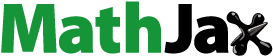
ABSTRACT
Introduction
Fatty liver disease affects almost 30% of the adult population worldwide. Most patients are asymptomatic, and there is not a linear relationship between exposure to risk factors and the risk of developing fibrosis. The combination of a very large, asymptomatic risk population where only a few percent will develop life-threatening liver disease is a growing diagnostic challenge for the health services. Accurate fibrosis assessment in primary care is limited by poor correlation with liver blood tests and low availability of elastography. Non-invasive tests are promising tools, but little is known about their diagnostic accuracy in low-risk populations.
Areas covered
A scoping review was conducted to identify articles that focused on the current use of biomarkers and algorithms in primary care for the detection of patients with fatty liver disease in need of referral for further work-up.
Expert opinion
Currently available algorithms for targeted screening for liver fibrosis perform better than the individual routine liver blood tests or liver ultrasonography. However, primary care physicians urgently need algorithms with even higher diagnostic accuracies than what is available today. The main limitation of the existing widely accessible algorithms, such as the FIB-4, is the large number of false-positive tests, resulting in overdiagnosis and futile referrals to secondary care.
1. Introduction
Fatty liver disease is an overall term for a range of conditions caused by an accumulation of fat in the liver. A 29% prevalence has been estimated among adults worldwide [Citation1]. Traditionally, fatty liver disease has been associated with excessive alcohol consumption (alcohol-related liver disease (ArLD)). In recent decades, the incidence of nonalcoholic fatty liver disease (NAFLD) is growing in parallel with the obesity and type 2 diabetes-epidemic [Citation2]. NAFLD has become the most prevalent chronic liver disease in the world and is present in up to 75% of at-risk populations, being patients with type 2 diabetes and obesity [Citation3]. More than one million deaths annually are attributed to cirrhosis due to fatty liver disease [Citation4], and NAFLD is the second leading indication for liver transplantation in the U.S.A. [Citation5].
Fatty liver disease is usually a silent disease with few or no symptoms, but once the disease advances it can be life threatening. Of patients who reach the stage of cirrhosis, 75% are not diagnosed until they experience their first decompensating event [Citation6]. At this stage, median survival is 3 years [Citation6]. ArLD and NAFLD share common histological liver lesions and often overlap due to the co-occurrence of lifestyle risk factors [Citation7].
The first step in the development from a healthy liver to irreversible cirrhosis is simple steatosis, which is the most common manifestation of both ArLD and NAFLD. Alcoholic steatohepatitis (ASH) and nonalcoholic steatohepatitis (NASH) are inflammatory stages, characterized by ballooned hepatocytes and leukocyte infiltration. The further progression to fibrosis (histological stages F1 for mild fibrosis, F2 for significant fibrosis, and F3 for advanced fibrosis) () marks the main events that predict liver-related morbidity and mortality [Citation8,Citation9] but the disease is still reversible if timely recognized. Ongoing inflammation and scarring finally lead to cirrhosis (histological stage F4) (). Only 2–5% of ArLD patients and 1–2% of NAFLD patients ever progress to F3 and F4, but without a clear linear relationship between exposure to risk factors and the risk of fibrosis [Citation10].
Figure 1. Fatty liver starts off as simple steatosis, which further can trigger inflammation and damage of the liver cells, potentially resulting in fibrosis of the liver. Further progression of the disease may lead to the end-stage of irreversible liver cirrhosis.
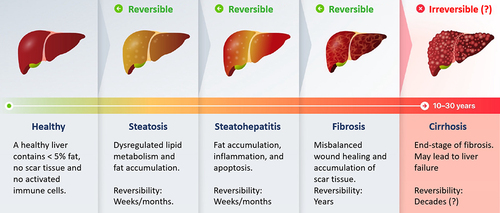
For decades, liver biopsy has been the reference standard for the assessment of liver fibrosis, but currently, transient elastography is taking over as the new reference standard to measure liver stiffness as a surrogate marker for liver fibrosis. Both methods are still primarily restricted to secondary care [Citation11].
In primary care, laboratory tests play an important role in assessing the liver health of patients. Alanine transaminase (ALT) is a common blood sample performed. ALT is an indicator of hepatocellular injury, and studies have demonstrated that high ALT levels are correlated with higher risk of having steatohepatitis [Citation12]. However, the sensitivity of ALT to detect ≥F3 is only 53%, making it a poor indicator of today’s challenge [Citation13].
Standard ultrasonography may be used as a diagnostic tool in primary care and can detect the two cornerstones of the disease spectrum, moderate-to-severe steatosis and contrary, severe cirrhosis, but is less useful in the case of early detection of mild to advanced fibrosis [Citation14,Citation15]. In contrast, FibroScan, a noninvasive imaging technique based on transient elastography, can provide quantitative measurements of liver stiffness, which correlates with the degree of fibrosis. FibroScan emerges as a valuable tool for the early detection and monitoring of liver fibrosis but is still not used in primary care.
Nonetheless, to be able to combat the rising burden of fatty liver disease, we need an investigation strategy for early detection of liver fibrosis, which is both economically efficient and acceptable to patients. In recent decades, biomarkers, algorithms, and machine learning have actively been investigated and may prove useful in primary care, to select the patients requiring further consultations.
The aim of this scoping review was to summarize the current evidence regarding biomarkers and algorithms used as diagnostic tools for the detection of fatty liver disease in primary care.
2. Methods
This scoping review was based on the Preferred Reporting Items for Systematic Reviews and Meta-analysis Protocols (PRISMA-ScR) for Scoping Reviews [Citation16]. We included papers that focused on the current use of biomarkers and algorithms for the detection of patients with fatty liver disease in primary care. Studies on at-risk populations and the general population were included, together with studies for staging fatty liver disease in primary care. Any methodology and study design were accepted.
We chose to limit the search to articles written in English and German. We searched the following databases: Embase, Ovid MEDLINE, Cochrane Library, Scopus, and Google Scholar, to ensure access to a wide spectrum of journals. The search was made without any restriction on the publication date, and the most recent search was conducted on 25 May 2022. The search strategy was drafted by a team discussion and further refined by an experienced librarian. The Boolean-search-string used is shown in Appendix, and it resulted in selection of 917 papers.
2.1. Exclusion criteria
Studies based on secondary care were excluded, since the aim of the review was to extract knowledge from primary care. In addition, reviews, papers on treatment and prognosis, clinical guidelines, and pediatric populations were excluded, together with editorials, conference abstracts, and papers related to other liver diseases than fatty liver diseases, such as hepatocellular carcinoma or viral hepatitis.
Two reviewers independently screened the titles and abstracts of all retrieved records. The Rayyan.ai online software was used for the review process. In case of disagreement, the two reviewers independently examined the abstracts again and thereafter made a joint agreement. Then, two other reviewers examined the full text of the studies selected by abstract. Where a difference in selection occurred, disagreements were resolved through a discussion between all the four reviewers.
3. Results
The selection process for the included articles is illustrated in . As shown, we identified 917 manuscripts of which 19 papers met the inclusion and exclusion criteria for the study (). The excluded papers were mainly the wrong type of publications and studies from secondary care. There was an increase in the number of published papers over the last 2 years.
Table 1. Overview of the included papers.
3.1. The included papers grouped by strategy and population
Since our focus is the current knowledge of biomarkers and algorithms used as diagnostic tools in primary care, we have taken a clinical approach and looked at the heterogenous NAFLD risk population rather than the histological subgroups of the disease. An effective investigation strategy should help us identify patients at intermediate or high risk of established fibrosis, due to the large proportion of liver steatosis in the general population.
3.1.1. Identification of patients with NAFLD (specifically fibrotic NASH) among risk groups
The Fibrotic NASH Index (FNI) was developed to detect fibrotic NASH (defined as NASH, NAFLD activity score ≥4 and ≥F2) among individuals at high risk for NAFLD [Citation17]. FNI is based on routine blood tests including aspartate aminotransferase (AST) and dysmetabolic parameters (). The derivation cohort included morbidly obese individuals and three external validation cohorts included two high-risk cohorts and participants from a cohort study. The diagnosis of fibrotic NASH was assessed by liver biopsy, liver stiffness measurement (LSM), and liver magnetic resonance imaging. The study found that the FNI predicted fibrotic NASH with an AUROC of 0.78 (95% CI 0.71–0.85) and outperformed FIB-4 in predicting fibrotic NASH.
Table 2. Overview of algorithms for the diagnosis of fatty liver disease and their characteristics and performance.
3.1.2. Characterize patients with recognized NAFLD in primary care
Two studies aimed to characterize patients with recognized NAFLD in primary care, to estimate the clinical significance of the disease.
The steatosis-associated fibrosis estimator score (SAFE) consists of age, BMI, status of diabetes, and four standard blood tests [Citation18] (). The prediction model was constructed based on data from patients with biopsy-proven NAFLD. The model was then evaluated in three validation cohorts, ranging from selected patients with biopsy-proven NASH, to real-world patients with NAFLD who underwent LSM by magnetic resonance elastography, to a sample from the general population with an ultrasound-diagnosis of liver steatosis. The aim was to distinguish ≥F2 from F0/1, and the model yielded AUROC ≥ 0.80, performed consistently higher than FIB-4 and NAFLD-fibrosis score and presented a negative predictive value in ruling out ≥F2 of 88–92%.
In the second study, steatosis was semi-quantitatively assessed using ultrasound and a scale from 0 to 4 (absent, mild, moderate, severe) [Citation15]. Then, Fibromax () and Fatty Liver Index (FLI) () were applied to patients with clinical and ultrasonographic features of NAFLD. The Fibromax algorithm provides information on the whole disease spectrum of disease, including liver fat infiltration (scored 0–3 (with Steatotest)), grade of inflammation (scored absent, borderline, present (with NASHtest)), and stage of fibrosis (scored 0–4 (with Fibrotest)).
The study concluded that ultrasound (US) poorly defined the grade of fat infiltration compared to Steatotest, since 30% of patients with Steatotest 3 were graded mild-to-moderate steatosis at ultrasound. Furthermore, ultrasound showed poor accuracy for diagnosing fibrosis (sensitivity 77%, specificity 89%). An important finding was that only 60% of the enrolled patients had hypertransaminasemia.
3.1.3. Screen for NAFLD in low-prevalence populations
Six studies were identified with the target of screening for NAFLD in low-prevalence populations. A common denominator for this target is the use of routinely collected blood samples. The NAFLD ridge score () originates from Hong Kong and consists of the presence of hypertension and five standard blood tests [Citation19]. It was developed and tested with the purpose of identifying patients with and without NAFLD, distinguished by intrahepatic fat content of more or less than 5%, determined by proton-magnetic resonance spectroscopy. The NAFLD ridge score had an AUROC of 0.88 (95% CI 0.84–0.91). The study concluded that the score was comparable to existing NAFLD scores in the ability to exclude NAFLD, based on its NPV of 96%, but the study did not directly compare the NAFLD ridge score to other existing NAFLD prediction models.
The CIRRhosis Using Standard tests (CIRRUS) () was created in the UK, from patients who underwent upper gastrointestinal endoscopy [Citation20]. CIRRUS predicted a first serious liver-related event within 5 years from the performed tests, with an AUROC of 0.90 (95% Cl 0.88–0.92) in the mixed cohort from primary and secondary care and an AUROC of 0.84 (95% CI 0.82–0.86) in the cohort from primary care alone.
A Danish study aimed to predict significant liver fibrosis, defined as LSM > 8 kPa, using routinely available patient data and artificial intelligence [Citation21]. The study population consisted of subjects at risk of NAFLD or ArLD or subjects randomly selected from the general population. Six LiverAID-models (XXS-4XL) with different complexities appropriately identified patients with elevated liver stiffness with AUROC 0.86–0.94 and NPV ≥ 0.98. LiverAID XXS () showed an AUROC of 0.86 and NPV 0.98 in a head-to-head comparison with FIB-4, Forns index, and APRI. Compared to LSM, LiverAID showed a good ability to rule out significant biopsy-assessed fibrosis stages.
In another study from the UK, patients had their liver function tests (bilirubin, albumin, ALP, GGT, ALT, or AST) performed in primary care and were followed for 2 years [Citation22]. The study population was initially derived from a laboratory database, and the validation cohort contained all patients registered in 19 practices in primary care. The ALFI model () is a prediction model to estimate the risk of having a liver disease diagnosis, based on the tests performed. In the external cohort, 0.5% were diagnosed with liver disease within 2 years from the test. Given the low prevalence of liver disease, the negative predictive values were high (almost 100%). Positive predictive values were low but increased to 20–30% for high-risk patients when this model was used. The AUROC for the final model applied to the external cohort was 0.78 (95% Cl 0.72–0.84).
In one study FIB-4 () and NAFLD Fibrosis score () were tested for their ability to detect hepatic fibrosis in low-prevalence populations [Citation23]. The participants were from the general population and from at-risk cohorts due to alcohol, diabetes, or obesity. They found that LSM was correlated with FIB-4 and NAFLD Fibrosis score, but as many as 28–29% of elevated FIB-4 and NAFLD Fibrosis scores were false-positives. Eleven percent of patients had LSM ≥ 8 kPa, though 43% of these had a normal FIB-4 and 31% had a normal NAFLD Fibrosis score. The proportion of false negatives was higher in at-risk patients than in the general population (8–9% vs 2–4%). For screening purposes, both tests turned out to be suboptimal because of futile and missed referrals.
A Finnish study extracted data from national health examination surveys to develop a risk-prediction model for chronic liver disease in the general population, the Chronic Liver Disease Score (CLivD) [Citation24] (). The prediction model includes age, sex, alcohol use, waist-hip ratio, diabetes, and smoking, with or without GGT, and it was externally validated in population studies in Denmark and the UK. Study endpoints were fatal and non-fatal advanced liver disease. The 15-year AUROC for the model that included GGT was 0.84 (95% CI 0.75–0.93) in the derivation cohort, and 0.78 (95% CI 0.68–0.87) in the validation cohort from Denmark.
3.1.4. Sequential testing of high-risk populations
The following seven studies used sequential testing based on a preselection of patients with an increased risk of liver fibrosis.
A Spanish population-based cohort study evaluated the accuracy of nine NITs (FLI, NAFLD Fibrosis score, metabolic syndrome criteria (MS criteria), Forns index, APRI, BARD, FIB-4, eLIFT, and AST/ALT) () to detect ≥F2 (LSM cut off >9.2/7.8 for M/XL probe) in the general population [Citation25]. Medical history, fasting blood tests, metabolic comorbidities, and alcohol risk consumption were registered, and LSM was performed. FLI and NAFLD Fibrosis score were the best performing tests, with AUROCs for ≥F2 of 0.89 (95% CI 0.84–0.95) and 0.82 (95% CI 0.74–0.90), respectively. AST/ALT had the lowest AUROC for prediction of ≥F2 (0.51 (95% CI 0.50–0.74)). The LSM ≥F2 estimate was 1.9% of which 75% of the subjects showed normal transaminases. A staged risk-stratification model improved the detection of ≥F2 and reduced unnecessary referrals.
In a study conducted on patients from both primary care and endocrinology practices, patients with either the diagnosis of type 2 diabetes or two other metabolic risk factors were included [Citation26]. Three NITs (APRI, FIB-4, and NAFLD Fibrosis Score) () were calculated to identify patients with high risk of fibrosis, and those who met the noninvasive test criteria were referred for LSM. The criterion for high-risk NAFLD (presumed high-risk NASH) was to have at least two NITs above certain thresholds (APRI > 1, NFS >-1.45, and FIB-4 >1.45). The study found that 60% of the included patients had LSM < 6 kPa and could be considered at minimal risk of adverse outcomes, and 8% had an LSM ≥ 12 kPa, indicating potentially advanced liver fibrosis. The study concluded that each single NIT had a limited accuracy, but a stepwise application of an algorithm using NITs and LSM could be used to identify patients with high-risk NAFLD.
Another study developed and tested an algorithm, called ADAPT (), consisting of age, type 2 diabetes, platelets, and plasma Pro-C3 (N-terminal type III collagen propeptide) [Citation27]. Pro-C3 is a new collagen biomarker, used to predict fibrogenesis and monitor disease progression. In the hospital-based cohorts, Pro-C3 increased with fibrosis stage (P < 0.0001). ADAPT showed AUROC of 0.88 (95% CI 0.77–0.95) for ≥F3 and AUROC 0.81 (95% CI 0.76–0.86) for ≥F2, which was superior to the existing fibrosis scores (APRI (P = 0.001), FIB-4 (P = 0.001), BARD (P = 0.001), NAFLD Fibrosis score (P = 0.04), and comparable with LSM (AUC 0.83 95% CI 0.77–0.88, P = 0.1). Serial combination of ADAPT with LSM had diagnostic accuracy of 93% and NPV of 100% for excluding ≥F3, improving risk stratification and decreasing the need for liver biopsy.
A study integrated a pathway of liver fibrosis assessment into the routine annual review of all patients with diabetes type 2 in primary care, using FIB-4 and LSM [Citation28]. Diabetics with a FIB-4 above the threshold >1.3/2.0 (age below/above 65 years) underwent LSM and were reviewed in secondary care if LSM was >8 kPa. Out of the 12% that were referred for LSM, 43% had an LSM >8 kPa and 22% had an LSM >15 kPa. Following a specialist review, 4.5% were found to have advanced liver fibrosis. From a cohort of 475 patients, 20 new cases of advanced liver disease were identified because of the pathway, representing almost a 7-fold increase from standard of care. Another important finding was that almost half of patients diagnosed with advanced liver disease had a normal ALT level, and only one in five patients with an abnormal ALT level were identified as having advanced liver disease.
Another study developed a two-step algorithm from participants with one or more risk factors identified as 1) hazardous alcohol use, 2) type 2 diabetes, and 3) persistently elevated ALT (other causes of chronic liver disease were excluded) [Citation29]. The biomarker AST/ALT-ratio was performed in group 1 and BARD-score () in groups 2 and 3; subsequently, liver stiffness was measured using LSM. Clinically significant liver disease was defined as LSM ≥8 kPa. A normal biomarker was found in 12%, which required no further investigation and 27% showed LSM ≥8 kPa. Importantly, 72% of patients with elevated liver stiffness had normal liver enzymes and would be missed by traditional investigation algorithms, and 91% diagnosed with fibrosis had normal ALT. There was also a 140% increase in new patients with cirrhosis.
A pathway was tested for patients with an ultrasound-diagnosis of NAFLD. Participants were first screened with FIB-4 and those with values >3.25 were recommended for referral to secondary care [Citation30]. Intermediate values (≥1.30 and <3.25) had second tier testing with an Enhanced Liver Fibrosis test (ELF test) (). Patients with ELF ≥ 9.5 were recommended for referral to secondary care. Use of the pathway reduced unnecessary referrals to secondary care by 81% and detected 5 times more cases of ≥F3. Still, 70% of referrals were considered unnecessary.
A study, on a population which was at the limit of our scope, was included due to important findings relevant for primary care. The patients were recruited from municipal alcohol rehabilitation centers and hospital liver clinics [Citation31]. All patients were at a significant risk of fibrogenic ArLD, which justified performing a liver biopsy. The study did a comparison of the ELF-test, the FibroTest, LSM, two-dimensional shear wave elastography, and six indirect serum markers (Age-platelet index, AST/ALT ratio, APRI, FIB-4, Forns index, and GGT-to-platelet ratio), in the detection of ≥F3. The ELF test identified patients with ≥F3 with an AUROC of 0.92 (95% Cl 0.89–0.96). The ELF test was more accurate than indirect markers, but ELF test and FibroTest had comparable diagnostic accuracies (AUROC of FibroTest, 0.90 (95% CI 0.86–0.94)). The results from the ELF test and FibroTest () did not differ significantly from those of LSM (AUROC 0.89 (95% CI 0.83–0.96)). Adding a serum marker to LSM did not increase the accuracy. For patients in primary care, ELF values below 10.5 and FibroTest values below 0.58 had negative predictive values for advanced liver fibrosis of 98% and 94%, respectively.
3.1.5. Usage of two-dimensional shear wave elastography for staging NAFLD in primary care
One study used two-dimensional shear wave elastography in primary care to risk-stratify patients with suspected NAFLD and compared it to FIB-4 [Citation32]. Most patients (92%) with NAFLD had an LSM value <8.0 kPa and were not referred to secondary care. Elevated liver stiffness was found in 8.5%, and they were subsequently referred for further diagnosis. Screening of the same population with FIB-4 (cutoff >1.3) would have led to a referral rate of 32%.
3.1.6. Association between biomarkers and fatty liver disease
Two studies tested markers for the detection of fatty liver disease. One study found that the calculation of the Lipid Accumulation Product (LAP) () was helpful to select subjects for ultrasound [Citation33]. The odds of more severe steatosis increased for increasing values of LAP (OR 4.28 95% CI 3.28–5.58). Another study considering Fibromax as the gold standard showed that adiponectin was an independent negative correlate of borderline NASH (OR: 0.92 95% CI 0.86–0.98/1 μg/ml), together with high-density lipoprotein, and leptin was a positive correlate (OR: 1.03; CI 1.01–1.06/1 ng/ml) [Citation34].
4. Discussion
4.1. General summary of main findings
This scoping review highlights the lack of efficient and standardized pathways for timely detection of advanced fatty liver disease. The current diagnostic tools used in primary care demonstrate low accuracy, emphasizing the need for a more precise first-tier test to correctly identify patients requiring further referral to specialist care. Existing algorithms used for diagnosis may lead to delays in detecting treatable yet life-threatening conditions and unnecessary referrals, resulting in harm to healthy individuals and the wastage of healthcare resources [Citation35,Citation36].
However, the utilization of noninvasive tests and algorithms offers promising solutions to address these challenges. These tests and algorithms can play a crucial role in reducing secondary care referrals by accurately identifying patients who truly require specialized intervention. By implementing efficient and accurate diagnostic tools, such as noninvasive biomarkers and advanced algorithms, primary care physicians can optimize the allocation of limited secondary care resources and provide timely interventions for individuals with fatty liver disease.
While only a small proportion of individuals with fatty liver disease progress to end-stage cirrhosis, the literature acknowledges the anticipated increase in the burden of liver disease and supports the identification of patients with fatty liver disease in primary care. General practitioners, who encounter most at-risk patients, commonly request liver function tests as part of routine screening. However, it is important to note that alanine transaminase (ALT) alone is not a reliable predictor of fibrosis or cirrhosis [Citation13]. Several studies included in this review reveal that patients with normal transaminase levels, but elevated liver stiffness measurements (LSM) would be missed by traditional investigation algorithms [Citation15,Citation28,Citation29]. Therefore, establishing a trusting relationship between patients and their primary care physician becomes crucial in identifying patients with fatty liver disease, as it allows for the identification of risk factors beyond relying solely on abnormal blood test results.
Within the reviewed studies, various noninvasive tests (NITs) have been developed to identify patients with advanced fibrosis using clinical and biochemical data. These tests, along with the utilization of advanced algorithms, can enhance the accuracy and efficiency of early detection in primary care. However, it is important to acknowledge potential limitations within the included studies, indicating the need for further research and refinement of these tests (28).
Most of the NITs are derived from a secondary care level, on already selected patients. This constitutes a risk of selection bias and may make the models less useful in primary care. Another limitation of this method is the spectrum effect, where models and algorithms are developed in high prevalence populations and validated in low prevalence populations. This may lead to an overestimation of the diagnostic accuracy. Normally, AUC values are used to assess the performance of NITs for fatty liver disease screening. However, in the context of screening in low-prevalence populations, the consideration of positive predictive values (PPVs) in NITs becomes advantageous as it optimizes resource utilization, reduces patient burden, and enhances clinical decision-making. PPVs represent the proportion of individuals with positive test results who truly have the condition, providing valuable information on accuracy and clinical utility. Information on alcohol consumption, smoking, and anthropometric measures, such as waist circumference or waist-hip-ratio, are often unknown or underreported. Several NITs depend on this information (), making them less reliable for clinical use, with a high risk of poor compliance to the NIT. Some of the algorithms include patented blood tests, not routinely performed, and limited by price and availability (e.g. ELF, Fibromax, and ADAPT) (). The algorithms that rely on fasting blood tests () have a potential source of inaccuracy when used in primary care. Interpreting NITs’ results requires careful examination to prevent false-positive or false-negative outcomes. Acute hepatitis may affect algorithms that include aminotransferases, and hemolysis or Gilbert’s syndrome can affect algorithms using bilirubin, leading to false-positive results [Citation37,Citation38]. The NAFLD fibrosis score requires manual calculation, making it more difficult and time-consuming for general practitioners to use. Three of the included studies lack a gold standard or a surrogate marker, such as TE, to evaluate the diagnostic precision of the tests [Citation15,Citation33,Citation34]. The two studies that looked at the association between fatty liver disease, LAP, leptin, and adiponectin did not succeed in selecting patients for further referral, because of an odds ratio close to 1 [Citation33,Citation34].
FIB-4 is considered a simple and low-cost tool for testing at-risk patients in primary care, but four studies have shown a high possibility of overdiagnosis and a significant percentage of false negatives [Citation23,Citation26,Citation32]. FNI was found to outperform FIB-4 in predicting fibrotic NASH (defined as NASH, NAFLD activity score ≥4 and fibrosis stage ≥2) in ⅔ of the cohorts [Citation17], but the 1.3 cutoff of FIB-4 has been designed to rule out advanced fibrosis rather than fibrotic NASH. The study that compared two-dimensional shear wave elastography in primary care to FIB-4 (30) showed a large proportion of redundant referrals when a risk population was screened with FIB-4. An interesting finding was that waist circumference outperformed FIB-4 for detection of patients with LSM ≥8kPa, in the general population, but not in at-risk cohorts [Citation23].
Studies support the effectiveness of transient elastography as a reliable indicator of liver fibrosis in populations with low prevalence [Citation32,Citation39]. However, this examination is limited to specialist healthcare due to the high cost of equipment, training requirements, and variability in results between observers.
Sequential testing with one or more NIT(s) on a preselected population, followed by LSM, increases the number of detected patients with advanced fibrosis and reduces the number of false positives. But this method also creates a risk of over-referrals, indicating that the NITs should be used for ruling out, rather than diagnosing advanced fibrosis in low prevalence populations.
Another important consideration is the heterogeneity of different patient populations when evaluating the effectiveness of tools for detecting NAFLD, because various factors such as genetic variations, lifestyle choices, and regional differences can significantly influence the presentation and progression of the disease [Citation40].
4.2. Comparison with current guidelines
Our findings are in line with the clinical guidelines from the European Association for the Study of the Liver [Citation41] and the American Association for the study of Liver Diseases [Citation42]. The guidelines recommend that NITs should be used for ruling out, rather than diagnosing advanced fibrosis in low prevalence populations. To reduce false positives, at least a two-tier pathway is recommended before referral to secondary care, either using LSM or ELF-test. However, strict application of existing guidelines would result in a likely over-referral to hepatologists, with considerable variation based on the specific biomarker [Citation43].
4.3. Future directions
The worldwide increase in metabolic disorders will lead to a significant rise in the clinical and economic impact of fatty liver disease. Despite this, awareness of the disease remains low, with fewer than 5% of people with NAFLD being aware of their liver’s health [Citation44]. General practitioners have good opportunities for identifying at-risk patients, seek secondary care if needed, monitor biochemistry, and address risk factors to slow the progression of the disease toward cirrhosis. To manage this, there is a requirement for increased education, targeting both future and current primary care physicians. Additionally, a more accurate tool and a standardized process for patient identification are necessary. This tool should be designed for primary care, utilizing existing resources, at a low cost. Future research is necessary to develop, validate, and assess the cost-effectiveness of NITs from a primary care perspective.
5. Conclusion
Efficient referral pathways for detecting advanced liver disease are lacking, and existing noninvasive tests (NITs) in primary care lack accuracy. A more precise and cost-effective test is needed for identifying patients requiring specialist care. Integrating noninvasive tests and algorithms in primary care has the potential to improve detection and reduce unnecessary secondary referrals. Further research and refinement are essential to enhance their accuracy and enable effective interventions in healthcare.
6. Expert opinion
Fatty Liver Disease is a common condition that is characterized by the accumulation of fat in the liver. It is a widespread condition that is associated with metabolic disorders such as obesity, insulin resistance, and dyslipidemia. The prevalence of Fatty Liver Disease is increasing globally, and it has become a significant public health concern. Unfortunately, the current tools available to primary care physicians to manage and detect Fatty Liver Disease have their limitations. While individual routine liver blood tests and liver ultrasonography can help identify patients with Fatty Liver Disease, they are not sensitive enough to detect early stages of liver fibrosis. Early detection of liver fibrosis is crucial as it can progress to more severe liver diseases such as cirrhosis and even cancer. Liver fibrosis is a condition where there is excessive scarring in the liver, which can impair liver function and eventually lead to liver failure. It is a critical stage in the progression of liver disease, and its early detection is essential for effective management and prevention of further progression. However, detecting liver fibrosis is challenging, and traditional methods such as liver biopsy are invasive and can cause complications.
To address these limitations, researchers have developed algorithms for targeted screening of liver fibrosis, such as the FIB-4 score. These algorithms combine various parameters such as age, liver enzyme levels, and platelet count to identify patients at high risk of liver fibrosis. While targeted screening algorithms have been shown to perform better than individual liver tests, they still have limitations, including a high rate of false-positive tests.
Primary care physicians urgently need more accurate algorithms for targeted screening of liver fibrosis. These algorithms should be cost-effective, easy to use, and widely accessible to allow for early detection and appropriate management of patients with Fatty Liver Disease and liver fibrosis. Research efforts should focus on developing algorithms that balance sensitivity and specificity to minimize false-positive and false-negative results.
In addition to accurate screening algorithms, the identification of patients at risk of developing Fatty Liver Disease and liver fibrosis is equally important. General practitioners have a crucial role in identifying at-risk patients and providing appropriate monitoring and interventions to prevent disease progression. Increased education targeting both future and current primary care physicians is necessary to improve awareness and knowledge of Fatty Liver Disease.
In conclusion, Fatty Liver Disease is a widespread condition that is associated with metabolic disorders, and the current tools available to primary care physicians for detecting and managing Fatty Liver Disease have limitations. Early detection of liver fibrosis is crucial, and accurate algorithms for targeted screening of liver fibrosis are critical to prevent disease progression and improve outcomes for patients with Fatty Liver Disease. Primary care physicians urgently need more accurate and widely accessible algorithms to enable early detection and appropriate management of patients with NAFLD and liver fibrosis. Further research efforts should focus on developing algorithms that balance sensitivity and specificity to minimize false-positive and false-negative results. Additionally, increased education and awareness among primary care physicians are necessary to improve the identification of at-risk patients and provide appropriate monitoring and interventions.
Article highlights
NAFLD has become the most prevalent chronic liver disease in the world and is present in up to 75% of at-risk populations.
Non-invasive tests and advanced algorithms can enhance the accuracy and efficiency of early detection of advanced fibrosis in primary care.
A trusting relationship between patients and their primary care physician can allow for the identification of risk factors beyond relying solely on abnormal blood test results.
Existing algorithms used for diagnosis may both lead to delays in detecting advanced fibrosis and unnecessary referrals, resulting in harm to healthy individuals and the wastage of healthcare resources.
A more precise and cost-effective test is needed for identifying patients requiring specialist care.
Abbreviations
ALFI | = | The Algorithm for Liver Function Investigations |
ALP | = | Alkaline phosphatase |
ALT | = | Alanine transaminase |
APRI | = | The AST to Platelet Ratio Index |
ArLD | = | Alcohol-related liver disease |
ASH | = | Alcoholic steatohepatitis |
AST | = | Aspartate Aminotransferase |
AUROC | = | The area under the receiver operating characteristic |
BMI | = | Body mass index |
CI | = | Confidence interval |
CIRRUS | = | The CIRRhosis Using Standard tests |
CLivD | = | The Chronic Liver Disease score |
ELF | = | Enhanced Liver Fibrosis Test |
F1 | = | Mild fibrosis |
F2 | = | Significant fibrosis |
F3 | = | Advanced fibrosis |
F4 | = | Cirrhosis FIB-4: The Fibrosis-4 |
FLI | = | Fatty Liver Index |
FNI | = | The Fibrotic NASH Index |
GGT | = | Gamma-glutamyltransferase |
HbA1c | = | Hemoglobin A1c |
HDL | = | High-density lipoprotein |
INR | = | International Normalized Ratio, |
kPa | = | Kilopascal, LAP: Lipid accumulation product |
MCV | = | Mean corpuscular volume |
LSM | = | Liver stiffness measurement Na: Sodium |
NAFLD | = | Non-alcoholic liver disease |
NASH | = | Non-alcoholic steatohepatitis |
NIT | = | Non-invasive tests |
NPV | = | Negative predictive value |
PPV | = | Positive predictive value |
SAFE | = | The Steatosis Associated Fibrosis Estimator score |
TE | = | Transient elastography |
TG | = | Triglycerides |
T2D | = | Type 2 diabetes |
US | = | Ultrasound |
WC | = | Waist circumference |
WBC | = | White blood cells. |
Declaration of interests
The authors have no relevant affiliations or financial involvement with any organization or entity with a financial interest in or financial conflict with the subject matter or materials discussed in the manuscript. This includes employment, consultancies, honoraria, stock ownership or options, expert testimony, grants or patents received or pending, or royalties.
Reviewer disclosures
Peer reviewers on this manuscript have no relevant financial or other relationships to disclose.
Acknowledgments
The authors would like to thank university librarian Geir Strandenæs Larsen at the Research Department at Stavanger University Library for his help and quality assurance of the literature search.
Additional information
Funding
References
- Zobair Younossi PG, Golabi P, Paik J, et al. The most recent and in-depth meta-analytic assessment of the global epidemiology of non-alcoholic fatty liver disease (NAFLD). J Hepatolo, Abstract Book. 2022;77:S144–S145. doi: 10.1016/S0168-8278(22)00668-7
- Younossi Z, Anstee QM, Marietti M, et al. Global burden of NAFLD and NASH: trends, predictions, risk factors and prevention. Nat Rev Gastroenterol Hepatol. 2018;15(1):11–20. doi: 10.1038/nrgastro.2017.109
- Cotter TG, Rinella M. Nonalcoholic fatty liver disease 2020: the state of the disease. Gastroenterology. 2020;158(7):1851–1864. doi: 10.1053/j.gastro.2020.01.052
- Asrani SK, Kouznetsova M, Ogola G, et al. Increasing health care burden of chronic liver disease compared with other chronic diseases, 2004–2013. Gastroenterology. 2018 Sep;155(3):719–729.e4. doi: 10.1053/j.gastro.2018.05.032
- Chedid MF. Nonalcoholic steatohepatitis: the second leading indication for liver transplantation in the USA. Dig Dis Sci. 2017;62(10):2621–2622. (1573–2568 (Electronic)). doi: 10.1007/s10620-017-4724-6
- Dam Fialla A, de Muckadell OB S, Touborg Lassen A. Incidence, etiology and mortality of cirrhosis: a population-based cohort study. Scand J Gastroenterol. 2012 Jun;47(6):702–709. doi: 10.3109/00365521.2012.661759
- Idalsoaga F, Kulkarni AV, Mousa OY, et al. Non-alcoholic fatty liver disease and alcohol-related liver disease: two intertwined entities. Front Med (Lausanne). 2020;7: (2296–858X (Print)).
- Lackner C, Spindelboeck W, Haybaeck J, et al. Histological parameters and alcohol abstinence determine long-term prognosis in patients with alcoholic liver disease. J Hepatol. 2017 Mar;66(3):610–618. doi: 10.1016/j.jhep.2016.11.011
- Ekstedt M, Hagström H, Nasr P, et al. Fibrosis stage is the strongest predictor for disease-specific mortality in NAFLD after up to 33 years of follow-up. Hepatology. 2015 May;61(5):1547–1554. doi: 10.1002/hep.27368
- Younossi Z, Henry L. Contribution of alcoholic and nonalcoholic fatty liver disease to the burden of liver-related morbidity and mortality. Gastroenterology. 2016;150(8):1778–1785. (1528–0012 (Electronic)). doi: 10.1053/j.gastro.2016.03.005
- Sandrin L, Fourquet B, Fau - Hasquenoph J-M, et al. Transient elastography: a new noninvasive method for assessment of hepatic fibrosis. Ultrasound Med Biol. 2003;29(12):1705–1713. (0301–5629 (Print)). doi: 10.1016/j.ultrasmedbio.2003.07.001
- Ulasoglu C, Enc FY, Kaya E, et al. Characterization of patients with biopsy-proven non-alcoholic fatty liver disease and normal aminotransferase levels. J Gastrointestinal and Liver Dis. 2019;28(4):427–431. (1842–1121 (Electronic)). doi: 10.15403/jgld-293
- Thong VD, Quynh BTH. Correlation of serum transaminase levels with liver fibrosis assessed by transient elastography in Vietnamese patients with nonalcoholic fatty liver disease. Int J Gen Med. 2021;14:1349–1355. doi: 10.2147/IJGM.S309311
- Allan R, Thoirs K, Fau - Phillips M, et al. Accuracy of ultrasound to identify chronic liver disease. World J Gastroenterol. 2010;16(28):3510. (2219–2840 (Electronic)). doi: 10.3748/wjg.v16.i28.3510
- Grattagliano I, Ubaldi E, Fau - Napoli L, et al. Utility of noninvasive methods for the characterization of nonalcoholic liver steatosis in the family practice. The “VARES” Italian multicenter study. Ann Hepatol. 2013;12(1):70–77. (1665–2681 (Print)). doi: 10.1016/S1665-2681(19)31387-0
- Tricco AC, Lillie E, Zarin W, et al. PRISMA extension for scoping Reviews (PRISMA-ScR): checklist and explanation. Ann internal med. 2018;169(7):467–473. (1539–3704 (Electronic)). doi: 10.7326/M18-0850
- Tavaglione F, Jamialahmadi O, De Vincentis A, et al. Development and validation of a score for fibrotic nonalcoholic steatohepatitis. Clin Gastroenterol Hepatol. 2023;21(6):1523–1532.e1. (1542–7714 (Electronic)). doi: 10.1016/j.cgh.2022.03.044
- Sripongpun P-O, Kim W-O, Mannalithara A, et al. The steatosis-associated fibrosis estimator (SAFE) score: a tool to detect low-risk NAFLD in primary care. Hepatology. 2023;77(1):256–267. (1527-3350 (Electronic)). doi: 10.1002/hep.32545
- Yip TC, Ma AJ, Wong VW-S, et al. Laboratory parameter-based machine learning model for excluding non-alcoholic fatty liver disease (NAFLD) in the general population. Aliment Pharmacol Ther. 2017;46(4):447–456. (1365–2036 (Electronic)). doi: 10.1111/apt.14172
- Hydes T, Moore M, Stuart B, et al. Can routine blood tests be modelled to detect advanced liver disease in the community: model derivation and validation using UK primary and secondary care data. BMJ Open. 2021 Feb 11;11(2):e044952. doi: 10.1136/bmjopen-2020-044952
- Blanes-Vidal V, Lindvig KP, Thiele M, et al. Artificial intelligence outperforms standard blood-based scores in identifying liver fibrosis patients in primary care. Sci Rep. 2022 Feb 21;12(1):2914. doi: 10.1038/s41598-022-06998-8
- McLernon DJ, Donnan PT, Sullivan FM, et al. Prediction of liver disease in patients whose liver function tests have been checked in primary care: model development and validation using population-based observational cohorts. BMJ Open. 2014;4(6):e004837–e004837. (2044–6055 (Print)). doi: 10.1136/bmjopen-2014-004837
- Graupera I, Thiele M, Serra-Burriel M, et al. Low accuracy of FIB-4 and NAFLD fibrosis scores for screening for liver fibrosis in the population. Clin Gastroenterol Hepatol. 2022 Nov;20(11):2567–2576. doi: 10.1016/j.cgh.2021.12.034
- Åberg F, Luukkonen PK, But A, et al. Development and validation of a model to predict incident chronic liver disease in the general population: the CLivD score. J Hepatol. 2022 Aug;77(2):302–311. doi: 10.1016/j.jhep.2022.02.021
- Coste P, Llop E, Perelló C, et al. Comparison of non-invasive fibrosis scores to predict increased liver stiffness in the general population with unknown liver disease: searching for the primary physician’s best friend. Digestive Liver Dis. 2022;54(9):1209–1214. (1878–3562 (Electronic)). doi: 10.1016/j.dld.2022.03.013
- Younossi ZM, Pham H, Felix S, et al. Identification of high-risk patients with nonalcoholic fatty liver disease using noninvasive tests from primary care and endocrinology real-world practices. Clin Transl Gastroenterol. 2021 Apr 6;12(4):e00340. doi: 10.14309/ctg.0000000000000340
- Eslam M, Wong GL, Hashem AM, et al. A Sequential algorithm combining ADAPT and liver stiffness can stage metabolic-associated fatty liver disease in Hospital-based and primary care patients. Am J Gastroenterol. 2021;116(5):984–993. (1572–0241 (Electronic)). doi: 10.14309/ajg.0000000000001059
- Mansour D, Grapes A, Herscovitz M, et al. Embedding assessment of liver fibrosis into routine diabetic review in primary care. JHEP Rep. 2021 Aug;3(4):100293. doi: 10.1016/j.jhepr.2021.100293
- Harman DJ, Ryder SD, James MW, et al. Direct targeting of risk factors significantly increases the detection of liver cirrhosis in primary care: a cross-sectional diagnostic study utilising transient elastography. BMJ Open. 2015 May 3;5(4):e007516. doi: 10.1136/bmjopen-2014-007516
- Srivastava A, Gailer R, Tanwar S, et al. Prospective evaluation of a primary care referral pathway for patients with non-alcoholic fatty liver disease. J Hepatol. 2019 Aug;71(2):371–378. doi: 10.1016/j.jhep.2019.03.033
- Thiele M, Madsen BS, Hansen JF, et al. Accuracy of the Enhanced liver fibrosis test vs FibroTest, elastography, and indirect markers in detection of advanced fibrosis in patients with alcoholic liver disease. Gastroenterology. 2018 Apr;154(5):1369–1379. doi: 10.1053/j.gastro.2018.01.005
- Shaheen AA, Riazi K, Medellin A, et al. Risk stratification of patients with nonalcoholic fatty liver disease using a case identification pathway in primary care: a cross-sectional study. CMAJ Open. 2020;8(2):E370–E376. (2291–0026 (Print)). doi: 10.9778/cmajo.20200009
- Bedogni G, Kahn Hs Fau - Bellentani S, Bellentani S, et al. A simple index of lipid overaccumulation is a good marker of liver steatosis. BMC Gastroenterol. 2010;10(1): (1471–230X (Electronic)). doi: 10.1186/1471-230X-10-98
- Zelber-Sagi S, Ratziu V, Fau - Zvibel I, et al. The association between adipocytokines and biomarkers for nonalcoholic fatty liver disease-induced liver injury: a study in the general population. Eur J Gastroenterol Hepatol. 2012;24(3):262–269. (1473–5687 (Electronic)). doi: 10.1097/MEG.0b013e32834f15dd
- Wong V-O, Zelber-Sagi S-O, Cusi K, et al. Management of NAFLD in primary care settings. Liver Int. 2022;42(11):2377–2389. doi: 10.1111/liv.15404
- Basu R, Noureddin M, Clark JM. Nonalcoholic fatty liver disease: review of management for primary care providers. Mayo Clin Proc. 2022;97(9):1700–1716. doi: 10.1016/j.mayocp.2022.04.005
- Kumar A, Pant S, Narang S. Significance of alanine aminotransferase testing in diagnosis of acute and chronic HBV infection. Asian Pac J Cancer Prev. 2009;10(6):1171–1172.
- Nowicki MJ, Poley JR. The hereditary hyperbilirubinaemias. Baillière’s Clinical Gastroenterology. 1998;12(2):355–367. (0950–3528 (Print)). doi: 10.1016/S0950-3528(98)90139-7
- Roulot D, Costes JL, Buyck J-F, et al. Transient elastography as a screening tool for liver fibrosis and cirrhosis in a community-based population aged over 45 years. Gut. 2011 Jul;60(7):977–984. doi: 10.1136/gut.2010.221382
- Pal P, Palui R, Ray S. Heterogeneity of non-alcoholic fatty liver disease: implications for clinical practice and research activity. World J Hepatol. 2021;13(11):1584–1610. (1948–5182 (Print)). doi: 10.4254/wjh.v13.i11.1584
- Berzigotti A, Tsochatzis E, Boursier J. EASL clinical practice guidelines on non-invasive tests for evaluation of liver disease severity and prognosis – 2021 update. J Hepatol. 2021 Sep;75(3):659–689. doi: 10.1016/j.jhep.2021.05.025
- Rinella ME, Neuschwander-Tetri BA, Siddiqui MS, et al. AASLD practice guidance on the clinical assessment and management of nonalcoholic fatty liver disease. LID. [doi] FAU - Rinella, Mary E. (1527–3350 (Electronic)) doi: 10.1097/HEP.0000000000000323
- Ciardullo S-O, Ronchetti C, Muraca E, et al. Impact of using different biomarkers of liver fibrosis on hepatologic referral of individuals with severe obesity and NAFLD. J Endocrinol Invest. 2020;43(7):1019–1026. (1720–8386 (Electronic)). doi: 10.1007/s40618-020-01188-7
- Alqahtani SA, Paik JM, Biswas R, et al. Poor awareness of liver disease among adults with NAFLD in the United States. Hepatol Commun. 2021;5(11):1833–1847. (2471–254X (Electronic)). doi: 10.1002/hep4.1765
Appendix
Date of the search: 27 May 2022
Selection criteria: