Elon Musk Is Nearly $15 Billion Richer Tuesday (forbes.com).
2 Although Rajgopal et al. (Citation2003) find the overall stock market misprices order backlog information, Chen et al. (Citation2024) document that mispricing is concentrated in upstream supplier firms’ valuation, possibly because the market's failure to properly incorporate the bullwhip effect. However, Chen et al. (Citation2024) find that common institutional investors, i.e., institutional investors having stocks in both supplier and customer firms in a supply chain, can utilize their knowledge to mitigate the magnitude of order backlog mispricing.
3 Capital IQ defines order backlog as … OB represents the dollar value of firm backlog orders (in millions) as of the company's fiscal year-end. It encompasses both sales backlog and unfunded backlog. However, this item is unavailable for railroads, finance, savings and loan, airline companies, or utility companies.
4 An alternative explanation exists for the positive values of ΔSALE-ΔOB. Specifically, a positive value might indicate that sales are growing faster than the order backlog, possibly signalling strong demand and operational efficiency, which could be viewed favorably by credit rating agencies.
References
- Akins, B. (2018). Financial reporting quality and uncertainty about credit risk among ratings agencies. The Accounting Review, 93(4), 1–22. https://doi.org/10.2308/accr-51944
- Alissa, W., Bonsall, S. B., IV, Koharki, K., & Penn, M. W., Jr. (2013). Firms’ use of accounting discretion to influence their credit ratings. Journal of Accounting and Economics, 55(2-3), 129–147. https://doi.org/10.1016/j.jacceco.2013.01.001
- Altman, E. I. (1968). Financial ratios, discriminant analysis and the prediction of corporate bankruptcy. The Journal of Finance, 23(4), 589–609. https://doi.org/10.1111/j.1540-6261.1968.tb00843.x
- Ashbaugh-Skaife, H., Collins, D. W., & LaFond, R. (2006). The effects of corporate governance on firms’ credit ratings. Journal of Accounting and Economics, 42(1-2), 203–243. https://doi.org/10.1016/j.jacceco.2006.02.003
- Attig, N., El Ghoul, S., Guedhami, O., & Suh, J. (2013). Corporate social responsibility and credit ratings. Journal of Business Ethics, 117(4), 679–694. https://doi.org/10.1007/s10551-013-1714-2
- Baik, B., & Ahn, T. S. (2007). Changes in order backlog and future returns. Seoul Journal of Business, 13(2), 105–126. https://doi.org/10.35152/snusjb.2007.13.2.005
- Baker, S. R., Bloom, N., & Davis, S. J. (2016). Measuring economic policy uncertainty. The Quarterly Journal of Economics, 131(4), 1593–1636. https://doi.org/10.1093/qje/qjw024
- Barber, R., & Hollie, D. (2021). Does order backlog matter for financial reporting quality? Evidence from revenue restatements. Advances in Accounting, 53, 100526. https://doi.org/10.1016/j.adiac.2021.100526
- Basu, R., Naughton, J. P., & Wang, C. (2022). The regulatory role of credit ratings and voluntary disclosure. The Accounting Review, 97(2), 25–50. https://doi.org/10.2308/TAR-2018-0286
- Behn, B. (1996). Value implications of unfilled order backlogs. Advances in Accounting, 14(1), 61–84.
- Benson, B. W., Iyer, S. R., Kemper, K. J., & Zhao, J. (2018). Director networks and credit ratings. Financial Review, 53(2), 301–336. https://doi.org/10.1111/fire.12157
- Black, D. E., & Christensen, T. (2009). US managers’ use of ‘pro forma’ adjustments to meet strategic earnings targets. Journal of Business Finance & Accounting, 36(3-4), 297–326. https://doi.org/10.1111/j.1468-5957.2009.02128.x
- Blomkvist, M., Löflund, A., & Vyas, H. (2021). Credit ratings and firm life-cycle. Finance Research Letters, 39, 101598. https://doi.org/10.1016/j.frl.2020.101598
- Bonsall, S. B., & Miller, B. P. (2017). The impact of narrative disclosure readability on bond ratings and the cost of debt. Review of Accounting Studies, 22(2), 608–643. https://doi.org/10.1007/s11142-017-9388-0
- Boumparis, P., Milas, C., & Panagiotidis, T. (2017). Economic policy uncertainty and sovereign credit rating decisions: Panel quantile evidence for the eurozone. Journal of International Money and Finance, 79, 39–71. https://doi.org/10.1016/j.jimonfin.2017.08.007
- Brown, K., Chen, V. Y., & Kim, M. (2015). Earnings management through real activities choices of firms near the investment–speculative grade borderline. Journal of Accounting and Public Policy, 34(1), 74–94. https://doi.org/10.1016/j.jaccpubpol.2014.09.002
- Chang, H., Chen, J., Hsu, S. W., & Mashruwala, R. (2018). The impact of the bullwhip effect on sales and earnings prediction using order backlog. Contemporary Accounting Research, 35(2), 1140–1165. https://doi.org/10.1111/1911-3846.12401
- Chen, J., Kim, S., Srinivasan, D., & Zhou, Y. (2024). Can common institutional investors in supply chains decode the bullwhip effect? Evidence from order backlog mispricing. Journal of Accounting, Auditing & Finance, online early. https://doi.org/10.1177/0148558X241229508.
- Cheng, M., & Subramanyam, K. R. (2008). Analyst following and credit ratings. Contemporary Accounting Research, 25(4), 1007–1044. https://doi.org/10.1506/car.25.4.3
- Chintrakarn, P., Treepongkaruna, S., Jiraporn, P., & Lee, S. M. (2020). Do LGBT-supportive corporate policies improve credit ratings? An instrumental-variable analysis. Journal of Business Ethics, 162(1), 31–45. https://doi.org/10.1007/s10551-018-4009-9
- Chu, Y. (2017). Shareholder litigation, shareholder-creditor conflict, and the cost of bank loans. Journal of Corporate Finance, 45, 318–332. https://doi.org/10.1016/j.jcorpfin.2017.05.005
- Coles, J. L., Daniel, N. D., & Naveen, L. (2014). Co-opted boards. Review of Financial Studies, 27(6), 1751–1796. https://doi.org/10.1093/rfs/hhu011
- Cornaggia, K. J., Krishnan, G. V., & Wang, C. (2017). Managerial ability and credit ratings. Contemporary Accounting Research, 34(4), 2094–2122. https://doi.org/10.1111/1911-3846.12334
- Dang, M., Puwanenthiren, P., Jones, E., Nguyen, T. Q., Vo, X. V., & Nadarajah, S. (2022). Strategic archetypes, credit ratings, and cost of debt. Economic Modelling, 114, 105917. https://doi.org/10.1016/j.econmod.2022.105917
- Datta, S., Doan, T., & Toscano, F. (2021). Top executive gender, board gender diversity, and financing decisions: Evidence from debt structure choice. Journal of Banking & Finance, 125, 106070. https://doi.org/10.1016/j.jbankfin.2021.106070
- Demerjian, P., Lev, B., Lewis, M., & McVay, S. (2013). Managerial ability and earnings quality. The Accounting Review, 88(2), 463–498. https://doi.org/10.2308/accr-50318
- Deng, K., Nichols, L., & Sun, L. (2020). Sales order backlog and corporate cash holdings. Asian Review of Accounting, 28(3), 351–371. https://doi.org/10.1108/ARA-08-2019-0147
- Even-Tov, O., & Ozel, N. B. (2021). What moves stock prices around credit rating changes? Review of Accounting Studies, 26(4), 1390–1427. https://doi.org/10.1007/s11142-020-09573-6
- Fama, E. F., & Jensen, M. C. (1983). Separation of ownership and control. The Journal of Law and Economics, 26(2), 301–325. https://doi.org/10.1086/467037
- Feldman, R., Govindaraj, S., Livnat, J., & Suslava, K. (2021). Market reaction to quantitative and qualitative order backlog disclosures. Journal of Accounting and Public Policy, 40(6), 106897. https://doi.org/10.1016/j.jaccpubpol.2021.106897
- Francis, J., Schipper, K., & Vincent, L. (2003). The relative and incremental explanatory power of earnings and alternative (to earnings) performance measures for returns. Contemporary Accounting Research, 20(1), 121–164. https://doi.org/10.1506/XVQV-NQ4A-08EX-FC8A
- Gounopoulos, D., & Pham, H. (2017). Credit ratings and earnings management around IPOs. Journal of Business Finance & Accounting, 44(1-2), 154–195. https://doi.org/10.1111/jbfa.12228
- Griffin, P. A., Hong, H. A., & Ryou, J. W. (2018). Corporate innovative efficiency: Evidence of effects on credit ratings. Journal of Corporate Finance, 51, 352–373. https://doi.org/10.1016/j.jcorpfin.2018.06.007
- Gu, L. I., & Huang, D. (2010). Sales order backlogs and momentum profits. Journal of Banking & Finance, 34(7), 1564–1575. https://doi.org/10.1016/j.jbankfin.2010.03.003
- Gulen, H., & Ion, M. (2016). Policy uncertainty and corporate investment. The Review of Financial Studies, 29(3), 523–564.
- Habib, A., & Ranasinghe, D. (2022a). Labor investment efficiency and credit ratings. Finance Research Letters, 48, 102924. https://doi.org/10.1016/j.frl.2022.102924
- Habib, A., & Ranasinghe, D. (2022b). Asset redeployability and credit ratings. Finance Research Letters, 50, 103295. https://doi.org/10.1016/j.frl.2022.103295
- Hainmueller, J. (2012). Entropy balancing for causal effects: A multivariate reweighting method to produce balanced samples in observational studies. Political Analysis, 20(1), 25–46. https://doi.org/10.1093/pan/mpr025
- Hasan, M. M., & Taylor, G. (2023). Brand capital and credit ratings. The European Journal of Finance, 29(2), 228–254. https://doi.org/10.1080/1351847X.2022.2029751
- Jensen, M. C., & Meckling, W. H. (1976). Theory of the firm: Managerial behavior, agency costs, and ownership structure. Journal of Financial Economics, 3(4), 305–360. https://doi.org/10.1016/0304-405X(76)90026-X
- Jiang, F., John, K., Li, C. W., & Qian, Y. (2018). Earthly reward to the religious: Religiosity and the costs of public and private debt. Journal of Financial and Quantitative Analysis, 53(5), 2131–2160. https://doi.org/10.1017/S002210901800039X
- Jiraporn, P., Jiraporn, N., Boeprasert, A., & Chang, K. (2014). Does corporate social responsibility (CSR) improve credit ratings? Evidence from geographic identification. Financial Management, 43(3), 505–531. https://doi.org/10.1111/fima.12044
- Jung, B., Lee, W. J., & Weber, D. P. (2014). Financial reporting quality and labor investment efficiency. Contemporary Accounting Research, 31(4), 1047–1076. https://doi.org/10.1111/1911-3846.12053
- Jung, B., Soderstrom, N., & Yang, Y. S. (2013). Earnings smoothing activities of firms to manage credit ratings. Contemporary Accounting Research, 30(2), 645–676. https://doi.org/10.1111/j.1911-3846.2012.01170.x
- Khatami, S. H., Marchica, M. T., & Mura, R. (2016). Rating friends: The effect of personal connections on credit ratings. Journal of Corporate Finance, 39, 222–241. https://doi.org/10.1016/j.jcorpfin.2016.04.006
- Kim, H., & Kung, H. (2017). The asset redeployability channel: How uncertainty affects corporate investment. Review of Financial Studies, 30(1), 245–280. https://doi.org/10.1093/rfs/hhv076
- Kisgen, D. J. (2006). Credit ratings and capital structure. The Journal of Finance, 61(3), 1035–1072. https://doi.org/10.1111/j.1540-6261.2006.00866.x
- Kothari, S. P., Leone, A. J., & Wasley, C. E. (2005). Performance matched discretionary accrual measures. Journal of Accounting and Economics, 39(1), 163–197. https://doi.org/10.1016/j.jacceco.2004.11.002
- Lee, S. M., Jiraporn, P., Kim, Y. S., & Park, K. J. (2021). Do co-opted directors influence corporate risk-taking and credit ratings? The Quarterly Review of Economics and Finance, 79, 330–344. https://doi.org/10.1016/j.qref.2020.07.003
- Lev, B., & Thiagarajan, S. R. (1993). Fundamental information analysis. Journal of Accounting Research, 31(2), 190–215. https://doi.org/10.2307/2491270
- Marquardt, D. W. (1970). Generalized inverses, ridge regression, biased linear estimation, and nonlinear estimation. Technometrics, 12(3), 591–612. https://doi.org/10.2307/1267205
- Marques, A. (2006). SEC interventions and the frequency and usefulness of non-GAAP financial measures. Review of Accounting Studies, 11(4), 549–574. https://doi.org/10.1007/s11142-006-9016-x
- McMullin, J. L., & Schonberger, B. (2020). Entropy-balanced accruals. Review of Accounting Studies, 25(1), 84–119. https://doi.org/10.1007/s11142-019-09525-9
- Norden, L., & Weber, M. (2004). Informational efficiency of credit default swap and stock markets: The impact of credit rating announcements. Journal of Banking & Finance, 28(11), 2813–2843. https://doi.org/10.1016/j.jbankfin.2004.06.011
- Oikonomou, I., Brooks, C., & Pavelin, S. (2014). The effects of corporate social performance on the cost of corporate debt and credit ratings. Financial Review, 49(1), 49–75. https://doi.org/10.1111/fire.12025
- Orden-Cruz, C., Paule-Vianez, J., & Lobão, J. (2023). The effect of economic policy uncertainty on the credit risk of US commercial banks. International Journal of Finance & Economics, 28(3), 3420–3436. https://doi.org/10.1002/ijfe.2600
- Park, J. W., Nam, G., Tsang, A., & Lee, Y. J. (2022). Firstborn CEOs and credit ratings. The British Accounting Review, 54(4), 101083. https://doi.org/10.1016/j.bar.2022.101083
- Penman, S. H. (2007). Financial statement analysis and security valuation (Vol. 3). McGraw-Hill.
- Pinnuck, M., & Lillis, A. M. (2007). Profits versus losses: Does reporting an accounting loss act as a heuristic trigger to exercise the abandonment option and divest employees? The Accounting Review, 82(4), 1031–1053. https://doi.org/10.2308/accr.2007.82.4.1031
- Pool, V. K., Stoffman, N., & Yonker, S. E. (2015). The people in your neighborhood: Social interactions and mutual fund portfolios. The Journal of Finance, 70(6), 2679–2732. https://doi.org/10.1111/jofi.12208
- Rajgopal, S., Shevlin, T., & Venkatachalam, M. (2003). Does the stock market fully appreciate the implications of leading indicators for future earnings? Evidence from order backlog. Review of Accounting Studies, 8(4), 461–492. https://doi.org/10.1023/A:1027364031775
- Roychowdhury, S. (2006). Earnings management through real activities manipulation. Journal of Accounting and Economics, 42(3), 335–370. https://doi.org/10.1016/j.jacceco.2006.01.002
- Safiullah, M., Kabir, M. N., & Miah, M. D. (2021). Carbon emissions and credit ratings. Energy Economics, 100, 105330. https://doi.org/10.1016/j.eneco.2021.105330
- Smith, C. W., & Warner, J. B. (1979). On financial contracting: An analysis of bond covenants. Journal of Financial Economics, 7(2), 117–161. https://doi.org/10.1016/0304-405X(79)90011-4
- Stock, J. H., & Yogo, M. (2005). Testing for weak instruments in linear IV regression, in identification and inference for econometric models: Essays in honour of Thomas Rothenberg, Working paper (Harvard University).
- Studenmund, A. H. (2016). Using econometrics: A practical guide (7th ed.). Pearson.
- Sun, L., Walkup, B., & Wu, K. (2019). Sales order backlog and corporate social responsibility. Advances in Accounting, 47, 100444. https://doi.org/10.1016/j.adiac.2019.100444
- Toynbee, S. (2017). Implications of disclosing order backlog [Doctoral dissertation]. University of Washington. https://digital.lib.washington.edu/researchworks/bitstream/handle/1773/39889/Toynbee_washington_0250E_16958.pdf?sequence=1&isAllowed=y
- Wooldridge, J. M. (2010). Econometric analysis of cross section and panel data. MIT Press.
Appendix
Variable definitions
Table