Abstract
Aim: Assessing the visual accuracy of two large language models (LLMs) in microbial classification. Materials & methods: GPT-4o and Gemini 1.5 Pro were evaluated in distinguishing Gram-positive from Gram-negative bacteria and classifying them as cocci or bacilli using 80 Gram stain images from a labeled database. Results: GPT-4o achieved 100% accuracy in identifying simultaneously Gram stain and shape for Clostridium perfringens, Pseudomonas aeruginosa and Staphylococcus aureus. Gemini 1.5 Pro showed more variability for similar bacteria (45, 100 and 95%, respectively). Both LLMs failed to identify both Gram stain and bacterial shape for Neisseria gonorrhoeae. Cumulative accuracy plots indicated that GPT-4o consistently performed equally or better in every identification, except for Neisseria gonorrhoeae's shape. Conclusion: These results suggest that these LLMs in their unprimed state are not ready to be implemented in clinical practice and highlight the need for more research with larger datasets to improve LLMs' effectiveness in clinical microbiology.
Plain language summary
This study looked at how well large language models (LLMs) could identify different types of bacteria using images, without having any specific training in this area beforehand.
We tested two LLMs with image analysis capabilities, GPT-4o and Gemini 1.5 Pro. These models were asked to determine whether bacteria were Gram-positive or Gram-negative and whether they were round (cocci) or rod-shaped (bacilli). We used 80 images of four stained bacteria from a labeled database as a reference for this test.
GPT-4o was more accurate in identifying both the Gram stain and shape of the bacteria compared with Gemini 1.5 Pro. GPT-4o had excellent accuracy in correctly classifying the Gram stain and bacterial shape of Clostridium perfringens, Pseudomonas aeruginosa and Staphylococcus aureus. Gemini 1.5 Pro had mixed results for these bacteria. However, both models struggled with Neisseria gonorrhoeae, failing to correctly identify its Gram stain and shape.
The study shows that while these LLMs have potential, they are not ready to be implemented in clinical practice. More research and larger datasets are needed to improve their accuracy in clinical microbiology.
Large language models (LLMs) are advanced artificial intelligence models, able to generate human-like text, with sophisticated natural language processing capabilities. They are trained on vast amounts of data and use deep learning techniques to understand complex inputs and produce language.
Recent studies have shown the potential of LLMs in medical image analysis across various fields like pathology and ophthalmology, demonstrating their ability to interpret complex medical visual data.
Clinical decisions on infection management such as initial antibiotic choice often rely on Gram stain results. Thus, it is crucial to ensure these tests are conducted and interpreted accurately.
Materials & methods
Two LLMs were used in this study: Open AI's generative pretrained transformer (GPT), version 4 Omni (GPT-4o) and Google's Gemini version 1.5 Pro.
To the best of our knowledge, this study represents the first known accuracy analysis of the latest and most advanced visual LLMs, GPT-4o and Gemini 1.5 Pro, in the domain of Gram stain and bacterial shape identification.
A publicly available database of bacterial Gram stains was used. 80 bacterial samples were divided evenly among four bacteria representing all possible combinations of Gram Stain and bacterial shape.
Results
GPT-4o correctly identified both the Gram stain and bacterial shape simultaneously with higher accuracy then Gemini 1.5 Pro (75 vs. 60%, respectively).
When examining the performance by specific bacteria, GPT-4o achieved 100% accuracy in identifying both the Gram stain and shape correctly for Clostridium perfringens, Pseudomonas aeruginosa and Staphylococcus aureus. Gemini 1.5 Pro, on the other hand, showed more variability in its performance for the same bacteria (45, 100 and 95%, respectively). However, both LLMs failed to correctly identify the Gram stain and bacterial shape in all cases (0% accuracy) with Neisseria gonorrhoeae.
Cumulative accuracy plots indicated that GPT-4o consistently performed equally or better in every identification, except for Neisseria gonorrhoeae‘s shape.
Discussion
The results from this study provide valuable insights into the potential and limitations of these LLMs in microbial classification tasks, demonstrating their potential for microbial classification tasks without prior domain-specific training.
The results suggest that these LLMs in their unprimed state are not ready to be implemented in clinical practice.
The results underscore the need for further research with larger and more diverse datasets, as well as offline clinical samples, to better understand and enhance the capabilities of these LLMs in clinical microbiology.
1. Background
Gram staining is one of the most important staining techniques in microbiology. It was first introduced by the Danish bacteriologist Hans Christian Gram in 1882 [Citation1]. It categorizes bacteria into two groups: Gram-positive (purple colored) and Gram-negative (red colored), based on their ability to retain the stain [Citation2]. Bacteria are further classified by morphology as cocci (spherical) or bacilli (rod-shaped). Gram stain interpretation is a task that is undertaken by physicians and trained medical technical assistants who need to navigate the slide images manually. This technique helps in prompt diagnosis of bacterial diseases. For example, Gram staining of sputum samples helped specifically identify the pathogens responsible for community acquired pneumonia in some patients [Citation3]. Clinical decisions on infection management such as initial antibiotic choice often rely on Gram stain results [Citation3]. Thus, it is crucial to ensure these tests are conducted and interpreted accurately.
Large language models (LLMs) are advanced artificial intelligence models, able to generate human-like text, with sophisticated natural language processing capabilities [Citation4]. Some of these most recent and advanced models are trained on vast amounts of data and use deep learning techniques to understand complex inputs and produce language [Citation5]. LLMs are trained in a self-supervised setting on large corpora of text and data to learn generic representations, significantly increasing with time model parameters and training data to enhance performance across various tasks [Citation6]. Open AI's Generative Pretrained Transformer (GPT) and Google's Gemini are cutting-edge LLMs that have garnered significant attention since their launch. GPT-4, a successor to GPT-3.5, was introduced in March 2023, while Gemini Pro 1.5 made its debut around the same time [Citation7]. GPT-4 and Gemini 1.5 Pro have made significant advancements in their visual analysis capabilities. The recent release of GPT-4's visual capabilities, known as GPT-4V, has enabled the combined analysis of text and visual data [Citation8]. Historically, visual capabilities in models like GPT-4 Vision, and Google Gemini 1.5 were limited to straightforward image recognition tasks. However, with advancements in these models, their capacity to integrate and interpret complex visual inputs has increased. Most recently, ChatGPT-4 Omni (GPT-4o), released on 13 May 2024, is a natively multimodal model capable of processing text, images, audio and video, providing faster responses and enhanced capabilities compared with its predecessors [Citation8].
Recent studies have shown the potential of LLMs in medical image analysis across various fields like pathology and ophthalmology, demonstrating their ability to interpret complex medical visual data [Citation9–11]. While specific machine learning models have been proposed for automating Gram stain analysis [Citation12–14], there is a notable gap in research evaluating the effectiveness of the latest publicly available LLMs, like GPT-4o and Gemini 1.5 Pro, in accurately identifying bacteria on Gram stains.
Classical laboratory methods for analyzing Gram staining require specialized knowledge and significant experience of microbiologists. Given the critical role of accurate bacterial identification in medical diagnostics and treatment, it is essential to assess the capabilities of cutting-edge technologies like LLMs in Gram stain analysis. Understanding the potential of LLMs, such as GPT-4o and Gemini 1.5 Pro, in correctly analyzing Gram stains and bacterial shapes could help streamline and automate microbiological practices, leading to more efficient diagnoses and management. Therefore, further research is warranted to evaluate the accuracy of the latest publicly available LLMs in this specific domain.
This study aims to assess the raw unprimed capabilities of these two advanced LLMs, GPT-4o and Gemini 1.5 Pro, in accurately distinguishing between Gram-positive and Gram-negative bacteria and to classify them as cocci or bacilli.
2. Materials & methods
This study is a diagnostic accuracy study involving two LLMs, GPT-4o and Gemini 1.5 Pro. The primary outcome measure was the accuracy of each LLM in correctly classifying Gram stains and bacterium shapes simultaneously or separately on the test set. The secondary objectives included assessing the agreement between the LLMs and a gold standard, analyzing confusion matrices to understand the types of errors made, calculating kappa agreements to measure the consistency of the models with the gold standard, and tasking these LLMs in identifying the bacteria genus and species.
A publicly available, labeled database of bacterial Gram stains was used [Citation13]. This database (DIBaS) includes 660 images of 33 different genus and species, composing a variety of Gram-positive and Gram-negative bacteria, classified as either bacilli or cocci. The dataset used for this article's purpose consisted of 80 bacterial samples, divided evenly among four bacteria representing all possible combinations of Gram Stain and bacterial shape: Staphylococcus aureus, Pseudomonas aeruginosa, Neisseria gonorrhoeae and Clostridium perfringens. Each bacterium had 20 images, annotated for genus, species, Gram stain and shape. The images were input into both LLMs in that specific order: 20 Gram-positive cocci, followed by 20 Gram-positive bacilli, then 20 Gram-negative cocci and finally 20 Gram-negative bacilli. Inputting the images in the same order for both GPT-4o and Gemini 1.5 Pro is important for accurately contrasting the cumulative accuracy trends of the two models. This consistency safeguards that differences in performance can be attributed to the models themselves rather than the order of image inserted. The images were subject to preprocessing steps including taking high-resolution screenshots of the images to remove any potential metadata and bias before uploading them into the models. This approach ensured that the evaluation focused solely on the models' inherent capabilities in interpreting Gram stain and bacterial shape without the confounding effects of varying image resolutions or the models' access to any metadata.
The following prompt was used for both LLMs went inputting the images: “Play the role of an expert microbiologist. I will provide you with microscopic images of Gram stains of bacteria to read. Give your answer in JSON format: if the bacteria are Gram-Positive or Gram-Negative, if the bacteria are bacilli, cocci, or diplococci, give your best guess on the bacteria's genus and species” (). The prompt used in this study was a mixture of a “role-play prompt” [Citation15] and “Expert Prompting” [Citation16], shown in studies to be a consistent and effective way of framing tasks for LLMs, enabling them to perform complex and context-specific analyses. By standardizing the prompt, we ensured that both GPT-4o and Gemini 1.5 Pro were evaluated under the same conditions, thus offering a fair comparison of their capabilities. Our study utilized GPT-4o and Gemini 1.5 Pro in their raw, unprimed state. The models were not trained or fine-tuned specifically for Gram stain or bacterial shape identification, which demonstrates their inherent capabilities in handling such tasks without prior domain-specific training.
Figure 1. Examples of input and output for both models.
Examples of inputs (including prompt and Gram stain images from the dataset) with generated JSON output, GPT-4o on the left and Gemini 1.5 Pro on the right.
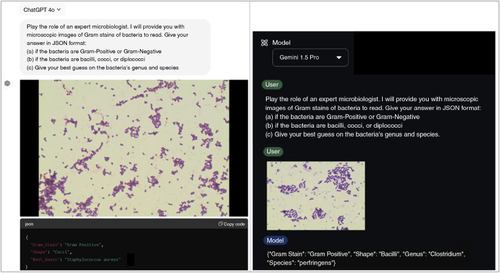
Accuracy was calculated as the proportion of correctly classified images out of the total number of images. Confusion matrices were generated for both LLMs to analyze the distribution of true positives, true negatives, false positives and false negatives. This analysis helped identify specific areas where each model performs well or poorly. The kappa statistic was calculated to measure the agreement between each LLM and the gold standard. The kappa value ranges from -1 to 1, with higher values indicating better agreement. Kappa values were interpreted based on standard guidelines: values <0 indicate no agreement, 0–0.20 slight agreement, 0.21–0.40 fair agreement, 0.41–0.60 moderate agreement, 0.61–0.80 substantial agreement, and 0.81–1.00 almost perfect agreement.
As this study involves secondary analysis of publicly available, de-identified data, it is exempt from institutional review board (IRB) approval. We ensured that the use of the data complies with the terms of use specified by the data provider.
3. Results
The dataset consisted of 80 bacterial samples, divided evenly among four bacteria: Staphylococcus aureus, Pseudomonas aeruginosa, Neisseria gonorrhoeae and Clostridium perfringens. Each bacterium had 20 images, annotated for genus, species, Gram stain and shape ().
Table 1. Distribution of dataset by bacterium genus and species, Gram stain and bacterial shape.
GPT-4o correctly identified both the Gram stain and bacterial shape simultaneously with higher accuracy then Gemini 1.5 Pro (75% vs. 60%, respectively) (). When examining the performance by specific bacteria, GPT-4o achieved 100% accuracy in identifying both the Gram stain and shape correctly for Clostridium perfringens, Pseudomonas aeruginosa and Staphylococcus aureus (). Gemini 1.5 Pro, on the other hand, showed more variability in its performance for the same bacteria (45, 100 and 95%, respectively). However, both LLMs failed to correctly identify the Gram stain and bacterial shape in all cases (0% accuracy) with Neisseria gonorrhoeae.
Figure 2. Accuracy of LLMs in simultaneously identifying Gram stain and bacterial shape. Accuracy was calculated as the proportion of correctly classified images out of the total number of images.
LLM: Large language model.
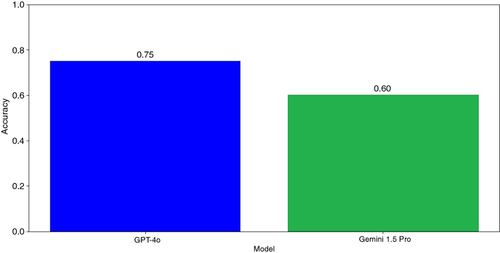
Figure 3. Accuracy of LLMs in identifying both Gram stain and bacterial shape by bacteria. Accuracy was calculated as the proportion of correctly classified images out of the total number of images.
LLM: Large language model.
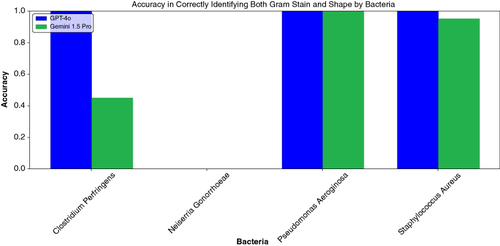
For Gram stain identification alone, GPT-4o achieved a higher accuracy than Gemini 1.5 Pro (80 and 61%, respectively) (). Both LLMs showed high accuracy in identifying bacterial shapes alone (95% for GPT-4o and 99% for Gemini 1.5 Pro).
Figure 4. Accuracy of LLMs in separately identifying Gram stain and bacterial shape. Accuracy was calculated as the proportion of correctly classified images out of the total number of images.
LLM: Large language model.
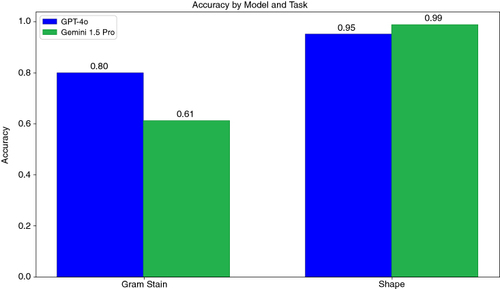
For the Gram stain predictions, GPT-4o demonstrated a high degree of accuracy, with 40 true positives and 24 true negatives. However, there were also 16 false positives (). On the other hand, Gemini 1.5 Pro correctly identified 29 positive cases and 20 negative cases but also had 11 false negatives and 20 false positives. For bacterial shape predictions, GPT-4o correctly identified 40 bacilli and 36 cocci and labeled falsely 4 cocci as bacilli. Gemini 1.5 Pro, in contrast, correctly identified 40 bacilli and 39 cocci, with one incorrect classification of cocci.
Figure 5. Confusion matrices for identifying Gram stain and bacterial shape by LLMs. Confusion matrices were generated for both LLMs to evaluate their performance by comparing the predicted labels with the actual labels. This analysis shows the distribution of true positives, true negatives, false positives and false negatives.
LLM: Large language model.
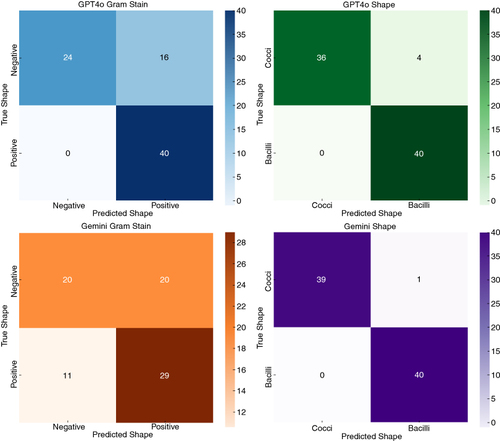
To further evaluate the agreement between the LLMs and the correct identification of Gram stain and bacterial shape, Cohen's kappa statistics were calculated. The Cohen's kappa for GPT-4o's Gram stain prediction was 0.60, indicating moderate agreement with the labeled database, whereas Gemini's kappa was 0.23, suggesting fair agreement. For shape predictions, GPT-4o had a kappa of 0.92 and Gemini had a kappa of 0.76, both indicating substantial agreement.
The cumulative accuracy trends for both LLMs in Gram stain and shape identification were plotted to visualize their performance over the sample set (). The cumulative accuracy plots indicated that GPT-4o consistently performed equally or better in every identification, except for the shape of Neisseria gonorrhoeae.
Figure 6. Cumulative accuracy trends in separately identifying Gram stain and bacterial shape by bacteria. The unlabeled images input into both LLMs was performed in that specific order: 20 Gram-positive cocci, followed by 20 Gram-positive bacilli, then 20 Gram-negative cocci and finally 20 Gram-negative bacilli. The graphs provide a visual representation of how the accuracy of each LLM accumulates over the sequence of image samples. Consistent high values near 1.0 indicate reliable performance, while any drops indicate areas where the LLM's accuracy decreases. Consistent low values near 0 indicate poor performance, while any uptrends indicate areas where the LLM's accuracy increases.
LLM: Large language model.
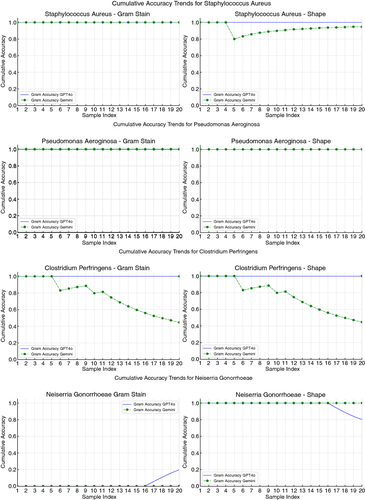
Both LLMs were tasked to identify the specific genus and species based on the Gram stains. For Staphylococcus aureus, GPT-4o identified both genus and species in 20 images, with 100% accuracy. On the other hand, Gemini 1.5 Pro identified the genus correctly in 50% of cases and the species correctly in 10% of cases. For Clostridium perfringens, both GPT-4o and Gemini correctly identified the genus in three images and accurately identified both the genus and species in only one image. Regarding both Neisseria gonorrhoeae and Pseudomonas aeruginosa, both models failed to correctly identify the genus and species in any of the cases.
4. Discussion
To the best of our knowledge, this study represents the first known accuracy analysis of the latest and most advanced visual LLMs, GPT-4o and Gemini 1.5 Pro, in the domain of Gram stain and bacterial shape identification. The novelty of this work lies in applying the technology of visual transformers to a specific technical visual task that has not been previously explored with these advanced LLMs. The results from this study provide valuable insights into the potential and limitations of these LLMs in microbial classification tasks. Both LLMs are highly proficient in identifying bacterial shapes. GPT-4o demonstrated higher accuracy in determining the Gram staining of bacteria alone and higher reliability in simultaneously identifying both Gram stain and shape of bacteria. Both LLMs had significant challenge in accurately classifying Neisseria gonorrhea.
Our study standardized the prompts and used close-ended questions across both models, ensuring consistency in the evaluation process. This standardization helps minimize any biases that could arise from varying input formats, thereby providing a fair interpretation of the LLMs' performances. Also, we used a balanced inclusion of four different bacteria, ensuring an equal distribution of Gram-positive and Gram-negative bacteria and cocci and bacilli shapes. This balanced representation allows for a comprehensive evaluation of the models across different bacterial types. While GPT-4o accurately identified the images of Gram-positive cocci as Staphylococcus aureus, this does not guarantee its ability to correctly identify other Staphylococci species or other Gram-positive cocci. This precise identification might have been a fortunate guess given the higher probability of encountering S. aureus in clinical settings compared with other Staphylococci species or other Gram-positive cocci [Citation17]. Given the nature of large-scale training datasets of LLMs, it is possible that some publicly available images could have been part of their training data. However, if the exact image files from the dataset we used in our study were part of the LLMs training data, we would expect the model to identify not only the Gram stain and shape with high accuracy but also the genus and species with equal precision (since this information was part of the initial images metadata). This indicates that the model is making its predictions based on learned patterns rather than memorized images.
It might not be surprising that both LLMs failed to identify Neisseria gonorrhoeae, as even many microbiologists find it challenging due to its morphological characteristic (diplococci). In fact, Gram staining is highly sensitive and specific in symptomatic males with gonorrheal urethritis [Citation18]. However, its sensitive and specificity decrease in females and asymptomatic males, necessitating the use of additional tests in these cases. Since these LLMs were not provided with clinical context, it might be more difficult to correctly read the Gram stains of N. gonorrhoeae. The failure to identify N. gonorrhoeae by both LLMs could be attributed to the limited representation of this bacterium in the intrinsic training data of the models (which is not something made publicly available by the mother companies). This can indicate a potential shortcoming in the training dataset rather than the model architecture itself. Therefore, future versions of LLMs, such as Med-Gemini, trained on additional sets of specialized medical data may be able to perform better [Citation19].
Our study utilized GPT-4o and Gemini 1.5 Pro in their raw, unprimed state [Citation7,Citation8]. The models were not trained or fine-tuned specifically for Gram stain or bacterial shape identification, which demonstrates their inherent capabilities in handling such tasks without prior domain-specific training. This approach provides a realistic assessment of how these LLMs perform when applied to new, unlearned tasks, showcasing their versatility and potential for generalization. Other studies have developed specialized artificial intelligence models trained to recognize images of specific infectious diseases. For instance, Smith et al. designed a convolutional neural network (CNN) model to classify blood-culture Gram stain [Citation20]. After training, their model achieved strong differentiation capabilities (accuracy of 93.1%) when tasked to identify 4,000 images into 4 different categories: Gram-positive cocci in chains/pairs, Gram-positive cocci in clusters, Gram-negative rods and background (no bacteria). Interestingly, they did not include Gram-negative cocci in their dataset, which is the bacterium type where the LLMs in our study failed the most. Another study aimed at optimizing a smartphone-based deep learning (DL) model for Gram-Stained Image Classification [Citation21]. It showed that for the DL model to be fast and compact, it needs to be tuned and simplified without losing much accuracy. Such DL tool was presented as a successful solution to resource-limited devices, showcasing the need to automate Gram stain readings.
While several studies have evaluated the diagnostic capabilities of Open AI's GPT-4 and GPT-4V in various medical subspecialties [Citation11]; to the best of our knowledge, our study is the first analysis evaluating their newest version, GPT-4o. For example, Pillai et al. investigated the potential of GPT-4V in dermatological diagnosis and treatment recommendations by using 54 images of common skin diseases [Citation11]. The analysis was performed in three setups: Image Prompt (image-based), Scenario Prompt (text-based) and Image and Scenario Prompt (combining both modalities). Their results showed the respective accuracy for each setup: 53%, 89% and 89%, demonstrating that GPT-4V showed promising capabilities, particularly in text-based scenarios, but less so in image-based scenarios alone. Furthermore, Antaki et al. aimed to assess the performance of the Gemini Pro vision language model (VLM) to diagnose macular diseases from 50 optical coherence tomography (OCT) scans [Citation10]. The study showed that the VLM achieved a correct diagnosis in only 34% of the cases.
Despite its strengths, this study has several limitations. One major limitation is the small sample size, with only four different bacteria included. While the equal distribution of Gram stains and shapes helps in providing a balanced evaluation, a larger and more diverse set of bacteria would offer a more robust assessment of the LLMs' capabilities. However, even with a small sample size, our results showed that these LLMs are not ready to accurately characterize both Gram stain and bacterial shape as they failed in 100% of the Gram-negative cocci. Another limitation is that the 80 images used in the study were not input randomly but rather in a specific order: 20 Gram-positive cocci, followed by 20 Gram-positive bacilli, then 20 Gram-negative cocci, and finally 20 Gram-negative bacilli. This sequence does not accurately reflect the randomness of microbiological representation in the real world. Also, the images used for this analysis may not accurately represent the resolution and quality of images observed under a microscope in a clinical setting. Consequently, the LLM's performance may vary when exposed to different image resolutions and qualities, which could affect their accuracy and reliability in real-world applications. Several factors could have influenced the accuracy of GPT-4o and Gemini 1.5 Pro in Gram stain and bacterial shape identification. These include but are not limited to the quality of input images, LLM training data, prompt design, inherent architectural differences of models and the dataset size and diversity we selected. To address these factors, we propose solutions such as higher resolution images and image capture conditions, fine-tuning these models on domain-specific data, experimenting with different prompt designs, using larger and more diverse datasets and conducting validation studies in real-world clinical environments.
These LLMs do not seem to be ready to transition from research tools to clinical laboratories. However, the ongoing enhancements in these models and their training on more extensive medical data may bring us closer to this goal. The promising results from initial studies, including ours, indicate that with further refinement, LLMs could significantly enhance microbiology diagnostics. As the scientific and medical communities continue to explore the potential of these LLMs, further research is essential to unlock their full capabilities [Citation22]. These models may be utilized in the future to prescreen and preclassify image data, thereby boosting productivity and enhancing diagnostic accuracy through collaboration between LLMs and microbiologists. These tools have the potential to provide accurate, efficient and low-cost diagnostic support, ultimately improving patient care and expanding access to advanced diagnostic techniques, mostly in underserved and poorly staffed clinical settings. Once established, image-based LLM analysis can be cost-effective and suitable for both local and remote diagnostic applications.
5. Conclusion
In conclusion, this study provides a pioneering comparative analysis of GPT-4o and Gemini 1.5 Pro in Gram stain and bacterial shape identification. The findings highlight the strengths and limitations of these LLMs, demonstrating their potential for microbial classification tasks without prior domain-specific training. However, the results suggest that these LLMs in their unprimed state are not ready to be implemented in clinical practice. There is a need for further research with larger and more diverse datasets, as well as offline clinical samples, to better understand and enhance the capabilities of these LLMs in clinical microbiology.
Financial disclosure
The authors have no financial involvement with any organization or entity with a financial interest in or financial conflict with the subject matter or materials discussed in the manuscript. This includes employment, consultancies, honoraria, stock ownership or options, expert testimony, grants or patents received or pending, or royalties.
Writing disclosure
No writing assistance was utilized in the production of this manuscript.
Competing interests disclosure
The authors have no competing interests or relevant affiliations with any organization or entity with the subject matter or materials discussed in the manuscript. This includes employment, consultancies, honoraria, stock ownership or options, expert testimony, grants or patents received or pending, or royalties.
References
- Bartholomew JW, Mittwer T. The Gram stain. Bacteriol Rev. 1952;16:1–29. doi:10.1128/br.16.1.1-29.1952
- O'Toole GA. Classic spotlight: how the Gram stain works. J Bacteriol. 2016;198:3128. doi:10.1128/JB.00726-16
- Ogawa H, Kitsios GD, Iwata M, et al. Sputum Gram stain for bacterial pathogen diagnosis in community-acquired pneumonia: a systematic review and Bayesian meta-analysis of diagnostic accuracy and yield. Clin Infect Dis. 2020;71:499–513. doi:10.1093/cid/ciz876
- Skryd A, Lawrence K. ChatGPT as a tool for medical education and clinical decision-making on the wards: case study. JMIR Form Res. 2024;8:e51346. doi:10.2196/51346
- Clusmann J, Kolbinger FR, Muti HS, et al. The future landscape of large language models in medicine. Commun Med. 2023;3:1–8. doi:10.1038/s43856-023-00370-1
- Naveed H, Khan AU, Qiu S, et al. A comprehensive overview of large language models [Internet]. ArXiv. 2024 [ cited 2024 Jun 30]. Available from: http://arxiv.org/abs/2307.06435
- Gemini – chat to supercharge your ideas [Internet]. Gemini. [ cited 2024 Jun 2]. Available from: https://gemini.google.com
- OpenAI [Internet]. [ cited 2024 Jun 2]. Available from: https://openai.com/
- Wang M, Li J. Interpretable predictions of chaotic dynamical systems using dynamical system deep learning. Sci Rep. 2024;14:3143. doi:10.1038/s41598-024-53169-y
- Antaki F, Chopra R, Keane PA. Vision-language models for feature detection of macular diseases on optical coherence tomography. JAMA Ophthalmology [Internet]. 2024 [ cited 2024 Jun 2]. doi:10.1001/jamaophthalmol.2024.1165
- Pillai A, Parappally Joseph S, Hardin J. Evaluating the diagnostic and treatment recommendation capabilities of GPT-4 vision in dermatology [Internet]. 2024 [ cited 2024 Jun 2]. doi:10.1101/2024.01.24.24301743
- García RG, Rodríguez SJ, Martínez BB, et al. Efficient deep learning architectures for fast identification of bacterial strains in resource-constrained devices [Internet]. ArXiv. 2021 [ cited 2024 Jun 1]. Available from: http://arxiv.org/abs/2106.06505
- Zieliński B, Plichta A, Misztal K, et al. Deep learning approach to bacterial colony classification. PLOS ONE. 2017;12:e0184554. doi:10.1371/journal.pone.0184554
- Kim H, Ganslandt T, Miethke T, et al. Deep learning frameworks for rapid gram stain image data interpretation: protocol for a retrospective data analysis. JMIR Research Protocols. 2020;9:e16843. doi:10.2196/16843
- Kong A, Zhao S, Chen H, et al. Better zero-shot reasoning with role-play prompting [Internet]. ArXiv. 2024 [ cited 2024 Jun 30]. Available from: http://arxiv.org/abs/2308.07702
- Xu B, Yang A, Lin J, et al. ExpertPrompting: instructing large language models to be distinguished experts [Internet]. ArXiv. 2023 [ cited 2024 Jun 30]. Available from: http://arxiv.org/abs/2305.14688
- Tong SYC, Davis JS, Eichenberger E, et al. Staphylococcus aureus infections: epidemiology, pathophysiology, clinical manifestations, and management. Clin Microbiol Rev. 2015;28:603–661. doi:10.1128/CMR.00134-14
- Goodhart ME, Ogden J, Zaidi AA, et al. Factors affecting the performance of smear and culture tests for the detection of Neisseria gonorrhoeae. Sex Transm Dis. 1982;9:63–69. doi:10.1097/00007435-198204000-00002
- Saab K, Tu T, Weng W-H, et al. Capabilities of Gemini models in medicine [Internet]. ArXiv. 2024 [ cited 2024 Jun 30]. Available from: http://arxiv.org/abs/2404.18416
- Smith KP, Kang AD, Kirby JE. Automated interpretation of blood culture gram stains by use of a deep convolutional neural network. J Clin Microbiol. 2018;56(3):e01521-17. doi:10.1128/jcm.01521-17
- Kim HE, Maros ME, Siegel F, et al. Rapid convolutional neural networks for gram-stained image classification at inference time on mobile devices: empirical study from transfer learning to optimization. Biomedicines. 2022;10:2808. doi:10.3390/biomedicines10112808
- Smith KP, Kirby JE. Image analysis and artificial intelligence in infectious disease diagnostics. Clin Microbiol Infection. 2020;26:1318–1323. doi:10.1016/j.cmi.2020.03.012