ABSTRACT
Introduction
Artificial intelligence (AI) that surpasses human ability in image recognition is expected to be applied in the field of gastrointestinal endoscopes. Accordingly, its research and development (R &D) is being actively conducted. With the development of endoscopic diagnosis, there is a shortage of specialists who can perform high-precision endoscopy. We will examine whether AI with excellent image recognition ability can overcome this problem.
Areas covered
Since 2016, papers on artificial intelligence using convolutional neural network (CNN in other word Deep Learning) have been published. CNN is generally capable of more accurate detection and classification than conventional machine learning. This is a review of papers using CNN in the gastrointestinal endoscopy area, along with the reasons why AI is required in clinical practice. We divided this review into four parts: stomach, esophagus, large intestine, and capsule endoscope (small intestine).
Expert opinion
Potential applications for the AI include colorectal polyp detection and differentiation, gastric and esophageal cancer detection, and lesion detection in capsule endoscopy. The accuracy of endoscopic diagnosis will increase if the AI and endoscopist perform the endoscopy together.
1. Introduction
With convolutional neural network (CNN) technology, high-speed computers, and abundant digital data, the image recognition capability of artificial intelligence (AI) has surpassed that of humans. In medical practice, diagnostic imaging support is the area most suited for the practical use of AI.
This paper discusses the latest research findings on CNN-based imaging diagnostic AI for detecting gastrointestinal tract lesions. A CNN is a type of machine learning model associated with accuracy and is the de facto standard in the field of image recognition. Therefore, we focused on CNN-based, clinically relevant, and physician-oriented studies in this review. A summary of the contemporary and future AI applications for diagnosing diseases affecting the stomach, esophagus, large intestine, and small intestine (using capsule endoscopy) is presented.
2. AI to diagnose stomach disease
2.1. Characteristics of stomach disease and the necessity for AI-based systems
Esophagogastroduodenoscopy (EGD) is the standard procedure used to diagnose diseases of the stomach. However, EGD performance significantly varies among endoscopists owing to long-term specialized training requirements and experience to detect and diagnose early stage gastric cancers and precursor lesions [Citation1]. Several studies have reported that the rate of undetected upper gastrointestinal (GI) cancers over the previous 3 years was 9.8%–25.8% [Citation2–Citation4], mostly because of various endoscopic errors. To address this problem, the imaging diagnostic capability of AI has also been applied to EGD (), even though many clinicians believe that AI application would be more difficult for the stomach than for other areas of the GI tract because of the anatomical features of the stomach and because stomach diseases often involve mucosal inflammation, making AI imaging accuracy difficult.
Figure 1. Representative images of gastric cancer detected by artificial intelligence.
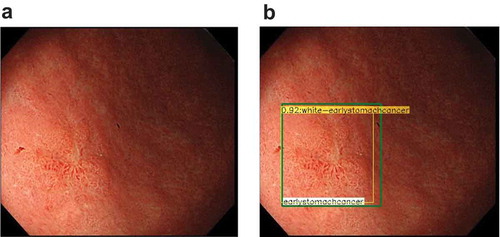
Figure 2. Representative images of esophageal cancer detected by artificial intelligence.
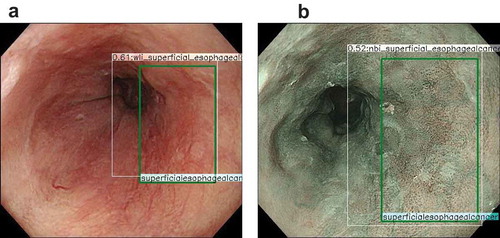
2.2. Malignant disease diagnosis
provides an overview of studies in which image recognition by AI was applied to the stomach. Many clinical AI studies on the stomach have focused on detecting and diagnosing gastric cancer. Hirasawa et al. first reported that AI-based detection may be useful in gastric cancer. Their system was trained using 13,584 high-resolution white light images (WLIs) of gastric cancer, narrowband images (NBIs), and chromoendoscopy using indigo carmine [Citation5]. A validation set of 2296 images showed a sensitivity of 92.2%; however, the positive predictive value (PPV) was only 30.6%, often misdiagnosing non-cancerous lesions as cancer. Ishioka et al. evaluated the performance of the same CNN when applied to videos, and the results were similar to those with still images [Citation6], with a sensitivity of 94.1%. However, PPV was not calculated as only gastric cancers that had previously been diagnosed were targeted. In another study that evaluated gastric cancer detection, Wu et al. reported on AI performance with a validation set of 100 gastric cancers and 100 nonmalignant images, obtaining sensitivity, specificity, and accuracy values of 94.0%, 91.0, and 92.5, respectively [Citation7]. Luo et al. developed a GI AI diagnosis system (GRAIDS) to demonstrate its capability in detecting upper and lower GI cancers, including esophageal cancers [Citation8]. The accuracy for identifying GI cancer ranged from 91.5% to 97.7% with seven validation sets used in their multicenter study. GRAIDS performance was compared with that of endoscopists; GRAIDS achieved a diagnostic sensitivity similar to the expert endoscopists (94.2% vs 94.5%) and superior sensitivity compared with competent (85.8%) and trainee (72.2%) endoscopists.
Table 1. Use of artificial intelligence in stomach field.
Kanesaka et al. identified early gastric cancer on magnified NBIs with an accuracy of 96% [Citation9]. Other studies subsequently reported that CNN technology was efficient for early gastric cancer diagnoses using magnified NBIs [Citation10,Citation11].
With regard to gastric cancer characterization, Zhu et al. applied an AI-based system to evaluate invasion depth using WLIs, with an overall accuracy of 89.16%, significantly higher than that of endoscopists [Citation12].
2.3. Diagnosis of non-neoplastic disorders
AI has also been applied to evaluate non-neoplastic disorders. Shichijo et al. detected and diagnosed Helicobacter pylori gastritis on WLIs, NBIs, and chromoendoscopy images, with a sensitivity of 81.9% and a specificity of 83.4% [Citation13].
Itoh et al. also demonstrated that AI could detect and diagnose H. pylori gastritis on WLIs with a sensitivity and specificity of 86.7% [Citation14]. Nakashima et al. optimized these results using blue-light (BL) imaging and linked color (LC) imaging; the areas under the curve (AUCs) in the receiver operating characteristics (ROC) analysis of WLIs, BL images, and LC images were 0.66, 0.96, and 0.95, respectively [Citation15]. Guimarães et al. also reported that CNN technology could be used to diagnose H. pylori gastritis on WLIs, with an accuracy of 92.9% [Citation16]. Machine learning can also be applied to assess gastroduodenal ulcers and predict the risk of recurrent bleeding [Citation17]; AI identified patients with recurrent ulcer bleeding after 1 year, with an AUC of 0.775 and an overall accuracy of 84.3%.
2.4. Anatomical classification of the stomach
AI systems have also been tested to classify the stomach anatomically. For instance, Takiyama et al. developed a CNN to classify the anatomical location of the upper digestive tract [Citation18], using 27,335 classified EGD images, including 20,202 stomach images, as the training dataset. The AUC of the ROC values was 0.99 for the stomach. In a study on gastric cancer detection, Wu et al. reported that AI could monitor blind spots in the stomach [Citation7]. For their study, they collected images of 26 typical sites in the stomach and used them to train the CNN. If the AI recognized the sites, classified into either 10 or 26 parts, the sites were defined as ‘observed.’ In this test, the AI achieved an accuracy of 90% or 65.9%, respectively, which was better than that of the endoscopists.
Wu et al. subsequently developed the AI system WISENSE (now renamed as ENDOANGEL) to perform a real-time stomach site check [Citation19]. They conducted a randomized controlled trial on 324 patients and reported that using the AI during EGD resulted in a 15% reduction in blind spots. Chen D et al. most recently reported the performance of ENDOANGEL in another clinical trial [Citation20]. They compared the blind spot rate of three EGD types (unsedated ultrafine, sedated conventional, and unsedated) with and without the AI system. They reported that the rate in conventional EGD was the lowest among the three groups and that AI had its largest additional effect in the sedated conventional EGD group.
2.5. Perspectives
Many studies have focused on AI use in the stomach, particularly in the detection and diagnosis of gastric cancer. Some investigations have used magnifying endoscopic images. Further AI studies on the stomach are expected to contribute to the detection and diagnosis of gastric cancer, because a diagnosis of H. pylori infection allows the evaluation of the risk of gastric cancer, while the anatomical classification of the stomach is helpful in the detection of gastric cancer because it ensures that all sites of the stomach are checked. AI systems can assist endoscopists in making accurate diagnoses and will hopefully improve survival rates by detecting gastric cancer at an early stage.
Most of the studies on AI have been reported in Japan and China. The reports in China have mainly assessed patients with gastric cancer at various stages, which provided valuable information on recognizing both early and advanced cancer. However, reports from Japan have focused more on early gastric cancer. Specifically, these reports focused mostly on small, flat lesions, which may be difficult to identify even for expert endoscopists. The use of AI-based diagnostic systems could be more beneficial for detecting smaller lesions or lesions more difficult to identify versus larger lesions, especially since endoscopists rarely miss larger advanced gastric cancerous lesions.
Early diagnosis of H. pylori infection can potentially contribute to premalignancy evaluations. For example, scoring systems for premalignant conditions using AI-based diagnosis of H. pylori infection status (present, eradicated, or non-eradicated), the degree of gastritis, and the degree of intestinal metaplasia might be useful. As another challenge, AI-based diagnostic systems trained by images of the normal stomach mucosa with/without gastric cancer, with/without multiple gastric cancer, with/without advanced gastric cancer, or with/without lymph node metastasis might also be useful for evaluating premalignant conditions. AI could be helpful in providing an appropriate endoscopic surveillance system by identifying candidates a high risk of gastric cancer for gastric cancer.
Furthermore, AI systems may have potential use in other fields. For example, some promising studies have used AI to diagnose non-neoplastic disorders or cancer invasion depth. However, there are currently more investigations on the detection and diagnosis of gastric cancer using AI. The application of AI-based diagnostic systems for gastric cancer invasion by using still images is thought to be difficult because diagnoses require observing tumor shapes that change depending on the degree of air-insufflation. If it is possible to train AI and validate the systems with video WL and EUS images, it may contribute to invasion depth assessments.
The study designs thus far have been mostly retrospective and involved still images, although some studies have used videos. Only a few studies have been conducted as clinical trials; therefore, more real-time clinical trials are needed to confirm the application of AI models in daily clinical practice.
AI has great potential in the field of gastroenterology. Accurate diagnosis using AI may allow for optical biopsies, which could reduce unnecessary biopsies or endoscopic resections, decreasing the risk of bleeding in patients and reducing health expenditures. AI may also help to standardize diagnostic approaches, which are subjective and vary among clinicians and may thus allow high-quality methods to be used, even in institutions with few expert endoscopists.
When AI technology is applied to a routine EGD, our role as endoscopists is to prepare optimal stomach conditions to provide an environment to establish correct diagnoses. The process involves inserting a scope smoothly for relieving discomfort and to resect the tumor safely. The focus should be on improving endoscopic skills even when using AI-based diagnostic systems.
Use of AI in a stomach field requires three factors for clinical usage: detection, classification, and blind spot monitoring. In the near future, when AI models are fully trained to assess all gastric neoplastic and non-neoplastic diseases, including rare ones, and when clinicians confirm the feasibility of the above three factors in a real-life setting, AI-based diagnostic systems may make a more important contribution to the endoscopic diagnosis of stomach diseases.
The use of AI systems for stomach disease evaluations can be summarized as follows: (1) many AI studies of the stomach have focused on the detection and diagnosis of gastric cancer; (2) research in this field has involved magnifying NBIs; (3) studies on the diagnosis of non-neoplastic lesions, particularly H. pylori infection, have also demonstrated promising results; (4) anatomical classification is used to monitor blind spots, with clinical trials being conducted in this field; and (5) the use of AI systems in the stomach requires more clinical trials with video images to confirm their applicability in daily clinical practice.
3. AI to diagnose esophageal disease
3.1. Characteristics of esophageal disease and the necessity for AI-based systems
Esophageal cancer is the seventh most common cancer worldwide [Citation21]. It has two distinct histological types: esophageal squamous cell carcinoma (ESCC), which is common in Asia, the Middle East, Africa, South America, and Japan, and esophageal adenocarcinoma (EAC), which is increasing in the United States and Europe [Citation22]. Diagnosed at an advanced stage, both types of esophageal cancer require highly invasive treatment, and their prognosis is poor. Therefore, early detection is of great importance. However, it is difficult to diagnose early stage esophageal cancer using conventional endoscopy with WLIs. Iodine staining has been used to detect ESCC in high-risk patients. However, it is associated with chest discomfort and increased procedure time [Citation23]. NB imaging is a revolutionary technology involving image-enhanced endoscopy; it can detect superficial ESCC more frequently [Citation24–Citation27]. NB imaging is superior to iodine staining because it is easy to use, involves pushing one button in a moment, and causes no discomfort to patients. However, NB imaging has insufficient sensitivity for detecting ESCC (53%) when used by inexperienced endoscopists [Citation28]; therefore, new effective technologies are needed to detect superficial ESCC.
3.2. ESCC diagnosis
Horie et al. reported a CNN-based AI system that can detect both ESCC and EAC [Citation29] (). They trained a CNN system using 8428 endoscopic images of esophageal cancer, including 365 cases of ESCC and 32 of EAC. They then validated the AI system using a different validation data set consisting of 1118 images from 97 patients, including 47 with esophageal cancer and 50 without esophageal cancer. The AI-based diagnostic system analyzed 1118 images in 27 patients and detected cancer in 98% (46/47) of cases. Notably, the system detected all seven lesions less than 10 mm in diameter. The sensitivity of the system for each image was 77%, with a specificity of 79%, a PPV of 39%, and a negative predictive value (NPV) of 95%. Moreover, the system could differentially diagnose superficial and advanced cancer with an accuracy of 98%. Although the PPV was not high, the authors mentioned that deep learning regarding normal structures and benign lesions in the esophagus would improve the PPV.
Ohmori et al. developed a CNN-based AI system that was trained using both non-magnified and magnified endoscopic images of ESCC, non-cancerous lesions, and normal esophagus tissue [Citation30]. The accuracy of the AI system for diagnosing ESCC was comparable to that of experienced endoscopists. The system also achieved a high PPV (76%) for detection using non-magnified images and in the differentiation of ESCC using magnified images.
Cai et al. developed a deep neural network (DNN)-based AI system, trained using 2428 images, to detect ESCC [Citation31]. The system was superior to both experienced and inexperienced endoscopists in detecting ESCC in endoscopic images, with higher sensitivity and specificity values. Interestingly, the sensitivity of endoscopists in detecting ESCC was greater in the image set with a rectangular frame displayed by the AI system to indicate ESCC. Guo et al. trained an AI system using 6473 NBIs and validated both NBIs and video datasets of non-magnified and magnified NBIs [Citation32]. The sensitivity for detecting ESCC in the video datasets was 100% in both the non-magnified and magnified NBIs.
3.3. Pharyngeal cancer diagnosis
Pharyngeal cancer often occurs in patients with ESCC. Recent advances in NB imaging and increased awareness among endoscopists have led to the increased detection of superficial pharyngeal cancer (SPC) during screening upper endoscopy [Citation24,Citation33]. Detected at an early stage, pharyngeal cancer can be treated using endoscopic resection, which ensures safe, effective, and minimally invasive treatment with good outcomes [Citation34–Citation36]. However, most reports on the detection and treatment of SPC are from Japan. These techniques are not prevalent worldwide, and an efficient detection system for SPC is necessary. Tamashiro et al. used a CNN-based AI system to detect SPC; the system was trained using 5403 images of pharyngeal cancer and detected all pharyngeal cancer lesions in the validation datasets, including small lesions [Citation37]. Thus, this AI system could prevent SPCs from being missed in Japan and worldwide.
3.4. Depth of invasion diagnosis
Tokai et al. used a CNN-based AI system to diagnose the invasion depth of ESCC, distinguishing EP-SM1 lesions from those deeper than SM2 in superficial ESCC [Citation38]. The accuracy score of this system exceeded that of 12 out of 13 board-certified endoscopists, and its AUC was greater than that of all endoscopists. Nakagawa et al. also reported good outcomes in a CNN-based AI system for diagnosing the invasion depth of ESCC using both non-magnified and magnified images [Citation39].
3.5. Endocytoscopic diagnosis
Intrapapillary capillary loops (IPCLs) are microvessels that were first characterized using magnifying endoscopy for ESCC [Citation40,Citation41]. They are now used as established markers to diagnose ESCC. Everson et al. reported the AI classification of IPCL patterns in patients with ESCC [Citation42]. Their CNN system could differentiate abnormal and normal IPCL patterns with 93.7% accuracy on magnified NBIs.
The endocytoscopic system (ECS) provides a magnifying endoscopic examination that enables surface epithelial cells to be observed using methylene blue staining [Citation43–Citation45]. The optical magnification power of the ECS is 500× [Citation44], which can be increased to 900× using digital magnification in the video processor. ECS has been used to diagnose benign and malignant lesions with high accuracy [Citation45]. Kumagai et al. used a CNN-based AI system to diagnose ESCC using ECS images [Citation46]. They trained the system using 4715 ECS images of the esophagus. The AI system correctly diagnosed 92.6% of ESCC cases and its overall accuracy was 90.9% in ESC images. They concluded that AI could be used to support endoscopists in diagnosing ESCC based on ECS images, without reference to biopsy-based histology.
3.6. EAC diagnosis
There are limited reports on EAC diagnosis. Ebigbo et al. developed a CNN-based AI system to detect EAC. Their system was trained using 148 images and achieved high sensitivity (92%) and specificity (100%) compared with 11 of the 13 endoscopists [Citation47]. They also validated the system in real time concurrently with the endoscopic examination using 62 images from 14 patients assessed using the AI system. All images were validated pathologically by resection or biopsy and differentiated the normal esophagus from EAC with a sensitivity of 83.7% and a specificity of 100% [Citation48]. One recent study by Hashimoto et al. developed a CNN system that was trained by 916 images of EAC caused by Barrett’s esophagus (BE) indicating high-grade dysplasia and T1 cancer. This system was validated by 458 images with a reported accuracy of 95.4% [Citation49]. The two other studies were reported by de Groof et al. who developed a computer-aided detection (CAD) system using a hybrid ResNet-UNet model system that included 1704 images of BE neoplasia and 669 images of nondysplastic BE [Citation50]. This CAD system achieved higher accuracy than any of the individual 53 international nonexpert endoscopists in the validation process using still images. de Groof et al. evaluated a CAD system during live endoscopic procedures in 20 patients; they obtained WLIs at every 2-cm level of BE and the CAD system reported the results immediately [Citation51]. They reported that 9 of 10 patients with neoplastic lesions were correctly diagnosed with a 90% accuracy rate per level in the analysis.
3.7. Perspectives
Although only a few reports have described AI-based diagnostic systems for esophageal cancer, the number of reports is now rising sharply. Most of these reports have both trained the AI and validated the results using still images, except for one recent report that validated ESCC using videos [Citation32]. Interestingly, two studies on EAC attempted to perform real time detection analysis during endoscopic procedures by still images, which may be useful for assessing conditions such as EAC, because the area of BE is limited. However, detection using videos is more useful as detection can be done promptly without the need to capture too many pictures. However, the detection in videos has to be analyzed well before endoscopists could use during endoscopy. To further investigate whether AI is useful to prevent missed diagnosis of cancers, validation videos should not be limited to slow observation videos but should include fast videos, However, this high accuracy is in high-quality video and is less accurate in videos containing low-quality images.. For detecting superficial cancers, AI systems can be valuable supportive tools for inexperienced endoscopists as well as experienced endoscopists. Because only specialists diagnose invasion depth and use magnifying endoscopy or ECS, Since the invasion depth diagnosis is related to treatment policy decision support, the accuracy of AI needs to exceed that of specialists, which require further improvement. . In addition to endoscopic images, a patient’s information such as past history and laboratory data may be integrated into the AI system to assess disease risk and accurately identify the diagnosis beyond the capabilities of human endoscopists.
4. AI to diagnose colorectal polyps
4.1. Deep learning-based CAD/diagnostic system for colorectal polyps
One of the main tasks of colonoscopy is to detect colorectal polyps. To this end, the roles of AI can be divided into detection and diagnosis of polyps. Recently, many studies have been conducted to develop a computer-assisted detection (CADe) or diagnosis (CADx) system that can be used in real time during colonoscopy.
Colorectal polyps are missed during examination for several reasons: insufficient preparation of the colorectum, the anatomical structure of the colorectum itself (haustra), peristaltic movement, instrumental issues (old scopes or monitors), and lack of expertise, fatigue, or careless mistakes on the part of physicians.
Colorectal polyps, especially adenomas and sessile serrated lesions (SSLs), are thought to be precancerous lesions, and missed polyps can be a cause of interval cancer [Citation52,Citation53]. Therefore, many efforts have been made to decrease the polyp missing rate. In this regard, technological progress in endoscopic devices may have improved the quality of colonoscopy, and image-enhanced endoscopy such as NB imaging has led to an increase in the polyp detection rate and adenoma detection rate (ADR) [Citation54]. In particular, the Endocuff Vision, which is mounted onto the distal tip of the colonoscope and stretches the colorectal fold, has been shown to increase ADR [Citation55], and a recent study showed that a per-oral methylene blue formulation increased ADR [Citation56].
However, even these technologies may not compensate for physician-related factors. In this regard, CADe systems may be a promising technology for next-generation endoscopy. Many studies have been conducted to develop a CADe system, and most indicate that deep learning-based CADe systems are the most promising technology.
4.2. Colorectal polyp diagnosis
Recently, several studies have been conducted on deep learning-based CADe systems of colorectal polyps. Apart from the deep-learning algorithms, there are three major differences between the CADe systems used: (1) training and testing images, which can be static and/or video, and can vary in number; (2) type of image mode included – conventional WLIs and/or enhanced images as well as magnified or unmagnified images; and (3) output styles – whether the CADe system localizes polyps or simply confirms the existence of polyps within the images.
shows the studies on AI-based CADe systems [Citation57–Citation64]. The results of each study cannot simply be compared, but taken together, the reports demonstrate that AI-based CADe systems have approximately 95% accuracy in detecting colorectal polyps in static images. However, the accuracy decreases in images from videos that include low-quality images. Thus, to make better CADe systems, low-quality images may need to be included in the training images, not only high-quality images. Additionally, the CADe systems should be tested on video images because they are closer to the actual situations in which the CADe systems would be used. Furthermore, even retrospective evaluation using video images is insufficient, and prospective studies in real-time settings should be conducted to evaluate the actual performance of the CADe system.
Table 2. Use of artificial intelligence in colonic polyp.
Table 3. Deep learning-based systems for capsule endoscopy.
In these studies, both sensitivity and specificity or PPV are important because these CADe systems are supposed to be used during colonoscopy; too many false positives can be annoying and may cause clinicians to overlook real events. Indeed, the CADe system created by Wang et al. showed good sensitivity, although the same value was fairly low in a prospective study using the same system [Citation74].
Wang et al. recently conducted a prospective randomized controlled trial and subsequent double-blind randomized controlled trial using their own CADe system [Citation63,Citation64]. These studies demonstrated that the CADe system was efficient; the ADR was significantly higher in the CADe system group than in the standard colonoscopy group (29.1% vs. 20.3%, p < 0.001) in the former study, and the CADe system also showed higher ADR than the sham system (34% vs. 28%; p = 0.030) in the latter study. The latter study used a sham system to create a double-blinded scenario, although a randomized tandem colonoscopy study may have been more appropriate to evaluate the benefits of the CADe system.
Although these studies show that CADe systems are promising for the detection and diagnosis of colorectal polyps, the system’s performance for small polyps or flat lesions is not clear, and further evaluation may be necessary.
4.3. Computer-assisted diagnostic systems for colorectal polyps
Recently, because of advances in histopathological knowledge, improvement in instruments, and progress of new image-enhanced technologies, expert colonoscopists may be able to perform optical diagnosis of colorectal polyps with high accuracy in both colorectal polyp classification and prediction of colorectal cancer depth.
In terms of the optical classification of polyps, because histopathological examination after removal of diminutive polyps is costly and time-consuming, the American Society for Gastrointestinal Endoscopy recommends ‘diagnose and leave’ and ‘resect and discard’ strategies. To achieve these strategies, optical classification with high accuracy is necessary. However, special skills and training are required to reach a sufficient level [Citation74]. The European Society of Gastrointestinal Endoscopy advises that optical diagnosis should be performed in a strictly controlled environment by experienced, trained, and audited endoscopists [Citation75]. Therefore, CADx systems with specialist-level diagnostic skills would be useful to support trainees and help reduce unnecessary biopsy or histopathological examinations.
The prediction of cancer depth is also an important issue. Owing to recent improvements in endoscopic devices, endoscopic submucosal dissection (ESD) is widely performed in cases of large benign colorectal polyps or submucosal colorectal cancers. However, ESD of submucosal cancers with deep invasion is technically difficult and can cause lymph node metastases in 10%–15% of cases. Thus, these cancers should be treated using radical surgery.
In Japan, examination of pit patterns using magnifying chromoendoscopy or the Japan NBI Expert Team classification is recommended to diagnose the classes of polyps and predict deep submucosal invasion [Citation76]. In Western countries, the NBI International Colorectal Endoscopic classification has been validated as a useful method for diagnosing polyps or predicting deep submucosal lesions [Citation77]. However, these classifications have considerable inter-observer differences and require expertise. Therefore, CADx systems may be a highly promising technology for predicting the histopathology of polyps and the presence of deep submucosal lesions. Recently, deep learning-based CADx systems have also been developed.
Five AI-based CADx systems using standard colonoscopy have been reported () [Citation78–Citation82]. Four of these were developed to perform binary classification: hyperplastic polyps (serrated polyps or non-adenomatous polyps) vs. adenomatous polyps [Citation78,Citation79,Citation82]. Two of them used only NBIs to differentiate adenoma from non-adenoma, with accuracy values of 90%–95% and NPVs of more than 90%, which meet the PIVI requirement [Citation78–Citation80]. Two studies used WLIs to develop the CADx systems; in one of these, the accuracy was only 75% to differentiate adenoma from non-adenoma. However, the number of training images used was only 1200 [Citation79]. In another study using both WLIs and NBIs, the accuracy was 92% using WLIs, which was comparable to that using NBIs [Citation82].
Another approach involves the use of an endocytoscope. Kudo et al. developed a CADx system for endocytoscopy, referred to as endocytoscopy Endo-BRAIN® [Citation83,Citation84]. Endocytoscopy has two important abilities: 1) in vivo observation of cell nuclei and crypt structure using methylene blue staining and (2) observation of microvessels using NBI mode. In this retrospective study using static endocytoscopy images from 100 patients, the CADx system showed 98% accuracy, 96.9% sensitivity, 100% specificity, 100% PPV, and 94.6% NPV for the differentiation of neoplastic vs. non-neoplastic polyps [Citation84]. Although endocytoscopy is still not widely used in clinical settings, these results show the promising role of Endo-BRAIN®.
Song et al. developed a CADx system to classify polyps into three classes: SSLs, benign adenoma/mucosal or superficial submucosal cancers, and deep submucosal cancers [Citation81]. The overall diagnostic accuracy of this system was 81%–82% higher than that of trainees and comparable to that of experts. Furthermore, the diagnostic accuracy of the trainees improved from 64%–72% to 83%–84% with the assistance of the CADx system.
Although these results show robust performance from CADx systems, it must be noted that physicians’ technical expertise is important to diagnose polyps properly; in cases of small polyps with uniform surface patterns and/or vascular patterns, it is not difficult to diagnose the class of polyps. The problem occurs when the polyp is large and has a non-uniform surface pattern/vascular pattern. Such polyps can conceal advanced adenoma or cancer in only a small part of the tumor. Magnifying colonoscopy or endocytoscopy is more useful for diagnosing polyps; however, endoscopists must still show the surface of the most advanced lesions appropriately. Thus, prospective studies in real-time should be conducted in the near future to evaluate the performance of CADx systems in real clinics.
4.4. Perspectives
In conclusion, CADe and CADx systems for diagnosing colorectal polyps have been progressing dramatically in recent years, and previous studies have shown robust performance of these systems. Furthermore, the combination of CADe/CADx systems with other technologies, such as full-spectrum colonoscopy, may produce synergistic effects [Citation85].
With regard to colorectal polyps, the accuracy of AI is about the same as that of human physicians. Furthermore, clinical studies and prospective trials have reported its applicability in actual clinical situations. Validations have been performed with previous still images and videos. Future studies should develop standards that can compare the excellent points of each AI, establish guidelines for its common use, and achieve cost-effectiveness to enable insurance coverage.
In clinical practice, large tumors are rarely missed during colonoscopy; therefore, AI may be less useful in finding large tumorous lesions. However, for large tumors, AI may be useful for predicting the efficacy of anticancer agents in combination with clinical course information and the efficacy of radiotherapy for tumors in combination with clinical information.
The large intestine has a length of 1.5 m or more, and the folds must be separated by an endoscopist during examination. With current AI systems, we have not been able to eliminate the obstacles in viewing the tissue among the folds, and because the large intestine is a winding tube, the prospects of being able to automate endoscopic operations are limited. Therefore, for AI application in these fields, new breakthroughs other than CNNs will be needed.
Although several issues remain to be resolved, including regulatory approval and legal issues, these technologies will likely be introduced within clinics in the near future.
5. AI in capsule endoscopy
5.1. Characteristics of capsule endoscopy and the necessity of automatic detection systems
Capsule endoscopy has marked a revolution for investigating various small bowel abnormalities such as mucosal breaks, angioectasia, protruding lesions, and presence of bleeding. (). Capsule endoscopy uses a tiny wireless camera to capture pictures of the GI tract. A capsule endoscopy camera is placed inside a vitamin-size capsule that patients swallow. As the capsule travels through the GI tract, the camera takes thousands of pictures that are transmitted to a recorder. At the time of this publication, around 10 types of capsule endoscopies from five companies are commercially available. Although it is relatively simple to perform during patient examination, capsule endoscopy has major drawbacks for physicians. The greatest characteristic of capsule endoscopy is the automatic capture of the GI tract. A review of around 50,000 images per patient is time-consuming for physicians. In addition, physicians fear the risk of oversight because abnormalities may appear in a few frames. Indeed, trainee endoscopists have been reported to overlook more abnormalities than expert endoscopists [Citation86]. A computer-aided supporting system would help reduce the reading time and the oversights by automatically detecting GI tract abnormalities.
Thus, automatic detection systems are necessary, and several attempts have been made to develop them. For example, capsule endoscopy systems tend to have their own proprietary software for decreasing images (e.g., the QuickView mode on PillCam® system, the omni mode on the EndoCapsule® system, and the express view mode on the MiroCam® system). In addition, a number of systems currently under investigation is based on machine learning methods, such as support vector machines, binary classifiers, or neural networks [Citation87]. However, these software and systems are not yet used in clinical practice. Recently, state-of-the-art deep learning-based methods have shown significantly improved recognition performance in various medical fields [Citation88,Citation89] and are expected to overcome problems in capsule endoscopy reading.
5.2. Developing deep learning-based systems for capsule endoscopy
Recent reports have adopted CNN architectures, which are deep learning-based systems [Citation90]. In general, the diagnostic ability of a CNN-based system depends on the training data quality and amount. However, capsule endoscopy image quality is limited by light limitations, poor focus, low resolution, unpredictable orientation due to free motion of the capsule, and obscuration by bubbles, debris, and bile. In addition, it is difficult to gather images of each abnormality because small bowel diseases are rare. Therefore, such systems are difficult to develop, especially for detecting various abnormalities in the small bowel.
As shown in , most previous studies have trained a CNN using images of one type of abnormality (polyp, celiac disease, hookworm, bleeding, erosion/ulcer, or angioectasia) and tested the ability of the CNN using selected still images. Most reports have focused on small bowel capsule endoscopy, although capsule endoscopy systems for other elements of the GI tract have been commercially available. The major capsule system studied in this field was the PillCam® system (Medtronic, Minneapolis, MN, USA).
Generally, these CNNs showed high accuracy. Almost all systems achieved more than 90% accuracy, sensitivity, and specificity, with an AUC of more than 0.9 in each image analysis. In particular, systems for detecting bleeding or angioectasia demonstrated extremely high performance (accuracy > 98% and AUC > 0.99) [Citation91], probably because the color cue of these findings was obvious.
CNN-based systems, which can automatically derive features from images, have shown better performance than previous handcrafted image-based analyzes. For example, a CNN-based method by Zou et al. classified digestive organs (the stomach, small bowel, or colon) in capsule endoscopy with an accuracy of 95.5% – higher than conventional scale-invariant feature transform- and SVM-based approaches (90.3%) [Citation90]. Similarly, a CNN-based method by Segui et al. classified motility events (turbid, bubbles, clear blob, wrinkle, or wall) in capsule endoscopy with an accuracy of 96.0% – higher than the conventional approach of combining handcrafted features (82.8%) [Citation92].
Among several CNN-based methods, methodological differences may affect the results. He et al. [Citation93] and Li et al. [Citation94] compared multiple CNN methods in their studies. He et al. reported that their own proprietary deep hookworm detection framework based on CNN showed a higher AUC (0.895) than previous CNN methods such as AlexNet (0.769) and GoogleNet (0.883). Le et al. demonstrated that LeNet was inferior to other types of CNN methods such as AlexNet, GoogLeNet, and VGG-Net regarding F-measure and sensitivity in bleeding detection.
Most recently, CNNs for detecting various lesions have been reported [Citation95–Citation97]. An outstanding report from Ding et al. showed high sensitivity (95.4%) and specificity (97.0%) of a CNN per image analysis. This system was developed and tested using copious amounts of data, including images of various abnormalities and normal images [Citation96].
5.3. Clinical usefulness of deep learning-based systems
It is important that researchers investigate the clinical usefulness of CNNs using full videos. However, only a few such studies have been conducted. Aoki et al. reported the clinical usefulness of their proprietary CNN for detecting erosion/ulcers using 20 full videos of small bowel capsule endoscopy that included a total of 37 erosions/ulcers [Citation99]. A comparison was made between two reading methods (endoscopist alone vs. endoscopist after first screening by a CNN) based on the reading time and detection rate of erosion/ulcers by endoscopists. The mean reading time of small bowel sections by expert endoscopists was significantly shorter after the first screening by the CNN (3.1 min) than by the endoscopist alone (12.2 min). For 37 erosion/ulcers, the detection rate by experts did not significantly decrease in endoscopist readings after the first screening by the CNN (87%) compared to endoscopist-alone readings (84%). Similar trends were observed when the CNN was used by trainee endoscopists, whereby the CNN could reduce the reading time of physicians without decreasing the detection rate of erosion/ulcers. This study suggested that the CNN could be used as a first screening tool.
Ding et al. reported the clinical usefulness of their original CNN for detecting various types of abnormalities, using 5000 full videos of small bowel capsule endoscopy that included a total of 4206 various lesions [Citation96]. In addition to the method reported by Aoki et al. [Citation99], two reading methods (endoscopist-alone readings vs. endoscopist readings after the first screening by the CNN) were compared in terms of reading time and detection rate of abnormalities by endoscopists. The mean reading time per patient was 96.6 min for endoscopist-alone readings and 5.9 min for CNN-based auxiliary readings (p < 0.001). Detection rates of abnormalities were significantly higher for CNN-based auxiliary readings than for endoscopist-alone readings (per-lesion analysis: 99.90% vs. 76.89%; per-patient analysis: 99.88% vs. 74.57%). The same report indicated that the auxiliary use of a CNN system could reduce oversight and decrease reading time. This system was developed and tested based on the Ankon capsule endoscopy system (Ankon Medical Technologies, Hubei, China).
5.4. Perspectives
Several promising CNN-based systems have been reported. The biggest advantage of those systems is reducing the reading time of physicians, which has been shown to play an important role in previous reports that investigated the clinical usefulness of CNNs. However, none of them are commercially available. To allow clinical use, CNN systems to diagnose various abnormalities should be tested using a large number of full videos. Many previous studies using full videos have concluded that the existing QuickView mode of the PillCam® system was unsuitable as a first screening tool because it had an unacceptable miss rate for noteworthy abnormalities, although the mode could reduce the reading time of physicians [Citation86]. In addition, the generalizability of CNNs to multiple capsule endoscopy systems can be evaluated by tests using full videos from several types of capsule endoscopy systems.
Although the aforementioned studies have reported that CNN systems show high performance, the results cannot be directly compared because different test datasets and different evaluation methods were used. Recently, Koulaouzidis et al. proposed the KID database using the MiroCam® system (IntroMedic Co., Seoul, Korea) [Citation98]. It is a publicly available database of annotated capsule endoscopy images and videos that can be used as a reference dataset. Such datasets would accelerate investigations into the generalizability of CNNs and would allow direct comparison between different CNN systems.
The output method of CNN findings may affect the clinical usefulness. For example, Single Shot MultiBox Detector can depict bounding boxes in images to localize the region of interest. Bounding boxes would be useful landmarks for reducing oversight and reading time if the CNN system is highly accurate. However, these boxes can be confusing for physicians if they emphasize areas other than abnormalities, which occurs with low-quality CNNs.
Furthermore, advances in capsule endoscopy are expected in the field, although we focused on CNN systems for small bowel capsule endoscopy in this review. Capsule endoscopy systems for the esophagus and colon are now available, and magnetically guided capsule endoscopies for gastric examination are performed in some institutions. Magnetically guided capsule endoscopy could be a promising alternative screening tool in an era of COVID-19, as this tool is less invasive and would have a lower risk of infection than gastroscopy. Developing CNN systems for different capsule endoscopic applications would be beneficial.
Capsule endoscopy applications can be summarized as follows: (1) capsule endoscopy has the unique characteristic of automatic capturing; (2) previous software and machine learning-based systems have failed to resolve the drawbacks of video reading; (3) promising CNN systems have recently been developed; and (4) these CNN systems require tests using a large number of full videos to investigate their clinical usefulness and generalizability to multiple types of capsule endoscopy systems.
6. Expert opinion
In recent years, CNN-based AI has been applied in image recognition. Evidently, the ability of AI in general image recognition exceeds that of humans. Although AI was initially applied only to radiographic images (chest X-ray, computed tomography (CT), magnetic resonance imaging (MRI), etc.), research and development (R&D) later expanded its application to GIT endoscopy.
Contemporary AI is only specialized in analyzing image information. A multipurpose AI methodology that can conduct a comprehensive diagnosis by combining medical history and clinical laboratory data does not exist. Developing such an AI method is impossible at present. Currently, AI assists doctors with diagnosis, but is unable to make independent diagnostic decisions.
AI can provide a probable diagnosis, but not a definitive diagnosis. Moreover, AI cannot perceive patients’ emotional status. Doctors can not only convey the diagnosis result in an easy-to-understand manner but also guide or give advice in consideration of the patient’s state of mind. Therefore, developing an AI method that can eliminate the need for the endoscopists is unlikely.
Developing AI to support endoscopic diagnoses benefits medical practitioners. By applying AI in endoscopic examinations, endoscopists can ensure high-quality endoscopy, reduce the burden of additional investigations, and reduce the number of errors. This can be achieved without changing the current technique because endoscopic AI only provides diagnostic assistance during endoscopic examinations. This may also lead to reduced medical errors and increase accuracy of test results in medical organizations. Improving examination accuracy will reduce medical errors and justify the cost of introducing AI for endoscopies.
The introduction of AI to endoscopic clinical practice will also decrease endoscopists’ training time, reduce the burden on training instructors, and compensate for age-related vision problems in experienced doctors. Currently, AI is being used as a tool to assist doctors in diagnosis. Doctors who employ AI-based diagnosis will provide a more accurate diagnosis and treatment.
As outlined in this review, it has become clear at the research level that endoscopic AI can be used with high accuracy in each organ of the gastrointestinal tract. In addition, accuracy validation of AI has moved past simply verifying the AI system capabilities against previous images or movies and is now being verified against the work of an endoscopist. AI has achieved results comparable or superior to those of endoscopists.
On the other hand, if each AI method is validated with different validation materials, it is not possible to determine which AI is superior.
If standardized validation criteria for each disease are established, it may be possible to differentiate with an accuracy that is easy to understand. At the same time, the accumulation of prospective research evidence will prove the effectiveness of AI in clinical practice, and the future of endoscopic AI as a standard medical device in medical practice guidelines will be possible.
There are a number of technical challenges with AI, the current CNN-based AI requires a large amount of teacher data (usually more than 1000 images), and thus AI development for rare diseases with a small amount of case data is difficult. In addition, there is currently a limit to the number of pixels that can be captured and analyzed by AI, and AI analyzes images of lower image quality than those taken with actual endoscopy equipment. A technical challenge remains as to whether AI accuracy can be improved, as the image quality of endoscopic devices progresses. However, there is a possibility that these challenges can be overcome by establishing a system to collect data not only in each country but globally and by developing a new AI algorithm.
It will be difficult for AI to perform endoscopy operations on behalf of humans for the time being because the shape of the digestive tract varies from person to person. Even if AI assists in diagnosis during the endoscopic examination, the endoscope will continue to be operated by a person. Therefore, efforts to improve the technique for operating endoscopes will continue to be essential for endoscopists.
Capsule endoscopy that can observe the entire digestive tract may be developed in the near future. In that case, there will be an era in which the screening examination is first performed with a capsule endoscopy, which is less invasive to the patient, and additional detailed examination is performed with the upper and lower endoscopies depending on the result. Considering the importance of image interpretation with a capsule endoscopy, highly accurate AI will play an important role in reducing this interpretation load on doctors.
In the near future, endoscopic AI will inevitably become commonplace if sufficient evidence is accumulated for endoscopic AI to be recommended in guidelines or covered by insurance. It has not been examined so far, but by that time, the evaluation of the medical economic impact of AI will be advanced.
If it is possible to develop AI that not only assists diagnosis with images but also comprehensively judges clinical progress and clinical test results, AI capabilities will expand to a range of activities to not only support diagnosis but also support treatment policy decisions.
In addition, endoscopic equipment may not only improve image quality but also become three-dimensional, and along with other sensing technologies, it may become possible to perform 3D mapping of organs with endoscopy. What future advances could there be if AI technology evolves along the same lines as the above-mentioned endoscope? There is no end to the possible development of this field.
7. Conclusion
This review outlines the current research and development status and the prospects of AI application in GI tract endoscopy. Employing AI-based endoscopes would enable early cancer detection and consequent improved prognosis. AI technology use for stomach conditions may be beneficial in terms of better diagnostic ability as early gastric cancer diagnostic ability and accuracy varies greatly among endoscopists. AI-based diagnosis of esophageal cancer has improved over the past few years. Without technical difficulty of surveillance, AI would eliminate the misdetection of esophageal cancer in the near future. The use of AI for assessing conditions of the large intestine may help lower cancer incidence by identifying polyps and performing polypectomies at an early stage, and differential diagnosis support may reduce unnecessary biopsy and pathological examination. Furthermore, implementing AI systems with capsule endoscopies would reduce the image interpretation time with unaltered accuracy, which could lead to further applications in capsule endoscopies.
AI will contribute to the development of endoscopic medicine by assisting doctors, but not by lowering their significance. Endoscopists will require the skills to understand and utilize AI.
Article highlights
AI is effective in image analysis, where in it outperforms the manual interpretations.
The introduction of the AI in the image analysis will support medical diagnosis.
AI-based gastric investigations were focused on the detection and diagnosis of the gastric cancers.
AI used for anatomical classification of the stomach, will monitor unnoticeable regions and reduce the number of undetected cancers.
AI-based investigations in the esophagus include esophageal squamous cell carcinoma and adenocarcinoma.
AI was employed in the detection and classification of the colon polyps in the GIT endoscopy.
AI-based capsule endoscopy would accurately detect the lesions and reduce the interpretation time.
Declaration of interest
Tada T is a shareholder of AI Medical Service Inc. The authors have no other relevant affiliations or financial involvement with any organization or entity with a financial interest in or financial conflict with the subject matter or materials discussed in the manuscript apart from those disclosed.
Reviewer disclosures
Peer reviewers on this manuscript have no relevant financial or other relationships to disclose
Acknowledgments
We would like to thank Mitsue Takahashi and Motoi Miura for providing assistance in editing manuscript.
We would like to thank Editage (www.editage.com) for English language editing.
Additional information
Funding
References
- Yao K, Uedo N, Muto M, et al. Development of an e-learning system for teaching endoscopists how to diagnose early gastric cancer: basic principles for improving early detection. Gastric Cancer. 2017;20:S28–S38.
- Menon S, Trudgill N. How commonly is upper gastrointestinal cancer missed at endoscopy? A meta-analysis. Endosc Int Open. 2014;2(2):E46–50.
- Yalamarthi S, Witherspoon P, McCole D, et al. Missed diagnoses in patients with upper gastrointestinal cancers. Endoscopy. 2004;36:874–879.
- Hosokawa O, Hattori M, Douden K, et al. Difference in accuracy between gastroscopy and colonoscopy for detection of cancer. Hepato-gastroenterol. 2007;54(74):442–444.
- Hirasawa T, Aoyama K, Tanimoto T, et al. Application of artificial intelligence using a convolutional neural network for detecting gastric cancer in endoscopic image. Gastric Cancer. 2018;21:653–660.
- Ishioka M, Hirasawa T, Tada T. Detecting gastric cancer from video images using convolutional neural networks. Dig Endosc. 2019;31:e34–e35.
- Wu L, Zhou W, Wan X, et al. A deep neural network improves endoscopic detection of early gastric cancer without blind spots. Endoscopy. 2019;51:522–531.
- Luo H, Xu G, Li C, et al. Real-time artificial intelligence for detection of upper gastrointestinal cancer by endoscopy: a multicentre, case-control, diagnostic study. Lancet Oncol. 2019;20:1645–1654.
- Kanesaka T, Lee T-C, Uedo N, et al. Computer-aided diagnosis for identifying and delineating early gastric cancers in magnifying narrow-band imaging. Gastrointest Endosc. 2018;87:1339–1344.
- Li L, Chen Y, Shen Z, et al. Convolutional neural network for the diagnosis of early gastric cancer based on magnifying narrow band imaging. Gastric Cancer. 2020;23:126–132.
- Horiuchi Y, Aoyama K, Tokai Y, et al. Convolutional neural network for differentiating gastric cancer from gastritis using magnified endoscopy with narrow band imaging. Dig Dis Sci. 2020;65(5):1355-1363.
- Zhu Y, Wang QC, Xu MD, et al. Application of convolutional neural network in the diagnosis of the invasion depth of gastric cancer based on conventional endoscopy. Gastrointest Endosc. 2019;89:806–815.
- Shichijo S, Nomura S, Aoyama K, et al. Application of convolutional neural networks in the diagnosis of Helicobacter pylori infection based on endoscopic images. EBioMedicine. 2017;25:106–111.
- Itoh T, Kawahira H, Nakashima H, et al. Deep learning analyzes Helicobacter pylori infection by upper gastrointestinal endoscopy images. Endosc Int Open. 2018;06:E139–E144.
- Nakashima H, Kawahira H, Kawachi H, et al. Artificial intelligence diagnosis of Helicobacter pylori infection using blue laser imaging-bright and linked color imaging: a single-center prospective study. Ann Gastroenterol. 2018;31:462–468.
- Guimarães P, Keller A, Fehlmann T, et al. Deep- learning based detection of gastric precancerous conditions. Gut. 2020;69:4–6.
- Wong GL, Ma AJ, Deng H, et al. Machine learning model to predict recurrent ulcer bleeding in patients with history of idiopathic gastroduodenal ulcer bleeding. Aliment Pharmacol Ther. 2019;49:912–918.
- Takiyama H, Ozawa T, Ishihara S, et al. Automatic anatomical classification of esophagogastroduodenoscopy images using deep convolutional neural networks. Sci Rep. 2018 14;8(1):7497.
- Wu L, Zhang J, Zhou W, et al. Randomised controlled trial of WISENSE, a real-time quality improving system for monitoring blind spots during esophagogastroduodenoscopy. Gut. 2019;68:2161–2169.
- Chen D, Wu L, Li Y, et al. Comparing blind spots of unsedated ultrafine, sedated, and unsedated conventional gastroscopy with and without artificial intelligence: a prospective, single-blind, 3-parallel-group, randomized, single-center trial. Gastrointest Endosc. 2020;91:332–339.
- Ferlay J, Colombet M, Soerjomataram I, et al. Estimating the global cancer incidence and mortality in 2018: GLOBOCAN sources and methods. Int J Cancer. 2019;144:1941–1945.
- Enzinger PC, Mayer RJ. Esophageal cancer. N Engl J Med. 2003;349:2241–2252.
- Shimizu Y, Omori T, Yokoyama A, et al. Endoscopic diagnosis of early squamous neoplasia of the esophagus with iodine staining: high-grade intra-epithelial neoplasia turns pink within a few minutes. J Gastroenterol Hepatol. 2008;23:546–550.
- Muto M, Minashi K, Yano T, et al. Early detection of superficial squamous cell carcinoma in the head and neck region and esophagus by narrow band imaging: a multicenter randomized controlled trial. J Clin Oncol. 2010;28:1566–1572.
- Nagami Y, Tominaga K, Machida H, et al. Usefulness of non-magnifying narrow-band imaging in screening of early esophageal squamous cell carcinoma: a prospective comparative study using propensity score matching. Am J Gastroenterol. 2014;109:845–854.
- Lee YC, Wang CP, Chen CC, et al. Transnasal endoscopy with narrowband imaging and Lugol staining to screen patients with head and neck cancer whose condition limits oral intubation with standard endoscope (with video). Gastrointest Endosc. 2009;69:408–417.
- Kuraoka K, Hoshino E, Tsuchida T, et al. Early esophageal cancer can be detected by screening endoscopy assisted with narrow-band imaging (NBI). Hepatogastroenterology. 2009;56:63–66.
- Ishihara R, Takeuchi Y, Chatani R, et al. Prospective evaluation of narrow-band imaging endoscopy for screening of esophageal squamous mucosal high-grade neoplasia in experienced and less experienced endoscopists. Dis Esoph. 2010;23:480–486.
- Horie Y, Yoshio T, Aoyama K, et al. The diagnostic outcomes of esophageal cancer by artificial intelligence using convolutional neural networks. Gastrointest Endosc. 2019 Jan;89(1):25–32.
- Ohmori M, Ishihara R, Aoyama K, et al. Endoscopic detection and differentiation of esophageal lesions using a deep neural network. Gastrointest Endsc. 2020;91(2):301-309.
- Cai SL, Li B, Tan WM, et al. Using a deep learning system in endoscopy for screening of early esophageal squamous cell carcinoma (with video). Gastrointest Endosc. 2019;90(5):745–753.e2.
- Guo L, Xiao X, Wu C, et al. Real-time automated diagnosis of precancerous lesions and early esophageal squamous cell carcinoma using a deep learning model (with videos). Gastrointest Endosc. 2020;91(1):41–51.
- Nonaka S, Saito Y. Endoscopic diagnosis of pharyngeal carcinoma by NBI. Endoscopy. 2008;40:347–351.
- Shimizu Y, Yamamoto J, Kato M, et al. Endoscopic submucosal dissection for treatment of early stage hypopharyngeal carcinoma. Gastrointest Endosc. 2006;64:255–259. discussion 260–2.
- Suzuki H, Saito Y. A case of superficial hypopharyngeal cancer treated by EMR. Jpn J Clin Oncol. 2007;37:892.
- Yoshio T, Tsuchida T, Ishiyama A, et al. Efficacy of double-scope endoscopic submucosal dissection and long-term outcomes of endoscopic resection for superficial pharyngeal cancer. Dig Endosc. 2017;29(2):152–159.
- Tamashiro A, Yoshio T, Ishiyama A, et al. Artificial-intelligence-based detection of pharyngeal cancer using convolutional neural networks. Dig Endsc. 2020 Feb 14. [Epub ahead of print]. DOI:10.1111/den.13653.
- Tokai Y, Yoshio T, Aoyama K, et al. Application of artificial intelligence using convolutional neural networks in determining the invasion depth of esophageal squamous cell carcinoma Esophagus. 2020 January. [Epub ahead of print].
- Nakagawa K, Ishihara R, Aoyama K, et al. Classification for invasion depth of esophageal squamous cell carcinoma using a deep neural network compared with experienced endoscopists. Gastrointest Endosc. 2019;90(3):407–414.
- Inoue H, Honda T, Yoshida T, et al. Ultra-high magnification endoscopy of the normal esophageal mucosa. Dig Endosc. 1996;8:134–138.
- Inoue H, Honda T, Nagai K, et al. Ultra-high magnification endoscopic observation of carcinoma in situ of the esophagus. Dig Endosc. 1997;9:16–18.
- Everson M, Herrera LCGP, Li W, et al. Artificial intelligence for the real-time classification of intrapapillary capillary loop patterns in the endoscopic diagnosis of early oesophageal squamous cell carcinoma: a proof-of-concept study. UEG journal. 2019;7(2):297–306.
- Kumagai Y, Monma K, Kawada K. Magnifying chromoendoscopy of the esophagus: in vivo pathological diagnosis using an endocytoscopy system. Endoscopy. 2004;36:590–594.
- Kumagai Y, Takubo K, Kawada K, et al. A newly developed continuous zoom-focus endocytoscope. Endoscopy. 2017;49(2):176–180.
- Kumagai Y, Kawada K, Higashi M, et al. Endocytoscopic observation of various esophageal lesions at ×600: can nuclear abnormality be recognized? Dis Esophagus. 2015;28:269–275.
- Kumagai Y, Takubo K, Kawada K, et al. Diagnosis using deep-learning artificial intelligence based on the endocytoscopic observation of the esophagus. Esophagus. 2019 Apr;16(2):180–187.
- Ebigbo A, Mendel R, Probst A, et al. Computer-aided diagnosis using deep learning in the evaluation of early oesophageal adenocarcinoma. Gut. 2019;68:1143–1145.
- Ebigbo A, Mendel R, Probst A, et al. Real-time use of artificial intelligence in the evaluation of cancer in Barrett’s oesophagus. Gut. 2020;69(4):615–616.
- Hashimoto R, Requa J, Dao T, et al. Artificial intelligence using convolutional neural networks for real-time detection of early esophageal neoplasia in Barrett’s esophagus (with video). Gastrointest Endosc. 2020;91(6):1264–1271.e1. [Epub ahead of print].
- de Groof AJ, Struyvenberg MR, van der Putten J, et al. Deep-learning system detects neoplasia in patients with Barrett’s Esophagus with higher accuracy than endoscopists in a multistep training and validation study with benchmarking. Gastroenterology. 2020;158(4):915–929.e4.
- de Groof AJ, Struyvenberg MR, Fockens KN, et al. Deep learning algorithm detection of Barrett’s neoplasia with high accuracy during live endoscopic procedures: a pilot study (with video). Gastrointest Endosc. 2020;91(6):1242–1250. [Epub ahead of print].
- He X, Wu K, Ogino S, et al. Association between risk factors for colorectal cancer and risk of serrated polyps and conventional adenomas. Gastroenterology. 2018;155(2):355–373 e18.
- Nayor J, Saltzman JR, Campbell EJ, et al. Impact of physician compliance with colonoscopy surveillance guidelines on interval colorectal cancer. Gastrointest Endosc. 2017;85(6):1263–1270. .
- Kominami Y, Yoshida S, Tanaka S, et al. Computer-aided diagnosis of colorectal polyp histology by using a real-time image recognition system and narrow-band imaging magnifying colonoscopy. Gastrointest Endosc. 2016;83(3):643–649.
- Ngu WS, Bevan R, Tsiamoulos ZP, et al. Improved adenoma detection with Endocuff Vision: the ADENOMA randomised controlled trial. Gut. 2019;68(2):280–288.
- Repici A, Wallace MB, East JE, et al. Efficacy of per-oral methylene blue formulation for screening colonoscopy. Gastroenterology. 2019;156(8):2198–2207 e1.
- Urban G, Tripathi P, Alkayali T, et al. Deep learning localizes and identifies polyps in real time with 96% accuracy in screening colonoscopy. Gastroenterology. 2018;155(4):1069–1078 e8.
- Misawa M, Kudo SE, Mori Y, et al. Artificial intelligence-assisted polyp detection for colonoscopy: initial experience. Gastroenterology. 2018;154(8):2027–2029 e3.
- Wang P, Xiao X, Glissen Brown JR, et al. Development and validation of a deep-learning algorithm for the detection of polyps during colonoscopy. Nat Biomed Eng. 2018;2(10):741–748.
- Yamada M, Saito Y, Imaoka H, et al. Development of a real-time endoscopic image diagnosis support system using deep learning technology in colonoscopy. Sci Rep. 2019;9(1):14465.
- Becq A, Chandnani M, Bharadwaj S, et al. Effectiveness of a deep-learning polyp detection system in prospectively collected colonoscopy videos with variable bowel preparation quality. J Clin Gastroenterol. 2020;54(6):554–557.
- Klare P, Sander C, Prinzen M, et al. Automated polyp detection in the colorectum: a prospective study (with videos). Gastrointest Endosc. 2019;89(3):576–582 e1.
- Wang P, Berzin TM, Glissen Brown JR, et al. Real-time automatic detection system increases colonoscopic polyp and adenoma detection rates: a prospective randomised controlled study. Gut. 2019;68(10):1813–1819.
- Wang P, Liu X, Berzin TM, et al. Effect of a deep-learning computer-aided detection system on adenoma detection during colonoscopy (CADe-DB trial): a double-blind randomised study. Lancet Gastroenterol Hepatol. 2020;5(4):343–351.
- Yuan Y, Meng MQ. Deep learning for polyp recognition in wireless capsule endoscopy images. Med Phys. 2017;44(4):1379–1389.
- Zhou T, Han G, Li BN, et al. Quantitative analysis of patients with celiac disease by video capsule endoscopy: A deep learning method. Comput Biol Med. 2017;85:1–6.
- Leenhardt R, Vasseur P, Li C, et al. A neural network algorithm for detection of GI angiectasia during small-bowel capsule endoscopy. Gastrointest Endosc. 2019;89(1):189–194.
- Aoki T, Yamada A, Kato Y, et al. Automatic detection of blood content in capsule endoscopy images based on a deep convolutional neural network. J Gastroenterol Hepatol. 2019. DOI:10.1111/jgh.14941.
- Aoki T, Yamada A, Aoyama K, et al. Automatic detection of erosions and ulcerations in wireless capsule endoscopy images based on a deep convolutional neural network. Gastrointest Endosc. 2019;89(2):357–363.e352.
- Fan S, Xu L, Fan Y, et al. Computer-aided detection of small intestinal ulcer and erosion in wireless capsule endoscopy images. Phys Med Biol. 2018;63(16):165001.
- Alaskar H, Hussain A, Al-Aseem N, et al. Application of convolutional neural networks for automated ulcer detection in wireless capsule endoscopy images. Sensors (Basel). 2019;19(6):1265.
- Klang E, Barash Y, Margalit RY, et al. Deep learning algorithms for automated detection of Crohn’s disease ulcers by video capsule endoscopy. Gastrointest Endosc. 2020;91(3):606–613.e602.
- Tsuboi A, Oka S, Aoyama K, et al. Artificial intelligence using a convolutional neural network for automatic detection of small-bowel angioectasia in capsule endoscopy images. Dig Endosc. 2020;32(3):382–390.
- Rex DK, Kahi C, O’Brien M, et al. The American Society for Gastrointestinal Endoscopy PIVI (Preservation and Incorporation of Valuable Endoscopic Innovations) on real-time endoscopic assessment of the histology of diminutive colorectal polyps. Gastrointest Endosc. 2011;73(3):419–422.
- Bisschops R, East JE, Hassan C, et al. Advanced imaging for detection and differentiation of colorectal neoplasia: European Society of Gastrointestinal Endoscopy (ESGE) guideline - Update 2019. Endoscopy. 2019;51(12):1155–1179.
- Iwatate M, Sano Y, Tanaka S, et al. Validation study for development of the Japan NBI expert team classification of colorectal lesions. Dig Endosc. 2018;30(5):642–651.
- Rees CJ, Rajasekhar PT, Wilson A, et al. Narrow band imaging optical diagnosis of small colorectal polyps in routine clinical practice: the Detect Inspect Characterise Resect and Discard 2 (DISCARD 2) study. Gut. 2017;66(5):887–895.
- Byrne MF, Chapados N, Soudan F, et al. Real-time differentiation of adenomatous and hyperplastic diminutive colorectal polyps during analysis of unaltered videos of standard colonoscopy using a deep learning model. Gut. 2019;68(1):94–100.
- Komeda Y, Handa H, Watanabe T, et al. Computer-aided diagnosis based on convolutional neural network system for colorectal polyp classification: preliminary experience. Oncology. 2017;93(Suppl 1):30–34.
- Chen PJ, Lin MC, Lai MJ, et al. Accurate classification of diminutive colorectal polyps using computer-aided analysis. Gastroenterology. 2018;154(3):568–575.
- Song EM, Park B, Ha CA, et al. Endoscopic diagnosis and treatment planning for colorectal polyps using a deep-learning model. Sci Rep. 2020;10(1):30.
- Zachariah R, Samarasena J, Luba D, et al. Prediction of polyp pathology using convolutional neural networks achieves “Resect and discard” thresholds. Am J Gastroenterol. 2020;115(1):138–144.
- Mori Y, Kudo SE, Misawa M, et al. Real-time use of artificial intelligence in identification of diminutive polyps during colonoscopy: a prospective study. Ann Intern Med. 2018;169(6):357–366.
- Kudo SE, Misawa M, Mori Y, et al. Artificial intelligence-assisted system improves endoscopic identification of colorectal neoplasms. Clin Gastroenterol Hepatol. 2019. DOI:10.1016/j.cgh.2019.09.009.
- Hassan C, Senore C, Radaelli F, et al. Full-spectrum (FUSE) versus standard forward-viewing colonoscopy in an organised colorectal cancer screening programme. Gut. 2017;66(11):1949–1955.
- Shiotani A, Honda K, Kawakami M, et al. Analysis of small-bowel capsule endoscopy reading by using Quickview mode: training assistants for reading may produce a high diagnostic yield and save time for physicians. J Clin Gastroenterol. 2012;46(10):e92–95.
- Iakovidis DK, Koulaouzidis A. Automatic lesion detection in capsule endoscopy based on color saliency: closer to an essential adjunct for reviewing software. Gastrointest Endosc. 2014;80(5):877–883.
- Gulshan V, Peng L, Coram M, et al. Development and validation of a deep learning algorithm for detection of diabetic retinopathy in retinal fundus photographs. JAMA. 2016;316(22):2402–2410.
- Esteva A, Kuprel B, Novoa RA, et al. Dermatologist-level classification of skin cancer with deep neural networks. Nature. 2017;542(7639):115–118.
- Zou Y, Li L, Wang Y, et al. Classifying digestive organs in wireless capsule endoscopy images based on deep convolutional neural network. In: 2015 IEEE international conference on Digital Signal Processing (DSP), Singapore; 2015. p. 1274–1278.
- Jia X, Meng MQ. A deep convolutional neural network for bleeding detection in wireless capsule endoscopy images. Conference proceeding IEEE engineering in medicine and biology society, Orlando, Florida, USA; 2016. p. 639–642.
- Seguí S, Drozdzal M, Pascual G, et al. Generic feature learning for wireless capsule endoscopy analysis. Comput Biol Med. 2016;79:163–172.
- He JY, Wu X, Jiang YG, et al. Hookworm detection in wireless capsule endoscopy images with deep learning. IEEE Trans Image Process. 2018;27(5):2379–2392.
- Li P, Li Z, Gao F, et al. Convolutional neural networks for intestinal hemorrhage detection in wireless capsule endoscopy images. In: 2017 IEEE International Conference on Multimedia and Expo (ICME), Hong Kong; 2017. p. 1518–1523.
- Iakovidis DK, Georgakopoulos SV, Vasilakakis M, et al. Detecting and locating gastrointestinal anomalies using deep learning and iterative cluster unification. IEEE Trans Med Imaging. 2018;37(10):2196–2210.
- Ding Z, Shi H, Zhang H, et al. Gastroenterologist-level identification of small-bowel diseases and normal variants by capsule endoscopy using a deep-learning model. Gastroenterology. 2019;157(4):1044–1054.e1045.
- Saito H, Aoki T, Aoyama K, et al. Automatic detection and classification of protruding lesions in wireless capsule endoscopy images based on a deep convolutional neural network. Gastrointest Endosc. 2020. DOI:10.1016/j.gie.2020.01.054.
- Koulaouzidis A, Iakovidis DK, Yung DE, et al. KID project: an internet-based digital video atlas of capsule endoscopy for research purposes. Endosc Int Open. 2017;5(6):E477–E483.
- Aoki T, Yamada A, Aoyama K, et al. Clinical usefulness of a deep learning-based system as the first screening on small-bowel capsule endoscopy reading. Dig Endosc. 2020;32(4):585–591.