1. Introduction
The novel severe acute respiratory syndrome coronavirus 2 (SARS-CoV-2) outbreak in 2019 was followed by an international emergency outcry. COVID-19 related illnesses have claimed over six million lives, a number that is still rising, and the SARS-CoV-2 virus only partly mitigated.
To combat this virus family, the world is in need of a potent medication with broad applicability and high efficacy. It would make sense to repurpose the already well characterized small molecules to address this emergency given that the clinical trials and approval process for novel designed small molecules take roughly 10–15 years in the United States (US).
Drug repurposing is a resourceful way of expanding the available number of therapies by exploiting existing and approved drugs. Unrelated proteins can share similar shapes and surfaces in their binding region [Citation1]. Therefore, many drugs may have multiple targets, albeit with varying affinities. A recent study showed that on average, a drug can bind up to 329 proteins in the human proteome [Citation2]. The current landscape of drug repurposing mostly relies on computational approaches.
The rapid increase in computing power and memory storage enabled by the increase in investment in research and development in both artificial intelligence (AI) programming and computer hardware has accelerated computational approaches for screening of approved or investigational drugs. In silico drug screening approaches as well as computational biophysics applications are frequently visited for drug repurposing studies. However, there is still room for improvement, which can be fueled by further improvement of algorithmic innovations and computing power.
AI has revolutionized drug discovery with its immense power to meticulously discover hidden patterns and evidence from biomedical data. Pharmaceutical companies and start-ups have started to widely use AI for drug discovery and development. Despite initial resistance on the research bench, the technology is well advanced in pharmaceutical industry laboratories [Citation3]. However, AI has yet to produce a drug from scratch.
Here, we focus on how AI impacts COVID-19 drug repurposing strategies and compare it to more traditional computational techniques used in drug repurposing. The urgent need to respond to the COVID-19 pandemic created an opportunity to quickly test AI-based drug repurposing approaches. We also discuss lessons to be taken from this opportunity to provide further insights for targeting other human diseases.
1.1. Current landscape of drug repurposing: the implications of drug repurposing in COVID-19 drug discovery research
The high cost of developing new drugs, which involves robust research and development and testing on a large human population for both safety and efficacy, has made drug repurposing an attractive alternative approach [Citation4]. Repurposing of existing drugs eliminates concerns over drug toxicity and other side effects that are potentially limiting for a newly developed drug candidate [Citation5]. The technics used for drug repurposing can be classified broadly into computational and experimental [Citation4]. Experimental approaches mainly involve high throughput screens of existing drugs for binding and/or inhibition of novel targets in affinity assays, and phenotypic screening of the drugs in vitro or in in vivo disease models. Computational approaches, on the other hand, comprise of a wide variety of methods, including but not limited to pathway mapping, identifying gene associations, signature matching, molecular docking and retrospective clinical analysis. Recently, with the advent of AI and machine learning (ML), there is a potential for some of the above computational approaches to be integrated to identify drugs that could be repurposed for other diseases using as diverse sourced data ranging from molecular signatures to the effects of the drug at the molecular and the system levels.
Drug repurposing is becoming more and more data driven, as opposed to previous decades when it was mainly retrospective clinical studies based on serendipitous discoveries. One of the famous examples of this is the blockbuster Pfizer drug Viagra, which was repurposed from sildenafil originally developed by researchers to treat cardiovascular diseases [Citation6]. Similarly, other drugs, such as canakinumab (arthiritis drug) [Citation7] and bimatoprost (eye health) [Citation8] were repurposed after patients reported favorable side effects that allowed their usage to treat other diseases. Carbamazepine (Tegretol, epilepsy) is another example. While researchers have understood the molecular underpinning of the effects of these drugs after realizing their repurposing potential, those discoveries are rooted in serendipity and astute observations of the side effects.
In recent times, however, researchers have employed large-scale computation-guided approaches for drug repurposing. For example, Dudley and coworkers [Citation9] have used drug-disease similarity approach to identify topiramate, an antiepileptic drug to treat inflammatory bowel disease (IBD) by comparing gene expression signature of the disease against that of approved drugs. In another example, Iorio and coworkers [Citation10] constructed a network map of approved drugs based on drug–drug similarity, which was then utilized to identify fasudil, a RhoA/ROCK pathway inhibitor, as an anti-neurodegenerative drug to treat ALS. Such advancements aided by high throughput computational screens have enabled fast repurposing of drugs in emergency times of the beginning of the recent pandemic.
Antivirals, as a group of drugs, have been more amenable for repurposing due to the similarities in host and viral proteins, and mechanism of action between viruses. Li and Peng [Citation11] have assembled a comprehensive list of antivirals that were repurposed based on their action against other viruses. There are several examples of direct-acting repurposed antivirals (DARA) and host-targeting repurposed antivirals (HTRA) that are currently at various stages of clinical trials [Citation11]. These repurposing efforts were accelerated more than ever before when the community had to deal with the global pandemic, which led to several treatment options with varying degrees of success [Citation12]. The first major ‘successful’ attempt of antiviral repurposing was treating SARS-CoV2 using remdesivir, which was carried out using a combination of computational and experimental drug centric approaches [Citation13]. Remdesivir is a broad spectrum antiviral in the form of adenosine analogue initially developed as an antiviral against HCV and then repurposed for treating Ebola infections [Citation14]. The drug centered repurposing approach showed effective binding of the drug to the viral RNA-dependent RNA polymerase (RdRP) protein in docking studies and efficacy of the drug against human coronaviruses in viral inhibition studies, which was then used to treat SARS-CoV2. However, there were several target-centric approaches that were readily applied, since knowledge of both the predecessor viruses (SARS-CoV and MERS), and host proteins were already available. Khelfaoui and coworkers [Citation15] performed molecular docking with the DrugBank database to identify compounds that target the ACE2 receptor. Ozdemir and coworkers [Citation16] performed a similar study to target host proteases and viral proteins that are involved in the entry of viral into the host cell, and identified potential viral inhibitors.
Although drug repurposing studies can be challenging due to the limited number of new drugs to repurpose, regulatory and patent consideration, as well as the limited efficacy of repurposed drugs [Citation17], data from the aforementioned studies, as well as many others, are providing data to identify drugs with AI-driven methods. Data is critical for AI-based approaches. A large amount of data has been generated on inhibitors for SARS-CoV-2 proteins such as Mpro and RdRp [Citation18], which can aid in the development of more powerful AI-driven drug design.
2. Recent advancements in AI: COVID-19 provided an opportunity to accelerate AI-based drug repurposing
The disadvantages of drug repurposing due to a lack of knowledge on the complete drug-target network can be overcome by incorporating AI and network medicine into the studies. Sometimes drug repurposing is referred to as ‘finding a needle in a haystack,’ but AI is assisting in mapping a blueprint of the haystack and demonstrating how to begin the search.
Deep learning, ML and several neural network models are examples of AI approaches that are referred to here. The most fundamental approach to integrate AI into drug repurposing is known as network medicine, and it refers to the construction of medical knowledge graphs. These graphs contain the existing links between diseases, drugs, and proteins and are used to predict new links between those [Citation19].
Here are some selected examples of several studies that identified COVID-19 drugs, including antiviral drugs and host-targeting therapies, combining the repurposing and AI approaches. Zhou et al. quantified the interplay between the virus–host interactome and drug targets in the human protein–protein interactome and identified 16 potential drugs including melatonin, mercaptopurine, and sirolimus as anti-human coronavirus drugs [Citation20].
A comprehensive medical knowledge graph was created by Zeng et al. using a large scientific corpus of 24 million PubMed publications to connect treatments, illnesses, proteins/genes, pathways, and expression [Citation21]. Dexamethasone, indomethacin, niclosamide, and toremifene were among the 41 drugs they discovered.
Ge et al. developed an integrative drug repositioning framework that uses ML and statistical analysis approaches to generate a knowledge graph. They validated their finding with experimental methods and showed that their potential drug candidate, CVL218, has the highest tissue distribution in lungs and can be used as an anti-SARS-CoV-2 agent, as lung is the therapeutically targeted tissue for COVID-19 [Citation22].
Beck et al. used a pre-trained deep learning-based drug–target interaction model to identify commercially available drugs such as atazanavir, remdesivir, efavirenz, ritonavir, and dolutegravir, for potential treatment of SARS-CoV-2 infection [Citation23].
These studies do not recommend specific drugs to be immediately used in COVID-19 treatment. However, they all show that AI methodology, deep learning, knowledge graph construction, data mining, ML and neural network models can be used to quickly prioritize existing drugs for further investigation toward potential treatment of COVID-19. Comparing to the current drug development pipeline, which requires almost 12–15 years of efforts, AI-based drug repurposing approaches can significantly reduce the cost and speed up the individual steps. The time required for drug discovery and pre-clinical steps, which is usually around 5 years, can be cut to months. A similar pattern for clinical trials is expected. Therefore, pharmaceutical companies are reshaping their business models to benefit from even a fraction of the cost and time reduction by AI-based approaches [Citation24]. Given that COVID-19 is a global emergency, any reduction in the time it takes to obtain treatment means more lives saved. summarizes common inputs that AI approaches can benefit from and potential outputs and future implications of these applications.
Figure 1. A summary of common inputs of AI approaches and potential outputs to target COVID-19. These outputs are expected to help in the development of personalized treatments for complex diseases in the future. (This figure is created with BioRender.).
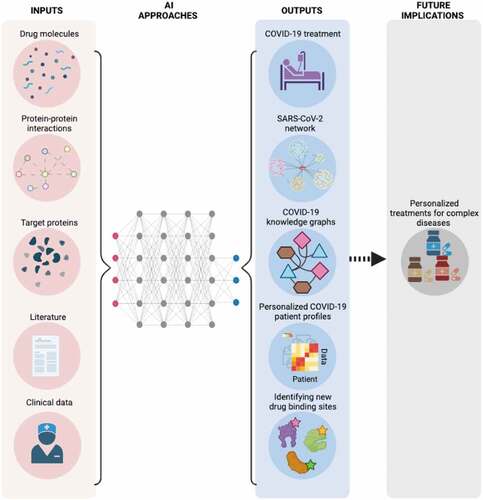
The information gathered and networks formed as a result of these studies may help in the creation of efficient treatment protocols for additional emerging infectious as well as emerging complex diseases. Moreover, the immense genomic data obtained using AI may represent an unparalleled opportunity for drug repurposing in precision medicine and personalized treatment. Another opportunity presented by the pandemic is to demonstrate how quickly a treatment can be developed when the scientific community – institutions, pharmaceutical companies, and academia – all collaborate. Other complex diseases with limited treatment options, such as some cancers and Alzheimer’s, should be studied in the same way, and we expect that many more treatments for many more diseases will be identified [Citation25].
On the other hand, AI and ML approaches suffer from some limitations and problems, which to date may constrain their usage for drug repurposing. Input data are crucial for AI and ML approaches to generate meaningful and accurate data. Data harmonization, that is, standardizing and integrating the heterogenous and low-quality input data, is still an important challenge [Citation19]. Also, the data can be insufficient in many cases due to data sharing policies of the pharmaceutical companies. The complexity of the biological systems is yet another challenge that AI approaches are struggling to keep up with. In order to address these challenges AI can be coupled with approaches such as enriching the biologically inspired visible networks and mechanistic computer modeling, which can better represent the mechanism of action of specific therapeutic strategies at the cellular and molecular levels [Citation26].
3. Expert opinion
Despite the promise, to date, artificial intelligence has not led to a COVID-19 drug in the clinic, and neither has it led to drugs in the clinic through repurposing. Among the common reasons advanced for this is the scarcity of openly available clinical patient data, a problem which is especially acute considering the heterogeneity of individuals in real-world patient settings. We do not expect this problem to go away any time soon. In cancer, the over-emphasis of genetic alterations, while often ignoring repurposing, which is not based on genomics data, was also faulted as contributing to inaccuracy [Citation27]. Here, we pivot to the vital importance of three-dimensional protein structural data. The major tenet of repurposing is the requirement for similar drug binding sites. Drugs bind in cavities with favorable chemistry and geometry. A recent review underscored the increasing availability of open and freely available structural data [Citation28]. While not a substitute for vital, diverse, and openly available patient data, it does provide an added key element in repurposing and deep learning in AI. Considering lessons for the future, we advocate increasing algorithmic and statistical emphasis on three dimensional structures, particularly of proteins. Experimental high-resolution structures, determined by crystallography, NMR and Cryo-EM will be complemented by large scale and increasingly accurate modeling, achieved e.g. by subsequent AlphaFold versions, also an AI-based approach. Repurposed drugs for patients can constitute a fast, and realizable venue toward precision medicine, fighting the still on-going global COVID-19 pandemic.
So how then has AI impacted COVID-19 drug repurposing? To date it holds a promise, but no tangible results yet. What lessons have we learned? We believe that AI in repurposing is a promising strategy, especially when three-dimensional structural data is learnt and exploited. Furthermore, successful repurposing is not only a question of science, but also an economic issue, which also forms an integral part of the drug development model adopted by Big Pharma.
Declaration of interests
The authors have no relevant affiliations or financial involvement with any organization or entity with a financial interest in or financial conflict with the subject matter or materials discussed in the manuscript. This includes employment, consultancies, honoraria, stock ownership or options, expert testimony, grants or patents received or pending, or royalties.
Reviewer disclosures
Peer reviewers on this manuscript have no relevant financial or other relationships to disclose.
Additional information
Funding
References
- Ozdemir ES, Halakou F, Nussinov R, et al. Methods for discovering and targeting druggable protein-protein interfaces and their application to repurposing. Methods Mol Biol. 2019;1903:1–21.
- Zhou H, Gao M, Skolnick J. Comprehensive prediction of drug-protein interactions and side effects for the human proteome. Sci Rep. 2015 Jun;9(5):11090.
- Mullin R. Sold on the new machine. C&EN Global Enterp. 2022 Feb 21;100(7):28–33.
- Pushpakom S, Iorio F, Eyers PA, et al. Drug repurposing: progress, challenges and recommendations. Nat Rev Drug Discov. 2019 Jan;18(1):41–58.
- Ashburn TT, Thor KB. Drug repositioning: identifying and developing new uses for existing drugs. Nat Rev Drug Discov. 2004 Aug;3(8):673–683.
- Jackson G, Gillies H, Osterloh I. Past, present, and future: a 7-year update of Viagra (sildenafil citrate). Int J Clin Pract. 2005 Jun;59(6):680–691.
- Fender AC, Dobrev D. Repurposing traditional immunomodulators to target the inflammatory burden of atherosclerosis. Int J Cardiol Heart Vasc. 2020 Jun;28:100535.
- Novack GD. Repurposing medications. Ocul Surf. 2021 Jan;19:336–340.
- Dudley JT, Sirota M, Shenoy M, et al. Computational repositioning of the anticonvulsant topiramate for inflammatory bowel disease. Sci Transl Med. 2011 Aug 17;3(96):96ra76.
- Iorio F, Isacchi A, Di Bernardo D, et al. Identification of small molecules enhancing autophagic function from drug network analysis. Autophagy 2010 Nov;6(8):1204–1205.
- Li X, Peng T. Strategy, progress, and challenges of drug repurposing for efficient antiviral discovery. Front Pharmacol. 2021;12:660710.
- Administration USFaD. Coronavirus treatment acceleration program (CTAP). 2022; [cited 2022 Aug 11]. Available from: https://www.fda.gov/drugs/coronavirus-covid-19-drugs/coronavirus-treatment-acceleration-program-ctap
- Beigel JH, Tomashek KM, Dodd LE. Remdesivir for the treatment of covid-19 - preliminary report. N Engl J Med. 2020 Sep 3;383(10):994.
- Tchesnokov EP, Feng JY, Porter DP, et al. Mechanism of Inhibition of Ebola virus RNA-dependent RNA polymerase by remdesivir. Viruses. 2019 Apr 4;11(4):326.
- Khelfaoui H, Harkati D, Saleh BA. Molecular docking, molecular dynamics simulations and reactivity, studies on approved drugs library targeting ACE2 and SARS-CoV-2 binding with ACE2. J Biomol Struct Dyn. 2021 Nov;39(18):7246–7262.
- Ozdemir ES, Le HH, Yildirim A, et al. In silico screening and testing of FDA-approved small molecules to block SARS-CoV-2 entry to the host cell by inhibiting spike protein cleavage. Viruses. 2022;14(6):1129.
- Ng YL, Salim CK, Chu JJH. Drug repurposing for COVID-19: approaches, challenges and promising candidates. Pharmacol Ther. 2021 Dec;228:107930.
- Macip G, Garcia-Segura P, Mestres-Truyol J, et al. A review of the current landscape of SARS-CoV-2 main protease inhibitors: have we hit the bullseye yet? Int J Mol Sci. 2021 Dec 27;23:1.
- Zhou Y, Wang F, Tang J, et al. Artificial intelligence in COVID-19 drug repurposing. Lancet Digit Health. 2020 Dec;2(12):e667–e676.
- Zhou Y, Hou Y, Shen J, et al. Network-based drug repurposing for novel coronavirus 2019-nCoV/SARS-CoV-2. Cell Discov. 2020;6:14.
- Zeng X, Song X, Ma T, et al. Repurpose open data to discover therapeutics for COVID-19 using deep learning. J Proteome Res. 2020 Nov 6;19(11):4624–4636.
- Ge Y, Tian T, Huang S, et al. An integrative drug repositioning framework discovered a potential therapeutic agent targeting COVID-19. Signal Transduct Target Ther. 2021 Apr 24;6(1):165.
- Beck BR, Shin B, Choi Y, et al. Predicting commercially available antiviral drugs that may act on the novel coronavirus (SARS-CoV-2) through a drug-target interaction deep learning model. Comput Struct Biotechnol J. 2020;18:784–790.
- Paul D, Sanap G, Shenoy S, et al. Artificial intelligence in drug discovery and development. Drug Discov Today. 2021 Jan;26(1):80–93.
- Notman N. Teaching old drugs new tricks to treat COVID-19. Nature Synthesis. 2022 Jan 01;1(1):2–5.
- Russo G, Di Salvatore V, Sgroi G, et al. A multi-step and multi-scale bioinformatic protocol to investigate potential SARS-CoV-2 vaccine targets. Brief Bioinform. 2021;23:1.
- Tanoli Z, Vaha-Koskela M, Aittokallio T. Artificial intelligence, machine learning, and drug repurposing in cancer. Expert Opin Drug Discov. 2021 Sep;16(9):977–989.
- Nussinov R, Jang H, Nir G, et al. Open structural data in precision medicine. Annu Rev Biomed Data Sci. 2022Apr;28:95–117.