ABSTRACT
Background
Von Willebrand disease (VWD) is underdiagnosed, often delaying treatment. VWD claims coding is limited and includes no severity qualifiers; improved identification methods for VWD are needed. The aim of this study is to identify and characterize undiagnosed symptomatic persons with VWD in the US from medical insurance claims using predictive machine learning (ML) models.
Research design and methods
Diagnosed and potentially undiagnosed VWD cohorts were defined using Komodo longitudinal US claims data (January 2015-March 2020). ML models were built using key characteristics predictive of VWD diagnosis from the diagnosed cohort. Two ML models predicted VWD diagnosis with the highest accuracy in females (random forest; 84%) and males (gradient boosting machine; 85%). Undiagnosed persons suspected to have VWD were identified using an 80% cutoff probability; profiles of key characteristics were constructed.
Results
The trained ML models were applied to the undiagnosed cohort (28,463 females; 20,439 males) with suspected VWD. Fifty-two percent of undiagnosed females had heavy menstrual bleeding, a key pre-diagnosis symptom. Undiagnosed males tended to have more frequent medical procedures, hospitalizations, and emergency room visits compared with undiagnosed females.
Conclusions
ML algorithms successfully identified potentially undiagnosed symptomatic people with VWD, although many may remain undiagnosed and undertreated. External validation of the algorithms is recommended.
1. Introduction
Von Willebrand disease (VWD) is the most common inherited bleeding disorder, affecting up to 1% of the general population, while only about 0.01% of the general population has clinically significant bleeding symptoms [Citation1]. Type 1 VWD is the mildest form and most common VWD type, whereas Types 2 and 3, which represent the most severe phenotypes, are much rarer [Citation2]. Persons with Type 1 VWD exhibit a mild to moderate bleeding phenotype, those with Type 2 exhibit variable bleeding, and those with Type 3 experience severe bleeding. A Type 1 diagnosis is defined by von Willebrand factor (VWF) levels <30 IU/dL, while ‘low VWF’ includes those with VWF levels of 0.30–0.50 IU/dL. Low VWF levels have been shown to be associated with significant bleeding, especially in the menorrhagia and dental domains [Citation3,Citation4].
Once diagnosed, persons with VWD can be successfully managed and treated with plasma-derived VWF concentrates or recombinant VWF [Citation1]. However, VWD is often under- or misdiagnosed in symptomatic people, which delays optimal bleeding treatment for persons with VWD [Citation1,Citation5,Citation6]. Bleeding phenotypes are associated with high healthcare resource utilization; a study by the Hemophilia Utilization Group Studies (HUGS) examined healthcare utilization in persons with VWD and found that persons with documented bleeding in the previous 6 months had a significantly higher rate of medical procedures and overnight hospitalizations and a higher proportion of emergency room (ER) and physician visits compared with those who did not have documented bleeding events during that period [Citation7]. Moreover, persons with VWD with low VWF were diagnosed and received VWD treatment at an older age and reported reduced quality of life compared with persons with Type 1–3 VWD, highlighting that low VWF is not necessarily a mild disorder compared with other VWD subtypes and that there is a need for earlier identification and diagnosis of persons with milder reductions of VWF [Citation7].
The 2021 international guidelines on the diagnosis of VWD stress the importance of ensuring accurate and timely diagnosis of patients [Citation3]. A claims study in 2017 showed that 66% of undiagnosed or ‘serially misrecognized’ persons with VWD sought care for at least three bleeds in the year before diagnosis (compared with 14% of all patients) and reported lower bleed frequency after diagnosis; thus, timely diagnosis and appropriate treatment could potentially alleviate the clinical and economic burden of VWD on patients and the healthcare system [Citation8].
Diagnosis of bleeding disorders has been reported to be more delayed in females than in males [Citation9]. Females are disproportionately affected by symptoms related to bleeding because of reproductive tract bleeding such as heavy menstrual bleeding (HMB) and postpartum hemorrhage [Citation10]. In a survey of hemophilia treatment centers across the United States, 62% of women diagnosed with VWD were reported to have HMB [Citation11]. Women with VWD live with symptoms such as HMB for an average of 16 years from the first symptom until diagnosis, often well into adulthood [Citation12].
The most common symptoms of VWD involve mucocutaneous bleeding such as HMB, epistaxis, postoperative bleeding, gastrointestinal bleeding, bruising, prolonged bleeding from wounds, and other internal bleeds. However, these symptoms are also prevalent among the healthy population; thus, it may be difficult to establish a VWD diagnosis based on bleeding symptoms, especially in persons with Type 1 VWD and low VWF [Citation10,Citation13,Citation14]. Thus, the diagnosis can be made or excluded based on the results of VWF testing.
Present methodology is inadequate to identify people at risk for VWD, as current International Classification of Diseases (ICD) Ninth/Tenth Revision codes only identify those patients with a VWD diagnosis [Citation15]. Machine learning approaches may be useful to predict the diagnosis of persons with rare diseases, thus clarifying the prognosis and improving treatment and, potentially, the quality of care [Citation16]. Therefore, a machine learning model that can help identify persons with VWD earlier, allowing for timely treatment initiation, would be helpful. This study focused on addressing the high unmet need for timely VWD diagnosis with the development of a machine learning approach using real-world claims data.
In the United States, all medical encounters (e.g. emergency, diagnoses, prescriptions, inpatient or virtual visits) for patients with insurance coverage result in an insurance claim or a request for reimbursement from healthcare providers for providing a service to the patient. These claims are reviewed by claims processors, and these data can be mined and used for administrative claims database (also called billing data) studies. Health claims have become a popular source of data for healthcare analytics, with numerous applications ranging from disease burden estimation and policy evaluation to drug event detection and advanced predictive analytics [Citation17].
Sociodemographic and clinical characteristics were used to develop a predictive model to identify and characterize potentially undiagnosed symptomatic persons with VWD in the United States. This is the first step in developing a machine learning algorithm that may support the timely diagnosis of VWD, thus potentially improving patients’ quality of care.
2. Patients and methods
The four main phases of the machine learning approach used to develop a predictive patient finding model are depicted in and described below.
Figure 1. Key project phases of the machine learning approach to identify potentially undiagnosed symptomatic patients with VWD. HCP, healthcare professional; VWD, von Willebrand disease.
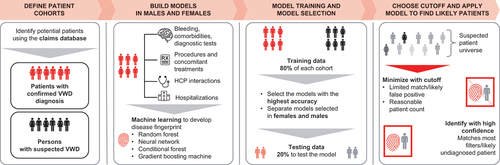
2.1. Patient selection and definition of cohorts
Sociodemographic and clinical characteristics and medical history data were sourced from the Komodo Health comprehensive dataset. Komodo includes longitudinal claims data from 150 million ‘payer complete’ profiles, >150 payers, 330 million patient encounters, and direct payer relationships [Citation18]. ‘payer complete’ or ‘closed’ (as opposed to ‘open’) claims data refer to information from payers that captures full medical or pharmacy transactions of each patient in a given time period. If only partial transactions are available for a patient, the data are considered ‘open’ for that patient. ‘Closed’ claims are reflective of completed patient encounters, representing a comprehensive view of the care provided and associated costs [Citation19].
The Komodo Health dataset includes both medical and pharmacy claims from Medicare and Medicaid, as well as commercial payers, reflecting a wide range of diverse patient populations [Citation18].
Predictors based on the Komodo Healthcare Map data (2015–2019) used to populate the models were obtained from demographics, bleeding, place of service, diagnostic tests, anemia diagnosis, blood transfusion, and comorbidity data (). Since Komodo data are sourced from a large number of diverse payers (>150 complete payer datasets), the data are well distributed across geographical and demographic factors. Data from the Komodo database are based on real-world data and are obtained void of personal identification information (i.e. blinded).
Table 1. Main predictors used to develop the prediction model.
Two cohorts, symptomatic diagnosed and symptomatic undiagnosed (suspected to have VWD), were defined using closed claims from the database (January 2015 through March 2020). ‘Closed claims’ from the Komodo database were derived directly from insurance providers and reveal nearly all of the patients’ healthcare activities during a specific period of enrollment; healthcare encounters paid by the patient out of pocket were not included. No additional filtering or selection was done. All diagnosis positions (primary and secondary) were used when searching for diagnoses.
The diagnosed cohort included patients who had VWD-related diagnoses and/or treatment claims, as well as claims that identify signs and symptoms of bleeding prior to diagnosis. On the other hand, the symptomatic undiagnosed cohort included patients who had relevant symptoms but did not have a VWD diagnosis. Selection criteria are included in . Specific codes evaluated for all selection criteria can be found in Supplementary Table S1 (ICD), Supplementary Table S2 (J-code), and Supplementary Table S3 (Current Procedural Terminology).
Table 2. Selection criteria.
2.2. Building of the model database and validation of the dataset
Machine learning was used to develop a disease fingerprint of confirmed persons with VWD by identifying variables to uniquely characterize them, including comorbidities, interaction with healthcare professionals and procedures, concomitant prescriptions, and hospitalizations. Of the 9285 confirmed, diagnosed persons with VWD, 5981 (64%) symptomatic persons had at least one bleeding code claim within 2 years before diagnosis, and 3304 (36%) asymptomatic persons had no bleeding code in the 2 years before diagnosis; 826 (9%) of these asymptomatic patients had diagnostic tests, and 2478 (27%) patients had no bleeding or test in the 2 years before diagnosis. These patients (3304) were excluded when training the model. Further analysis across all histories was conducted to validate the dataset and understand how those with no bleeding or tests appeared with a VWD diagnosis. When examining the entire history of confirmed, diagnosed persons with VWD, 7897 (85%) had at least one bleeding code claim, and 1388 (15%) had no bleeding code. Of the 15% with no bleeding code in all history, 604 (7%) had diagnostic tests, and relatively few (784 [8%]) had no bleeding or test; reasons for not having a bleeding or test code in all history can be hypothesized, such as discrepancies in data capture, but overall these findings validate the dataset. Following this, a population of 5981 confirmed, diagnosed persons with VWD population was used to train the models.
2.3. Model training and model selection
Four different machine learning models were built and trained on the confirmed VWD patient cohort: random forest model (RFM), neural network, conditional forest, and gradient boosting machine (GBM). All four models were applied to the cohort of patients with suspected VWD (i.e. symptomatic, undiagnosed). The criteria used to select patients for model training and prediction are described in Supplementary Table S4. Machine learning models were applied separately for males and females since the timing and presentation of bleeding symptoms may vary depending on sex.
There were no missing data for demographic variables. For all other variables regarding medical history (e.g. comorbidities, treatment, specialty visits), the value inputted was ‘0’ if no records were available.
An a priori decision was that the overall best performing model will be determined by evaluating the sensitivity (i.e. true positive rate) and precision (i.e. positive predictive value [PPV]) of each model. All available coding history was evaluated. Key patient characteristics that were highly predictive of VWD diagnosis were identified in persons with a confirmed VWD diagnosis.
Two models (one in males and one in females) with the highest accuracy were selected to predict the people who are symptomatic and undiagnosed. An example of how the best-performing model was selected, including calculation of overall accuracy and determination of cutoff, is provided in . Both confirmed and suspected groups of both males and females were down-sampled to 10,000 patients before training and testing the models. To generate training and testing data, a random sampling of 80% of the symptomatic diagnosed cohort and 80% of the symptomatic undiagnosed cohort were put into training data. The remaining 20% of each cohort were combined into testing data.
Table 3. Models and cutoff probability applied in male and female patients of undiagnosed cohort.
The RFM was chosen for females (overall accuracy, 84%; PPV, 93%; sensitivity, 73%) and the GBM for males (overall accuracy, 85%; PPV, 92%; sensitivity, 77%). An 80% cutoff probability of a person having VWD was selected considering the balance between the number of patients and PPV for both of the selected machine learning models. This helps to minimize the possibility of false positives and maintains high accuracy in predicting persons with VWD. Using a cutoff value of 80%, confidence interval levels were 98.3% for males and 97.9% for females. Next, the trained model was applied to the full suspected cohort to identify the predicted patients.
Profiles of the highly predictive characteristics of VWD were constructed for males and females suspected to have VWD.
2.4. Cutoff selection and application of models
2.4.1. Statistical methods
The statistical programs used to build and apply the machine learning models were Python, SQL (Structured Query Language) for data cleaning, data manipulation, and database creation and R for modeling.
2.4.2. Using the prediction model
For a new group of patients suspected to have VWD, the database was constructed according to the variable list, inserted into the trained and validated model to obtain the outcome (between 0 and 1), and then compared with the cutoff value of 80% to determine the final prediction result.
3. Results
3.1. Number and age distribution of persons suspected to have VWD
The VWD symptomatic diagnosed cohort with prediagnosis symptoms included 5981 patients; the symptomatic undiagnosed cohort included 4,869,518 people suspected to have VWD (). Based on the predicted results, the number of US patients suspected to have VWD was 48,902 (28,463 female; 20,439 male; ). The average age of females suspected to have VWD was higher (33 years) than that of males (21 years). Out of the 247 persons taking rVWF, 31 had hemophilia A. Notably, a higher percentage of identified males suspected to have VWD (44%) were in the younger age bracket (2–11 years old) compared with 5% of females ().
3.2. Profiles of key characteristics in male and female persons suspected to have VWD
The key characteristics of symptomatic undiagnosed persons suspected to have VWD are included in .
Figure 3. Key characteristics of symptomatic patients suspected to have undiagnosed VWD. aRespiratory bleeding includes: epistaxis, hemorrhage from throat, hemorrhage from respiratory passages, unspecified, hemorrhage from other sites in respiratory passages, hemoptysis. bBlood chemistry panel tests include: collection of venous blood by venipuncture, blood count, comprehensive metabolic panel, lipid panel, basic metabolic panel, ferritin, hemoglobin glycosylated (A1c), iron, iron binding capacity; hemoglobin. cThe products used to treat bleeding are not mutually exclusive; patients may have received combinations of treatments. DDAVP, 1-deamino-8-D-arginine vasopressin; ER, emergency room; FVIII:C, factor VIII coagulant activity; IUD, intrauterine device; PTT, partial thromboplastin time; VWD, von Willebrand disease; VWF, von Willebrand factor; VWF:Ag, von Willebrand factor antigen; VWF:RCo, VWF ristocetin cofactor.
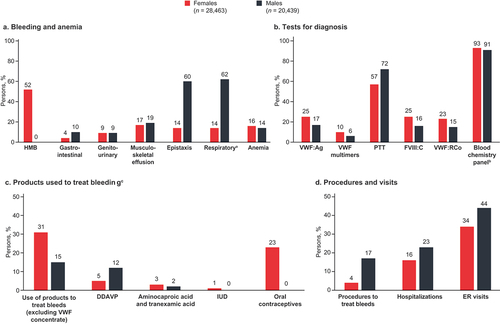
3.2.1. Bleeding types
Among the people suspected to have VWD, greater than half (52%) of the females were diagnosed with HMB. The rates of reported epistaxis and respiratory bleeding were more than four times higher for males (60% and 62%, respectively) than for females (14% and 14%). Anemia was diagnosed similarly for females (16%) and males (14%). Note that respiratory bleeding included epistaxis, hemorrhage from the throat, hemorrhage from respiratory passages, unspecified, hemorrhage from other sites in respiratory passages, and hemoptysis. Other bleeding symptoms included gastrointestinal (up to 10%), genitourinary (9%), and musculoskeletal (up to 19%) bleeding ().
3.2.2. Diagnostic tests
Partial thromboplastin time (PTT) and blood chemistry panel testing were the most commonly used diagnostic tests among both males and females. Other less commonly used tests included VWF antigen testing, VWF multimer analysis, and VWF ristocetin cofactor functional assays. Notably, there were no noticeable differences between males and females when evaluating the diagnostic testing performed on the symptomatic undiagnosed people suspected to have VWD ().
3.2.3. Products used to treat bleeding
Claims for desmopressin, aminocaproic acid, tranexamic acid, oral contraceptives, and hormonal intrauterine devices were included to evaluate products used to treat bleeding. Twice as many females (31%) compared with males (15%) used products to treat bleeding (not including VWF concentrate). These treatments included oral contraceptives (23%) and intrauterine devices (1%) in females, DDAVP (12% of males; 5% of females), and antifibrinolytics such as aminocaproic acid or tranexamic acid (2% of males; 3% of females; ).
3.2.4. Procedures, hospitalization, and ER visits to treat bleeding
In terms of resource utilization, ER visits were most frequently reported, followed by hospitalization and procedures that treat bleeds. Overall, a greater percentage of males underwent procedures were hospitalized and visited the ER related to their bleeding events compared with females ().
4. Discussion
4.1. Machine learning approach to identify persons suspected to have VWD
The conventional approach for the diagnosis of VWD and its subtypes is complex and time-consuming and can be challenging [Citation20]. Accurate and swift diagnosis of VWD and its subtypes is challenged by several factors, including poor understanding of differences between normal and abnormal bleeding, lack of clarity around an appropriate diagnostic approach, and access to and expertise about specialized laboratory tests [Citation3]. The delay in VWD diagnosis from the onset of bleeding symptoms can be 15 years or more, which translates to treatment delays [Citation3]. Moreover, there can be further testing-related delays and feasibility issues among individuals with Type 1 disease with borderline VWF levels (0.3–0.5 IU/mL) that warrant expensive repeat specialized coagulation testing to confirm the diagnosis [Citation3].
Several studies have shown that machine learning approaches [Citation6,Citation16] or other modeling approaches [Citation21,Citation22] using real-world data can help to predict VWD diagnosis in patients suspected to have VWD with high accuracy. The predictive model approach presented here can help identify potentially undiagnosed symptomatic people who could be screened for a diagnosis of VWD. Results of this study indicate that high-accuracy machine learning algorithms can help predict VWD diagnosis among symptomatic females and males. Moreover, unlike other machine learning approaches, this study developed and utilized sex-specific predictive models to account for sex-specific differences in bleeding phenotype between males and females (i.e. HMB, postpartum hemorrhage) [Citation9].
Earlier diagnosis of VWD means patients can have access to timely and accurate treatment, improve their health-related quality of life, and mitigate detrimental long-term outcomes [Citation7,Citation9,Citation23]. Identifying highly predictive characteristics of a disease can represent a unique profile or disease ‘fingerprint,’ and screening of suspected patients for these characteristics may facilitate a quick and accurate diagnosis [Citation24].
Here, two different models were identified to predict VWD diagnosis in males (GBM) and females (RFM) with high accuracy, which may reflect differences in the proportion of key characteristics between the two sexes. Symptoms of VWD in the cohort with suspected VWD included anemia (males, 14%; females, 16%; ). Notably, 52% of females with undiagnosed VWD were diagnosed with HMB (). This is in line with a study that examined bleeding patterns in patients before and after VWD diagnosis using a US medical claims database, which demonstrated that prior to diagnosis, 41% to 65% had claims for HMB [Citation25]. Acute HMB and anemia may mask VWD diagnosis because VWF levels may be elevated when testing during these events [Citation22]. In addition, HMB is a predictive symptom of VWD, but it is also the most common bleeding symptom reported in healthy females [Citation26,Citation27]. Finally, females have generally been shown to be referred more often for treatment of sex-specific bleeding symptoms (77% of females ages 12 and older) and experience a longer diagnostic delay than males (11.6 ± 16.4 years vs 7.7 ± 16.6 years for males; p = 0.002) [Citation9]. This is in line with our study demonstrating that a higher percentage (44%) of undiagnosed males suspected to have VWD were in the younger age bracket (2–11 years old) compared with 5% of females (). A US-based study reported that less than 20% of postpubescent and adolescent girls with HMB undergo screening for VWD, despite recommendations by the American College of Obstetricians and Gynecologists [Citation26]. In our study, symptomatic undiagnosed male patients tended to undergo more procedures to stop bleeding and had more hospitalizations and ER visits compared with females ().
A substantial proportion of potentially undiagnosed symptomatic persons with VWD present clear indicators of the disease but remain undiagnosed. A study by HUGS identified that the average age at VWD diagnosis and treatment increased as disease severity decreased [Citation7], which signifies that patients with mild to moderate disease experience longer delays in diagnosis and treatment. A study based in the Netherlands compared the attributes of autosomal inherited bleeding disorders between males and females. Females had a longer diagnostic delay compared with males, despite having bleeding symptoms. This can cause a lack of timely, disease-appropriate treatment for bleeding episodes or prophylaxis for surgery and can negatively impact quality of life [Citation9]. Moreover, the majority of these females needed endometrial ablation or curettage (16.7%), hysterectomy (11.6%), blood transfusion (7%), or iron supplements (41.5%) to manage HMB-related complications. Also, 50% of these females who had at least one child had postpartum hemorrhage, and 20% needed a blood transfusion [Citation9]. Females referred to gynecologists for HMB refractory to conventional treatments have a high chance of undergoing hysterectomy (43%); this includes patients with undiagnosed bleeding disorders. Thus, timely diagnosis can mitigate unnecessary risks of complications in females with VWD [Citation23].
A retrospective medical claims analysis reported that an accurate diagnosis can have a positive impact on persons with VWD in terms of disease burden and highlighted the importance of a systematic approach for accurate VWD diagnosis [Citation8]. Claims for presenting to hospitalists or ER physicians were more common in patients prior to diagnosis than in diagnosed patients [Citation8]. Furthermore, misrecognition of VWD was common, leading to frequent episodic bleeding and 25% of patients visiting the same specialist type to treat a bleed prior to diagnosis [Citation8]. Finally, claims and physician visits related to bleeding were reduced after a confirmed VWD diagnosis, underscoring the importance of timely diagnosis [Citation8].
This machine learning algorithm indicated that the cohort with suspected VWD had fewer claims for VWD confirmation tests (≤25%) recommended by the 2021 international guidelines [Citation3] than for blood chemistry (>90%) or PTT tests (female, 57%; male, 72%; ). In the majority of VWD cases, PTT is normal, and simple chemistry tests do not generally aid in diagnosis. Thus, a machine learning approach can help identify patients who likely have VWD on a population level, thereby prompting earlier VWF testing.
4.2. Study limitations and next steps
This machine learning approach is based on administrative claims data, which may not fully represent real-world diagnosis rates (e.g. persons with VWD not coded for VWD claims, persons without VWD miscoded as persons with VWD). In addition, two different models were used to predict the key characteristics in males and females; this should be considered when comparing the differences in the proportion of key characteristics between the two sexes. This machine learning model has not been tested with any datasets outside of the United States. However, it could be applied in the same manner if the datasets contain the same level of information (coding for procedures, medication, etc). Finally, the accuracy and potential for the real-world application of the machine learning algorithms developed in this study should be externally validated using other data sources.
5. Conclusion
A high-accuracy machine learning algorithm was developed to identify males and females with potentially undiagnosed symptomatic VWD based on real-world data on the unique characteristics of persons diagnosed with VWD. This is the first step in developing a machine learning algorithm that may support the timely diagnosis of VWD, thus potentially improving the quality of care of patients. The bleeding symptoms and diagnostic test claims profile may indicate an absence of suitable protocols or a lack of access to specialized VWD testing for persons with clear indicators of a potential VWD diagnosis. Further external validation using other databases for VWD diagnosis is needed.
Declaration of interest
R.F. Sidonio Jr has received paid compensation for consultancy and research funding from Takeda and Octapharma, and for consultancy from Catalyst, Guardian Therapeutics, Sanofi/Sobi, Bayer, Novo Nordisk, BioMarin, Pfizer, and Genentech. R.F. Sidonio Jr also has investigator-initiated funding for a study in VWD (ATHN 9). A. Lu has received funding for this work from Takeda Pharmaceuticals U.S.A. S. Hale, J. Caicedo, M. Bullano, and S. Xing are employees of Takeda Pharmaceuticals U.S.A., Inc., Lexington, MA, USA. and are holders of Takeda stock. The authors have no other relevant affiliations or financial involvement with any organization or entity with a financial interest in or financial conflict with the subject matter or materials discussed in the manuscript apart from those disclosed.
Komodo Health, Inc. makes no representation or warranty as to the accuracy or completeness of the data set forth herein and shall have, and accept, no liability of any kind, whether in contract, tort (including negligence) or otherwise, to any third party arising from or related to use of the Komodo Materials by Takeda Pharmaceuticals U.S.A. Any use which Takeda Pharmaceuticals U.S.A. or a third party makes of the Komodo Materials, or any reliance on it, or decisions to be made based on it, are the sole responsibilities of Takeda Pharmaceuticals U.S.A. and such third party. In no way shall any data appearing in the Komodo Materials amount to any form of prediction of future events or circumstances, and no such reliance may be inferred or implied.
Reviewer disclosures
Peer reviewers on this manuscript have no relevant financial or other relationships to disclose.
Author contributions
R.F. Sidonio Jr provided critical insights into study design and disease characteristics. A. Lu designed and performed the data analysis. S. Hale, J. Caicedo, M. Bullano, and S. Xing provided insights into the study design and data analysis. All authors contributed to the development of the manuscript and approved the final version of the manuscript.
Previous presentations
Poster (1147) presented at the American Society of Hematology Annual Meeting and Exposition; New Orleans, LA, U.S.A.; December 10–13, 2022.
Supplemental Material
Download MS Word (55 KB)Acknowledgment
Shuai Zhang and Imrran Halari, both employees of Charles River Associates, contributed to the methodology of the analysis and were funded by Takeda Pharmaceuticals U.S.A., Inc., Lexington, MA, USA. Medical writing support was provided by Zela Keuylian, an employee of Parexel, and was funded by Takeda Pharmaceuticals U.S.A., Inc.
Supplementary material
Supplemental data for this article can be accessed online at https://doi.org/10.1080/17474086.2024.2354925
Additional information
Funding
References
- Castaman G, Linari S. Obstacles to early diagnosis and treatment of inherited von Willebrand disease: current perspectives. J Blood Med. 2021;12:165–175. doi: 10.2147/JBM.S232758
- Favaloro EJ. Rare forms of von Willebrand disease. Ann Transl Med. 2018;6(17):345. doi: 10.21037/atm.2018.09.10
- James PD, Connell NT, Ameer B, et al. ASH ISTH NHF WFH 2021 guidelines on the diagnosis of von Willebrand disease. Blood Adv. 2021;5(1):280–300. doi: 10.1182/bloodadvances.2020003265
- Lavin M, O’Donnell JS. How I treat low von Willebrand factor levels. Blood. 2019;133(8):795–804. doi: 10.1182/blood-2018-10-844936
- Colonne CK, Reardon B, Curnow J, et al. Why is misdiagnosis of von Willebrand disease still prevalent and how can we overcome it? A focus on clinical considerations and recommendations. J Blood Med. 2021;12:755–768. doi: 10.2147/JBM.S266791
- Sidonio RF Jr, Zia A, Fallaize D. Potential undiagnosed VWD or other mucocutaneous bleeding disorder cases estimated from private medical insurance claims. J Blood Med. 2020;11:1–11. doi: 10.2147/JBM.S224683
- Roberts JC, Kulkarni R, Kouides PA, et al. Healthcare utilization and health related quality of life in persons with von Willebrand disease. Blood. 2020;136(Supplement 1):3–4. doi: 10.1182/blood-2020-143055
- Sidonio RF, Haley KM, Fallaize D. Impact of diagnosis of von Willebrand disease on patient outcomes: analysis of medical insurance claims data. Haemophilia. 2017;23(5):743–749. doi: 10.1111/hae.13292
- Atiq F, Saes JL, Punt MC, et al. Major differences in clinical presentation, diagnosis and management of men and women with autosomal inherited bleeding disorders. EClinicalMedicine. 2021;32:100726. doi: 10.1016/j.eclinm.2021.100726
- Nichols WL, Hultin MB, James AH, et al. von Willebrand disease (VWD): evidence-based diagnosis and management guidelines, the National Heart, Lung, and Blood Institute (NHLBI) Expert Panel report (USA) 1. Haemophilia. 2008;14(2):171–232. doi: 10.1111/j.1365-2516.2007.01643.x
- Ragni MV, Machin N, Malec LM, et al. Von Willebrand factor for menorrhagia: a survey and literature review. Haemophilia. 2016;22(3):397–402. doi: 10.1111/hae.12898
- Kirtava A, Crudder S, Dilley A, et al. Trends in clinical management of women with von Willebrand disease: a survey of 75 women enrolled in haemophilia treatment centres in the United States. Haemophilia. 2004;10(2):158–161. doi: 10.1046/j.1351-8216.2003.00832.x
- Baronciani L, Peyvandi F. How we make an accurate diagnosis of von Willebrand disease. Thromb Res. 2020;196:579–589. doi: 10.1016/j.thromres.2019.07.010
- James PD, Goodeve AC. von Willebrand disease. Genet Med. 2011;13(5):365–376. doi: 10.1097/GIM.0b013e3182035931
- Lee SJ. Re: Request for new ICD-10-CM codes for von Willebrand disease. 2020 [cited 2022 Dec 12]. Available from: https://www.hematology.org/-/media/hematology/files/advocacy/testimony-and-correspondence/2020/ash-letter-re-wvd-icd-10-codes.pdf
- Schaefer J, Lehne M, Schepers J, et al. The use of machine learning in rare diseases: a scoping review. Orphanet J Rare Dis. 2020;15(1):145. doi: 10.1186/s13023-020-01424-6
- Konrad R, Zhang W, Bjarndottir M, et al. Key considerations when using health insurance claims data in advanced data analyses: an experience report. Health Syst (Basingstoke). 2019;9(4):317–325. doi: 10.1080/20476965.2019.1581433
- Komodo. Healthcare map: the most complete view of the patient. 2022 [cited 2022 Dec 12]. Available from: https://www.komodohealth.com/technology/healthcare-map
- Baser O, Samayoa G, Yapar N, et al. Use of open claims vs closed claims in health outcomes research. J Health Econ Outcomes Res. 2023;10(2):44–52. doi: 10.36469/jheor.2023.87538
- Roberts JC, Christopherson PA, Morateck PA, et al. A von Willebrand factor (VWF) functional screening assay and its correlation with conventional clinical assays of VWF function. Blood. 2014;124(21):4239. doi: 10.1182/blood.V124.21.4239.4239
- Bucciarelli P, Siboni SM, Stufano F, et al. Predictors of von Willebrand disease diagnosis in individuals with borderline von Willebrand factor plasma levels. J Thromb Haemost. 2015;13(2):228–236. doi: 10.1111/jth.12799
- Brown MC, White MH, Friedberg R, et al. Elevated von Willebrand factor levels during heavy menstrual bleeding episodes limit the diagnostic utility for von Willebrand disease. Res Pract Thromb Haemost. 2021;5(4):e12513. doi: 10.1002/rth2.12513
- Djukic SM, Lekovic D, Jovic N, et al. Unnecessary hysterectomy due to menorrhagia and disorders of hemostasis: an example of overuse and excessive demand for medical services. Front Pharmacol. 2016;7:507. doi: 10.3389/fphar.2016.00507
- Guncar G, Kukar M, Notar M, et al. An application of machine learning to haematological diagnosis. Sci Rep. 2018;8(1):411. doi: 10.1038/s41598-017-18564-8
- Roberts JC, Malec LM, Halari I, et al. Bleeding patterns in patients before and after diagnosis of von Willebrand disease: analysis of a US medical claims database. Haemophilia. 2022;28(1):97–108. doi: 10.1111/hae.14448
- Jacobson AE, Vesely SK, Koch T, et al. Patterns of von Willebrand disease screening in girls and adolescents with heavy menstrual bleeding. Obstet Gynecol. 2018;131(6):1121–1129. doi: 10.1097/AOG.0000000000002620
- Spradbrow J, Letourneau S, Grabell J, et al. Bleeding assessment tools to predict von Willebrand disease: utility of individual bleeding symptoms. Res Pract Thromb Haemost. 2020;4(1):92–99. doi: 10.1002/rth2.12256