ABSTRACT
Background and aims
Acute severe ulcerative colitis (ASUC) is strongly associated with poor prognosis. We aimed to establish and validate a model predicting ASUC occurrence within 1 year after ulcerative colitis(UC) diagnosis.
Methods
A cohort of UC patients diagnosed between 2018 and 2020 at Northern Jiangsu People’s Hospital, who were followed up for one year, was used to develop a risk prediction model. An independent cohort from January to December 2021, monitored until December 2022 at the at the First Affiliated Hospital of Nanjing Medical University, was used for external validation. A multivariable logistic regression analysis was conducted to investigate the adjusted association between six risk factors and ASUC. Subsequently, a simplified model was developed by eliminating a relatively insignificant risk factor to create an easy-to-use index.
Results
The prediction model incorporates five parameters: disease extent, endoscopic appearance, histopathology, baseline response medication, and relapse frequency. It generates a nomogram in the end. The discriminant ability (c-index) was separately calculated as 0.982 and 0.925 in the development and validation cohorts.
Conclusions
The risk prediction model for developing ASUC within one year demonstrated excellent reliability and validity, which could be a straightforward and clinically valuable tool for predicting ASUC occurrence within 1 year.
Clinical trial registration
ChiCTR2300071794
1. Introduction
Ulcerative colitis (UC) is a chronic inflammatory disorder that often starts from the rectum and extends more proximally. The exact etiology of UC remains unknown [Citation1].
Approximately 25% of patients with UC experience at least one severe attack in their lifetime [Citation2]. Acute severe ulcerative colitis (ASUC) is defined by the Truelove and Witts criteria, which is > 6 bloody stools per day and systemic toxicity with at least one of: temperature > 37.8°C, pulse >90 bpm, hemoglobin (Hb)<105 g/L or erythrocyte sedimentation rate(ESR)>30 mm/h [Citation3]. Despite improvements in medical management, ASUC still remains a medical emergency, with mortality around 1%, 20% risk of colectomy at first admission and colectomy rates of 40% after two hospitalizations [Citation4]. Therefore, early identification of high-risk ASUC patients and prompt intervention or conversion therapy can significantly improve their prognosis. Numerous reports have been published on the prediction models for ASUC treatment efficacy [Citation5–7], However, there is limited research on the risk factors for predicting the occurrence of ASUC in patients with UC. The reported prediction model for ASUC within 3 years falls short of meeting the requirements for clinical close monitoring, as it does not account for UC’s potential rapid progression and the relatively long time frame involved [Citation8]. In addition to elevated C-reactive protein (CRP) levels, decreased albumin (Alb) levels, and severe endoscopic scores, recent studies have revealed that certain clinical indicators are also indicative of disease severity. These include elevated platelet(Plt) counts [Citation9], increased fecal calprotectin [Citation10], proximal progression of inflammation [Citation11], high cumulative CT severity score [Citation12], nonadherence to maintenance therapy [Citation13], poor response to corticosteroids [Citation14], and others. Therefore, the objective of this study is to develop a more comprehensive and timelier model that integrates clinical, biochemical, endoscopic, and pathological data for predicting the occurrence of ASUC within one year.
2. Methods
All patients diagnosed with UC between 2018 and 2020 at Northern Jiangsu People’s Hospital, who were followed until December 2021, were included in the development of the predictive model. Patients were identified from a registry and located through electronic medical records. The electronic medical record system has a highly advanced follow-up function that uses a patient’s identification as a unique identifier to record all outpatient and inpatient visits, even if the patient does not consult with the same doctor each time. So, we have complete access to all the medical records of a case. The diagnosis of UC was established based on standard clinical, endoscopic, and histopathological criteria [Citation15]. The primary endpoint was hospitalization due to ASUC, as defined by the Truelove and Witts criteria [Citation3]. An independent cohort from the First Affiliated Hospital of Nanjing Medical University was utilized for external validation. This cohort consisted of all patients diagnosed with UC between January and December 2021, who were followed up until December 2022. Exclusion criteria encompassed: (1) hospitalization due to ASUC within one month after diagnosis; (2) patients initially diagnosed with UC but subsequently re-diagnosed with Crohn’s disease during treatment; (3) instances where data were missing during follow-up.
Generic ethical permission was granted by the Clinical Research Ethics Committee of the First Affiliated Hospital of Nanjing Medical University, Nanjing, Jiangsu, all patients provided consent for monitoring and collection of biological samples during their initial visit, as well as informed consent for the review of their clinical data in support of clinical research.
2.1. Risk factors
Age, gender, extent of disease at initial assessment based on the Montréal classification [Citation16], hemoglobin (Hb, g/L), white blood cell counts (WBC, ×109/L), neutrophil counts (N, ×109/L), Plt counts (×109/L) at diagnosis, CRP level in mg/L, ESR in mm/hour and Alb level in g/L at diagnosis as well as endoscopic and histological severity at diagnosis prior to treatment were identified as potential risk factors through a literature review. The severity of endoscopic findings was evaluated using the Ulcerative Colitis Endoscopic Index of Severity (UCEIS) [Citation17], which categorizes activity as mild (1–3 points), moderate (4–6 points), or severe (7–8 points). The patients who developed ASUC within one month were excluded, while the remaining subjects underwent endoscopy to ascertain the extent of their disease. Histological severity was assessed utilizing the Nancy index(NI) [Citation18]. Furthermore, we integrated specific clinical disease characteristics to encompass the assessment of risk factors including relapse frequency (infrequent: ≤once/year, frequent: ≥twice/year, continuous: active UC symptoms with no relief) and the medication administered to induce a clinical response during the initial episode (referred to as baseline response medication), which was categorized into three main groups: antibiotics and/or probiotics, aminosalicylic acid, and glucocorticoids. The inclusion of antibiotics and/or probiotics in the selection of baseline response medication is a topic of controversy, as it deviates from the UC guidelines for treatment. However, in real-world clinical practice, not all patients can be definitively diagnosed with UC during their initial visit and prescribed guideline-recommended medication. In such cases, doctors rely on their experience and prescribing habits to alleviate symptoms, which mainly involve the use of antibiotics and/or probiotics. So, antibiotics and/or probiotics are still used as a parameter for baseline response medication in this study. The clinical response is defined as a ≥ 50% reduction in the patient-reported outcome (PRO) score before and after treatment, with a PRO = 0 indicating clinical remission [Citation19]. PRO score is determined by assessing the frequency of stool and the presence of bloody stool [Citation20]. For patients who developed ASUC, we recorded the frequency of relapse prior to the onset of ASUC. All of scoring and rating underwent a thorough review process conducted by at least two professionals.
2.2. Develop prediction model and validation
Prior to developing the prediction model, the data is randomly divided into two sets in a 2:1 ratio. One set is utilized for establishing the prediction model, referred to as the training set, while the other set is employed for internal validation purposes. If the prediction model established using the training set exhibits corresponding characteristics (discrimination and consistency) on the verification set, it indicates that the predictive model is effective. In this study, Bootstrapping was employed to better preserve the sample size of the training set and reduce underfitting risks associated with modeling. External validation helps to more effectively evaluate predictive performance by utilizing independent data from the First Affiliated Hospital of Nanjing Medical University in this study.
2.3. Statistical methods
Disease phenotype and clinical parameters were collected and subjected to statistical analysis. Continuous variables were presented as medians with interquartile ranges (IQRs), while discrete data was expressed as percentages. Nonparametric categorical data between groups were compared using either Chi-square or Fisher’s exact tests. Logistic regression analysis was employed to examine the univariate impact of various predictive parameters on the risk of ASUC occurrence within a one-year timeframe. Additionally, a multivariate regression analysis was conducted to account for potential interdependencies among these parameters.
After performing stepwise forward selection, only the factors that were statistically significant (p < 0.05) remained in the final optimized model. The discrimination performance of the prediction model was assessed using the area under the receiver-operating characteristic curve (AUROC) and consistency index (C-index). Calibration curves were generated to evaluate the calibration of the prediction model. All statistical analyses were conducted using SPSS 27.0 software (SPSS, Chicago, IL, U.S.A.). The nomogram was constructed in R Studio (version 2023.03.0–386, rms package) to visually represent the prediction model.
3. Results
3.1. Patient clinical characteristics
Features of patients at diagnosis are presented in . In the development cohort, a total of 658 patients meeting the inclusion criteria were identified between 2018 and 2020.Out of the 658 patients included in the study, 71 (10.8%) were admitted with ASUC at least once within one year from diagnosis, with a median time of 4.3 months after diagnosis and a range of 1–12 months. All ASUC patients received standard intensive therapy according to AGA Clinical Practice Guidelines on the Management of Moderate to Severe Ulcerative Colitis (2020) [Citation21]. In hospitalized adult patients with ASUC refractory to intravenous corticosteroids, it is suggested by the AGA that infliximab or cyclosporine be used. Typically, glucocorticoid therapy for 3 to 5 days in patients with ASUC. If there is no response, alternative treatment options such as cyclosporine, infliximab or tofacitinib can be considered. Colectomy if there is no improvement after 4–7 days of rescue therapy. Of these patients, 40 out of 71 (56.3%) achieved endoscopy remission(UCEIS ≤1 point) [Citation19], while 14 out of those who achieved remission received infliximab, vedolizumab(1 case combined with prednisone on account of an allergic reaction in the second dose of infliximab) or tofacitinib as rescue therapy and four underwent surgery due to failure of rescue therapy. Two other patients underwent surgery without receiving any rescue therapy, giving a colectomy rate of 6/71 (8.5%).
Table 1. Clinical characteristics of patients in the development and validation cohorts.
3.2. Univariate and multivariable logistic regression
Results of univariate and multivariable logistic regression analyses on risk factors in the Development cohort are summarized in . Univariate analyses revealed no significant differences for age and gender, while all other factors exhibited significant differences at diagnosis with ASUC within one year(p < 0.001). Multivariable logistic regression confirmed that disease extent(p = 0.002), endoscopy appearance(p = 0.006), histopathology(p = 0.029), baseline response medication(p < 0.001), frequency of relapse(p < 0.001), and ESR(p = 0.049) were significant predictors of ASUC ()
Table 2. Univariate logistic regression for prediction of the occurrence of ASUC within 1 year in development and validation cohorts.
Table 3. Univariate and multivariate logistic regression model for prediction of the occurrence of ASUC within 1 year.
Considering that the OR value of ESR was 1.028,p value was 0.049, which meant that although ESR was significant in the multivariate regression analysis, its influence on the model was relatively insignificant, so we eliminated ESR and included the remaining five factors to establish the multivariate regression model. Finally, the multivariate model demonstrates the robustness of predicting the occurrence of ASUC within one year after UC diagnosis, as evidenced by an impressive area under the receiver operating characteristic curve of 0.982 (95% CI, 0.964–1.000) (). Moreover, it exhibits a sensitivity of 90.0% and specificity of 98.6%. The calibration curve further confirms the high accuracy and reliability of this model ().
Figure 1. Receiver operating characteristic (ROC) curves of five predictors were plotted to predict the occurrence of ASUC within 1 year. The red line represents the development cohort, while the green line represents the validation cohort. The area under the ROC curve in the training cohort was determined to be 0.982 (95% CI, 0.964–1.000). Similarly, in the validation group, it was found to be 0.925 (95% CI, 0.865–0.984).

Figure 2. Calibration curves were generated for the training model in the development cohort (a) and validation cohort (b). The x-axis denotes predicted ASUC risk, while the y-axis represents actual ASUC rate. The green line indicates model performance, with 45-degree dotted lines representing perfect prediction. A closer fit between the green line and dotted line signifies greater accuracy of the model.

3.3. Validation cohorts
A total of 140 patients with UC were included in the external validation cohort. There were some differences in baseline demographic characteristics between the validation and development cohorts, as indicated in . Due to the hierarchical diagnosis and treatment system in China, the, the First Affiliated Hospital of Nanjing Medical University holds a higher rating, attracting relatively more severe cases among patients seeking treatment there. Based on the Montreal classification, a significantly higher proportion of patients in the validation group exhibited type E2 and E3 disease extent compared to those in the development cohort (42.9%vs 29.0%; 37.1% vs 27.4%, respectively). Notably, few of the patients(only 2 patients) received baseline response medication antibiotics and/or probiotics, which may reflect differences in patient severity and physician treatment preferences. The rates of ASUC and corresponding p-values in both the development and validation cohorts are summarized in . Specifically, within the validation cohort, 33 patients (23.6%) developed ASUC after a one-year follow-up period. The variables found to be significantly associated with ASUC included the extent(p < 0.001), endoscopic appearance(p=0.004), histopathology(p < 0.001), Hb (p = 0.008), CRP(p < 0.001), ESR(p < 0.001), Plt(p=0.001), WBC(p < 0.001), N(p < 0.001), and Alb(p < 0.001). By utilizing the algorithm for predicting ASUC, the area under the receiver operating characteristic curve was 0.925 (95% CI 0.865–0.984) in the validation cohort().This result was in close proximity to the value observed in the development cohort, with a sensitivity and specificity of 87.9% and 85.0%, respectively. While the calibration curve for the validation cohort demonstrated reasonable fit, it did not match that of the model ().
3.4. Nomogram for individual risk
In order to visually calculate individual risk, a nomographic chart was developed to align the patient’s results with predicted points on the top axis [. The cumulative points for each element are tallied to determine the ‘total points’, which assesses the probability of ASUC in an individual patient over the next year from diagnosis by drawing a line down from the ‘total points’ axis.
Figure 3. Nomogram constructed using coefficients derived from multivariate analysis as weights to predict the risk of developing ASUC within one year of diagnosis in a given patient. To calculate the probability of ASUC, each predictor’s value was obtained by drawing a vertical line straight upward from that factor to the point axis. The points achieved for each predictor were then summed and located on the total point axis of the nomogram. By drawing a vertical line downward, one could locate the probability of ASUC.
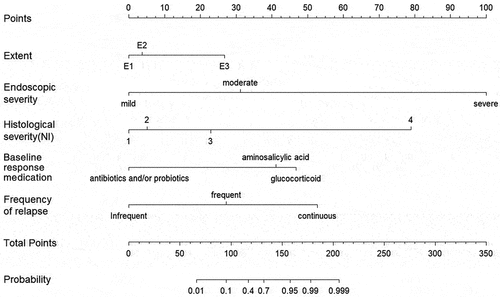
4. Discussion
Monica Cesarini developed a model to predict the risk of ASUC within 3 years in populations from England and Sweden, taking into account factors such as disease extent, CRP and Hb [Citation8]. However, due to unpredictable variables like changes in treatment protocols, poor adherence to medication regimens, and comorbidities et al, the accuracy of this prediction over a period as long as three years may be compromised. Additionally, rapid progression of the disease can render the 3-year prediction model irrelevant.
In our study, the incidence of ASUC at 1 year was 10.8% in the development cohort and 23.6% in the validation cohort, consistent with previous reports [Citation2,Citation22]. However, significant differences were observed between these two cohorts, which may be attributed to regional disparities and China’s hierarchical diagnosis and treatment system.
The ACG Clinical Guideline (2019) has identified age younger than 40 years at diagnosis as a prognostic factor for the severity of UC [Citation23]. Additionally, studies have suggested that age ≤27 years old and female are risk factors for relapse [Citation24,Citation25]. However, our study found no significant association between gender or age of onset and the incidence of ASUC within one year based on univariate logistic regression analyses. The occurrence of ASUC within the following 1 year was found to be associated with various factors, including disease extent, endoscopic appearance, histopathology, baseline response medication, frequency of relapse, and laboratory parameters such as CRP, ESR, WBC, N, Plt, Alb, and Hb. Parameters that demonstrated statistical significance in the ASUC model were selected for inclusion in an optimized risk model using a stepwise approach. Unfortunately, none of the biological markers were incorporated into the final multivariate logistic regression model. Multivariable logistic regression analysis revealed no significant differences for CRP, WBC, N, PLT, Alb and Hb. Although ESR showed a significant difference, its OR value was 1.028 indicating that ESR had minimal impact on the model and was therefore excluded from the final prediction model. The differences between groups for the following factors remained statistically significant in the optimized model: extent of disease, endoscopic appearance, histopathology, baseline response medication, and frequency of relapse within one year. These combined values were utilized to establish a prognostic index with high predictive capacity for assessing the likelihood of ASUC occurrence within the subsequent year. Despite the subjective nature of baseline clinical response therapy, the inclusion of relapse frequency in efficacy analysis of previous medication therapy better reflects real-world medical conditions and potentially enables objective identification of patients requiring intensive monitoring or early intervention with optimal therapy.
We have found that clinical and endoscopic indicators are effective predictors, while biological markers appear to be less significant. This may be attributed to their susceptibility to opportunistic infections and rapid improvement with medication treatment.
There are limitations. Most notably, the study was retrospective, so many patients with missing follow-up data were excluded. Such patients are more likely to have less severe disease, and fecal calprotectin levels has been considered to be correlated significantly with clinical or endoscopic disease activity in inflammatory bowel diseases [Citation26]. However, due to the unavailability of fecal calprotectin in our region at 2018, there was a higher incidence of missing data, led us to exclude it as an observation index in this study. Although retrospective, the criteria are entirely objective. We conducted external validation using data from a hospital in another city. The data collection includes treatment details, but selection may involve uncontrollable variables such as individual physician treatment preferences and patient treatment adherence. Disease extent assessment timing also varied; while extent was defined at first colonoscopy, precise timing (dependent on severity and access to colonoscopy) was not standardized.
5. Conclusion
In conclusion, we have successfully developed a simple and reliable tool for predicting the occurrence of ASUC within one year using data from 658 consecutive patients with UC. The prediction nomogram was also validated in an external cohort of 140 patients. Our findings suggest that disease extent, endoscopic appearance, histopathology, baseline response medication, and frequency of relapse are important factors influencing the occurrence of ASUC within one year. The occurrence of ASUC in the 1-year prediction nomogram can serve as a clinical decision support tool for managing patients with UC.
Declaration of interest
The authors have no relevant affiliations or financial involvement with any organization or entity with a financial interest in or financial conflict with the subject matter or materials discussed in the manuscript. This includes employment, consultancies, honoraria, stock ownership or options, expert testimony, grants or patents received or pending, or royalties.
Reviewer disclosure
Peer reviewers on this manuscript have received an honorarium from Expert Review of Gastroenterology & Hepatology for their review work but have no other relevant financial relationships to disclose.
Additional information
Funding
References
- Ordás I, Eckmann L, Talamini M, et al. Ulcerative colitis. Lancet. 2012;380(9853):1606–1619. doi: 10.1016/S0140-6736(12)60150-0
- Sebastian S, Myers S, Nadir S, et al. Systematic review: efficacy and safety of accelerated induction regimes in infliximab rescue therapy for hospitalized patients with acute severe colitis. Dig Dis Sci. 2019 May;64(5):1119–1128. doi: 10.1007/s10620-018-5407-7
- Truelove SC, Witts LJ. Cortisone in ulcerative colitis; final report on a therapeutic trial. Br Med J. 1955 Oct 29;2(4947):1041–1048. doi: 10.1136/bmj.2.4947.1041
- Rosiou K, Selinger CP. Acute severe ulcerative colitis: management advice for internal medicine and emergency physicians. Intern Emerg Med. 2021 Sep;16(6):1433–1442. doi: 10.1007/s11739-021-02704-0
- Gisbert JP, Chaparro M. Predictors of primary response to biologic treatment [anti-TNF, Vedolizumab, and ustekinumab] in patients with inflammatory bowel disease: from basic Science to clinical practice. J Crohns Colitis. 2020 Jun 19;14(5):694–709. doi: 10.1093/ecco-jcc/jjz195
- Yoshida A, Matsuoka K, Ueno F, et al. Serum PR3-ANCA is a predictor of primary nonresponse to anti-TNF-α agents in patients with ulcerative colitis. Inflamm Intest Dis. 2021 May;6(2):117–122. doi: 10.1159/000515361
- Colman RJ, Dhaliwal J, Rosen MJ Predicting therapeutic response in pediatric ulcerative colitis-A journey towards precision medicine. Front Pediatr. 2021 Feb 17;9:634739. doi: 10.3389/fped.2021.634739
- Monica C, Collins GS, Rönnblom A, et al. Predicting the individual risk of acute severe colitis at diagnosis.Journal of Crohn’s and colitis. Journal Of Crohn’s & Colitis. 2017;11(3):335–341. doi: 10.1093/ecco-jcc/jjw159
- Akpinar MY, Ozin YO, Kaplan M, et al. Platelet-to-lymphocyte ratio and neutrophil-to-lymphocyte ratio predict mucosal disease severity in ulcerative colitis. J Med Biochem. 2018;37(2):155–162. doi: 10.1515/jomb-2017-0050
- Hart L, Chavannes M, Kherad O, et al. Faecal calprotectin predicts endoscopic and Histological activity in clinically quiescent ulcerative colitis. J Crohns Colitis. 2020 Jan 1;14(1):46–52. doi: 10.1093/ecco-jcc/jjz107
- Qiu Y, Chen B, Li Y, et al. Risk factors and long-term outcome of disease extent progression in Asian patients with ulcerative colitis: a retrospective cohort study. BMC Gastroenterol. 2019 Jan 10;19(1):7. doi: 10.1186/s12876-018-0928-2
- Patel B, Mottola J, Sahni VA, et al. MDCT assessment of ulcerative colitis: radiologic analysis with clinical, endoscopic, and pathologic correlation. Abdom Imaging. 2012 Feb;37(1):61–9. doi: 10.1007/s00261-011-9741-x
- Feagins LA, Iqbal R, Spechler SJ Case-control study of factors that trigger inflammatory bowel disease flares. World J Gastroenterol. 2014;20(15):4329–34. doi: 10.3748/wjg.v20.i15.4329
- Deva Rajoo G, Tan L, Lopez A, et al. Early response to corticosteroid and baseline C-Reactive protein predicts outcomes in children with Moderate to severe ulcerative colitis. Dig Dis Sci. 2019 Jul;64(7):1929–1937. doi: 10.1007/s10620-019-05486-w
- Magro F, Gionchetti P, Eliakim R, et al. European Crohn’s and colitis Organisation [ECCO]. Third European Evidence-based Consensus on diagnosis and management of ulcerative colitis. Part 1: definitions, diagnosis, extra-intestinal manifestations, pregnancy, cancer surveillance, surgery, and ileo-anal pouch disorders. J Crohns Colitis. 2017 Jun 1;11(6):649–670. doi: 10.1093/ecco-jcc/jjx008
- Silverberg MS, Satsangi J, Ahmad T, et al. Toward an integrated clinical, molecular and serological classification of inflammatory bowel disease: report of a working party of the 2005 Montreal world congress of Gastroenterology.Can J Gastroenterol.2005 Sep;19 Suppl A:5A–36A. doi: 10.1155/2005/269076
- Travis SP, Schnell D, Krzeski P, et al. Reliability and initial validation of the ulcerative colitis endoscopic index of severity. Gastroenterology. 2013 Nov145(5):987–95. doi: 10.1053/j.gastro.2013.07.024
- D’Amico F, Guillo L, Baumann C, et al. Histological disease activity measured by the Nancy index is associated with long-term outcomes in patients with ulcerative colitis. J Crohns Colitis. 2021;15(10):1631–1640. doi: 10.1093/ecco-jcc/jjab063
- Turner D, Ricciuto A, Lewis A, et al. International organization for the study of IBD. STRIDE-II: an update on the selecting therapeutic targets in inflammatory bowel disease (STRIDE) initiative of the international organization for the study of IBD (IOIBD: determining therapeutic goals for treat-to-target strategies in IBD. Gastroenterology. 2021 Apr;160(5):1570–83. doi: 10.1053/j.gastro.2020.12.031
- Restellini S, Chao CY, Martel M, et al. Clinical parameters correlate with endoscopic activity of ulcerative colitis: a systematic review. Clin Gastroenterol Hepatol. 2019 Jun;17(7):1265–1275.e8. doi: 10.1016/j.cgh.2018.12.021
- Feuerstein JD, Isaacs KL, Schneider Y, et al. AGA Institute clinical guidelines committee. AGA clinical practice guidelines on the management of Moderate to severe ulcerative colitis. Gastroenterology. 2020 Apr;158(5):1450–1461. doi: 10.1053/j.gastro.2020.01.006
- Seah D, De Cruz P. Review article: the practical management of acute severe ulcerative colitis. Aliment Pharmacol Ther. 2016 Feb;43(4):482–513. doi: 10.1111/apt.13491
- Rubin DT, Ananthakrishnan AN, Siegel CA, et al. ACG clinical guideline: ulcerative colitis in adults. Am J Gastroenterol. 2019 Mar;114(3):384–413. doi: 10.14309/ajg.0000000000000152
- Jonefjäll B, Simrén M, Lasson A, et al. Psychological distress, iron deficiency, active disease and female gender are independent risk factors for fatigue in patients with ulcerative colitis. United Eur Gastroenterol J. 2018 Feb;6(1):148–158. doi: 10.1177/2050640617703868
- Marti-Aguado D, Ballester MP, Tosca J, et al. Long-term follow-up of patients treated with aminosalicylates for ulcerative colitis: predictive factors of response: an observational case-control study. United Eur Gastroenterol J. 2019;7(8):1042–1050. doi: 10.1177/2050640619854277
- Jukic A, Bakiri L, Wagner EF, et al. Calprotectin: from biomarker to biological function. Gut. 2021 Oct;70(10):1978–1988. doi: 10.1136/gutjnl-2021-324855