Abstract
The use of agent-based models (ABMs) for investigating land-use science questions has been increasing dramatically over the last decade. Modelers have moved from ‘proofs of existence’ toy models to case-specific, multi-scaled, multi-actor, and data-intensive models of land-use and land-cover change. An international workshop, titled ‘Multi-Agent Modeling and Collaborative Planning—Method2Method Workshop’, was held in Bonn in 2005 in order to bring together researchers using different data collection approaches to informing agent-based models. Participants identified a typology of five approaches to empirically inform ABMs for land use science: sample surveys, participant observation, field and laboratory experiments, companion modeling, and GIS and remotely sensed data. This paper reviews these five approaches to informing ABMs, provides a corresponding case study describing the model usage of these approaches, the types of data each approach produces, the types of questions those data can answer, and an evaluation of the strengths and weaknesses of those data for use in an ABM.
1. Introduction
To understand the complex interactions between human societies and the land resources on which they depend, a growing number of scientists have turned to computer-based simulations. Simulations serve a number of purposes in the science of coupled human–natural systems, including (a) integrating multiple disciplinary perspectives, (b) training intuition about the causes of observed patterns and dynamics, (c) testing for plausibility of candidate explanations, (d) developing conceptual frameworks for empirical data collection, (e) creating scenarios about future system states, and (f) testing the possible effects of alternative policy or management interventions in the system. In contrast to other modeling approaches (e.g. cellular automata, Markov simulation), agent-based models (ABMs) or multi-agent systems (MAS)Footnote 1 can explicitly formalize simple to complex representations of the behavior and cognitive processes of actors who make land and resource use decisions within the system. Because the ABM approach explicitly represents actor behavior, these models may be viewed as more process-based and deductive than the statistical or mathematical models common in land change science, in which emphasis is placed on fitting parameters to observations (Brown et al. Citation2004, Verburg et al. Citation2006).
In designing an ABM, the modeler takes a ‘bottom-up’ approach by considering the relevant actors and decisions at the micro-level that may produce an observable macro-phenomenon (e.g. system-level outcome). The model development is guided by and compared to existing patterns in the target system that act as indicators of the underlying micro-processes and structure (Grimm et al. Citation2005). Therefore the use of an ABM approach to improve our understanding or support the rigorous analysis of potential outcomes of that system (e.g. scenario and policy analysis) requires that ABMs have credible and defensible representations of micro-processes. This requirement raises important questions about available empirical approaches for capturing micro processes and their relative merits.
1.1 Empirical approaches for understanding micro-processes
This paper is a product of an international workshopFootnote 2 in which participants discussed their experiences with alternative approaches to empirical grounding for ABMs. Together we developed a typology of empirical approaches that support the representation of micro-level processes in ABMs. We used that typology to structure our discussion, and employ it in this paper. The intent of this paper is to provide a review of several data collection methods available, the types of data they produce, the types of questions those data can answer, and the strengths and weaknesses of those data for use in an ABM. We recognized five different approaches:
-
Sample surveys
-
Participant observation
-
Field and laboratory experiments
-
Companion modeling
-
GIS and remotely sensed spatial data
These approaches differ on a range of dimensions, including the degree of a priori theory they rely on; their ability to obtain social and biophysical data; the types of measurements recorded by each method (i.e. qualitative versus quantitative); their ability to elicit agent types, characteristics, beliefs, knowledge, and/or behaviors; the richness of the behavioral information they yield, and the degree to which they can capture temporal and spatial information. Each approach has its own (inter-)disciplinary heritage.
Participants distinguished two reasons ABMs need empirical data: to document the macro-phenomena and to inform micro-process modeling. The projects discussed in this paper reserve the use of macro-level data for model validation, providing an independent test of the micro-level processes encoded in the model.Footnote 3 This paper focuses on the requirement for data on micro-processes because it is the representation of these processes that distinguishes ABMs from other modeling approaches.
The five approaches discussed all generate data and understanding at the micro-level (i.e. at the level of the decision-making agent). Such information for modeling has both qualitative and quantitative value for building models of agent-level decision-making. In qualitative terms, we might wish to test a hypothesized decision-making strategy. Such tests provide generalized knowledge about strategies for decision-making, but rarely provide specific quantitative values that can be used in models. Other methods might be used to provide quantitative information about the relative value of various factors used in decision making, or about the relative abundance of various agent characteristics.
In the next section, we define and describe each of the above empirical approaches, and identify questions they address and the kinds of data they produce. We summarize each method with a listing of strengths and weaknesses and describe an example case study in which the method was used. Section 3 concludes with a discussion on the comparative advantages of the different approaches, recognizing that each method has its strengths and weaknesses.
2 Description of alternative approaches
2.1 Sample surveys
2.1.1 Definition and description
Sample surveys are quantitative methods for collecting data on individuals, households, and communities using mostly closed-ended questions. Typically a fraction of the population is sampled using techniques (e.g. random, stratified) that capture the distribution of characteristics found in the entire population. To support a one-to-one representation of real-world to software agents, most sample surveys are employed at the household level, since households are commonly the atomic unit of land use ABMs.
Increasingly, existing household surveys and well documented data sets are becoming publicly available (Grosh and Glewwe Citation2000, World Bank Citation2005a, Citation2005b, IFPRI Citation2005) and may serve as input to ABMs in some contexts. However, modelers often need to design new surveys in order to tailor data collection to the needs of a particular model application or case study.
2.1.2 Types of data produced
Responses from sampled households are commonly used to parameterize behavioral models based on microeconomic theory or to generate statistical descriptions of the attributes of the agents in a population. Two approaches have been used in applying these statistical descriptions within ABMs. One uses Monte Carlo techniques to generate heterogeneous populations of unique agents (e.g. Berger and Schreinemachers Citation2006, Brown and Robinson Citation2006). The other uses typical agents based on representative cases from the survey, in numbers proportional to their representation in the population (e.g. Deffuant et al. Citation2005, Happe Citation2004).
2.1.3 Questions that can be addressed with the technique
Household surveys can be used to:
-
provide information on the distributions of characteristics, beliefs and preferences within a population of agents;
-
estimate behavioral models based on economic theory;
-
provide rough estimates of local-level change variables; and
-
identify constraints on decision-making.
2.1.4 Strengths and weaknesses
The strengths and weaknesses of the sample survey method stem from its reliance on a restricted and structured set of questions about the characteristics and beliefs of agents and those of their neighbors (). By restricting the number of respondents and the questions, it is possible to produce quantitative data that are statistically representative of the broader population, without the expense of surveying all agents. The information that has been obtained from surveys can be used to cross-check observations and to assess the heterogeneity of households, and specialized household surveys can be used to map social networks of interaction. However, some information may be difficult to gather from respondents as they may not know how to express themselves in a quantifiable way or may not wish to reveal certain information. Sample surveys can also be conducted on classes of agents other than households, such as company managers, providers of public infrastructure, and policy-makers. However, such surveys are less common, and are often conducted with more open-ended questions and interviews.
Table 1. Strengths and weaknesses of household survey data
2.1.5 Case study: simulating soil fertility decline, population growth, and poverty dynamics in Uganda
Problem: Stagnating productivity and persistent poverty still characterize farming systems in much of sub-Saharan Africa. Biophysical and social scientists tend to interpret the situation in widely different ways. Biophysical scientists point to soil fertility decline and population growth as the main causes and suggest technology improvement, while social scientists point at malfunctioning markets and institutions, suggesting corrective policy action. The objective of this study (Schreinemachers Citation2006) was to disentangle the combined effects of soil fertility decline, population growth, and market institutions on the dynamics of poverty and productivity.
Study area: The 12 km2 research area included two densely populated villages in southeastern Uganda comprising ∼520 households. Climatic conditions in the area allow for the cultivation of two sequential crops per year. Cassava, sweet potato, plantain, and beans are the main subsistence crops, coffee is the main cash crop, and maize is both home-consumed and sold. The nearest town is ∼15 km away, which provides good market access but only limited off-farm employment. Soil fertility is generally low but varies across locations. The landscape is gently sloping with large flat areas and moderate levels of soil erosion. Households typically are large, with more than nine members on average; they commonly rely on hand tools, rarely using fertilizers and improved seeds. Intercropping is common, and farm households usually allocate only small parts of a plot to a single crop combination.
The model: An ABM model building on Berger (Citation2001) was used to integrate biophysical models simulating crop yields and soil dynamics with mathematical programming-based economic models that simulate decision-making and poverty levels.Footnote 4 Each real-world farm household was represented as an agent in the model. In line with microeconomic theory, it was assumed that three objectives guide agent land use decisions: cash income from selling farm produce and off-farm employment; in-kind income from consuming own farm produce; and future income from investments in livestock and coffee (Schreinemachers and Berger Citation2006).
Data collection: Various models of production and consumption behaviour and constraints on decision-making were estimated from household survey data (Berger and Schreinemachers Citation2006) and used to calibrate the mathematical programming model. The main components included econometrically estimated production and consumption functions and behavioral constraints relating to crop rotations, gender-specific farm tasks, and input and output prices. These constraints were further specified with qualitative information from field observation and literature. Survey data proved suitable for this purpose, as all model agents were farm households and their land use decisions could be modeled by using well-established quantitative models of production and consumption behavior.
2.2 Participant observation
2.2.1 Definition and description
Participant observation is an anthropological research method, in which the researcher both observes and participates in the target system. The aim is generally not to test a predefined theoretical model of how the system under study functions, but to build a conceptual model in line with field observations. Hypotheses are drawn from collected data and subsequently tested in the field; participant observation therefore involves building theory through iteration with observation (Strauss and Corbin Citation1990).
The technique of participant observation can support the building of ABMs by helping to identify the key agents in a system and by generating plausible explanations for the actions and interactions of agents. However, few papers have been published on its use (Bharwani et al. Citation2005 is a useful example), and even fewer in the domains of land and natural resource use (Huigen Citation2004, Huigen et al. Citation2006 are the only examples found).
2.2.2 Types of data produced
Primarily, participant observation produces qualitative rather than quantitative data. It can provide highly detailed information about interactions between individuals, about their motivations and perception of the world, and about the range of heterogeneity among these individuals, but is not likely to provide precision about the relative numbers having different characteristics. In some cases, participant observation can also generate simple quantitative data, based on counting ‘everything you see’ such as the number of households with gardens, or the amount of time people spent at a location. Much of the collected information will have been omitted in the final ABM because either the detail is too extensive to be incorporated, the hypotheses or experiments being tested are more focused, fewer mechanisms have been chosen to be modelled, or the model has been simplified to improve tractability and understanding. However, the very detailed information gleaned from participant observation is likely to improve the model-builder's understanding of the forces driving the system, and of what they are leaving out, than a modeller relying on statistical data such as collected by standardized living standard surveys.
2.2.3 Questions that can be addressed with the technique
Participant observation can help a modeller to determine:
-
the driving forces in the system;
-
how actors in the target system conceptualize their situation;
-
the importance of contextual (e.g. cultural) and/or temporal dynamics;
-
how individuals influence the social system, and vice versa; and
-
the structure and functioning of local social networks, including the way that collective decision-making is carried out (an issue of great relevance to the construction of ABMs).
2.2.4 Strengths and weaknesses
The strengths and weaknesses of the participant observation approach grow out of its intensive, on-the-ground requirements (). Because the researcher is involved in the lives of those being studied, research is ‘pushed toward the scale of action’. This naturally leads to a concentration on research questions relevant at that scale. The length of time the researcher spends with the group studied allows for the building of trust. This can help move beyond the tendency of informants to tell a researcher either what they think will be advantageous to tell them, or what they think the researcher wants to hear.Footnote 5 Also the researcher's involvement in everyday activities makes it harder for stakeholders to withhold relevant information, and should increasingly allow the researcher to frame the right questions.
Table 2. Strengths and weaknesses of participant observation
Participant observation is highly dependent on the abilities, and to some extent the preconceptions, of the individual researcher.Footnote 6 It is best suited to a relatively small spatial scale: a village, or a relatively self-contained community in a city. Given time to question and rethink hypotheses, participant observation allows the researcher a deeper understanding of the causal links between different aspects of the local situation.
2.2.5 Case study: the effects of land-use policies and programs on biodiversity conservation in San Mariano, Isabela, the Philippines
Problem: Increasing population, decreasing land availability, and decreasing values for agricultural produce at the borders of the Northern Sierra Madre Nature Park have resulted in an increase of small-scale illegal logging and cultivation in the buffer zone and the national park. These illegal activities threaten biodiversity preservation and require mitigation through policy. The goal of this study (Huigen Citation2004) was to evaluate the effects of potential land use policies on illegal logging along the border of the largest national park in the Philippines.
Study area: The study took place in the Disabungan River watershed, in the municipality of San Mariano, Isabela, Philippines. Thirteen barangays (i.e. villages) exist within the watershed, which are populated by various ethnicities that each have their own agricultural practices. Initially, settlers in the area performed slash-and-burn agriculture, which was then followed by permanent agricultural systems involving irrigated and rain-fed rice, yellow hybrid corn, bananas, and government programs inducing tree growth. The average farm is 1.5 ha in size and most farming households are linked to a local crop-trader who provides them with credit for crop production.
The model: An ABM was constructed using the MameLuke framework (Huigen Citation2004). The model has three categories of agents (logging companies, crop market actors, and farm households) and variation among the households is defined by ethnic identity and religion. Each ethnic group has different preferences, rules of decision-making and interaction and strategies for farming. The modeled farm households and crop market actors make land use decisions and interact on a monthly basis, via a two-dimensional GIS landscape. The initial land use conditions of the landscape are established using remote-sensing observations and function as a starting point for scenario analysis. Several government land use program scenarios are then introduced, such as development of irrigated areas, introduction of agro-forestry and market reforms. Other important scenario components that vary in the different models are demographics (e.g. immigration control and family planning) and climate.
Data collection: Data collection for the representation of (land use) decision strategies was conducted by participant observation. Researchers lived for extended periods with a number of farm households to understand their options and motivations for land use decisions. Using semi-structured interviews and progressive contextualization techniques, the researchers created a snapshot of the farmers’ life, which included ranking different actions. The Action-in-Context methodology (De Groot Citation1992) was used to understand farmers’ knowledge of potential options, their attitudes toward change and their potential behavior in case of a change in context. The overarching goal was to produce a numeric, behavioral representation of how social networks affect decision-making along with cultural and economic motivations. These data cover, inter alia:
-
ethnic differences in rules on where and when to plant which crop and other farm system aspects such as labor investments and use of new technologies;
-
ranking the preferences for crops (existing and potential) and future investments;
-
learning mechanisms (i.e. estimating the farmers’ thinking on the effects of governmental and NGO programs, workshops and other interventions);
-
understanding household attitudes towards family planning issues;
-
identifying farmers’ visions of changes and their behavior in potential future scenarios.
The numerous models contain one generic template for the behavioral and strategic aspects of the households with minor variations depending on the scenarios. This template consists of more than 200 potential actions that are available to farm households who make land-use decisions in a changing social and physical environment.
2.3 Field and laboratory experiments
2.3.1 Definition and description
Laboratory experiments have been used for decades in psychology, more recently in economics (Camerer Citation2003), and are beginning to surface in geography (Evans et al., Citation2006). In this paper we focus on economic experiments that capture land-use decision-making behavior. The typical subjects in these studies are undergraduate students, who get monetary or grade awards. Field experiments are less abstract than laboratory experiments, and are adjusted so that they can be run in the field with resource users and villagers (Cardenas and Ostrom Citation2004, Henrich et al. Citation2004). Because the contextual variables (such as culture and the presence of indigenous knowledge) are less tightly controlled, they result in noisier data. Both types of experiments are focused on understanding and testing alternative theories of decision-making. Participants are faced with a specific problem and certain rules, and the researchers observe the outcomes of the decision process and either observe or infer the way the participants go about solving the problem.
2.3.2 Types of data produced
The focus of controlled experiments is on falsification of hypotheses and testing alternative models of decision-making, not on generating data for ABM development of a specific applied case study of land use. The experiments generate information on the decisions individuals take in a setting where the monetary incentives are known. This detailed information on decisions can be used to fit behavioral models to the data, which provide evidence for the use of a specific decision-making strategy in an ABM to represent an actor in the target system. The goal is usually to keep the behavioral models as simple as possible, so that the results are general enough to be used in other contexts. Models that are simple yet comprehensive enough to fit data can be applied qualitatively in situations where the decision-makers are similar to the subjects used in the lab and field experiments. The experiments provide information about the structure of the decision-making model, but the precise parameter values found may not hold in a different situation. Specific parameter values may depend on the empirical case itself, since the conditions in a case study are never exactly the same as those in experiments.
2.3.3 Questions that can be addressed with the technique
Typical questions that can be addressed with experiments include the following.
-
How are decisions about resource use made? Do subjects strategically forecast the behavior of others, or do they rely only on past observations?
-
How do specific rules of the game affect resource use? For example, using different rules (i.e. treatments) the impact of communication can be quantified (Cardenas et al. Citation2000).
-
Which of a number of competing theories can best explain behavior? For example, which type of learning theory best explains how subjects learn to perform a certain task?
2.3.4 Strengths and weaknesses
Because experiments can be designed to test specific hypotheses, they can help to develop general models of decision-making processes such as learning processes, decision-making in social dilemmas, preference utility functions, and bounded rationality (e.g. Castillo and Saysel Citation2005, Deadman Citation1999, Duffy Citation2006, Jager and Janssen Citation2002). Using different treatments, experiments can also test the effects of institutional influences such as monitoring, incentives, and sanctioning on individual and group behavior. Alternatively, the ABM could be used to predict behavior under new treatments (Goldstone et al. Citation2006).
There are a number of drawbacks of controlled experiments for use with ABM (). Controlled experiments are focused on testing general models and theories of decision making. The output is a challenged and improved theory, not empirical data to develop an ABM for a specific applied situation outside the lab or field setting. Moreover, controlled experiments try to eliminate contextual and cultural factors, which might be the crucial factors in explaining observations in specific applications. Although there are many statistical tools used to test theories on experimental data, from an ABM perspective there are serious challenges to be met in order to test the suitability of models on different scales (Janssen and Ahn Citation2006).
Table 3. Strengths and weaknesses of field and laboratory experiments
2.3.5 Case study: collective action of fishermen and crab hunters on Providence Island, Colombian Caribbean Sea
Problem: Castillo and Saysel (Citation2005) sought to formalize the behavioral theory of collective action in common property resource situations, where collective action is required to reduce resource depletion by self-interested actors (Ostrom Citation1998). The theory includes assumptions on relationships between face-to-face communication and the development of norms, and relations between trust, reciprocity, reputation and cooperation.
Study area: Fish and crab resources are essential to the livelihoods of the inhabitants of Providence Island, Colombian Caribbean Sea. Black crab production has increased in the past 15 years to satisfy both trade and tourist demands. Depletion of the fishery has caused observable increases in labor inputs, and more extensive harvesting by the fishers.
The model: Castillo and Saysel (Citation2005) developed a systems dynamics model based on the behavioral theory of collective action. They formulated causal relationships among the components of the theory through feedback loops, representing the interactions between trust, reputation, and reciprocity among the residents. The model operates at the individual level, whereby a five-player real-life setting is represented as five artificial decision-making agents. These agents can be either homogeneous or heterogeneous; however, the model is run separately for different categories of agents (i.e. fishers and crab hunters).
Data collection: The model was calibrated using experimental data. The experiments used traditional common pool resource settings where a group of five villagers play 10 rounds without communication and external regulation and then 10 rounds with one of the two different treatments. The subjects were recruited from fisher and crab hunter communities.
The experimental subjects had the opportunity to harvest from a virtual common resource. There is a discrepancy between the individual and collective interest measured in monetary incentives. The two different treatments in this experiment were communication and punishment. Thus, in half of the experiments the subjects were allowed to communicate between each round during the last 10 rounds of the experiment. In the punishment treatments, each round one player was chosen randomly and received a penalty when (s)he harvested more than was allowed. The experiments with communication converged to the cooperative solution, while punishment performed well initially, but over time produced more defection from cooperative behavior.
Simulations using the model were able to replicate the experimental data for the communication and punishment treatments. Additionally, a rigorous analysis was performed to test whether the developed model was robust for more extreme conditions and assumptions. The model was found to be structurally robust and the theory of Ostrom (Citation1998), as implemented by Castillo and Saysel, was found to be a plausible model of the behavior of the fishermen and crab hunters at Providence Island in Colombia.
2.4 Companion modeling
2.4.1 Definition and description
Companion modeling was developed in the context of adaptive and collective ecosystem management, which is aimed at both increasing ecosystem adaptability and accounting for social processes that cause a particular ecological state. First, an initial model is constructed based on a virtual world that is mapped and parameterized from field studies, surveys, and literature. The initial model is constructed as a physical representation where stakeholders can alter the environment and observe the alterations made by others. This interaction often creates its own rules and dynamics. Next, role-playing games (RPGs) are designed to make use of the virtual world and to collect information about the perceptions of stakeholders concerning the situation depicted in the model, including decision-making rules and behavior. Finally, the output from the game is analyzed to verify or modify processes within the model, and for the purposes of encoding agent behaviors within a computerized ABM. The rules and structure of the RPG are often simplified to promote player understanding, while ABMs capture greater detail. Together they serve as shared representations of the target system and as tools to simulate scenarios with stakeholders (Bousquet and Trébuil Citation2005).
2.4.2 Types of data produced
The RPG and post-game interviews provide data on stakeholders’ decision-making processes and behavior in the defined problem domain. To minimize error, individual interviews and group discussions are used to cross-check researcher interpretations of what the players did during the game and why. These decision-making processes are transformed into rule-based agents appearing in the ABM. Far more than in any other approach, changes in the perception and the future action of involved stakeholders are to be expected during the model-building process, because their understanding of the situation might change through the RPG, and the interaction with the ABM and the researchers.
2.4.3 Questions that can be addressed with the technique
Specific research questions depend on context and are subject to evolution and modification during the research process, as new questions or hypotheses can be added. In general, companion modelling can help modellers to:
-
analyze the interactions among actors, their institutions, and the natural environment;
-
evaluate the process of collective decision-making as observed within the RPG context; and
-
improve the stakeholder's knowledge of the diversity of perceptions and beliefs held in the community.
2.4.4 Strengths and weaknesses
In most cases companion modeling requires a multidisciplinary research team that includes both natural and social scientists. Sometimes it is difficult to define the exact task and role of the researcher, which may range from facilitator to mediator, observer or stakeholder. RPGs are very costly, time-consuming, and difficult to set up when there are serious social and political conflicts between stakeholders. However, the RPG can elicit tacit knowledge since, within a particular environment and game, a participant may base responses on individual knowledge that (s)he may not be able to express in other acquisition methods.
The quality of data depends heavily on the quality of the selected stakeholders who play the RPG and how well the researchers can assess the interactions that emerge during the game. Also, some games allow open communication and interaction among players, and it is often too difficult to record every action and contextual variable. To maintain understanding, game sessions are limited by the number of players and rounds of play (). Unfortunately there is always a risk of failure, even if the game is pre-tested,Footnote 7 because players may have varying degrees of willingness to participate and varying motives or reasons for participating. However, failure of a session does not necessarily mean the failure of the RPG itself, as the RPG may reveal new approaches, methods, or issues that would engage stakeholders in the companion modeling process. Also, in the event of a failed RPG, researchers still derive new insights into the problem being investigated by the increased interaction among stakeholders and researchers.Footnote 8
Table 4. Strengths and weaknesses of companion modeling
2.4.5 Case study: access to credit in Northern Thailand
Problem: Collective learning is evolutionary: solving a problem might raise a new one. This is what happened when an experiment conducted in the highlands of Northern Thailand sought to address a soil erosion problem (Trébuil et al. Citation2002) and discovered the real issue was access to credit. Ethnic minorities located in these highlands were accused by the Thai government of generating soil erosion and were threatened with restricted access to land. To solve the problem, companion modeling was used to stimulate a learning process on this issue within a community of highland farmers. The participants identified perennial crops as a promising solution to limiting soil erosion while securing higher and more stable incomes. But they also raised a social equity issue: in which many poor small land-holders did not have access to credit for investment in perennial crops. This case study is focused on the latter issue associated with credit access, and subsequent effects of credit access on crop choices and soil erosion (Barnaud et al. Citation2005).
Study area: The physical system is a 369 ha watershed, containing the Mae Salaep village, in Chiang Rai Province, Northern Thailand. In the last two decades, Mae Salaep farmers have integrated into the market economy, and their former farming system based on swidden cultivation has been replaced by permanent and cash crop-based agriculture. These changes have led to an extensive socioeconomic differentiation among farming households having different amounts of resources, socio-economic interests and land use practices.
Informal and formal credit systems co-exist in the village and were represented in the model. Informal credit is given and taken among villagers, via social networks or informal money lending contracts. Formal credit is available through government sources, but currently only accessible to well-off households. This unequal access to credit is only partially compensated by its redistribution through informal loans within networks of acquaintances.
The model: In the model as in the game, the players search for and/or pay back credit each year if needed, make decisions regarding off-farm employment, allocate crops in their fields, harvest products and sell them in the market, and pay family expenses. The spatial interface is a reproduction of the gaming board, i.e. a simplified representation of a small watershed with various slopes and small squares representing farmers’ plots. The main agents in the model are 12 farmers with various amounts of resources, corresponding to the 12 players of the RPG. In the model, farmers’ decision-making processes are represented, whereas in the game, players make decisions themselves. Likewise, networks of acquaintances were also represented in the model that were not explicitly represented in the RPG. The model was used to explore with the villagers various scenarios suggested by them with new rules of allocation of formal and informal credit.
Data collection: data collected through participant observation and semi-structured interviews were used to conceive and calibrate agent behavior of the first model and the RPG focusing on erosion. A second set of field interviews were conducted on the request of the participants to analyze the interaction between the adoption of perennial crops and access to credit. The RPG sessions allowed us to validate and to better understand farmers’ behavior as it revealed tacit knowledge about the credit system. In addition to observing participant behaviors during the gaming sessions, post-RPG interviews clarified this behaviorFootnote 9 and assessed villagers’ perceptions of how closely the game corresponded to the way they perceived reality. The collective discussions with local stakeholders stimulated by the use of the model provided information about people's preoccupations and were used to adjust the model accordingly.Footnote 10
2.5 GIS and remotely sensed spatial data
2.5.1 Definition and description
A wide range of spatially explicit data has become available on various aspects of land use and land cover. These spatial data are extremely useful for deriving input variables that reflect the drivers of land use suggested, for example, by the Von Thünen and Ricardian theoretical frameworks. These frameworks suggest that both accessibility to markets and biophysical suitability influence land use. Spatial data may also reveal important influences on decision-making outside market contexts. For example, neighborhood relationships may affect technology adoption (Berger Citation2001, Case Citation1992), and local accessibility may affect the probability of forest conversion (Mertens and Lambin Citation2000, Deadman et al. Citation2004).
Spatial data can be analyzed using spatial statistical inference methods. A goal of this approach for supporting empirical ABMs is to analyze land use histories in order to test hypotheses about relationships between agent decisions (e.g. land-use change) and a range of spatial and contextual variables, and to establish quantitative parameters for those relationships. A number of analytical methods are available for pursuing this approach, including panel data analysis (Hsiao Citation1986, Seto and Kaufmann Citation2003), survival analysis (An and Brown, in review), and multivariate limited dependent variable models (Mertens and Lambin Citation2000, Parker and Munroe Citation2006).
2.5.2 Types of data produced
Spatial data can be used to derive maps of physical networks (e.g. waterways), mobility impedance (or friction) surfaces, biophysical characteristics (e.g. soil), demographic variables, political and institutional boundaries, land use, and land cover. These data can then be used as input into ABMs, as maps of the environment within which the agents interact, through derived measures such as travel costs or neighborhood relationships that may serve as inputs to agent decision models, or through spatial statistical modeling to calibrate agent preference or behavior parameters that describe agent–environment relationships.
2.5.3 Questions that can be addressed with the technique
Spatial data inputs are most suited to answering questions about the relationships between factors external to the agents and the agent decisions, where the agent decisions are readily observable. Such questions can include:
-
What is the relative influence of biophysical factors, such as soil fertility, on the probability that an agent will convert from one land use to another?
-
How do biophysical factors interact to affect particular decisions?
-
How do neighborhood characteristics affect decision-making?
-
How do spatial relationships vary over time and space?
2.5.4 Strengths and weaknesses
Spatial data are often readily available, often inexpensive, cover large geographic areas, and may extend to include historical conditions (e.g. remote sensing; ). Because the method relies on inference from existing data, it is limited to questions that involve existing data, and requires a pre-specified set of hypotheses—there is no mechanism to discover new decision-making frameworks or structures. In particular, actions, characteristics, and motivations of human actors are rarely directly revealed through data on spatial outcomes. A host of other issues also exist, such as those associated with matching the scale of spatial data to the scale of agent decision-making (Liverman et al. Citation1998), disaggregating data to inform agents (the ecological fallacy; Schuessler Citation1999), unobserved variables driving the underlying processes, non-stationarity in time and space, the fact that observed land use outcomes may be the result of competition between multiple agent groups, and incongruity between the observations used to fit the statistical model and the agents in the ABM.
Table 5. Strengths and weaknesses of GIS and remotely sensed spatial data
2.5.5 Case study: interacting agents, spatial externalities and the evolution of residential land use patterns
Problem: recent changes in urban land use patterns in the United States are characterized not only by urban decentralization and the formation of new suburban subcenters, but also by low density, fragmented residential development in outer suburban and urban–rural fringe areas. The traditional bid–rent model based on transportation costs to the central city fails to explain the observed fragmentation of the residential land use pattern. A study by Irwin and Bockstael (Citation2002) aimed to investigate whether the repelling effect of negative interactions, e.g. due to congestion externalities, offers an agent-level explanation for scattered development.
Study area: the analysis used georeferenced data on land parcels from an exurban region of central Maryland. Washington D.C. is the major urban center in the study region.
The model: agents were conceived of as rural landowners associated with an individual parcel and thus are fixed in space. Agents were assumed to be profit-maximizers and respond to underlying market forces that influence the relative net returns of converting their parcel to development. Probability of parcel conversion was related to factors that include proximity to major urban centers, which is hypothesized to bid up the returns to development; zoning restrictions, which are hypothesized to depress the potential returns to development; parcel characteristics influencing conversion costs, such as steepness of slopes; and the opportunity cost of developing the parcel, which is approximated by its soil type because better farmland has a higher opportunity cost associated with it. To represent neighborhood interactions, the percent of developed neighboring land within a certain distance was measured for each developable parcel to capture the potential spillover effects of neighbors on a parcel's conversion probability.
A constant regional demand for new housing was assumed, and the parcel with the highest probability of conversion in each time period was chosen for conversion, where a time period was arbitrarily defined as the period long enough for one conversion to take place. To account for the changing landscape, neighborhood interaction effects were recalculated after each predicted conversion for the unrestricted case.
Data collection: a GIS database on land use, values, suitability, and accessibility was developed from publicly available data sources. A Cox's proportional hazards model was estimated using all parcels that could have been converted to residential land use as of 1991, based on the year in which subdivision of an undeveloped parcel took place between 1991 and 1997. Furthermore, the estimated hazard rate of parcel development was a function of the relative amount of neighboring development and the other explanatory variables.
Estimated parameters from the statistical models were used in the simulation model in two forms: the unrestricted model, in which an interaction effect is accounted for, and a restricted model, in which the interaction effect is set to zero. Simulations using both the full and restricted models’ estimated parameters were carried out for multiple development events, and the results were then compared with the actual pattern of subdivision development that occurred for a given sub area of the study region. The simulation results show that the inclusion of the negative interaction effects generates a pattern that is significantly more fragmented and one that appears to mimic more closely the actual pattern of residential subdivision development. Based on this evidence, the authors concluded that the estimated negative interactions effect among landowners is sufficiently strong to cause a repelling effect among developed parcels.
3 Discussion
Multiple methods exist to gather data on micro processes for land use systems. Since these systems are composed of multiple actors that interact with each other and their environment in complex ways across space, time, and scale, ABM provides one formalism for integrating the many elements that comprise the land use system (Parker et al. Citation2003). We identify the following key questions that should be answered about the micro processes of an ABM designed to explore questions relevant to land use science:
-
Social and biophysical environment
-
what environmental or social factors influence actor decisions and what are their relative strengths of influence?
-
-
Agents
-
what are the primary classes of actors and how many are there of each?
-
who interacts with whom (type of interaction, frequency and conditionality)?
-
-
Agent behavior
-
what decision models and cognitive processes do actors use to make decisions?
-
do actors adjust their decision making or learn—if so, when and how?
-
what differences exist between actors with regard to these processes?
-
-
Temporal aspects
-
what is the sequence and duration of agent actions and interactions, event occurrences, and information updates for agents?
-
Each of the five empirical methods (sample surveys, participant observation, field and laboratory experiments, companion modeling, and GIS and remotely sensed spatial data) have comparative advantages and complementarities for informing these ABM components. While our discussion focuses on a comparison of the empirical methods for informing each of these components in land use ABMs, other axes of measurement and comparison exist (Agarwal et al. Citation2002, Parker et al. Citation2002, Janssen and Ostrom Citation2006, Parker et al., in press).
3.1 Comparisons of methods
There is no perfect single data collection method that can inform all aspects of a complex ABM of land use and land cover change. Each method is different in its focus. For example, surveys target individuals’ characteristics, constraints, and previous decisions of households; field and laboratory experiments target individuals’ behaviors in a controlled setting; companion modeling targets group dynamics; participant observation reveals the contextual factors influencing individual and group decision-making; and spatial data analysis focuses on social and biophysical characteristics encoded into the landscape. Graphing the methods according to the ABM components listed above () provides a comparison of the capability of each empirical technique for obtaining information on (a) biophysical versus social systems, (b) quantitative versus qualitative descriptions of agent characteristics and behavior, (c) agent interaction versus learning, and (d) temporal versus spatial information. While the placement of each empirical approach on the axes could be considered subjective, we have reached a general consensus among the coauthors regarding their relative positions to each other. The graphs provide a much needed holistic view of how different types of empirical data inform ABMs and how the different approaches compare relative to each other. Next we compare each approach for informing the ABM components listed above.
Figure 1. Comparison of five empirical approaches on several different axes. The five approaches as discussed throughout the paper are sample surveys (SUR), participant observation (PO), field and laboratory experiments (EXP), companion modeling (CM), and GIS and remotely sensed spatial data (SPAT). The graphs compare empirical approaches on (A) their ability to reveal spatial and socioeconomic heterogeneity in agent characteristics and behaviors; (B) their ability to reveal agent interactions and agent learning; (C) their ability to produce quantitative or qualitative information about agents; and (D) the depth of information available and the source of information as stated versus revealed.
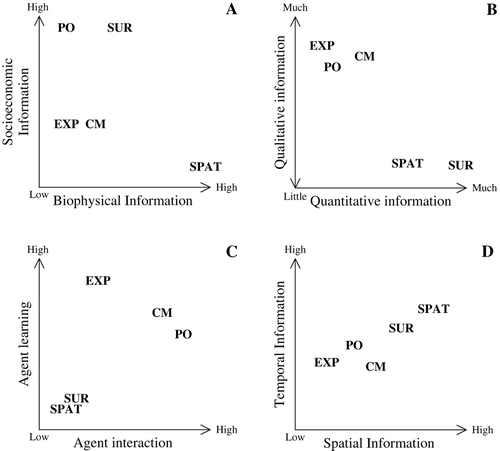
Social and biophysical environment: spatial data are the most readily available and abundant source of multi-date data on the spatial heterogeneity of the biophysical environment (, ). However, like surveys and field and laboratory experiments that are constrained by their structure of questioning or testing, it is extremely difficult to identify new processes or their underlying processes using spatial analysis methods. The flexibility of participant observation and companion modeling provide a more detailed representation of the social environment, and can identify social networks that may influence actor decisions. However, spatial data, like surveys, provide quantitative measures of outcomes of agent behaviors through the quantity and location of social and environmental changes across the landscape; whereas the other methods produce qualitative interpretations.
Agents: our ability to identify types of agents, the abundance within a type of agent, the proportion of the overall population that is composed of different agent types, and variation in agent characteristics within a single type of agent acting in a target system is dependent on the scope and scale of analysis, as well as the degree of heterogeneity one wishes to capture. Heterogeneity, through agent categorization (i.e. types of agents) and variation (i.e. continuous variation in the characteristics of agents; Brown and Robinson Citation2006) is a central component of most ABMs because it influences adaptation (Axelrod and Cohen Citation2000) and acts as a trigger initiating different behaviors among agents within an ABM (Holland Citation1995). Each of the empirical methods addresses one or both aspects of heterogeneity to identify the types and characteristics of the agents that represent the actors in the target system.
Empirical data collection by surveys is guided a priori by theory and therefore the researcher often develops questions and structures the survey to target specific actors in the target system. In the Uganda case study discussed above, a survey of all farming households obtained extensive quantitative information on the characteristics and heterogeneity of those households. However, subsequent subdivision of households into multiple household (agent) types was imposed based on interpretation by the researchers and their analysis of household characteristics derived from the survey. In some cases the defined agent types may not have any relevance to those agents acting within the system (Axelrod and Cohen Citation2000).
Similar to surveys, the use of field and laboratory experiments, and GIS and remotely sensed spatial data to obtain information on agent types, abundances, and variation within types is highly constrained by the theory guiding experimental design and spatial analyses. Therefore, like surveys, these methods also trade-off the ability to identify agent types for increased knowledge of agent variation. As well, spatial data rarely ever directly reflect agent characteristics. While spatial analyses are strictly quantitative, experiments involve a great deal of qualitative measurement, especially when trying to identify and compare decision models, and decision making behaviors and reasoning.
In contrast, through participant observation, Huigen (Citation2004) identified different farming practices and behaviors in the Philippines based on ethnic and religious background of farming households. However, participant observation by itself was not able to quantify variation in the demographic, socio-economic, and network characteristics of the actors, as well as the landscape within which they were situated. Thus, qualitative characteristics (e.g. high- or low-income earners) provided heterogeneity and variation among agents in the developed ABM. The qualitative nature of participant observation may also identify benchmarks that act as guiding principles when defining the range of an agent characteristic.
Companion modeling provides a special case, whereby agent heterogeneity is initially guided by surveys, literature, and existing data for the initial design of the RPG, but the highly iterative and adaptive nature of the game permits further identification of agent categories. Like participant observation, companion modeling primarily produces qualitative data (). Because companion modeling often has a one-to-one representation between agents and actors in the real system there is often a small number of participants and therefore much of the heterogeneity of actor/agent variation is lost or simplified in order to maintain a comprehensible model for stakeholder participation. Both participant observation and companion modeling can be used to develop theory.
Agent behavior: it is extremely difficult if not impossible to validate the correspondence between the structure of the actor's behavior in the real system and our agent-based representation of that actor in our ABM (Couclelis Citation1986). Instead, we are forced to observe the outcomes of actor behaviors and fit our behavioral models to those data. While some empirical data collection methods are better at testing alternative behavioral models (e.g. lab and field experiments) others are better at identifying thresholds or ranges influencing a change in the behavior of an actor (e.g. surveys).
Typically, agent behavior has been described based on the type of behavioral model implemented in an ABM, such as heuristics (e.g. Deadman et al. Citation2004); optimization or utility maximization (e.g. Caruso et al. Citation2005, Schreinemachers and Berger Citation2006); bounded rationality (Arthur Citation1994); satisficing; belief, desires and intentions; and evolutionary processes (Reschke Citation2001). However, to frame our discussion on empirically informing agent behavior we define three classes of behavioral models: (1) decision-making, (2) learning, and (3) adaptation models. The level of complexity increases with each class, respectively, and the data required to inform each model differs.
Heuristics: decision-making behavior includes all functions that transform a stimulus into a response. In the case of heuristics, simple ‘IF … THEN’ clauses are often used to describe the outcome behavior of a stimulus. The dynamic and repeated observation and logging of actor behaviors under different scenarios by participant observation and companion modeling makes them well-suited to derive ‘IF … THEN’ heuristic information. To a lesser extent, experiments are also able to derive ‘IF … THEN’ types of decision-making behavior; however, experiments are more abstract and may be better suited to general and qualitative questions. It may be possible to obtain quantitative data from surveys to define ranges or thresholds whereby actors change their behavior in the target system. However, gleaning this information from GIS and remotely sensed data is much more difficult.
Optimization: a utility value or fitness measurement can also be used to evaluate potential decisions. If the agent has complete information and can select the behavior that maximizes his/her utility among all possible options then the model incorporates completely rational agents. If the agent attempts to maximize its utility over some constrained range of options or achieve a minimum level of utility then the model incorporates bounded rationality or satisficing, respectively. Survey data are particularly well suited to obtaining preference information influencing agent decisions. However, the predefined structure of sample surveys methods tend to be poor at identifying the underlying mechanisms driving landscape patterns, such as the decision-making approach (e.g. profit maximizing versus satisficing), agent learning, or agent interaction (). In contrast, experimental methods are well suited for testing alternative theories of decision-making.
Preference data can also be ordered, ranked, or measured from survey collection methods to develop weights that are applied to stimulus factors in the behavioral model used by an agent. Similarly, if lab and field experiments are designed for a specific problem, this type of quantitative data may be obtained. Utility functions and calculations derived from participant observation, companion modeling, and GIS and remotely sensed data are subject to greater uncertainties and are not recommended as data collection methods for this behavioral approach.
While decision-making involves transforming a stimulus to a response, learning adds an additional level of complexity by incorporating memory. Some agent behaviors incorporate learning in the form of mimicry, experimentation, or self-reinforcing behavior among others. Learning may permit an agent to improve its performance and efficiency to exploit a specific behavior under a given environmental and socio-economic context, but may also cause it to be harmed if the situation changes and it has not explored alternative behaviors or strategies (March Citation1991).
Directly measuring learning is difficult but possible using each empirical approach. For example, surveys could question how long a respondent had knowledge of a potential crop before it was adopted for plantation; participant observation may ask such a question verbally, or record the number of times a visitation (reconnaissance) occurred between an early adopter and a laggard before the laggard adopted; in companion modeling, researchers could test out different types of information diffusion strategies and measure the relative adoption rate among actors. As far as we are concerned with land use and land cover change decisions, a multi-temporal analysis using GIS and remotely sensed spatial data can derive the adoption rate of new farming practices or crops chosen among a community.
Laboratory and field experiments are perhaps best suited to identifying the structure of the learning process where the repeated decisions of actors are recorded (e.g. Evans et al., Citation2006). Because these experiments test alternative models in controlled settings, they provide the least amount of discrepancy between the conceptually defined ABM and the operationally defined experiments. While the contextual variables that are identified by companion modeling and participant observation approaches assist the modeler to better understand the target system, most of these data rarely enter into the ABM. However, both methods are useful for identifying agent behaviors, and when participants are allowed to reflect on their choices, agent motivations.
Temporal aspects: the data collection methods listed above rarely address issues related to the timing of agent actions: in an absolute sense (e.g. timing of a decision, especially in relation to timing of potential drivers), in a relative sense (e.g. agent A does not make a decision until agent B has), or in an evolutionary sense (e.g. how do agents’ decisions change over time in response to changing internal and external conditions?). Various temporal aspects of decision making may be implicitly assumed based on the conceptual model. For example, if a land-use model of residential location is demand-driven, then the residents would make decisions before developers; if the model was supply driven then the opposite would likely occur. We are still ill-equipped in our data collection methods to define the frequency, duration, and order of agent actions, agent decision-making processes, and event occurrences over time.
Perhaps the most important issue in using data collection methods to support ABM is that modeling is a process that involves an iterative cycle of observation, modeling, prediction and testing. This process may involve multiple data sources (especially to ensure separation of calibration and validation data) and development of multiple models. Clearly the best way to empirically inform an ABM is to use some combination of approaches. The examples given of participant observation and companion modeling illustrate this point. It is also evident that some combinations may prove more useful than others. Defining all relevant combinations is outside the scope of this paper, as empirical data methods are best tailored to suit the specific research question and modeling needs of each project.
4 Conclusions
Development of models applied to the social, environmental and resource sciences has presented a number of unprecedented opportunities to formalize, synthesize, and improve our understanding of complex human–environment interactions. In modeling land-use and land-cover change as heterogeneous human and environment systems, important questions are raised about how land managers collect information about the environment, form beliefs, make decisions, take action, and learn from those actions. To answer these questions and test land-management and decision-making hypotheses, a growing number of projects are using ABM techniques. However, a general lack of literature summarizing the alternatives for providing micro-level data has produced a need for a summary of existing methods being used to inform ABM.
This paper reviewed five empirical approaches used to inform ABMs in land-use science. We describe the strengths and weaknesses, as well as compare the uses of each approach for typical ABM components. Our focus has been on methods associated with collecting information about human and social actors. The diversity of these methods and their disciplinary pedigrees highlights the importance of working in multi-disciplinary teams when developing ABMs of land-use systems. This same diversity points to the potential for ABM as a platform for integration of multiple social science perspectives with ecological perspectives. By providing modelers with a framework for evaluating the utility of various data collection methods, we hope that our description and comparison of methods will contribute to improved integrated modeling of human and natural systems.
Acknowledgements
The authors would like to express gratitude to the Robert Bosch Foundation, the CoCE project funded by the German Ministry of science and Education (BMBF), and the Center for Development Research (ZEF—Bonn) for providing funding and accommodations that permitted us to conduct our ‘Multi-Agent Modeling and Collaborative Planning—Method2Method Workshop’. In addition to the authors of this paper we would like to acknowledge the contributions of the following individuals who also participated in the workshop: Richard Aspinall, Nicolas Becu, Kathrin Happe, Oliver Jungklaus, Maction Komwa, Pieter van Oel, Koen Overmars, Claudia Pahl-Wostl, and Ole Benjamin Schroeder. Additional support was provided by the Scottish Executive Environment and Rural Affairs Department US NSF grant 0414565 and NSF Biocomplexity in the Environment program (BCS-0119804) for project SLUCE at the University of Michigan.
Notes
1 The two terms are roughly synonymous and we use agent-based model (ABM) throughout.
2 The workshop, titled ‘Multi-Agent Modeling and Collaborative Planning—Method2 Method Workshop,’ was held in Bonn, Germany, 8–9 October 2005, as a pre-conference workshop of the Open Meeting of the Human Dimensions of Global Change.
3 An alternative method for identifying micro-level parameters is to calibrate decision models based on fit to macro outcomes (e.g. Caruso et al. Citation2005, Evans and Kelley Citation2004).
4 The model is freeware that can be downloaded from http://www.uni-hohenheim.de/mas/software/. A manual is available electronically from the same location.
5 There is, however, no way to guarantee avoiding such strategic behavior. One way to deal with this is by using group interview techniques, in which person A is asked to explain the actions and motivations of person B. Person A may then be less inclined to be strategically motivated in his/her answers; the answers are likely to derive from a mixture of the motivations of the two people. If B is given the option to react to A's description of B's actions then there is a good chance of getting closer to the truth.
6 There are techniques which aim to minimize the effects of bias. The researcher should take sufficient time to reflect on his/her findings. In these reflective phases, (s)he reconstructs the exposed realities based on notes, and links the data fragments into a coherent structure—for example, with the action-in-context approach (De Groot Citation1992). Each link between data fragments then consists of new hypotheses to be checked in the field, until the researcher is confident about the consistency of the findings. An anthropological training is therefore highly advantageous to the participant researcher.
7 Testing is best done with students or scientists since testing the game with real stakeholders may distort the behaviors expressed in the real game.
8 The ComMod group, which has initiated this type of approach, has published a charter to explain their scientific posture (http://cormas.cirad.fr/en/reseaux/ComMod/charte.htm), and to stimulate debate among scholars in this field.
9 It is important to make the distinction between behaviors corresponding to the way villagers behave in reality (most of them) and ones reflecting trials or wishes (‘I wanted to try this in the game’).
10 A family of models was obtained in which each model is seen as a subjective representation of the system at a particular moment of the collective learning process among a particular set of stakeholders.
References
- Agarwal, C., Green, G. M., Grove, J. M., Evans, T. and Schweik C., 2002, A review and assessment of land-use change models: dynamics of space, time, and human choice. USDA Forest Service Northeastern Forest Research Station Publication NE-297 http://www.fs.fed.us/ne/newtown_square/publications/technical_reports/pdfs/2002/gtrne297.pdf (Accessed: 23 August 2006 ).
- An , L. and Brown , D. G. Accepted, Survival analysis in land-change science: integrating with GIS and remote sensing in Southeastern Michigan . Annals of Association of American Geographers ,
- Arthur , B. W. 1994 . Inductive reasoning and bounded rationality . The American Economic Review , 84 ( 2 ) : 406 – 411 .
- Axelrod , R. M. and Cohen , M. D. 2000 . Harnessing Complexity: Organizational Implications of a Scientific Frontier , New York, NY : Basic Books .
- Barnaud , C. , Promburom , P. , Trébuil , G. and Bousquet , F. . Companion modelling to support collective land management in the highlands of Northern Thailand . Proceedings of the International conference on Natural Resources Related Conflicts Management in Southeast Asia . September 6–8 2005 . Khon Kaen, , Thailand : Institute of Dispute Resolution, Khon Kaen University .
- Berger , T. 2001 . Agent-based spatial models applied to agriculture: a simulation tool for technology diffusion, resource use changes, and policy analysis . Agricultural Economics , 25 ( 2–3 ) : 245 – 260 .
- Berger, T. and Schreinemachers, P., 2006, Creating agents and landscapes for multiagent systems from random samples. Ecology and Society, 11(2): 19 http://www.ecologyandsociety.org/vol11/iss2/art19/ (Accessed: 20 February 2007 ).
- Bharwani , S. , Bithell , M. , Downing , T. E. , New , M. , Washington , R. and Ziervogel , G. 2005 . Multi-agent modelling of climate outlooks and food security on a community garden scheme in Limpopo, South Africa . Philosophical Transactions of the Royal Society B , 360 : 2183 – 2194 .
- Bousquet , F. and Trébuil , G. . Synergies between multi-agent systems and role-playing games in companion modeling for integrated natural resource management in Southeast Asia . SIMMOD 05 International Conference on Simulation and Modeling 2005 . January 17–19 . Edited by: Kachitivichyanukul , V. , Purintrapidan , U. and Utayopas , P. pp. 461 – 469 . Bangkok, , Thailand : Asian Institute of Technology . Asian Institute of Technology, Bangkok, Thailand
- Brown, D. G. and Robinson, D. T., 2006, Effects of heterogeneity in preferences on an agent-based model of urban sprawl. Ecology and Society, 11(1). http://www.ecologyandsociety.org/vol11/iss1/art46/ (Accessed: 8 August 2006 ).
- Brown , D. G. , Walker , R. , Manson , S. and Seto , K. 2004 . “ Modeling land use and land cover change ” . In Land Change Science: Observing, Monitoring and Understanding Trajectories of Change on the Earth's Surface , Edited by: Gutman , G. , Janetos , A. C. , Justice , C. O. , Moran , E. F. , Mustard , J. F. , Rindfuss , R. R. , Skole , D. L. , Turner , B. L. and Cochrane , M. A. 395 – 409 . New York : Springer .
- Camerer , C. F. 2003 . Behavioral Game Theory: Experiments in Strategic Interaction , Princeton, NJ : Princeton University Press .
- Cardenas , J. C. , Stranlund , J. and Willis , C. 2000 . Local environmental control and institutional crowding-out . World Development , 28 ( 10 ) : 1719 – 1733 .
- Cardenas , J. C. and Ostrom , E. 2004 . What do people bring into the game? Experiments in the field about cooperation in the commons . Agricultural Systems , 82 ( 3 ) : 307 – 326 .
- Caruso , G. , Rounsevell , M. and Cojocaru , G. 2005 . Exploring a spatio-dynamic neighbourhood-based model of residential behaviour in the Brussels periurban area . International Journal of Geographical Information Science , 19 ( 2 ) : 103 – 123 .
- Case , A. 1992 . Neighborhood influence and technological change . Regional Science and Urban Economics , 22 : 491 – 508 .
- Castillo , D. and Saysel , A. K. 2005 . Simulation of common pool resource field experiments: a behavioral model of collective action . Ecological Economics , 55 ( 3 ) : 420 – 436 .
- Chambers , R. 1997 . Whose Reality Counts? Putting the First Last , London : Intermediate Technology Publications .
- Couclelis , H. 1986 . A theoretical framework for alternative models of spatial decision and behavior . Annals of the Association of American Geographers , 76 ( 1 ) : 95 – 113 .
- De Groot , W. T. 1992 . Environmental Science Theory: Concepts and Methods in a One-World Problem Oriented Paradigm , Amsterdam and New York : Elsevier Science Publishers .
- Deadman , P. J. 1999 . Modelling individual behaviour and group performance in an intelligent agent-based simulation of the tragedy of the commons . Journal of Environmental Management , 56 : 159 – 172 .
- Deadman , P. J. , Robinson , D. T. , Moran , E. and Brondizio , E. 2004 . Colonist household decisionmaking and land-use change in the Amazon Rainforest: an agent-based simulation . Environment and Planning B: Planning and Design , 31 ( 5 ) : 693 – 709 .
- Deffuant , G. , Huet , S. and Amblard , F. 2005 . An individual-based model of innovation diffusion mixing social value and individual benefit . American Journal of Sociology , 110 ( 4 ) : 1041 – 1069 .
- Duffy , J. 2006 . “ Agent-based models and human-subject experiments ” . In Handbook in Agent-Based Computational Economics , Edited by: Tesfatsion , L. and Judd , K. New York : Elsevier .
- Evans , T. P. and Kelley , H. 2004 . Multi-scale analysis of a household level agent-based model of landcover change . Journal of Environmental Management , 72 : 57 – 72 .
- Evans , T. P. , Sun , W. and Kelley , H. 2006 . Spatially explicit experiments for the exploration of land use decision-making dynamics . International Journal of Geographic Information Science , 20 ( 9 ) : 1013 – 1037 .
- Goldstone , R. L. , Jones , A. and Roberts , M. 2006 . Group path formation . IEEE Transactions on System, Man, and Cybernetics, Part A Systems and Humans , 36 ( 3 ) : 611 – 620 .
- Grimm , V. , Revilla , E. , Berger , U. , Jeltsch , F. , Mooij , W. M. , Railsback , S. F. , Thulke , H. -H. , Weiner , J. , Wiegand , T. and Deangelis , D. L. 2005 . Pattern-oriented modeling of agent-based complex systems: lessons from ecology . Science , 310 ( 5750 ) : 987 – 991 .
- Grosh , M. and Glewwe , P. 2000 . Designing Household Survey Questionnaires for Developing Countries: Lessons From 15 Years of the Living Standards Measurement Study , Vol. 1–3 , Washington, DC : World Bank .
- Happe, K., 2004, Agricultural policies and farm structures: agent-based modelling and application to EU-policy reform. Studies on the Agricultural and Food Sector in Central and Eastern Europe, 30, IAMO http://www.iamo.de/dok/sr_vol30.pdf (Accessed: 8 August 2006 ).
- Henrich , J. , Boyd , R. , Bowles , S. , Camerer , C. , Fehr , E. and Gintis , H. 2004 . Foundations of Human Sociality: Economic Experiments and Ethnographic Evidence from Fifteen Small-Scale Societies , Oxford : Oxford University Press .
- Holland , J. H. 1995 . Hidden Order: Hoe Adaptation Builds Complexity , Cambridge, MA : Perseus Books .
- Hsiao , C. 1986 . Analysis of Panel Data , Cambridge, , UK : Cambridge University Press . (Economic Society Monographs No. 11)
- Huigen , M. G. A. 2004 . First principles of the MameLuke multi-actor modeling framework for land use change, illustrated with a Philippine case study . Journal of Environmental Management , 72 : 5 – 21 .
- Huigen, M. G. A., Overmars, K. P. and De GROOT, W. T., 2006, Multi-actor modeling of settling decisions and behavior in San Mariano watershed, the Philippines: a first application with the MameLuke framework. Ecology and Society, 11(2): 33 http://www.ecologyandsociety.org/vol11/iss2/art33/
- International Food Policy Research Institute (IFPRI), 2005, Data sets. http://www.ifpri.org/data/data set.asp (Accessed: 8 August 2006 ).
- Irwin , E. G. and Bockstael , N. E. 2002 . Interacting agents, spatial externalities and the evolution of residential land use patterns . Journal of Economic Geography , 2 ( 1 ) : 31 – 54 .
- Jager , W. and Janssen , M. A. 2002 . “ Using artificial agents to understand laboratory experiments of common-pool resources with real agents ” . In Complexity and Ecosystem Management: The Theory and Practice of Multi-Agent Systems , Edited by: Janssen , M. A. 75 – 102 . Cheltenham : Edward Elgar Publishers .
- Janssen, M. A. and Ahn, T. K., 2006, Learning, signaling and social preferences in public good games. Ecology and Society, 11(2): 21 http://www.ecologyandsociety.org/vol11/iss2/art21/
- Janssen, M. A. and Ostrom E. 2006, Empirically based agent-based modelling, Ecology and Society 11(2): 37 http://www.ecologyandsociety.org/vol11/iss2/art37/
- Liverman , D. , Moran , E. F. , Rindfuss , R. R. and Stern , P. C. 1998 . People and Pixels: Linking Remote Sensing and Social Science , Washington, DC : National Academy Press .
- March , J. G. 1991 . Exploration and exploitation in organizational learning . Organizational Science , 2 ( 1 ) : 71 – 87 .
- Mertens , B. and Lambin , E. F. 2000 . Land-cover change trajectories in southern Cameroon . Annals of the Association of the American Geographers , 90 : 467 – 494 .
- Ostrom , E. 1998 . A behavioral approach to the rational choice theory of collective action . American Political Science Review , 92 ( 1 ) : 1 – 22 .
- Parker, D. C., Berger, T. and Manson, S. M. (Eds), 2002, Agent-based Models of Land-use and Land-cover Change. LUCC Report Series, 6 (Louvain-la-Neuve: LUCC International Project Office) http://www.indiana.edu/∼act/focus1/ABM_Report6.pdf (www.indiana.edu/~act/focus1/ABM_Report6.pdf) (Accessed: 14 August 2006 ).
- Parker , D. C. , Manson , S. M. , Janssen , M. A. , Hoffmann , M. J. and Deadman , P. J. 2003 . Multi-agent systems for the simulation of land-use and land-cover change: a review . Annals of the American Association of Geographers , 93 ( 2 ) : 314 – 337 .
- Parker , D. C. and Munroe , D. K. 2007 . The geography of market failure: edge-effect externalities and the location and production patterns of organic farming . Ecological Economics , 60 ( 4 ) : 821 – 833 .
- Parker , D. C. , Brown , D. G. , Polhill , J. G. , Manson , S. M. and Deadman , P. J. in press . “ Illustrating a new ‘conceptual design pattern’ for agent-based models and land use via five case studies: the MR POTATOHEAD framework ” . In Agent-based Modelling in Natural Resource Management , Edited by: Paredes , A. L. and Iglesias , C. H. Pearson Education .
- Reschke, C. H., 2001, Evolutionary perspectives on simulations of social systems. Journal of Artificial Societies and Social Simulation, 4(4) http://jasss.soc.surrey.ac.uk/4/4/8.html (Accessed: 15 August 2006 ).
- Schreinemachers, P., 2006, The (Ir-)Relevance of the Crop Yield Gap Concept to Food Security in Developing Countries. With an Application of Multi-Agent Modeling to Farming Systems in Uganda. University of Bonn Dissertation, Cuvillier Verlag Göttingen http://hss.ulb.uni-bonn.de/diss_online/landw_fak/2006/schreinemachers_pepijn (Accessed: 8 August 2006 ).
- Schreinemachers , P. and Berger , T. 2006 . Land-use decisions in developing countries and their representation in multi-agent systems . Journal of Land Use Science , 1 ( 1 ) : 29 – 44 .
- Schuessler , A. A. 1999 . Ecological inference . Proceedings of the National Academy of Sciences , 96 : 10,578 – 10,581 .
- Seto , K. C. and Kaufmann , R. K. 2003 . Modeling the drivers of urban land-use change in the Pearl River Delta, China: integrating remote sensing with socio-demographic data . Land Economics , 79 ( 1 ) : 106 – 121 .
- Strauss , A. and Corbin , J. 1990 . Basics of Qualitative Research: Grounded Theory Procedures and Techniques , Newbury Park, CA : Sage Publications .
- Trébuil , G. , Bousquet , F. , Baron , C. and Shinawatra-Ekasingh , B. . Collective creation of artificial worlds can help govern concrete natural resource management problems: a Northern Thailand experience . International Symposium on Sustaining Food Security and Managing Natural Resources in Southeast Asia: Challenges for the 21st Century . January 8–11 2002 , Chiang Mai, Thailand.
- Verburg , P. , Kok , K. , Pontius , R. G. and Veldkamp , A. 2006 . “ Modelling of land use and land cover changes ” . In Land Use and Land Cover Change: Local Processes, Global Impacts , Edited by: Lambin , E. and Geist , H. 117 – 135 . New York : Springer .
- World Bank, 2005a, Africa Household Survey Databank http://www4.worldbank.org/afr/poverty/databank/default.cfm (Accessed: 8 August 2006 ).
- World Bank, 2005b, Living Standards Measurement Study (LSMS) Database http://www.worldbank.org/lsms/ (Accessed: 8 August 2006 ).