1. Introduction
An average cost of $314 million to $2.8 billion is associated with the development of a new drug with a minimum development period of ten years [Citation1]. The traditional drug discovery process typically starts with target identification and validation, followed by assay development and high-throughput screening, hit generation and lead optimization. For the discovered lead compounds, preclinical studies in disease models are initiated for investigations regarding efficacy and safety. For these disciplines, artificial intelligence (AI) has gained enormous traction [Citation2].
Pharmacogenomics studies commonly investigate the role of genetic events in drug responses, and have strongly benefited from the advent of omics technologies, i.e. genomics, transcriptomics, epigenomics, and proteomics [Citation3]. Such studies ought to yield a molecular biomarker, which can be used to choose or develop an optimal treatment strategy for this particular patient group. Pharmacogenomic studies leverage large amounts of biomedical data, including electronic health records and deep molecular characterizations of human patients, in vitro or in vivo models.
In recent years, advances in computational biology, application of machine learning (ML) algorithms and deep learning (DL) algorithms empowered drug discovery [Citation4]. The impact of AI on precision medicine and subsequent deployment in clinical practice was facilitated by large-scale pharmacogenomic studies [Citation3]. Here, we discuss the history, state-of-the-art and our vision of AI in precision medicine. This is exemplified by applying AI to pharmacogenomic studies, i.e. we demonstrate how biomarkers are derived, and how AI may facilitate the next generation of precision medicine.
2. Artificial intelligence in precision medicine
AI is used as an umbrella term covering techniques which are aiming for training computer systems to act like humans and make rational decisions. A first attempt at AI was a binary input/output artificial neuron proposed by Warren McCulloch and Walter Pitts in 1943 [Citation5]. Several established concepts such as backpropagation, multi-layer perceptrons and recurrent neural networks (RNN) have existed since the 1970s and 1980s. However, the enhancement in the computational power and availability of large datasets in the last decade have enabled the implementation of these mathematical concepts in drug discovery and precision medicine.
AI comprises ML and DL, whilst DL is a subcategory of ML (). ML implements statistical learning algorithms to learn the behavior of a system and it is divided into supervised and unsupervised methods. Under the supervised method, inputs and outputs are available, with the goal of predicting the output based on input features. Regression and classification algorithms are considered to be supervised methods, while clustering algorithms are unsupervised methods since the outputs are missing. DL methods are using neural networks composed of multiple layers. Convolutional neural networks (CNN), natural language processing (NLP) and RNN are examples of DL algorithms.
Figure 1. Artificial intelligence for precision medicine. (a) Categorization of AI, ML, and DL. (b) Performance of ML and DL as a function of sample size. (c) The relation between the model complexity, interpretability and translatability. (d) Gartner hype cycle for AI in precision medicine.
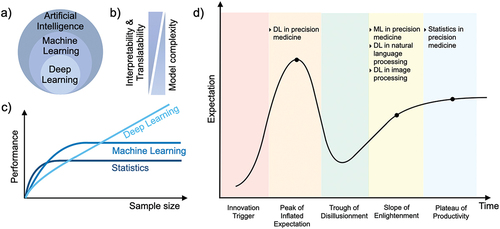
In general, there is a trade-off between the predictive power and interpretability of a model. Those models with the best predictive power are often complex, and therefore challenging to interpret due to their nonlinearity (). It is desirable to interpret predictions and gain insights into the importance of different input features. Hence, it is vital to find a reasonable balance regarding model complexity and interpretability. ML methods are usually less complex and require less training data to obtain a sufficiently precise prediction, whilst maintaining interpretability. In contrast, DL models are often more complex and benefit from larger datasets to increase predictive power, however, are more challenging to interpret ().
Here, we inspect AI for precision medicine according to the Gartner hype cycle (). Stages of a technology rise consist of five distinct phases: innovation trigger, peak of inflated expectations, trough of disappointment, slope of enlightenment, plateau of productivity. Today, deep learning for NLP and CNN for image analysis are at the slope of enlightenment, and have become indispensable tools for these tasks (). Precision medicine with traditional statistical methods has been actively practised in healthcare and drug discovery for decades, therefore, resides at the plateau of productivity (). Only recently, the applications of ML have gained traction for many tasks in drug discovery and development, drug repurposing and de novo drug design [Citation6]. Compared to ML, fewer DL applications are being regularly used in drug discovery and development stages [Citation7]. However, DL has not yet met the extremely high expectations that stem from its immense success in natural language processing and image analysis. For example, real-time recommendation systems for guiding treatment decisions using electronic health records and molecular data are not feasible yet [Citation3]. Due to the small number of examples for which AI in precision medicine impacted clinical practice, it is currently dropping from the peak of the inflated expectations to the trough of disillusionment [Citation8] ().
3. Leveraging artificial intelligence in pharmacogenomics for precision medicine
Statistical methods have been a foundation for increasing productivity of the drug discovery process. The rise of molecular datasets facilitated the discovery of molecular biomarkers for disease risk, diagnosis, progression, and treatment customization. In the past, the time from target identification to drug approval used to take 12 to 15 years [Citation9], which can be accelerated by biomarker discovery. For example, it only took 11 years between the identification of HER2 amplification and the approval of trastuzumab, a potent HER2 inhibitor [Citation10]. The discovery of BRAFV600E activation in malignant melanoma led to vemurafenib (PLX-4032, a BRAF inhibitor) approval in only 9 years [Citation11]. The aim of pharmacogenomics screens is to discover such associations through high-throughput screens (HTS) in in vitro and in vivo disease models [Citation12,Citation13].
Here, we exemplify the process of biomarker discovery by the BRAF inhibitor PLX-4720 in a cancer cell line HTS [Citation12]. In this screen, 34 melanoma cell lines were previously characterized by the mutational status of 28 genes (mutant or wild type) [Citation12]. Drug response is quantified by the half maximal inhibitory concentration (IC50). Systematic applications of ANOVA models revealed differences in drug response, depending on the mutational status of several cancer genes (). Accordingly, BRAF mutations in melanoma are confirmed as PLX-4720 drug sensitivity biomarker (). In , the Cohen’s d effect size is equal to the difference between two means divided by a standard deviation.
Figure 2. Artificial intelligence in pharmacogenomics and precision medicine. (a) Statistical methods are the foundation of precision medicine, e.g. (b) leveraging ANOVA models to investigate genetic biomarkers in melanoma cell lines treated with the BRAF inhibitor PLX-4720. (c) BRAF mutant cancer cell lines are sensitive to PLX-4270 treatment. (d) ML models enable predictive modeling in pharmacogenomics. (e) Clustering of melanoma cell lines according to their mutational status visualized in the UMAP space. (f) Prediction of IC50 values of cell lines treated with a BRAF inhibitor using a linear regression model. (g) Classification of drug response using a random forest model. (h) DL architectures empower modeling of complex associations in drug discovery. For instance, (i) an autoencoder architecture for creating a two dimensional manifold of melanoma cell lines for consecutive downstream analysis, and (j) a feed-forward neural network architecture for drug response classification.
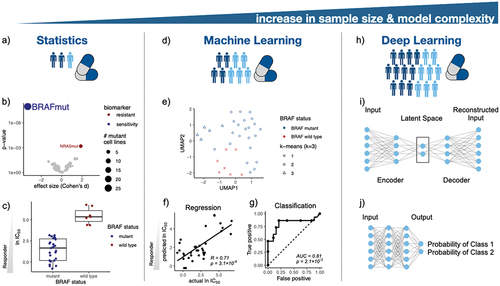
Increasing molecular landscapes and greater sample sizes enabled AI models in biomarker discovery [Citation12,Citation14,Citation15]. The field of pharmacogenomics is pioneering AI-driven biomarker discovery by predicting drug response through molecular features of disease models. For example, the binary mutational status of 28 genes from the previous ANOVA analysis will be used as input features. ML and DL algorithms used in biomarker discovery are clustering, regression and classification approaches () [Citation16,Citation17]. Here exemplified, we visualized the embedding of mutational status of melanoma cell lines (). K-means clustering on the mutational status revealed that melanoma cell lines can be distinguished by their BRAF status. A linear regression model on 28 mutations in melanoma cell lines was able to predict IC50 values of cell lines treated with a BRAF inhibitor (Pearson correlation = 0.71, ). This regression problem can be simplified to a classification task by binarizing drug response, e.g. assuming drug responder for IC50 values lower than 10 μM. For instance, a random forest model achieved a good classification performance of AUC = 0.81 (). The models were trained using a nested 5-fold cross-validation (CV). The inner CV was used to choose the hyperparameters of the implemented ML methods, whereas the outer CV was used to report a nearly unbiased test performance.
Drug response may be modeled using DL, for which data is processed in layers in order to extract higher-level features. Autoencoders create a lower-dimensional latent space embedding of molecular characterizations for downstream clustering (). Multi layer perceptrons (MLPs) can be used for regression and classification tasks, here exemplified, to classify responders and non-responders (). For demonstrating differential drug responses of BRAF mutant melanoma cell lines treated with BRAF inhibitors, linear models suffice. However, non-linear relationships are common in pharmacogenomics due to diversity of the molecular composition of the investigated diseases. DL techniques are well equipped to capture non-linear and very complex dependencies.
DL is the fastest growing field of AI, and offers unique opportunities to address challenges in pharmacogenomics. For example, transfer learning techniques can leverage datasets with large sample sizes to predict drug response in disease models for which only few samples are available [Citation18]. Another example for the application of DL in HTS are autoencoders and convolutional neural networks, which can identify novel compounds and may reveal biomarkers by examining feature weights [Citation19–21]. Moreover, DL advanced the prediction of drug combinations for overcoming the resistance mechanisms which may occur in monotherapies [Citation22].
4. Expert opinion
Our growing ability to produce, integrate and interpret biomedical data empowers the usage of complex AI models. This may enhance predictive power, lead to efficient drug discovery and development processes at reduced costs. However, increased model complexity comes at the cost of interpretability. Hence, as a guideline we recommend to start with simple statistical models and increase complexity until acceptable performance is achieved, or predictive power saturates. In other words, use the appropriate computational model for the correct task, and know its limitations. The scientific community and industry are recently beginning to appreciate the advantages of AI in drug discovery, and its adoption may lead to new clinical implementation of precision medicine.
It is our opinion that AI has the potential to enhance the success rate of clinical trials, save time and reduce the overall costs in drug discovery and development. Therefore, it has attracted the attention of the pharmaceutical industry and researchers. Applications of AI in drug development are being pioneered by cancer pharmacogenomics and are mainly used in research and early stages of drug development. Some of the applications in which ML and DL can advance drug discovery are drug repurposing, biomarker discovery, patient stratification and subtyping, optimization of high-throughput screens, multi-omics integration, tumor detection from medical images, and precision medicine. Despite the attractive use cases of AI, the interpretability and clinical translatability of complex AI models such as DL models are still challenging in drug development due to imposed regulatory hurdles. In particular, AI needs to overcome ethical and liability issues, i.e. who is responsible for erroneous predictions of an AI algorithm which may lead to poor treatment outcomes? Therefore, we believe that advances in interpretable AI will determine the success of AI in precision medicine.
Notably, precision medicine can only make use of big data if privacy and data security issues posed by sensitive patient data are addressed. For that, novel methods such as federated learning and swarm learning have been developed, which are largely pioneered by DL algorithms in a decentralized manner while avoiding data exchange [Citation23,Citation24]. Access to high-quality data, novel measurements, and software technologies will accelerate the revolution of AI in drug discovery. However, data security and the integration of novel AI methods into validated systems are still challenging in the highly regulated pharmaceutical industry. We strongly believe that this AI research sector will expand in the coming years.
Once the above-mentioned challenges are addressed, it is our belief that AI will unfold its full power across all stages of drug discovery and development. The presence of AI algorithms already revolutionized early drug development and will further impact the later drug development stages. This is, we anticipate that AI will shape clinical studies by providing more insights into the patient data and biomarker analysis, which will finally lead to an AI-driven treatment plan. Empowered by the impressive use-cases of AI and growing trust in this innovative technology, we predict that AI will undoubtedly accelerate preclinical drug discovery and precision medicine.
Declaration of Interest
MP Menden is a former employee of AstraZeneca who collaborates with GlaxoSmithKline, AstraZeneca and Roche. He also declares research funding from GlaxoSmithKline and Roche. The authors have no other relevant affiliations or financial involvement with any organization or entity with a financial interest in or financial conflict with the subject matter or materials discussed in the manuscript apart from those disclosed.
Reviewer disclosures
Peer reviewers on this manuscript have no relevant financial or other relationships to disclose.
Additional information
Funding
References
- Wouters OJ, McKee M, Luyten J. Estimated research and development investment needed to bring a new medicine to market, 2009-2018. JAMA. 2020;323(9):844–853.
- Bender A, Cortés-Ciriano I. Artificial intelligence in drug discovery: what is realistic, what are illusions? Part 1: ways to make an impact, and why we are not there yet. Drug Discov Today. 2021;26(2):511–524.
- Boniolo F, Dorigatti E, Ohnmacht AJ, et al., Artificial intelligence in early drug discovery enabling precision medicine. Expert Opin Drug Discov. 16(9): 991–1007. 2021.
- de Azevedo WF. Application of machine learning techniques for drug discovery. Curr Med Chem. 2021;28(38):7805–7807.
- McCulloch WS, Pitts W. A logical calculus of the ideas immanent in nervous activity. Bull Math Biophys. 1943;5(4):115–133.
- Wójcikowski M, Siedlecki P, Ballester PJ. Building machine-learning scoring functions for structure-based prediction of intermolecular binding affinity. Methods Mol Biol. 2019;2053:1–12.
- Vamathevan J, Clark D, Czodrowski P, et al., Applications of machine learning in drug discovery and development. Nat Rev Drug Discov. 18(6): 463–477. 2019.
- Fröhlich H, Balling R, Beerenwinkel N, et al. From hype to reality: data science enabling personalized medicine. BMC Med. 2018;16(1):150.
- Roses AD. Pharmacogenetics in drug discovery and development: a translational perspective. Nat Rev Drug Discov. 2008;7(10):807–817.
- Slamon DJ, Clark GM, Wong SG, et al., Human breast cancer: correlation of relapse and survival with amplification of the HER-2/neu oncogene. Science. 235(4785): 177–182. 1987.
- Davies H, Bignell GR, Cox C, et al., Mutations of the BRAF gene in human cancer. Nature. 417(6892): 949–954. 2002.
- Iorio F, Knijnenburg TA, Vis DJ, et al., A landscape of pharmacogenomic interactions in cancer. Cell. 166(3): 740–754. 2016.
- Gao H, Korn JM, Ferretti S, et al. High-throughput screening using patient-derived tumor xenografts to predict clinical trial drug response. Nat Med. 2015;21(11):1318–1325.
- Garnett MJ, Edelman EJ, Heidorn SJ, et al., Systematic identification of genomic markers of drug sensitivity in cancer cells. Nature. 483(7391): 570–575. 2012.
- Quaranta M, Knapp B, Garzorz N, et al. Intraindividual genome expression analysis reveals a specific molecular signature of psoriasis and eczema. Sci Transl Med. 2014;6(244):244ra90–244ra90.
- Schaebitz A, Hillig C, Farnoud A, et al. Low numbers of cytokine transcripts drive inflammatory skin diseases by initiating amplification cascades in localized epidermal clusters. bioRxiv. 2021. https://www.biorxiv.org/content/ https://doi.org/10.1101/2021.06.10.447894v1.
- Cancer Cell Line Encyclopedia Consortium and Genomics of Drug Sensitivity in Cancer Consortium. Pharmacogenomic agreement between two cancer cell line data sets. Nature. 2015;528(7580):84–87.
- Turki T, Wei Z, Wang JTL. Transfer learning approaches to improve drug sensitivity prediction in multiple Myeloma patients. IEEE Access. 2017;5:7381–7393.
- Rampášek L, Hidru D, Smirnov P, et al. Dr.VAE: improving drug response prediction via modeling of drug perturbation effects. Bioinformatics. 2019;35(19):3743–3751.
- Chang Y, Park H, Yang H, et al. Cancer Drug Response Profile scan (CDRscan): a deep learning model that predicts drug effectiveness from cancer genomic signature. Sci Rep. 2018;8(1):8857.
- Manica M, Oskooei A, Born J, et al. Toward explainable anticancer compound sensitivity prediction via multimodal attention-based convolutional encoders. Mol Pharm. 2019;16(12):4797–4806.
- Menden MP, Wang D, Mason MJ, et al., Community assessment to advance computational prediction of cancer drug combinations in a pharmacogenomic screen. Nat Commun. 10(1): 2674. 2019.
- Konečný J, McMahan HB, Yu FX, et al. Federated learning: strategies for improving communication efficiency. arXiv Preprint arXiv;2017. https://arxiv.org/abs/1610.05492
- Warnat-Herresthal S, Schultze H, Lingadahalli Shastry K, et al., Swarm learning for decentralized and confidential clinical machine learning. Nature. 594(7862): 265–270. 2021.