1. Introduction
Over the past 25 years, fragment-based drug discovery (FBDD) has emerged as a highly successful, complementary approach to high-throughput screening (HTS) to identify quality leads for optimization into clinical candidates and approved medicines. Four drugs on the market and over 40 new chemical entities in clinical trials discovered via FBDD campaigns have established their place in the armamentarium of medicinal chemistry, and most pharmaceutical and biotechnology companies, along with many academic institutions, have implemented FBDD [Citation1].
The theoretical basis of FBDD was established by the concept of using ‘small pieces of chemistry’ that are found to bind to the active sites of proteins [Citation2]. This idea was advanced by the observation that individual functional groups contributed to the energy of binding between a drug and its protein target [Citation3]. Another theoretical premise (the complexity model) postulates that there is a higher probability of a match between a ligand and its receptor if there are fewer interactions to get right, and higher complexity can lead to unfavorable interactions. Balanced with the probability of detection by biophysical assay methods, this leads to the concept that two to three pharmacophoric interactions per fragment are optimal [Citation4].
Fragment libraries sample a much larger chemical space than HTS libraries due to their smaller size, and a fragment library of only a few thousand members can routinely provide quality hits for hit to lead campaigns [Citation5]. Furthermore, hit rates from fragment screens are often used to assess whether a biological target is ‘ligandable’ and potentially druggable [Citation6]. The emerging success of FBDD has increased expectations of addressing more challenging new target classes, such as protein-protein and protein–DNA interactions.
Typical fragment hits have weak affinities, with equilibrium dissociation constant (Kd) values ranging from 1 mM to 100 µM, as compared with HTS hits, which have Kd values in the nM to 10 µM range [Citation6]. Such weak affinities are difficult to measure in standard biochemical assays used for HTS screens, so more sensitive biophysical techniques have been developed and successfully used in fragment screening: NMR, surface plasmon resonance, X-ray crystallography, thermal shift, affinity capture, and others [Citation7]. At least two orthogonal methods are used for validating screening hits. Thus, fragments need to be highly soluble (>10 mM in dimethyl sulfoxide) for screening at such high concentrations.
FBDD uses fragment linking, merging, and growing approaches during hit to lead and lead optimization stages of a discovery program. Structure-based drug design is normally used in most fragment-based optimizations to help guide synthetic efforts to increase binding affinity and navigate physicochemical properties [Citation8]. Another fragment-based metric, ligand efficiency (LE), has evolved to monitor affinity improvement so that increase in molecular weight and lipophilicity remain within productive ranges [Citation9]. LE is a ratio of affinity divided by the number of heavy atoms: a value of >0.3 is considered desirable.
2. New insights from 20 years of FBDD experience
New insights into drug discovery obtained over the past two decades have helped to develop new guidelines for more efficient practice of FBDD. One of the largest causes of drug attrition in clinical studies is toxicity, some of which is likely due to off-target interactions driven by lipophilicity [Citation10]. The recognition that compounds with enthalpy-dominated binding tend to be more drug-like because of their better physicochemical and pharmacokinetic properties [Citation11] suggests that early emphasis on polar interactions by the fragment approach is an advantage in drug discovery. Another insight gained is that protein ‘hot spots’ prefer fragments that contribute to enthalpy-driven binding, and fragments that bind overlapping the ‘hot spot’ preserve their binding mode as they evolve to drug-like ligands, whereas fragments that bind to other locations on the protein may change their binding mode upon elaboration [Citation12]. Such ‘hot spot’ interactions drive the specificity of ligand binding to target proteins (e.g. 2-aminopyridine to the hinge region of kinases). Dispersion forces evidenced in hydrophobic groups are now understood to contribute favorable entropy to binding affinity via both complementarity of protein-binding pockets and desolvation of ligands and pockets [Citation13].
Initially, fragment size and diversity centered around the ‘rule of three’, an adaptation of the Lipinski ‘rule of five’: a fragment should have a molecular weight (MW) of <300 Da, an octanol water coefficient (cLogP) less than three, three or fewer hydrogen bond donors, and three or fewer hydrogen bond acceptors. Three or fewer rotatable bonds and a total polar surface area of ≤60 were also considered beneficial [Citation14]. After multiple fragment screens and FBDD campaigns, these ‘rules’ have been revised to guidelines that keep most parameters flexible, with the only significant change being an MW of 100–250 Da or 7–20 non-hydrogen atoms [Citation7].
Early libraries were composed of 800–2000 fragments, but now there are no rules for the number of compounds that can be included in a library. Sets of up to 20,000 fragments have been reported, addressing the breadth and depth of diversity represented [Citation15]. Multiple fragment libraries are now available commercially, and the size of any customized library should be dependent on the available screening method. Obviously, the success of any FBDD campaign is highly dependent on the initial fragment hits, so it is essential that the fragments selected for such a library be carefully chosen.
3. Principles that define a good fragment
What makes a good fragment for inclusion in a fragment library? Several guidelines can be drawn based on the observations discussed in the previous sections and are summarized in .
Table 1. Guidelines for selection of a good fragment for FBDD
FBDD, fragment-based drug discovery
The starting point for good fragment selection is guideline 3, selecting the hydrogen bond donor- and acceptor-binding motifs as the first elements to drive future enthalpy-driven interactions. A list of a few such structural motifs are shown in ). Motifs of one to three hydrogen bond donors and one to three hydrogen bond acceptors are first selected (examples 1–16) and used to identify fragments of a variety of shapes that contain them, as exemplified in ) for the pyrazole motif 1 (examples 17–26), to generate a set of key pharmacophores. This set comprises additions of aryl and heteroaryl rings, which are polarizable (guideline 8), can provide favorable binding entropy via desolvation (guideline 4), and are driven by dispersion forces (guideline 5) which yield productive hydrophobicity. Selection of 3D shapes is encouraged at this point (examples 20, 22–23); further use of 3D shapes is expected to emerge during the hit to lead phase via fragment growing. In the final fragment selection phase, shown for the pyrazole example in ), additional groups can be added to modulate polarizability (examples 25–30) and small modifications can be made to increase functionality and 3-dimensionality (examples 31–35). These additions to each key pharmacophore increase the depth of analogs in the library and increase confidence in the hit results, along with structure–activity relationships that emerge from screening.
Figure 1. Examples of fragments for fragment-based drug discovery and the selection process for fragment library construction. (a) Examples of molecular recognition polar motifs (hydrogen bond donors and acceptors); (b) expansion of pyrazole moiety 1 to include some key pharmacophore shapes (in blue and magenta); and (c) pyrazole pharmacophores with variety of polarizable groups (in red) and additional groups (in brown) increase depth of coverage of the chemotype
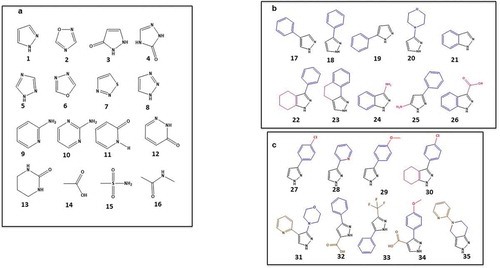
4. Expert opinion
The success of FBDD programs is dependent on the initial fragment hits; therefore, it is important that fragments constituting the library are carefully selected. Emphasis should be put on the enthalpy-driven pharmacophores, followed by diversity of shapes of fragments containing these molecular recognition motifs. A careful balance between polar functionality, pharmacophores, hydrophobicity, complexity, size, and diversity should be pursued during fragment selection. The number of analogs to be used per polar functionality will be dependent on the size of the library desired.
Fragments that are chemically unstable, are reactive, have toxicophoric liability, have aggregator potential, are chelators, or are pan-assay interference compounds should be avoided. Also, compounds that show up as frequent screening hits but do not advance further should be avoided [Citation1,Citation9]. Recent renewed interest in covalent inhibitors has increased the need to include covalent fragments in FBDD. Covalent fragments should still be selected based on the guidelines discussed here, and this library can be a separate, specialized library, as are existing fluorine-containing fragments used for screening by 19F-NMR and bromine-containing fragments used for screening by X-ray crystallography.
FBDD has become a full partner in drug discovery and holds great promise for new, innovative therapeutics; however, it is far from perfect and improvements are still needed. Better fragment screening techniques and methods are needed to decrease the dependency on high solubility, so that more fragments can be screened. Recent developments in crystallography and cryo-electron microscopy resolution need to be advanced so that difficult-to-crystallize proteins and protein complexes can become targets for FBDD. Synthetic methodology is needed for novel fragments to tackle targets that are not currently addressable. Synthesis of fragments that combine polar functionality with enriched 3D character is highly needed. New synthetic routes to all potential growth vectors of key fragments to enable faster fragment hit to lead elaboration remain a challenging step in FBDD.
Artificial intelligence approaches to understand SAR and attempt multiparameter optimization have emerged recently and offer great promise for designing new, advanced molecules. These approaches use large datasets and multi-QSAR methods to extract pieces of molecules (fragments) that represent the pharmacophoric elements and are devoid of toxicophores [Citation16]. Combining these fragments into molecules is expected to produce more drug-like compounds that can lead to better drugs. These methods provide another view as to how to identify and use virtual fragments.
Declaration of Interest
Z Konteatis is an employee of and holds stock in Agios Pharmaceuticals, Inc. He has no other relevant affiliations or financial involvement with any organization or entity with a financial interest in or financial conflict with the subject matter or materials discussed in the manuscript apart from those disclosed.
Reviewer disclosures
Peer reviewers on this manuscript have no relevant financial or other relationships to disclose.
Acknowledgments
Formatting assistance was provided by Helen Varley, PhD, CMPP, Excel Medical Affairs, Horsham, UK, supported by Agios Pharmaceuticals, Inc.
Additional information
Funding
References
- Erlanson DA, Fesik SW, Hubbard RE, et al., Twenty years on: the impact of fragments on drug discovery. Nat Rev Drug Discov. 2016;15(9):605–619.
- Jencks WP. On the attribution and additivity of binding energies. Proc Natl Acad Sci U S A. 1981;78(7):4046–4050.
- Andrews PR, Craik DJ, Martin JL. Functional group contributions to drug-receptor interactions. J Med Chem. 1984;27(12):1648–1657.
- Hann MM, Leach AR, Harper G. Molecular complexity and its impact on the probability of finding leads for drug discovery. J Chem Inf Comput Sci. 2001;41(3):856–864.
- Murray CW, Rees DC. The rise of fragment-based drug discovery. Nat Chem. 2009;1(3):187–192.
- Edfeldt FNB, Folmer RHA, Breeze AL. Fragment screening to predict druggability (ligandability) and lead discovery success. Drug Discov Today. 2011;16(7–8):284–287.
- Keserű GM, Erlanson DA, Ferenczy GG, et al., Design principles for fragment libraries: maximizing the value of learnings from pharma fragment-based drug discovery (FBDD) programs for use in academia. J Med Chem. 2016;;59(18):8189–8206.
- Ferenczy GG, Keserű GM. How are fragments optimized? A retrospective analysis of 145 fragment optimizations. J Med Chem. 2013;56(6):2478–2486.
- Hopkins AL, Keserü GM, Leeson PD, et al. The role of ligand efficiency metrics in drug discovery. Nat Rev Drug Discov. 2014;13(2):105–121. .
- Segall MD, Barber C. Addressing toxicity risk when designing and selecting compounds in early drug discovery. Drug Discov Today. 2014;19(5):688–693.
- Vogt M. Progress with modeling activity landscapes in drug discovery. Expert Opin Drug Discov. 2018;13(7):605–615. .
- Kozakov D, Hall DR, Jehle S, et al., Ligand deconstruction: why some fragment binding positions are conserved and others are not. Proc Natl Acad Sci U S A. 2015;112(20):E2585–E2594.
- Ferenzy GG, Keseru GM. Thermodynamic profiling for fragment-based lead discovery and optimization. Expert Opin Drug Discov. 2020;15(1):117–129.
- Congreve M, Carr R, Murray C, et al., A ‘rule of three’ for fragment-based lead discovery? Drug Discov Today. 2003;8(19): 876–877.
- Bollag G, Tsai J, Zhang J, et al., Vemurafenib: the first drug approved for BRAF-mutant cancer. Nat Rev Drug Discov. 2012;11(11):873–886.
- Kleandrova VV, Speck-Planche A. PTML modeling for Alzheimer’s Disease: design and prediction of virtual multi-target inhibitors of GSK3B, HDAC1, and HDAC6. Curr Top Med Chem. 2020;20(19):1657–1672.