Abstract
The antipsychotic drug Olanzapine was crystallized with aromatic acid, 2,5-dihydroxybenzoic acid in isopropyl alcohol by slow evaporation which led to the formation of olanzapinium 2,5-dihydroxybenzoate crystalline salt. The structure of the compound was characterized by 1H-NMR, 13C-NMR, and single-crystal X-ray diffraction analysis. The Hirshfeld analyses were performed to quantify the order and nature of intermolecular interactions in the crystal network. Employing computational approaches, the compound was tested for its affinity against antipsychotic activity by molecular docking and molecular dynamic simulation to attest the conformational stability over time step of 100 ns. Besides, bioactivity and ADMET properties were also predicted to ratify the result. The compound asserted neither carcinogenic nor mutagenic activity and has high oral bioavailability. Hence, this synthesized novel compound Olanzapinium 2,5-dihydroxybenzoate recognized in the study possesses high potential as an effective antipsychotic compound, and can further be examined for its efficiency by in vivo studies. The synthesized compound was submitted to NCBI PubChem database using accession substance ID: 441329256. The crystal structure was submitted to CCDC (Cambridge Crystallographic Data Centre) with submission ID: 2010899.
1. Introduction
Antipsychotic medication, also known as neuroleptics is a class of psychotropics primarily manoeuvred for short- or long-term treatments of psychotic disorders (bipolar depression, delusions, paranoia, schizophrenia, etc.). Studies have revealed that Olanzapine, a novel atypical antipsychotic drug elicits a high response with low dosage on the patients suffering from psychotic ailments. It consists of a thieno-benzodiazepinyl structure and exhibits a similar in vitro binding profile with an atypical anti-psychotic agent; an FDA-approved drug, Clozapine [Citation1,Citation2]. According to the Biopharmaceutical Classification System (BCS), Olanzapine belongs to class II (low solubility, high permeability) cadre, and is highly effective in positive, negative, and cognitive symptoms for acute and relapsing schizophrenics. This drug is marketed under the trademark, Zyprexa by Eli Lilly and Company [Citation3,Citation4].
Pharmacokinetic studies have indoctrinated that the efficacy of Olanzapine is mediated through the combined effect of dopamine and serotonin type 2 form but in an antagonistic manner. Olanzapine shows a selective monoaminergic antagonistic property with augmented binding affinity to serotonin 5HT2A/2C, dopamine D14, muscarinic M1-5, and adrenergic α1 receptors, thereby manifesting a broad pharmacological profile [Citation5–7]. Despite myriad studies, the complete mechanism of action and efficiency of the drug Olanzapine is obfuscated, chiefly while dealing with schizophrenia.
For decades, an innumerable amount of antipsychotic drugs have emerged as therapeutic agents for schizoaffective disorders, bipolar mania, delusional ailments, etc. [Citation8–11]. Moreover, most of them encompass high affinity for blocking D2 receptors but exhibit severe side effects, such as motor abnormalities, weight gain, diabetes, cardiovascular disorders, etc.[Citation12,Citation13] Therefore, there is a requirement for new chemically tailored drugs with abridged after-effects.
In the current study, synthesis, and crystallization of a new compound, Olanzapinium 2,5-dihydroxybenzoat has been done by solution method with slow evaporation technique. Identification of the crystal structure with potentiation points is appraised via X-ray diffraction technique. The intermolecular interactions in the crystal lattice were assessed by employing the Hirshfeld surface analysis [Citation14]. Further analysis was performed using computational approaches for testing the affinity against potassium protein structure by molecular docking and molecular dynamic tools. Bioactivity and ADMET properties were also gauged via computer-aided programs. The molecular structure of the Olanzapinium 2,5-dihydroxybenzoate is illustrated in .
2. Materials and methods
2.1. Chemicals and reagents
Olanzapine and 2,5-dihydroxybenzoic acid were used as generic from Sigma-Aldrich (Steinheim, USA). Isopropyl Alcohol was purchased from Merck scientific Inc. (Darmstadt, Germany) and used as an effective solvent. All the chemicals and solvent were used without further purification.
Melting Points (mp) were measured in open capillaries on Nessler digital Auto melting point apparatus and were uncorrected. The 1H-NMR spectra was recorded on a 300 MHz (Bruker) spectrometer in appropriate solvents using Tetra methyl silane (TMS) as an internal standard. The solvent signals as secondary standards and the chemical shifts were reported in δ values (ppm). Coupling constants J were expressed in Hertz (Hz). The Signal multiplicities are represented by the following abbreviations: s (singlet), d (doublet), dd (double doublet), t (triplet), q (quartet), and m (multiplet). 13C-NMR spectra was recorded on 75 MHz spectrometers.
2.2. Synthesis and crystallization
Olanzapine (156 mg, 0.5 mmol) and 2,5-dihydroxybenzoic acid (77 mg, 0.5 mmol) were dissolved in 30 mL of isopropyl alcohol and stirred for 5 hours at 330K. The mixture was filtered and allowed slow evaporation of the solvent at room temperature; crystals were obtained within 2 weeks. To maintain a constant temperature of the solution, the growth vessel was kept in a constant temperature bath.The compound was fully characterized by 1H-NMR, 13C-NMR analysis ( and ). Further single-crystal X-ray diffraction analyses of the compound was unambiguously confirmed. The structure and stereochemistry of the compounds is illustrated in .
2.3. Structural analysis of the compound
Good optical quality and well-shaped single crystal were selected under high-resolution microscope for the single-crystal X-ray diffraction data collection. The intensity data were collected at room temperature using a Bruker Smart Apex CCD diffractometer with graphite monochromated MoKα radiation (λ = 0.71073 Å) by the ω-scan method. Preliminary lattice parameters and orientation matrices were obtained from four sets of frames. Integration and scaling of intensity data were accomplished using the program SAINT [Citation15]. The structures were solved by direct methods using SHELXS97 and refinement was carried out by full-matrix least-squares technique using SHELXL97.
2.4. Ligand and protein preparation
The module of the Schrodinger suite, PrepWiz was used to prepare the protein [Citation16–23]. The protein was pre-processed by assigning the bond orders and hydrogen, creating zero-order bonds to metals, and adding disulphide bonds. Further, the module of Schrodinger was used to fill up missing side chains and loops. Beyond 5 Å, all the water molecules were eliminated from the hetero groups. The H bonds were assigned, followed by energy minimization by OPLS 2005 force field after obtaining the pre-processed protein structure [Citation24–39]. The final refined structure of the protein was saved in.pdb format for further investigation and research. The OPLS 2005 force field algorithm embedded in the LigPrep module of Schrödinger suite, 2013 (Schrodinger. LLC, New York, NY) was used to optimize the ligand. The original state was retained by the ionizations of the ligand and was further desalted. The optimized protein structure was stored in an .sdf format for further docking studies [Citation40–47].
2.5. Prediction of B-factor and evaluation
The B-factor value was calculated from the newly obtained folded template protein in combination with the human potassium voltage-gated channel (KCNA) protein sequenceprofiles derived from the SwissProt - UniProt database [Citation48–55].The Debye–Waller factor is also known as the B-factor when measuring the flexibility of the protein structure [Citation24]. The B-factor parameters () represent the normalized B-factor of the target KCNA, defined by B = (B′−u)/s, where B′ is the raw B-factor value, u and s are the mean and the standard deviation of the raw B-factors, respectively, along with the target KCNA () [Citation24, Citation56–69].
2.6. Prediction of channel in the protein
EBI’s PORE WALKER 1.0 server (Pellegrini-Calace et al., 2019) (http://www.dev.ebi.ac.uk/thornton-srv/software/PoreWalkerhttp://www.dev.ebi.ac.uk/thornton/srv/software/PoreWalker) which includes a fully-automated method for the detection and characterization of transmembrane protein channels from their 3 D structure was employed to detect the cavity in the protein [Citation46]. The channel is predicted by the identification of the longest and widest cavity across the protein [Citation70–75].
2.5. Molecular docking
Molecular virtual Docker (MVD) is a flexible platform that offers high-quality docking based on high potential pair-wise linear potential (PLP), and the Mol Dock scoring function was utilized for molecular docking. The compound was docked at the predicted active site of the protein [Citation76–87]. The cavity bearing the highest volume was selected for the docking of the compound [Citation88–95]. The docking parameters were set to 0.20 Å as grid resolution maximum iteration of 1500 and maximum population size of 50. For optimizing the complex energy of ligand–protein interaction, the Nelder Mead Simplex Minimization (using non-grid force field and H-bond directionality) was used after the docking [Citation96–105]. The parameter of the Simplex evolution was set at maximum steps of 300 with a neighbourhood distance factor of 1 [Citation106–115]. Binding affinity and interactions of the compound with protein were evaluated based on the internal hydrogen bond interactions, the internal electrostatic interaction, and sp2–sp2 torsions [Citation116–125]. The efficient interacting compound was selected based on the re-rank score from the dataset, which determines the most promising docking result [Citation113, Citation126–135].
2.6. ADMET
The free interface of the admetSAR database (http://lmmd.ecust. edu.cn:8000) was used to calculate ADMET properties of a compound to deal with its absorption, distribution, metabolism, excretion, and toxicity in the human body which play an essential role in the discovery of a compound [Citation133, Citation136–143]. The admetSAR database is based on the 5 quantitative regressions and 22 qualitative classification models which provide a more precise result and were essential in evaluating pharmacodynamics activities [Citation144–153].
2.7. Molecular dynamics simulation
The molecular dynamic stimulation is for entailing time-dependent molecular motion of the biological macromolecules. It has replaced the single-point model with the complex dynamic model to explore the conformational space of the compound [Citation154–162]. The simulation was run for 100 ns timestep in Desmond module of Schrodinger Suite wields the OPLS5 force field for molecular modeling. The geometry and thermodynamic property of the solution were fluctuated during the simulation period and the structural and the functional details of the molecule was obtained; which was studied with simulation diagram, wherein, RMSD, RMSF, protein–ligand interaction and ligand properties were analyzed [Citation163–168].
2.8. Computing details
Crystallographic data collection: SMART [Citation169]; cell refinement: SAINT [Citation169]; data reduction: SAINT [Citation169]; program(s) used to solve structure: SHELXS97 [Citation170]; program(s) used to refine structure: SHELXL97 [Citation171]; molecular graphics: SHELXTL [Citation171] and Mercury [Citation172]; software used to prepare material for publication: SHELXL97 [Citation171] and PLATON [Citation173].
3. Results and discussion
3.1. Analysis of spectral data
Novel olanzapinium 2,5-dihydroxybenzoate:
3.2. Structural commentary
The ORTEP diagram of Olanzapinium 2,5-dihydroxybenzoate with thermal displacement ellipsoids are drawn at the 30% probability is shown in bond length, bond angles of atoms are given in and , respectively. The bond lengths of C14–N4, C15-N4 and C17-N4 are 1.484 (4), 1.484 (4) and 1.483 (4) Å, respectively. These distances are longer than the bond length of a C18–O1 (1.251 (4) Å) and the system formed by the O–H (ionic) bond and the aromatic systems ( and ). The bond angle of acetate group (O1–C18 = O2) and C20–C19–C18 are 123.9° and 121.3°, respectively ().
Table 1. Bond length (Å) of Olanzapinium 2,5-dihydroxybenzoate.
Table 2. Bond angles (in degree) of Olanzapinium 2,5-dihydroxybenzoate.
Naturally, the number of OH groups capable of binding the drug would define the amount of the drug that can be carried by the matrix or the carrier agent. Many investigations indicated that the presence of at least one aryl group, one or two-electron donor atoms, and/or an NH group in a special spatial arrangement is necessary for anticonvulsant activity. Currently clinically used mostly anticonvulsant agents possess cyclic amide or acyclic amide or hetero atom present in the ring. According to them, the most common structural elements of clinically active drugs against epilepsy appeared to be a nitrogen hetero atomic system. The new compound possesses two Hetro atom –NH functional groups present in the ring lead to improve anticonvulsant activity. In this study, it is the NH group and C =O group, which define both the binding and diffusion of the drug (Olanzapine) to the attached molecule (2,5-dihydroxybenzoic acid). The solid-state 13C NMR study allowed us to determine the drug (Olanzapine) which is attached to the supporting molecule (2,5-dihydroxybenzoic acid).
3.3. Refinement
Crystal data collection and structure refinement details are presented in Supplementary Table S1. The hydroxy-H atom was located in a different Fourier map and freely refined. The C-bound H atoms were positioned geometrically and allowed to ride on their parent atoms: C–H = 0.93–0.96 Å with Uiso (H) = 1.5 Ueq (C-methyl) and 1.2 Ueq (C) for other H atoms. Molecular graphics were done using DIAMOND program [Citation174]. Olanzapinium 2,5-dihydroxybenzoate was crystallized with centro symmetric space group P2(1)/n in the monoclinic system. The unit cell parameters of the compound are: a = 8.4867(6) Å, b = 29.764(2) Å, c = 10.6334(8) Å, α = 90°, β = 94.3810° (10), γ = 90°.
3.4. Hirshfeld surface (HS) analysis
The Hirshfeld surface analysis [Citation175–177] was performed to understand the intermolecular interactions in the crystal structure of the compound and was constructed in the crystal environment using CrystalExplorer 17.5 [Citation178]. The various non-covalent interactions were quantified with decomposed, two-dimensional fingerprint plots [Citation178].
The Hirshfeld surface plotted over dnorm is shown in with red areas indicating distances shorter (in closer contact) and blue those longer (distant contact) than the van der Waals radii. The contacts with distances equal to the sum of van der Waals radii are indicated in white [Citation178]. From , the bright red spots appearing near the hydrogen atoms H2N, H4N, H10 and H13 in the cation indicate that these hydrogen atoms are involved in the intermolecular interactions. The shape-index (SI) diagram, a tool to visualize π-π stacking interactions, for the cation, anion, and the solvent molecule is shown in . The overall two-dimensional fingerprint (2 D–FP) plots are illustrated in . The H…H contacts make the highest contribution (53.8%) to the total crystal packing (broad peaks at de+ di =∼2.3 Å). The second highest contribution is from H⋯C/C⋯Hcontacts (25.7%) and is indicated by the broad wing-like structure at de+di = ∼2.1 Å. The symmetrical sharp spikes at de+di= ∼1.6 Å are attributed to H⋯O/O⋯H contacts (14.3%).
3.5. Protein preparation
KCNA gene is a comparably conserved region; however, some substitutions in its amino acids have resulted in significant antipsychotic activity, indicating that KCNA docks against antipsychotic compound Olanzapinium 2,5-dihydroxybenzoate. A comprehensive set of the amino acid sequence and atomic coordinates were analyzed from the obtained 3 D structure of the KCNA (PDB ID: 4BGC) [Citation179] using EMBOSS. All proteinogenic amino acids possess common structural features directly proportional to the biochemical properties of the model, which contain 117 aliphatic residues, 66 aromatic residues, 224 polar amino acids, 271 non-polar residues, 65 acidic amino acids, 65 negative residues and 47 positive residues. The X-ray diffraction data of the protein was visualized with RasMol software, which suggests the target protein has two chains: chain A and chain B with 101 groups and total of 913 atoms. There are 952 absolute bonds with four alpha-helices in red colour and four beta-strands in green colour; however, there is no mention of turns or loops in data. The ribbon backbone model of potassium voltage-gated channel obtained from the protein database bank is depicted in . These predicted values of the KCNA are proved to be useful in the analysis of protein packing, protein recognition, and ligand design.
3.6. Protein channel detection
Pore Walker detected the transmembrane protein channels and characterised them from the 3D structure of KCNA protein. It represents that the funnel prediction shape of the protein is DUD (Hourglass–Diamond-Complex). In , the red spheres represent pore centres at 3Å steps, and their radii are directly proportional to the corresponding measured diameters. In contrast, grey and blue areas show pore-lining atoms and corresponding residues, respectively. The remaining portions of the proteins are shown in green. The number of found lines is 4, the points in straight areas were 54.55, and the total RMSD value is 2.33. Hence, the cavity predicted was close to that of the potassium channel protein structure available in PDB (), therefore, we proceeded for the docking analysis to estimate the affinity of the compound against the channel. Evident from the re-rank score, the compound shows appreciably good affinity against the channel.
3.6. Molecular docking results
The molecular docking of the compound was performed using Molegro Virtual Docker to estimate the affinity of the compound Olanzapinium 2,5-dihydroxybenzoate against the KCNA. Evident from re-rank score ranging between −83.2566 KJ/mol and −60.6982 KJ/mol of the compound in different poses shows appreciably good affinity against the KCNA. The complete affinity analysis with different interacting descriptors is shown in .
Table 3. Molecular docking results.
3.7. Molecular dynamics simulation
The MD simulation provided details of conformational changes in protein and ligand over the trajectory of 100 ns. The simulation diagram is for structural analysis by RMSD and RMSF, ligand properties, and protein–ligand interaction. Based on the structural assessment, the functionality of ligand molecules could be anticipated. The RMSD (root mean square deviation) graph suggests structural activity of protein–ligand interaction; lower the RMSD confers greater stability of the interface. Whilst RMSF (root mean square fluctuation) graph refers to the mobility of proteins Cα residue; a greater number of peaks stipulates more flexibility in proteins backbone.
The inter-residue interaction of olanzapinium 2,5-dihydroxybenzoate against the KCNA is in . The RMSD value for protein ranged from 0.9 to 2.4Å with recurrent deviation. The graph was in equilibrium amid 20–50 ns followed by trough around 60 ns. Again, the protein graph was stable for few nanoseconds, next ascend to maximum 2.4Å then decreased further till 100 ns (). The ligand graph for this complex span between 3 and 45 Å, it was stable for first 20 ns with scarce variation followed by gradual increase to 20 Å for next 10 ns and sudden spike around 30 ns. Thereafter, it was stable from 40 to 100 ns with mini-fluctuation. The sudden spike around 30 ns indicates toward flexible atom in ligand around which the configuration of the ligand can change. The RMSF graph remained less than 2 Å throughout the simulation with few blunt peaks around 20 and 40 ns (II).
3.8. Protein–ligand interaction
Protein–ligand interactions elucidate the stability of the interaction by taking into account hydrogen bonds, hydrophobic bonds, ionic interactions, and water bridges analyzed with histogram and heatmap in . ARG_53, VAL_55, PRO_81, ARG_84, PHE_88, ASN_93, PHE_151 and GLU_140 residues were predominantly interacting with the ligand. Hydrogen bond is formed between hydrogen atom of ligand and strongly electro-negatively charged group of reside atom, it plays crucial role in ligand binding and drug specificity.VAL_55, ASN_93, and GLU_140 residues are bounded by hydrogen bond ().The hydrophobic bonds are π-π, π-cation and other non-specific interaction involving hydrophobic amino acid and aromatic or aliphatic group of the ligand. The inter-residue interaction come across as more hydrophobic, as most of residues are hydrophobically interacting with ligand of which PHE_88, PRO_81, PRO_90, and PHE_151 are predominant (). Also, there are ionic (polar) interaction by GLU_140 residue, formed by attraction forces other than hydrogen bonds. In detailed structural study of ligand is in pharmacophore mapping. Water bridges are water mediated hydrogen bonds. Majority of residues are forming water bridges: ARG_53, ARG_84, ASN_93, GLU_149, and PHE_151. The timeline of residue contacts with ligand is in . The top panel illustrate number of specific contacts the target protein makes with ligand in each and every trajectory frame, it varies from zero to nine. First 20 ns there are more interactions and equilibrium fluctuate between 3 and 6, afterwards the contact ratio declines and equilibrium shifts to 0–3 for further timestep. The bottom panel affirm contribution of each amino acid residue interaction in each trajectory. The number of contacts is represented with orange shade; darker the shade means a greater number of contacts and lighter shade indicates lesser contacts. GLU_140, ASN_93, ARG_84 residues show deeper shade of orange asserting they have more than one type of interaction.
3.9. Ligand property
The ligand property of the olanzapinium 2,5-dihydroxybenzoate against the KCNA, includes parameters: ligand RMSD, radius of gyration (rGyr), polar surface area (PSA), molecular surface area (MolSA), and solvent surface area (SAS) (). The RMSD value is around 1–3 Å with scarce fluctuation over span of 100 ns and equilibrium at 2 Å. The rGyr graph signifies the moment of inertia of the ligand and distribution of mass, it ranged from 5 to 6 Å and was stable around 20–35 ns with few peaks then gradually coming to equilibrium towards 5.50 Å. The molecular surface area is calculated with probe radius 1.4 Å and indicates the Van Der Waal surface area, it ranged between 456 and 472 Å2 with minimal fluctuations and sharp trough near 20 ns, the equilibrium is around 464 Å2. The solvent surface area signifies area accessible by a water molecule. It ranges from 300 to 800 Å2, for 20 ns it was between 300 and 450 Å2, followed by sudden spike where it reaches more than 750 Å2 then gradually descend to 600Å2 for further time lapse, the equilibrium is around 600Å2. The polar surface area is surface accessible for binding of polar residues. The PSA of ligand is constant throughout the simulation with recurrent fluctuations, the equilibrium is at 105Å2. The overall ligand property shows stability of the KCNA-Olanzapinium 2,5-dihydroxybenzoatecomplex.
3.10. Pharmacophore studies
The docking profile of the compound energy values of descriptors of external ligand interactions contributes to higher stability than internal ligand interactions. Moreover, the external ligand interactions were stabilized mostly by stearic energy guided by PLP while in internal ligand interactions; the torsional strain contributes to the stability of the ligand–receptor interactions. We speculate the 3 H-bond interactions of the compound Olanzapinium 2,5-dihydroxybenzoate which are present between MET_51, ASN_93 and VAL_55 in KCNA as shown in .
Figure 12. The most effective pose of compound Olanzapinium 2,5-dihydroxybenzoate with lowest re-rank score shows hydrogen bond interaction with a channel protein KCNA 1 (Residues in colour cyan, ligand in colour pink and hydrogen bond in black dots.)
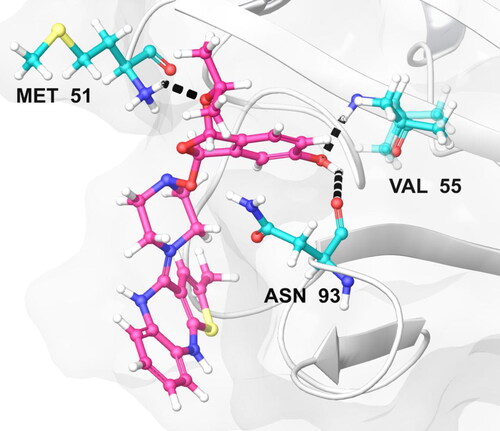
Electrostatic interactions of the compound Olanzapinium 2,5-dihydroxybenzoate within the channel shown in general, the Potassium voltage-gated channel subfamily A member 1 protein there exhibits a dominant in electronegativity. But when the compound is introduced, the electro positivity it also enhances, thus creating a neutral tendency to facilitate the activity of the compound. Arginine is one of the dominant residues in the region of potassium voltage-gated channel. The electrostatic interaction manifests that clusters of charged and polar residues that are detected on protein–protein interfaces may intensify complex stability, although the total effect of electrostatics is generally net destabilizing. The compound Olanzapinium 2,5-dihydroxybenzoate is embedded in the protein cavity and were sheltered more towards the highly electronegative residues which are shown in red shades. The compound possesses less toxic, low melting point, average molecular weight and electronegative nature to facilitate oxygen enrichment, as self-supporting transporter through transvascular route (TVR) at blood–brain barrier (BBB) and blood cerebral spinal fluid barrier (BCSFB) that shuttle compound toward and away from neural tissue [Citation94].
Figure 13. Electrostatic interaction of compound Olanzapinium 2,5-dihydroxybenzoate with the channel protein KVNA 1.
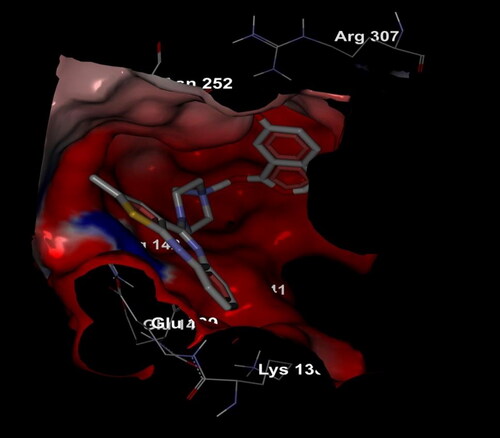
The van der Waals and some covalent interaction of the Olanzapinium 2,5-dihydroxybenzoate is illustrated in . This also includes electrostatic forces, water bridges and ionic bonds. As predicted from MD simulation the ARG_53, VAL_55, MET_51, ARG_84, PHE_88, ASN_93, TYR_95 and GLU_94 residues strongly interact with the compound. The residues encircled in pink are polar in nature forming electrostatic bonds. The residues encircled in green are making non-covalent interaction, i.e., Van der Waals interaction hence loosely bonded. The green arrow suggests TYR_95, VAL_55 and MET_51 residues are forming hydrogen bond by accepting with side chain atom, among these, TYR_95 is form strong bonding by backbone acceptor of methyl atom. The orange line evinces π-π interaction of the residue with aromatic ring. PHE_88, TYR_95 and ARG_84 are interacting by π-πbond while MET_51 is forming π-cation interaction. The blue shadowed atoms are exposure of the ligand. It could be said that TYR_95 and ARG_84 residuesare strongly interacting with the Olanzapinium 2,5-dihydroxybenzoate. This concurs with the simulation histogram of protein–ligand interaction.
3.10. ADMET studies
The compound Olanzapinium 2,5-dihydroxybenzoate further tested for their in silico ADMET profile and solubility properties (). We anticipate that the compound had appreciable ADMET profile, in addition, was neither carcinogenic nor mutagenic. The optimal probabilistic values of ADMET models, in addition, show the compound to have drug-like properties. As shown in and , the predicted bioactivity score of the compound is highest against Potassium channel modulator target, showing compound to have a high and targeted affinity for potassium voltage-gated channel. The targeted activity we assume is important, as needless activation of the targets may raise serious path physiological concerns. In the next approaches, we tested for the compound for its solubility and bioavailability. The compound showed excellent soluble properties along with high oral bioavailability.
Table 4. Predicted ADMET properties of the compound by AdmetSAR program.
Table 5. ADMET predicted profile – regression.
4. Conclusions
In the study conducted, the antipsychotic drug olanzapine was synthesized and crystallized with aromatic acid 2,5-dihydroxybenzoic in isopropyl alcohol that led to the formation of novel Olanzapinium 2,5-dihydroxybenzoate. From the profile, it is evident that the external ligand’s contribution is predominant than the internal which in turn stabilizes the compound’s integrity. The simulation trajectory validates the conformational stability of the compound. Further, when the new compound is introduced in the KCNA environment, it completely neutralizes the environment to facilitate the activity of the compound viz., KCNA is highly electronegative where as our compound is highly electropositive. Besides, protein–ligand interaction contemplated the hydrophobic nature of the compound. The predicted ADMET properties completely support the affinity of the compound through the KCNA channel, viz., the predominance of arginine and tyrosine residue in all directions. The bio activity score is also very high. In conclusion, together with molecular docking analysis, bioavailability analysis, and ADMET predictions, we foresee that this new compound Olanzapinium 2,5-dihydroxybenzoate may serve as a highly potent and active antipsychotic drug.
Author contributions
All authors have been personally and actively involved in substantive work leading to the manuscript, and will hold themselves jointly and individually responsible for its content.
Compliance with ethical standards
Ethical approval
Ethical approval and informed consent not required for this manuscript
Acknowledgements
This work was supported by Taif University Researchers Supporting Program (project number: TURSP-2020/128), Taif University, Saudi Arabia. The authors are grateful to the Deanship of Scientific Research, King Saud University for funding through Vice Deanship of Scientific Research Chairs.
Disclosure statement
The authors declare that they have no conflict of interest.
References
- Adams CE, Fenton MK, Quraishi S, et al. Systematic meta-review of depot antipsychotic drugs for people with schizophrenia. Br J Psychiatry. 2001; 179:290–299.
- Chakrabarti JK, Hotten TM, Tupper DE. 2-Methyl-10-(4-methyl-1-piperazinyl)-4H-thieno-[2,3-b][1,5]benzodiazepine US Patent No. 5229382 A, 1993.
- Amidon GL, Lennernas H, Shah VP, et al. A theoretical basis for a biopharmaceutic drug classification: the correlation of in vitro drug product dissolution and in vivo bioavailability. Pharm Res. 1995;12(3):413–420.
- Smith RC, Infante M, Singh A, et al. The effects of olanzapine on neurocognitive functioning in medication-refractory schizophrenia. Int J Neuropsychopharmacol. 2001; 4(3):239–250.
- Moore NA, Tye NC, Axton MS, et al. The behavioral pharmacology of olanzapine, a novel “atypical” antipsychotic agent. J Pharmacol Exp Ther. 1992; 262(2):545–551.
- Moore NA, Calligaro DO, Wong DT, et al. The pharmacology of olanzapine and other new antipsychotic agents. Curr Opin Invest Drugs. 1993; 2:281–293.
- Tamminga CA. Partial dopamine agonists in the treatment of psychosis. J Neural Transm (Vienna)). 2002; 109(3):411–420.
- Dargham A, Laruelle M. Mechanisms of action of second generation antipsychotic drugs in schizophrenia: insights from brain imaging studies. Eur Psychiatry. 2005;20(1):15–27.
- Seeman P, Lee T, Chau-Wong M, et al. Antipsychotic drug doses and neuroleptic/dopamine receptors. Nature. 1976;261(5562):717–719.
- Breden EL, Liu MT, Dean SR, et al. Metabolic and cardiacs side effects of second- generation antipsychotics: what every clinician should known. J Res Pharmacy Pract. 2009;22(5):478–488.
- Jones B, Basson BR, Walker DJ, et al. Weight change and atypical antipsychotic treatment in patients with schizophrenia. J Clin Psychiatry. 2001; 62:41–44.
- Gardner DM, Baldessarini RJ, Waraich P. Modern antipsychotic drugs: a critical overview. CMAJ. 2005;172(13):1703–1711.
- Mathews M, Muzina JD. Atypical antipsychotics: new drugs, new challenges. Cleve Clin J Med. 2007;74(8):597–606.
- Gümüş M, Babacan ŞN, Demir Y, et al. Discovery of sulfadrug–pyrrole conjugates as carbonic anhydrase and acetylcholinesterase inhibitors. Arch Pharm. 2022;355(1):2100242.
- Bruker SAINT (version 6.28a) & SMART (version 5.625). Madison, Wisconsin: Bruker AXS Inc, 2001.
- Sharma K, Patidar K, Ali MA, et al. Structure-based virtual screening for the identification of high affinity compounds as potent VEGFR2 inhibitors for the treatment of renal cell carcinoma. Curr Top Med Chem. 2018;18(25):2174–2185.
- Sahila MM, Babitha PP, Bandaru S, et al. Molecular docking based screening of GABA (A) receptor inhibitors from plant derivatives. Bioinformation. 2015;11(6):280–289.
- Vuree S, Dunna NR, Khan IA, et al. Pharmacogenomics of drug resistance in breast cancer resistance protein (BCRP) and its mutated variants. J Pharm Res. 2013;6(7):791–798.
- Monteiro AFM, Viana JDO, Nayarisseri A, et al. Computational studies applied to flavonoids against Alzheimer’s and Parkinson’s diseases. Oxid Med Cell Longev. 2018;2018:7912765.
- Bandaru S, Sumithnath TG, Sharda S, et al. Helix-coil transition signatures B-Raf V600E mutation and virtual screening for inhibitors directed against mutant B-Raf. Curr Drug Metab. 2017;18(6):527–534.
- Kelotra A, Gokhale SM, Kelotra S, et al. Alkyloxy carbonyl modified hexapeptides as a high affinity compounds for Wnt5A protein in the treatment of psoriasis. Bioinformation. 2014;10(12):743–749.
- Basak SC, Nayarisseri A, González-Díaz H, et al. Editorial (thematic issue: chemoinformatics models for pharmaceutical design, part 1). Curr Pharm Des. 2016;22(33):5041–5042.
- Basak SC, Nayarisseri A, González-Díaz H, et al. Editorial (thematic issue: chemoinformatics models for pharmaceutical design. Curr Pharm Des. 2016;22(34):5177–5178.
- Prajapati L, Khandelwal R, Yogalakshmi KN, et al. Computer-aided structure prediction of bluetongue virus coat protein VP2 assisted by optimized potential for liquid simulations (OPLS). Curr Top Med Chem. 2020;20(19):1720–1732.
- Nayarisseri A, Khandelwal R, Madhavi M, et al. Shape-based machine learning models for the potential novel COVID-19 protease inhibitors assisted by molecular dynamics simulation. Curr Top Med Chem. 2020;20(24):2146–2167.
- Nayarisseri A. Most promising compounds for treating COVID-19 and recent trends in antimicrobial & antifungal agents. Curr Top Med Chem. 2020;20(24):2119–2125.
- Pochetti G, Mitro N, Lavecchia A, et al. Structural insight into peroxisome proliferator-activated receptor gamma binding of two ureidofibrate-like enantiomers by molecular dynamics, cofactor interaction analysis, and site-directed mutagenesis . J Med Chem. 2010;53(11):4354–4366.
- Soares Rodrigues GC, dos Santos Maia M, Muratov EN, et al. Quantitative structure–activity relationship modeling and docking of monoterpenes with insecticidal activity against reticulitermes chinensis snyder and Drosophila melanogaster. J Agric Food Chem. 2020;68(16):4687–4698.
- Wang Y, Wang LF, Zhang LL, et al. Molecular mechanism of inhibitor bindings to bromodomain-containing protein 9 explored based on molecular dynamics simulations and calculations of binding free energies. SAR QSAR Environ Res. 2020;31(2):149–170.
- Marunnan SM, Pulikkal BP, Jabamalairaj A, et al. Development of MLR and SVM aided QSAR models to identify common SAR of GABA uptake herbal inhibitors used in the treatment of schizophrenia. Curr Neuropharmacol. 2017;15(8):1085–1092.
- Sweta J, Khandelwal R, Srinitha S, et al. Identification of high-affinity small molecule targeting IDH2 for the clinical treatment of acute myeloid leukemia. Asian Pac J Cancer Prev. 2019;20(8):2287–2297.
- Nayarisseri A. Prospects of utilizing computational techniques for the treatment of human diseases. Curr Top Med Chem. 2019;19(13):1071–1074.
- Schrodinger, LLC, NY, USA, 2009.
- LigPrep, Schrodinger LLC, New York, NY, 2017.
- Prime, Schrodinger, LLC, Ney York, NY, 2019.
- Protein Preparation Wizard, Schrodinger, LLC, Ney York, NY, 2017.
- Qikprop, Schrodinger, LLC, Ney York, NY, 2011.
- Wang LF, Wang Y, Yang ZY, et al. Revealing binding selectivity of inhibitors toward bromodomain-containing proteins 2 and 4 using multiple short molecular dynamics simulations and free energy analyses. SAR QSAR Environ Res. 2020;31(5):373–398.
- Brugnoni M, Scotti A, Rudov AA, et al. Swelling of a responsive network within different constraints in multi-thermosensitive microgels. Macromolecules. 2018;51(7):2662–2671.
- Montanari R, Saccoccia F, Scotti E, et al. Crystal structure of the peroxisome proliferator-activated receptor gamma (PPARgamma) ligand binding domain complexed with a novel partial agonist: a new region of the hydrophobic pocket could be exploited for drug design. J Med Chem. 2008;51(24):7768–7776.
- Wang J, Qian Y, Li L, et al. Atomic force microscopy and molecular dynamics simulations for study of lignin solution self-assembly mechanisms in organic-aqueous solvent mixtures. ChemSusChem. 2020;13(17):4420–4427.
- Liguori N, Croce R, Marrink SJ, et al. Molecular dynamics simulations in photosynthesis. Photosynth Res. 2020;144(2):273–295.
- Kuzmanic A, Bowman GR, Juarez-Jimenez J, et al. Investigating cryptic binding sites by molecular dynamics simulations. Acc Chem Res. 2020;53(3):654–661.
- Klesse G, Rao S, Tucker SJ, et al. Induced polarization in molecular dynamics simulations of the 5-HT3 receptor channel. J Am Chem Soc. 2020;142(20):9415–9427.
- Shiau AK, Barstad D, Loria PM, et al. The structural basis of estrogen receptor/coactivator recognition and the antagonism of this interaction by tamoxifen. Cell. 1998;95(7):927–937.
- Berman H, Henrick K, Nakamura H, et al. The worldwide protein data bank (wwPDB): ensuring a single, uniform archive of PDB data. Nucleic Acids Res. 2007;35(Database issue):D301–D303.
- Natchimuthu V, Bandaru S, Nayarisseri A, et al. Design, synthesis and computational evaluation of a novel intermediate salt of N-cyclohexyl-N-(cyclohexylcarbamoyl)-4-(trifluoromethyl) benzamide as potential potassium channel blocker in epileptic paroxysmal seizures. Comput Biol Chem. 2016;64:64–73.
- Bandaru S, Alvala M, Akka J, et al. Identification of small molecule as a high affinity β2 agonist promiscuously targeting wild and mutated (Thr164Ile) β 2 adrenergic receptor in the treatment of bronchial asthma. CPD. 2016;22(34):5221–5233.
- Majhi M, Ali MA, Limaye A, et al. An in silico investigation of potential EGFR inhibitors for the clinical treatment of colorectal cancer. Curr Top Med Chem. 2018;18(27):2355–2366.
- Khandelwal R, Chauhan AP, Bilawat S, et al. Structure-based virtual screening for the identification of high-affinity small molecule towards STAT3 for the clinical treatment of osteosarcoma. Curr Top Med Chem. 2018;18(29):2511–2526.
- Sinha K, Majhi M, Thakur G, et al. Computer-aided drug designing for the identification of high-affinity small molecule targeting cd20 for the clinical treatment of chronic lymphocytic leukemia (CLL). Curr Top Med Chem. 2018;18(29):2527–2542.
- Chandrakar B, Jain A, Roy S, et al. Molecular modeling of Acetyl-CoA carboxylase (ACC) from jatropha curcas and virtual screening for identification of inhibitors. J Pharm Res. 2013;6(9):913–918.
- Nayarisseri A, Moghni SM, Yadav M, et al. In silico investigations on HSP90 and its inhibition for the therapeutic prevention of breast cancer. J Pharm Res. 2013;7(2):150–156.
- Udhwani T, Mukherjee S, Sharma K, et al. Design of PD-L1 inhibitors for lung cancer. Bioinformation. 2019;15(2):139–150.
- Shukla P, Khandelwal R, Sharma D, et al. Virtual screening of IL-6 inhibitors for idiopathic arthritis. Bioinformation. 2019;15(2):121–130.
- Nayarisseri A, Hood EA. Advancement in microbial cheminformatics. Curr Top Med Chem. 2018;18(29):2459–2461.
- Jain D, Udhwani T, Sharma S, et al. Design of novel JAK3 inhibitors towards rheumatoid arthritis using molecular docking analysis. Bioinformation. 2019;15(2):68–78.
- Nayarisseri A, Singh SK. Functional inhibition of VEGF and EGFR suppressors in cancer treatment. CTMC. 2019;19(3):178–179.
- Gokhale P, Chauhan APS, Arora A, et al. FLT3 inhibitor design using molecular docking based virtual screening for acute myeloid leukemia. Bioinformation. 2019;15(2):104–115.
- Ali MA, Vuree S, Goud H, et al. Identification of high-affinity small molecules targeting gamma secretase for the treatment of alzheimer’s disease. CTMC. 2019;19(13):1173–1187.
- Patidar K, Panwar U, Vuree S, et al. An in silico approach to identify high affinity small molecule targeting m-TOR inhibitors for the clinical treatment of breast cancer. Asian Pac J Cancer Prev. 2019;20(4):1229–1241.
- Pandey N, Yadav M, Nayarisseri A, et al. Cross evaluation of different classes of alpha-adrenergic receptor antagonists to identify overlapping pharmacophoric requirements. J Pharm Res. 2013;6(1):173–178.
- Shelley JC, Cholleti A, Frye LL, et al. Epik: a software program for pK a prediction and protonation state generation for drug-like molecules. J Comput Aided Mol Des. 2007;21(12):681–691.
- Baby K, Maity S, Mehta CH, et al. Targeting SARS-CoV-2 main protease: a computational drug repurposing study. Arch Med Res. 2021;52(1):38–47.
- Gahlawat A, Kumar N, Kumar R, et al. Structure-based virtual screening to discover potential lead molecules for the SARS-CoV-2 main protease. J Chem Inf Model. 2020;60(12):5781–5793.
- Shivanika C, Deepak Kumar S, Ragunathan V, et al. Molecular docking, validation, dynamics simulations, and pharmacokinetic prediction of natural compounds against the SARS-CoV-2 main-protease. J Biomol Struct Dyn. 2020.
- Karplus M, McCammon JA. Molecular dynamics simulations of biomolecules. Nat Struct Biol. 2002;9(9):646–652.
- Beura S, &Prabhakar C, In-silico strategies for probing chloroquine based inhibitors against SARS-CoV-2. J Biomol Struct Dyn. 2021;39(10):3747–2597. Xu X, Mao L, Xu W, Tang W, Zhang X, Xi B, … Zhang L. 2016; AC0010, an irreversible EGFR inhibitor selectively targeting mutated EGFR and overcoming T790M-induced resistance in animal models and lung cancer patients. Molecular Cancer Therapeutics.
- Sharda S, Khandelwal R, Adhikary R, et al. A computer-aided drug designing for pharmacological inhibition of mutant ALK for the treatment of non-small cell lung cancer. CTMC. 2019;19(13):1129–1144.
- Limaye A, Sweta J, Madhavi M, et al. In silico insights on GD2: a potential target for pediatric Neuroblastoma. Curr Top Med Chem. 2019;19(30):2766–2781.
- Nayarisseri A, Yadav M. Editorial (thematic issue: mechanistics in drug design-experimental molecular biology vs. molecular modeling). CTMC. 2015;15(1):3–4.
- Kleandrova VV, Scotti MT, Scotti L, et al. Cell-based multi-target QSAR model for design of virtual versatile inhibitors of liver cancer cell lines. SAR QSAR Environ Res. 2020;31(11):815–836.
- Nayarisseri A. Experimental and computational approaches to improve binding affinity in chemical biology and drug discovery. Curr Top Med Chem. 2020;20(19):1651–1660.
- Kaushik AC, Kumar S, Wei DQ, et al. Structure based virtual screening studies to identify novel potential compounds for GPR142 and their relative dynamic analysis for study of type 2 diabetes. Front Chem. 2018;6:23.
- Toledo Warshaviak D, Golan G, Borrelli KW, et al. Structure-based virtual screening approach for discovery of covalently bound ligands. J Chem Inf Model. 2014;54(7):1941–1950.
- Sert Y, Gümüş M, Gökce H, et al. Molecular docking, hirshfeld surface, structural, spectroscopic, electronic, NLO and thermodynamic analyses on novel hybrid compounds containing pyrazole and coumarin cores. J Mol Struct. 2018;1171:850–866.
- Çapan İ, Gümüş M, Gökce H, et al. Synthesis, dielectric properties, molecular docking and ADME studies of pyrrole-3-ones. J Biomol Struct Dyn. 2021;1–17.
- Lyne PD. Structure-based virtual screening: an overview. Drug Discov Today. 2002;7(20):1047–1055.
- Dighe SN, Deora GS, De la Mora E, et al. Discovery and structure-activity relationships of a highly selective butyrylcholinesterase inhibitor by structure-based virtual screening. J Med Chem. 2016;59(16):7683–7689.
- Schrödinger L. 2011. QikProp: rapid ADME predictions of drug candidates.
- Lionta E, Spyrou G, K Vassilatis D, et al. Structure-based virtual screening for drug discovery: principles, applications and recent advances. Curr Top Med Chem. 2014;14(16):1923–1938.
- Vidler LR, Filippakopoulos P, Fedorov O, et al. Discovery of novel small-molecule inhibitors of BRD4 using structure-based virtual screening. J Med Chem. 2013;56(20):8073–8088.
- Pitt WR, Calmiano MD, Kroeplien B, et al. 2013. Structure-based virtual screening for novel ligands. In: Protein-Ligand interactions. Totowa, NJ: Humana Press. p. 501–519.
- Choudhary S, Malik YS, Tomar S. Identification of SARS-CoV-2 cell entry inhibitors by drug repurposing using in silico structure-based virtual screening approach. Front Immunol. 2020;11:1664.
- Adhikary R, Khandelwal R, Hussain T, et al. Structural insights into the molecular design of ROS1 inhibitor for the treatment of non-small cell lung cancer (NSCLC). CAD. 2021;17(3):387–401.
- Aher A, Udhwani T, Khandelwal R, et al. In silico insights on IL-6: a potential target for multicentric castleman disease. Curr Comput Aided Drug Des. 2020;16(5):641–653.
- Qureshi S, Khandelwal R, Madhavi M, et al. A multi-target drug designing for BTK, MMP9, proteasome and TAK1 for the clinical treatment of mantle cell lymphoma. CTMC. 2021;21(9):790–818.
- Yadav M, Khandelwal R, Mudgal U, et al. Identification of potent VEGF inhibitors for the clinical treatment of glioblastoma, a virtual screening approach. Asian Pac J Cancer Prev. 2019;20(9):2681–2692.
- Nayarisseri A, Khandelwal R, Tanwar P, et al. Artificial intelligence, big data and machine learning approaches in precision medicine & drug discovery. Curr Drug Targets. 2021.
- Mendonça-Junior FJB, Scotti MT, Nayarisseri A, et al. Natural bioactive products with antioxidant properties useful in neurodegenerative diseases. Oxid Med Cell Longev. 2019;2019:7151780.
- Celik I, Erol M, Puskullu MO, et al. Evaluation of activity of some 2, 5-disubstituted benzoxazole derivatives against acetylcholinesterase, butyrylcholinesterase and tyrosinase: ADME prediction, DFT and comparative molecular docking studies. Polycyclic Aromat Compd. 2020;1–12.
- Pawar VS, Lokwani DK, Bhandari SV, et al. Design, docking study and ADME prediction of isatin derivatives as anti-HIV agents. Med Chem Res. 2011;20(3):370–380.
- Dincel ED, Gürsoy E, Yilmaz-Ozden T, et al. Antioxidant activity of novel imidazo[2,1-b]thiazole derivatives: design, synthesis, biological evaluation, molecular docking study and in silico ADME prediction. Bioorg Chem. 2020;103:104220.
- Kumar S, Saini V, Maurya IK, et al. Design, synthesis, DFT, docking studies and ADME prediction of some new coumarinyl linked pyrazolylthiazoles: potential standalone or adjuvant antimicrobial agents. PloS One. 2018;13(4):e0196016.
- Erol M, Celik I, Temiz-Arpaci O, et al. Synthesis, molecular docking and ADME prediction of some new benzimidazole carboxamidines derivatives as antimicrobial agents. Med Chem Res. 2020;29(11):2028–2038.
- Kashid AM, Dube PN, Alkutkar PG, et al. Synthesis, biological screening and ADME prediction of benzylindole derivatives as novel anti-HIV-1, anti-fungal and anti-bacterial agents. Med Chem Res. 2013;22(10):4633–4640.
- Kalin TN, Kilic D, Arslan F, et al. Synthesis, molecular modeling studies, ADME prediction of arachidonic acid carbamate derivatives, and evaluation of their acetylcholinesterase activity. Drug Dev Res. 2020;81(2):232–241.
- Kumar A, Rathi E, Kini SG. E-pharmacophore modelling, virtual screening, molecular dynamics simulations and in-silico ADME analysis for identification of potential E6 inhibitors against cervical cancer. J Mol Struct. 2019;1189:299–306.
- Bhatt JD, Chudasama CJ, Patel KD. Pyrazole clubbed triazolo [1, 5-a] pyrimidine hybrids as an anti-tubercular agents: Synthesis, in vitro screening and molecular docking study. Bioorg Med Chem. 2015;23(24):7711–7716.
- Dofe VS, Sarkate AP, Lokwani DK, et al. Novel O‐alkylated chromones as antimicrobial agents: Ultrasound mediated synthesis, molecular docking and ADME prediction. J Heterocyclic Chem. 2017;54(5):2678–2685.
- Malik R, Mehta P, Srivastava S, et al. Pharmacophore modeling, 3D-QSAR, and in silico ADME prediction of N-pyridyl and pyrimidine benzamides as potent antiepileptic agents. J Recept Signal Transduction. 2017;37(3):259–266.
- da Silva Hage-Melim LI, Federico LB, de Oliveira NKS, et al. Virtual screening, ADME/tox predictions and the drug repurposing concept for future use of old drugs against the COVID-19. Life Sci. 2020;256:117963.
- Upadhyay S, Tripathi AC, Paliwal S, et al. 2-Pyrazoline derivatives in neuropharmacology: Synthesis, ADME prediction, molecular docking and in vivo biological evaluation. Excli J. 2017;16:628–649.
- Pandey RK, Narula A, Naskar M, et al. Exploring dual inhibitory role of febrifugine analogues against plasmodium utilizing structure-based virtual screening and molecular dynamic simulation. J Biomol Struct Dyn. 2017;35(4):791–804.
- Sadhasivam A, Vetrivel U. Identification of potential drugs targeting L,L‐diaminopimelate aminotransferase of Chlamydia trachomatis: an integrative pharmacoinformatics approach. J Cell Biochem. 2019;120(2):2271–2288.
- Zakerali T, Shahbazi S. Rational druggability investigation toward selection of lead molecules: impact of the commonly used spices on inflammatory diseases. Assay Drug Dev Technol. 2018;16(7):397–407.
- Suma KB, Kumari A, Shetty D, et al. Structure based pharmacophore modelling approach for the design of azaindole derivatives as DprE1 inhibitors for tuberculosis. J Mol Graph Model. 2020;101:107718.
- Sukumar N, Pask JE. Classical and enriched finite element formulations for bloch‐periodic boundary conditions. Int J Numer Meth Eng. 2009;77(8):1121–1138.
- Rohini K, Ramanathan K, Shanthi V. Multi-dimensional screening strategy for drug repurposing with statistical framework—a new road to influenza drug discovery. Cell Biochem Biophys. 2019;77(4):319–333.
- Halgren TA, Murphy RB, Friesner RA, et al. Glide: a new approach for rapid, accurate docking and scoring. 2. Enrichment factors in database screening. J Med Chem. 2004;47(7):1750–1759.
- Sastry GM, Adzhigirey M, Day T, et al. Protein and ligand preparation: parameters, protocols, and influence on virtual screening enrichments. J Comput Aided Mol Des. 2013;27(3):221–234.
- Lenselink EB, Beuming T, Sherman W, et al. Selecting an optimal number of binding site waters to improve virtual screening enrichments against the adenosine A2A receptor. J Chem Inf Model. 2014;54(6):1737–1746.
- Verma P, Tiwari M, Tiwari V. In silico high-throughput virtual screening and molecular dynamics simulation study to identify inhibitor for AdeABC efflux pump of Acinetobacter baumannii. J Biomol Struct Dyn. 2018;36(5):1182–1194.
- Sherman W, Beard HS, Farid R. Use of an induced fit receptor structure in virtual screening. Chem Biol Drug Des. 2006;67(1):83–84.
- Bhachoo J, Beuming T. Investigating protein–peptide interactions using the schrödinger computational suite. Modeling Peptide-Protein Interactions. 2017;1561:235–254.
- Deb PK, Chandrasekaran B, Mailavaram R, et al. Molecular modeling approaches for the discovery of adenosine A2B receptor antagonists: current status and future perspectives. Drug Discov Today. 2019;24(9):1854–1864.
- Vanajothi R, Hemamalini V, Jeyakanthan J, et al. Ligand-based pharmacophore mapping and virtual screening for identification of potential discoidin domain receptor 1 inhibitors. J Biomol Struct Dyn. 2020;38(9):2800–2808.
- Politi A, Durdagi S, Moutevelis-Minakakis P, et al. Development of accurate binding affinity predictions of novel renin inhibitors through molecular docking studies. J Mol Graph Model. 2010;29(3):425–435.
- Liu K, Kokubo H. Exploring the stability of ligand binding modes to proteins by molecular dynamics simulations: a cross-docking study. J Chem Inf Model. 2017;57(10):2514–2522.
- Munnaluri R, Sivan SK, Manga V. Molecular docking and MM/GBSA integrated protocol for designing small molecule inhibitors against HIV-1 gp41. Med Chem Res. 2015;24(2):829–841.
- Lyne PD, Lamb ML, Saeh JC. Accurate prediction of the relative potencies of members of a series of kinase inhibitors using molecular docking and MM-GBSA scoring. J Med Chem. 2006;49(16):4805–4808.
- Peddi SR, Sivan SK, Manga V. Molecular dynamics and MM/GBSA-integrated protocol probing the correlation between biological activities and binding free energies of HIV-1 TAR RNA inhibitors. J Biomol Struct Dyn. 2018;36(2):486–503.
- Cheng F, Li W, Zhou Y, et al. admetSAR: a comprehensive source and free tool for evaluating chemical ADMET properties. J Chem Inf Model. 2012; 52(11):3099–3105.
- Shen M, Zhou S, Li Y, et al. Discovery and optimization of triazine derivatives as ROCK1 inhibitors: molecular docking, molecular dynamics simulations and free energy calculations. Mol Biosyst. 2013;9(3):361–374.
- Bathini R, Sivan SK, Fatima S, et al. Molecular docking, MM/GBSA and 3D-QSAR studies on EGFR inhibitors. J Chem Sci. 2016;128(7):1163–1173.
- Pandey RK, Kumbhar BV, Sundar S, et al. Structure-based virtual screening, molecular docking, ADMET and molecular simulations to develop benzoxaborole analogs as potential inhibitor against Leishmania donovani trypanothione reductase. J Recept Signal Transduct Res. 2017;37(1):60–70.
- Lagarias P, Barkan K, Tzortzini E, et al. Insights to the binding of a selective adenosine A3 receptor antagonist using molecular dynamic simulations, MM-PBSA and MM-GBSA free energy calculations, and mutagenesis. J Chem Inf Model. 2019;59(12):5183–5197.
- Tang X, Wang Z, Lei T, et al. Importance of protein flexibility on molecular recognition: modeling binding mechanisms of aminopyrazine inhibitors to Nek2. Phys Chem Chem Phys. 2018;20(8):5591–5605.
- Negron C, Pearlman DA, Del Angel G. Predicting mutations deleterious to function in beta-lactamase TEM1 using MM-GBSA. Plos One. 2019;14(3):e0214015.
- Paissoni C, Spiliotopoulos D, Musco G, et al. GMXPBSA 2.1: a GROMACS tool to perform MM/PBSA and computational alanine scanning. Comput Phys Commun. 2015;186:105–107.
- Salas-Burgos A, Iserovich P, Zuniga F, et al. Predicting the three-dimensional structure of the human facilitative glucose transporter glut1 by a novel evolutionary homology strategy: insights on the molecular mechanism of substrate migration, and binding sites for glucose and inhibitory molecules. Biophys J. 2004;87(5):2990–2999.
- Shukla R, Chetri PB, Sonkar A, et al. Identification of novel natural inhibitors of opisthorchis felineus cytochrome P450 using structure-based screening and molecular dynamic simulation. J Biomol Struct Dyn. 2018;36(13):3541–3556.
- Thirumal KD, Mendonca E, Priyadharshini CJ, et al. A computational model to predict the structural and functional consequences of missense mutations in O6-methylguanine DNA methyltransferase. Adv Protein Chem Struct Biol. 2019;115:351–369.
- Shukla R, Shukla H, Kalita P, et al. Structural insights into natural compounds as inhibitors of Fasciola gigantica thioredoxin glutathione reductase. J Cell Biochem. 2018;119(4):3067–3080.
- Kubarenko A, Frank M, Weber ANR. Structure–function relationships of toll-like receptor domains through homology modelling and molecular dynamics. Biochem Soc Trans. 2007;35(Pt 6):1515–1518.
- Baul HS, Rajiniraja M. Mechanistic study of the inhibition of monoamine oxidase-B by quercetin as the potential therapeutic strategy for Parkinson’s disease: an in silico approach. JCM. 2018;18(4):1067–1073.
- Di Prinzio CL, Pereyra RG. Molecular dynamics simulations of tilt grain boundaries in ice. Modelling Simul Mater Sci Eng. 2016;24(4):045015.
- Shah M, Anwar MA, Yesudhas D, et al. A structural insight into the negative effects of opioids in analgesia by modulating the TLR4 signaling: an in silico approach. Sci Rep. 2016;6(1):1–15.
- Tian J, Wang P, Gao S, et al. Enhanced thermostability of methyl parathion hydrolase from ochrobactrum sp. M231 by rational engineering of a glycine to proline mutation. Febs J. 2010;277(23):4901–4908.
- Joshi T, Sharma P, Joshi T, et al. In silico screening of anti-inflammatory compounds from lichen by targeting cyclooxygenase-2. J Biomol Struct Dyn. 2020;38(12):3544–3562.
- Torktaz I, Najafi A, Golmohamadi R, et al. Molecular dynamics simulation (MDS) analysis of Vibrio cholerae ToxT virulence factor complexed with docked potential inhibitors. Bioinformation. 2018;14(3):101–105.
- Raftopoulou S, Nicolaides NC, Papageorgiou L, et al. 2020. Structural study of the DNA: Clock/Bmal1 complex provides insights for the role of cortisol, hGR, and HPA axis in stress management and sleep disorders. In: GeNeDis 2018. Cham: Springer. p. 59–71.
- Muthuvel SK, Elumalai E, K G, et al. Molecular docking and dynamics studies of 4-anilino quinazolines for epidermal growth factor receptor tyrosine kinase to find potent inhibitor. J Recept Signal Transduct Res. 2018;38(5-6):475–483.
- Gajendrarao P, Krishnamoorthy N, Sakkiah S, et al. Molecular modeling study on orphan human protein CYP4A22 for identification of potential ligand binding site. J Mol Graphics Modell. 2010;28(6):524–532.
- Tanwar H, Kumar DT, Doss CGP, et al. Bioinformatics classification of mutations in patients with mucopolysaccharidosis IIIA. Metab Brain Dis. 2019;34(6):1577–1594.
- Kumari R, Kumar R, Lynn A. Open source drug discovery consortium g_mmpbsa-a GROMACS tool for high-throughput MM-PBSA calculations. J Chem Inf Model. 2014;54(7):1951–1962,
- Paissoni C, Spiliotopoulos D, Musco G, et al. GMXPBSA 2.0: a GROMACS tool to perform MM/PBSA and computational alanine scanning. Comput Phys Commun. 2014;185(11):2920–2929.
- Wang E, Sun H, Wang J, et al. End-point binding free energy calculation with MM/PBSA and MM/GBSA: strategies and applications in drug design. Chem Rev. 2019;119(16):9478–9508.
- Kumar A, Srivastava G, Negi AS, et al. Docking, molecular dynamics, binding energy-MM-PBSA studies of naphthofuran derivatives to identify potential dual inhibitors against BACE-1 and GSK-3β. J Biomol Struct Dyn. 2019;37(2):275–290.
- Botelho FD, Dos Santos MC, Gonçalves ADS, et al. Ligand-based virtual screening, molecular docking, molecular dynamics, and MM-PBSA calculations towards the identification of potential novel ricin inhibitors. Toxins. 2020;12(12):746.
- Elkarhat Z, Charoute H, Elkhattabi L, et al. Potential inhibitors of SARS-cov-2 RNA dependent RNA polymerase protein: molecular docking, molecular dynamics simulations and MM-PBSA analyses. J Biomol Struct Dyn. 2020;40:361–374.
- Martins LC, Torres PHM, de Oliveira RB, et al. Investigation of the binding mode of a novel cruzain inhibitor by docking, molecular dynamics, ab initio and MM/PBSA calculations. J Comput Aided Mol Des. 2018;32(5):591–605.
- Kumari R, Lynn A. Application of MM/PBSA in the prediction of relative binding free energy: Re-scoring of docking hit-list. J Nat Sci, Biol Med. 2011;2(3):92–92.
- Shenai PM, Xu Z, Zhao Y. Applications of principal component analysis (PCA) in materials science. Principal Component Analysis–Engineering Application. 2012. p. 25–40.
- Chen J, Wang J, Zhu W. Molecular mechanism and energy basis of conformational diversity of antibody SPE7 revealed by molecular dynamics simulation and principal component analysis. Sci Rep. 2016;6(1):36900–36912.
- Sittel F, Filk T, Stock G. Principal component analysis on a torus: theory and application to protein dynamics. J Chem Phys. 2017;147(24):244101.
- Riccardi L, Nguyen PH, Stock G. Free-energy landscape of RNA hairpins constructed via dihedral angle principal component analysis. J Phys Chem B. 2009;113(52):16660–16668.
- Buslaev P, Mustafin K, Gushchin I. Principal component analysis highlights the influence of temperature, curvature and cholesterol on conformational dynamics of lipids. Biochim Biophys Acta Biomembr. 2020;1862(7):183253.
- Nguyen PH. Complexity of free energy landscapes of peptides revealed by nonlinear principal component analysis. Proteins Struct Funct Bioinf. 2006;65(4):898–913.
- Yamamoto N. Hot spot of structural ambivalence in prion protein revealed by secondary structure principal component analysis. J Phys Chem B. 2014;118(33):9826–9833.
- Michielssens S, van Erp TS, Kutzner C, et al. Molecular dynamics in principal component space. J Phys Chem B. 2012;116(29):8350–8354.
- Grasso G, Deriu MA, Tuszynski JA, et al. Conformational fluctuations of the AXH monomer of ataxin‐1. Proteins. 2016;84(1):52–59.
- Verma S, Singh A, Kumari A, et al. Natural polyphenolic inhibitors against the antiapoptotic BCL-2. J Recept Signal Transduct Res. 2017;37(4):391–400.
- Mouritsen OG, Khandelia H. Molecular mechanism of the allosteric enhancement of the umami taste sensation. Febs J. 2012;279(17):3112–3120.
- Swain SS, Paidesetty SK, Dehury B, et al. Molecular docking and simulation study for synthesis of alternative dapsone derivative as a newer antileprosy drug in multidrug therapy. J Cell Biochem. 2018;119(12):9838–9852.
- Villa A, Stock G. What NMR relaxation can tell us about the internal motion of an RNA hairpin: a molecular dynamics simulation study. J Chem Theory Comput. 2006;2(5):1228–1236.
- Ng HW, Laughton CA, Doughty SW. Molecular dynamics simulations of the adenosine A2a receptor: structural stability, sampling, and convergence. J Chem Inf Model. 2013;53(5):1168–1178.
- Bruker S. SAINT and SADABS. Madison, Wisconsin: Bruker AXS Inc.; 2005.
- Sheldrick GM. A short history of SHELX. Acta Crystallogr A. 2008; 64(Pt 1):112–122.
- Macrae CF, Bruno IJ, Chisholm JA, et al. Mercury CSD 2.0 - new features for the visualization and investigation of crystal structures. J Appl Crystallogr. 2008;41(2):466–470.
- Spek AL. Structure validation in chemical crystallography. Acta Crystallogr D Biol Crystallogr. 2009;65(Pt 2):148–155.
- Brandenburg K, Putz H. DIAMOND, crystal impact GbR. Germany: Bonn; 2005.
- McKinnon JJ, Jayatilaka D, Spackman MA. Towards quantitative analysis of intermolecular interactions with hirshfeld surfaces. Chem Commun. 2007;37:3814–3816.
- McKinnon JJ, Mitchell AS, Spackman MA. Hirshfeld surfaces: a new tool for visualising and exploring molecular crystals. Chem Eur J. 1998;4(11):2136–2141.
- McKinnon JJ, Spackman MA, Mitchell AS. Novel tools for visualizing and exploring intermolecular interactions in molecular crystals. Acta Crystallogr B. 2004;60(Pt 6):627–668.
- Turner MJ, McKinnon JJ, Wolff SK, et al. Crystal explorer. Perth, Australia: University of Western Australia; 2017.
- Spackman MA, McKinnon JJ. Fingerprinting intermolecular interactions in molecular crystals. CrystEngComm. 2002;4(66):378–392.
- Venkatesan P, Thamotharan S, Ilangovan A, et al. Crystal structure, Hirshfeld surfaces and DFT computation of NLO active (2E)-2-(ethoxycarbonyl)-3-[(1-methoxy-1-oxo-3-phenylpropan-2-yl)amino] prop-2-enoic acid. Spectrochim Acta A Mol Biomol Spectrosc. 2016; 153:625–636.
- Kremer W, Weyand M, Winklmeier A, et al. 1.2 Å X-ray structure of the renal potassium channel K v 1.3 T1 domain. Protein J. 2013;32(7):533–542.