Abstract
This study examines the determinants of urbanized area across a 10,000-mile square swath in central North Carolina, an area undergoing extensive conversion of forest and agricultural land. We model the temporal and spatial dimensions of these landscape changes using a database that links five satellite images spanning 1976–2001 to a suite of socioeconomic, ecological and GIS-created explanatory variables. By specifying the complementary log-log derivation of the proportional hazards model, we employ a methodology for modeling a continuous time process—the conversion of land to impervious surface—using discrete-time satellite data. Spatial hypotheses are tested using several variables derived from the imagery that measure the landscape configuration surrounding a pixel. Empirical results confirm the significance of several determinants of urbanization identified elsewhere in the literature, including proximity to roads and population density, but also suggest that the parameterization of these variables is biased when the influence of landscape configuration is unaccounted for. We conclude that the inclusion of spatial pattern metrics significantly improves both the explanatory and predictive power of the estimated model of urbanization.
1. Introduction
Over the past two decades, the conversion of farm and forestlands on city fringes throughout the United States has continued unabated, with the urbanized area expanding from approximately 51 to 76 million acres between 1982 and 1997 (Fulton et al., Citation2001). While partly reflecting growing prosperity and preferences for increased living space, this trend has raised concerns on several fronts. Through its strong association to the increase in impervious surfaces, expansion of the urban frontier degrades and fragments natural habitats, contributes to poor air quality through increased reliance on vehicle travel, and disrupts a multitude of ecosystem services, such as aquifer recharge and nutrient cycling. In turn, such disruptions can impose significant costs on municipalities, including higher medical costs for air quality-related illnesses and increased expenditures for the provision of public services and infrastructure. Aesthetic, social and cultural costs may further compound these ecological and health impacts. The movement of populations away from central city areas has been argued to not only contribute to urban blight (Jargowsky, Citation2001), but also a loss of cultural heritage when farmland and forest is replaced by helter-skelter development characterized by strip malls, office parks, and disconnected residential communities (Kunstler, Citation1994).
To the extent that development decisions create landscape mosaics that alter ecological function and constrain the choice set of future land-use alternatives, efforts to understand urban expansion can contribute greatly to land-use planning and environmental policy processes. Models that are fine-scale and spatially explicit are particularly meaningful because ecologists and allied disciplines perceive an intimate connection between the provision of habitat and other services by ecosystems and the pattern of the landscape mosaic in which the ecosystems function. When patches of impervious surface manifest in an open-space matrix, the environment is adversely affected as ecosystems are fragmented and the ratio of edge to interior area increased. Similarly, where development takes place (e.g. in terms of proximity to surface waters) may be as relevant as how much when considering impacts (e.g. aquatic ecosystem stress).
A shift toward spatial explicitness and—to some degree—a finer scale is noticeable in the recent land use science literature. A recurring theme is how the spatial configuration of land use, by virtue of its association to both accessibility and spatially determined amenities, is, itself, an important determinant of conversion of open space to developed uses. In recent years, an increasing number of studies have combined principles from landscape ecology with spatial-econometric methods to account for how human decision-making, ecosystem function, and their interaction effect landscape changes across different spatial scales (e.g. Fang et al., Citation2005; Geoghegan et al., Citation1997; Hite et al., Citation2003; Irwin and Bockstael, Citation2002; Kline et al., Citation2001). Many of these studies have used data from satellite imagery to test hypotheses from behavioral models in which the influence of space plays a prominent role (Chomitz and Gray, Citation1996; Nelson and Hellerstein, Citation1997; Pfaff Citation1999; Srinivisan, Citation2005; Turner et al., Citation1996; Vance and Iovanna, Citation2005). While this has proved a valuable data source for linking social phenomena to observed changes in land cover, to date only limited use has been made of the rich information derivable from the imagery itself for capturing the influence of landscape pattern as a determinant of that change. This information is not only useful for handling statistical problems emerging from spatial autocorrelation (e.g. Nelson and Hellerstein, Citation1997), but can also illuminate the still poorly understood role of natural amenities as drivers of development (Alig et al., Citation2004).
The goal of the present paper is to explore the potential of satellite imagery, and the hazard models derived from it, to explain and predict the conversion of open space to impervious surface. The novelty of our approach relates to both the degree to which we rely on satellite imagery and the use of a modeling specification well suited to a continuous process that is measured in discrete time (by the imagery). Using data from five images spanning the years 1976–2001, the paper examines the drivers of built-up area across a 25,900 km2 swath in central North Carolina, an area that has undergone extensive conversion of forest and agricultural land over the last two decades. The analysis takes as its point of departure a dynamic, profit-maximizing framework that suggests several possible determinants of land conversion from commodity-based to urban uses. We subsequently test for the significance of these determinants with a complementary log-log model derived from the proportional-hazards empirical specification. In addition to including a broad array of time-varying covariates that measure the land allocation response to the site and locational attributes associated with each pixel, the model also includes several GIS-created pattern metrics that serve as controls for spatial autocorrelation, landscape amenities and spatial externalities from neighboring land uses.
Our empirical results confirm the hypothesis that pixel-level characteristics—particularly what surrounds a pixel—have a major influence on the likelihood of its conversion. We also find that the omission of landscape pattern variables can lead to biased inferences regarding the influence of other covariates, such as proximity to the urban core, which are commonly identified as important determinants of land-use change. In addition to goodness-of-fit estimates, cartographic and nonparametric validation exercises provide added support to an unconstrained model that includes such variables. The paper concludes with a simple policy simulation that illustrates the model's practical merit.
2 The study region
The amount of privately owned land, the mix of land uses, and the pace of change among them were leading considerations for site selection of this exploratory exercise. The study region straddles portions of the Piedmont and the Inner Coastal Plain of North Carolina, two distinct physiogeographic zones along a north-south axis across the state (). Over the centuries of human occupation, what had been completely forested has been transformed into a patchwork that now includes croplands, fields in varying stages of abandonment, and, increasingly, built-up areas.
Figure 1. The study region boundaries and physio-geographic zones of North Carolina. Source: Adapted from T.E. Stear, “Population Distribution,” pp. 30–51, in North Carolina's Changing Population (University of North Carolina, Carolina Population Center, Citation1973).
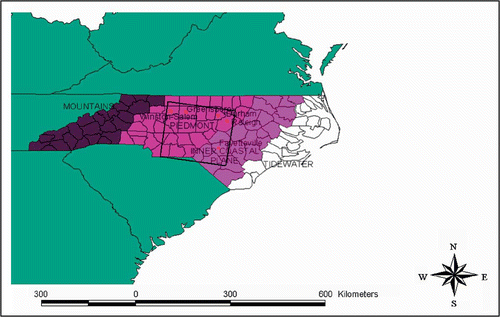
The regional economy has transitioned from one based largely on agriculture to one based on the service sector and on manufacturing, with heavy reliance on the forest-products sector. Although the state remains a major producer of tobacco, sweet potatoes, and hog products, the spatial extent of agricultural production has declined drastically since its peak in the early 1900s (Lilly, Citation1998). And while the extent of forests has remained relatively stable, peaking in the early 1970s at 20.13 million acres and then dropping back down to approach the 1938 level of 18.1 million acres by 1990 (Brown, Citation1993), the U.S. Forest Service anticipates a loss of 30% of North Carolina's privately owned forest by 2040, with the Interstate 85 corridor extending southward from Raleigh-Durham designated as a ‘hotspot’ of forest loss due to continuing urbanization (Prestemon and Abt, Citation2002; Wear and Greis, Citation2002).
In general, North Carolina is a national leader in terms of land-use change. A highly publicized report recently released from Smart Growth America (Ewing et al., Citation2003) ranked Greensboro and Raleigh-Durham as second and third among a listing of 83 U.S. cities in which the spread of development far outpaces population growth. In Raleigh, for example, the population increased by 32% between 1990 and 1996, while its urbanized land area increased nearly twofold (Sierra Club, Citation1998).
3 Theoretical and empirical model
Responding to concern about the rate and extent of land-use change requires understanding the causes, timing and location of land-use change. If we know why and when pressures to develop increase for a given tract, we will be in a better position to evaluate where significant ecological consequences are likely to occur, as well as the merit of conservation responses. The decision to convert depends on a complex multiplicity of factors, including the market value of output from the land in alternative uses, expectations about the future use of neighboring lands, and the surrounding composition of land ownership. Following the work of Capozza and Helsley (Citation1989) and Boscolo et al. (Citation1998), the theoretical approach taken here attempts to structure this complexity by assuming that a unit of land (referred to hereafter as a ‘pixel’ to keep this discussion consistent with the data we ultimately use) will be converted if the net present discounted benefits of doing so are greater than the net present discounted benefits of leaving the land under its present use. In other words, the land manager converts pixel i in period T to maximize the following objective function:
-
Ait is the return derived from a commodity-based use of the pixel in period t, i.e. the agricultural or forestry rent;
-
Dit is the return to development in period t, i.e. the development rent;
-
Xit is a vector of variables that determine returns to development and commodity uses;
-
CT is the cost associated with conversion; and
-
δ is the discount rate, 1/(1+r).
Assuming irreversibility of the conversion process, there are two necessary conditions for conversion to take place: The first is that the discounted stream of returns derived from conversion are greater than that of leaving the pixel in its present use, net of the one-time conversion costs:
The operative condition, however, is one that will be met well after that specified by Equationequation (2)2: conversion will occur when the development rent just equals the opportunity cost, OC, of developing that period as opposed to the next. Before time T and assuming development rents are rising over time and conversion costs are declining, it is more profitable to the landowner to defer development for at least another period. After T, the landowner loses money every period that development is deferred. More formally, a developed pixel is one in which
With equality between development rent in period t and the sum of agricultural rent and the cost savings from deferring development, the pixel converts. EquationEquation (3)3 indicates that higher development rents hasten conversion, while higher agricultural rents, conversion costs, and the rate of decline in costs defer conversion for one pixel relative to another.
The error term inserted in Equationequation (3)3 accounts for unobserved idiosyncratic factors associated with pixel i at time t; the greater it is, ceteris paribus, the less likely is conversion. If we further specify ∊∗ as the amount that makes Equationequation (3)
3 an equality, then we find the likelihood of conversion at time t to simply be the cumulative density of ∊ evaluated at ∊∗. In other words, if the error for pixel i at time t is less than or equal to ∊∗, conversion occurs.
In taking the above framework to the empirics, we focus on the critical role that timing plays in the risk of conversion. Given that conversion may occur at any point in time during the period under observation and that the factors influencing conversion are often continuous processes, survival modeling is uniquely suited to the task of estimating the parameters of interest. Rather than modeling the direct influence of a covariate on conversion probabilities, survival models are concerned with the hazard rate underlying the probabilities, i.e. the instantaneous risk that pixel i is cleared in period t conditional on not having been converted before t. While conventional methods such as linear or logistic regression have been applied in these contexts, they are ill-equipped to handle the features that often characterize survival data, including time-varying explanatory variables and censoring or truncation of the dependent variable.
Derived from satellite imagery, our data are interval censored. We know simply whether or not an observation's survival time falls somewhere between two dates. Accordingly, the dependent variable assumes a value of one if conversion occurs over an interval between the dates and zero otherwise. To reconcile the temporal continuity of the conversion process being modeled with this coarseness in the measurement of timing, and because alternative link functions for binary data are inappropriate for such processes, we specify a complementary log-log survival model. By doing so, the relationship between the X covariates and the probability that opportunity costs (OC) are low enough for conversion to occur (i.e. that ∊ is less than or equal to ∊∗) is assumed to be
It is to the researcher's considerable advantage to rely on the complementary log-log link when formulating the generalized linear model for estimation since it can be derived directly from interval-censored data of event time. As a consequence, a coefficient estimate's relationship to the hazard of conversion is unaffected by time, itself. For alternative estimators, such as the logit or probit, the model fundamentally changes as one shifts consideration from one interval to another (Allison, Citation1995).
As a proportional hazards model and a discrete analogue to that developed by Cox (Citation1972), the complementary log-log model readily accommodates time-varying covariates and requires no assumptions regarding the functional form of the baseline hazard rate. This enables attention to be focused specifically on the effect of the covariates on the relative risk of a transition, which is obtained in a straightforward manner. Unlike the Cox model (such as that used in Irwin et al., Citation2003), the complementary log-log model is estimated using maximum likelihood, rather than partial likelihood, allowing one to readily generate estimates for the effect of time on the odds of a transition and thereby facilitating the model's use for prediction (see Allison, Citation1995, for further discussion).
4 Measurement of conversion
The econometric model presented in this paper is estimated using a time series of five classified Thematic Mapper (TM) and Landsat Multispectral Scanner (MSS) satellite images over central North Carolina for the years 1976, 1980, 1986, 1993, and 2001. The process of imagery classification was preceded by the standard pre-processing activities, including geometric correction, spectral-spatial clustering, and radiometric normalization. Classification then proceeded according to a hybrid change detection methodology combining radiometric and categorical change techniques on a pixel-by-pixel basis. This procedure produced four land cover classes: forest, non-forest vegetation, impervious surface, and water. From these classes, we generated a binary dependent variable equaling one if a conversion from forest or non-forest vegetation to impervious surface occurred between two dates and zero otherwise. The data indicate a 67% increase in impervious surfaces between 1976 and 2001. Although no accuracy assessment of the classification was undertaken, this figure is roughly consistent with the NRI estimate of a 62% increase in urban areas for NC as a whole between 1982 and 1997 (USDA, Citation1997a). In this regard, it also bears noting that errors in the classification of individual pixels, to the extent they are random, will be captured by the error term of the model and not bias coefficients.
Conversions to water were eliminated from the observations used for estimation, as were those relating to pixels whose classification in the first year (1976) was either water or impervious surface. Transitions between forest and non-forest vegetation were also treated as censored as these may be attributable more to forest rotations than permanent conversion from one land use to another. After overlaying two GIS layers of tenure data from ESRI (Citation2000a,Citationb) and the North Carolina Department of Parks and Recreation (2003), those pixels falling under public ownership (e.g. national, state, and municipal parks) were also eliminated.
Upon classifying the imagery, a systematic sample of pixels was drawn that provided 65,991 pixels for model estimation. The grid pattern across the satellite scene was such that roughly 1.2 km separated each pixel from their nearest neighbors. Systematic sampling is a commonly applied technique to handle spatial correlation of unobserved variables that may emerge from the clustering of behavior produced by shared attributes among neighboring units (Cropper et al., Citation2001; Kline et al., Citation2001; Turner et al., Citation1996). The consequences of spatial autocorrelation include inefficient, though asymptotically unbiased estimates. However, in cases in which the unobservable variables are spatially correlated with the included explanatory variables, the coefficient estimates on the included variables will be biased (Irwin and Bockstael, Citation2001). A major source of spatial autocorrelation arises from multiple observations falling under common landowners (Kline et al., Citation2001). Given that the average size of private forest ownership in North Carolina is 9.7 hectares (Powell et al., Citation1992), while the average farm size is approximately 65 hectares (USDA, Citation1997b), 1.2 km pixel separation was deemed an adequate distance to minimize the likelihood of drawing pixels for our sample that belong to the same owner.
5 Explanatory variables and hypothesized effects
Several static and time-varying covariates are included in the model, the values for which correspond to the start year of the interval given by the dates of the satellite imagery (). The suite of site and locational attributes is intended to reflect the supply and demand side factors that influence the likelihood of land-use change. In principle, many of these factors could work in both directions on the conversion hazard, but in what follows we hypothesize those effects that are likely to dominate.
Table 1. Descriptive statistics
5.1 Window metrics
To capture the influence of what Healy (Citation1985) has termed juxtaposition effects—or ‘spatially bounded externalities that affect adjoining or nearby land’ (Alig and Healy, Citation1987, p. 225)—we derived four time-varying window-based metrics from the imagery that measure the landscape configuration surrounding a pixel. The first metric is the percent of the area within a window of approximately 2 km2 that is classified as impervious (inner impervious surface), which serves as a spatial lag variable to control for an additional source of spatial autocorrelation that may emerge from the diffusion of behavior between neighboring units. The window size covers an admittedly arbitrary area, but one which was based both on best professional judgment of a typical developer's spatial frame of reference and on previous studies that have found window sizes of similar magnitude to capture spatial externalities (Fleming, Citation1999; Geoghegan et al., Citation1997; Irwin and Bockstael, Citation2002). Given the likely predominance of agglomeration effects associated with impervious surface in the immediate vicinity of the pixel, we hypothesize the sign of this variable to be positive.
The second metric complements the first, and is the percent of impervious surface in a region between the aforementioned window and a larger one with sides twice as large (outer impervious surface). The inclusion of this variable, which is non-overlapping with inner impervious surface, allows for varying parameters with increased distance from the pixel. Such variation may arise, for example, from spatial externalities associated with neighboring pixels. The effect of such externalities is expected to vary depending on the surrounding configuration of land uses, so that the sign of the variable is ultimately an empirical question. Evidence obtained from Irwin and Bockstael (Citation2002) suggests the net effect to be negative, a finding that they attribute to ‘repelling effects’ associated with low-density residential development. Later work from Carrión-Flores and Irwin (Citation2004), however, finds the proportion of neighboring residential and commercial land to have a positive effect on development, possibly reflecting agglomeration economies. It bears noting that these studies use parcel-level data (i.e. each observation being a tract of land under common ownership), allowing them to readily identify the effects of neighboring land parcels. We acknowledge that the absence of parcel boundary information here makes it difficult to distinguish true externalities associated with a neighboring parcel from spatial effects within the parcel itself. With respect to outer impervious surface, however, there are two reasons why any difference between a window-based calculation of imperviousness centered on the parcel and that on an undeveloped pixel within the parcel is likely to be negligible. First, the calculation covers a surface area that is a kilometer in width and a kilometer removed from the pixel on all sides, so that overlap with the parcel is difficult to conceive. Moreover, to the extent that the undeveloped pixels comprising the sample are within parcels that are themselves undeveloped, the amount of impervious surface used in the calculation of the metric will be primarily—if not exclusively—associated with neighboring parcels.
The remaining metric, based on the smaller window, is the percent of area classified as water (percent water). The effect of percent water cannot be hypothesized a priori: while developers are expected to covet increased water surface area as a residential amenity, this feature could also confer benefits to agricultural activities.
5.2 Remaining explanatory variables
In addition to the window-based metrics, two time-varying proximity-based metrics are also included in the specification. The first is the Euclidean distance to the nearest woodchip mill (distance to chipmill), which is a potentially important cost attribute of forestry operations (Prestemon et al., Citation2000). Between 1980 and 1998 the number of such mills in this region increased from 2 to 18, a trend that many perceive as hastening environmental degradation and biodiversity loss by facilitating the clear-cutting of non-industrial woodlots that is required to develop the underlying land (Schaberg et al., Citation2000). Whether the mills thereby increase the hazard of conversion or alternatively serve to maintain forested land in a rotation cycle, however, is a question left to the empirical results. The second proximity metric is the Euclidean distance to the nearest major road (distance to road), which is expected to have a negative effect on the conversion hazard given higher access costs.
Three time-invariant variables are included in the model that capture the effects of proximity to other landscape features. The first of these, distance to city, measures the Euclidean distance to the nearest city with a population of over 50,000 (i.e. Charlotte, Durham, Fayetteville, Greensboro, Raleigh, and Winston-Salem). As a measure of the influence of market proximity, an increase in this variable is expected to exert a negative effect on the conversion hazard. The second, near public lands, is binary and indicates whether public lands are nearby the pixel (within the outer window mentioned, above) (ESRI, Citation2000a, Citation2000b; NC Division of Parks and Recreation, Citation2003). The third proximity metric, distance to hazardous waste site, measures the Euclidean distance to the nearest hazardous waste site (NC CGIA, 1998). These latter two variables are hypothesized to have positive and negative coefficients, respectively, through their effects on the amenity value of the pixel.
Additional pixel-level variables are included in the model that also do not change with time, including elevation, slope, and dummy variables indicating forest cover (forest) or wetlands (wetland) (USGS, Citation1992). All of these variables are expected to have negative effects given higher conversion costs as well as higher opportunity costs associated with pixels under mature or ecologically important vegetation.
Varying by county and time interval, a returns-to-agriculture metric is in the model to capture the opportunity costs of commodity uses (agricultural returns). This metric, which is expected to negatively affect the conversion hazard, is calculated as county total farm receipts less costs, divided by farm acreage in the county (USDA, Citation1997b). This metric was associated with even forested pixels, pertaining as it does to the mix of uses to which a farmer may put their land, including forestry. Two additional time-varying indicators of county-level socioeconomic conditions included in the model are the deflated per capita income and population density (U.S. Department of Commerce, Citation2001). As proxies for increased demand for developed land, both variables are expected to increase the conversion hazard.
Finally, we include a set of county dummies representing the counties in the region that experienced at least one instance of conversion and a set of year dummies indicating the beginning of each interval. The former serve to limit omitted variable effects arising from county-level differences in governance, zoning, and other factors that may be fixed over time while the latter control for the effects of autonomous shifts in the policy and economic environment that occur over time in the region as a whole.
6 Results
presents results of two complementary log-log models of the determinants of the hazard of conversion. The second model (the unconstrained model) is distinguished from the first (the constrained model) by its inclusion of the window-based metrics. Although interpretation of the coefficient estimates from the complementary log-log model is complicated by the log-odds transformation of the dependent variable, we can readily calculate their ‘risk ratio.’ In the case of the continuous covariates, the risk ratio is interpreted as the percent change in the hazard rate from a unit increase in the covariate. These values are obtained by subtracting one from e β and multiplying the resulting value by 100. In the case of the dichotomous variables, the risk ratio is simply equal to e β, and can be interpreted as the ratio of the estimated hazard for observations with a value of one to the estimated hazard for those with a value of zero (Allison, Citation1995).
Table 2. Complementary log-log model of the hazard of conversion to impervious surface
Before discussing the parameter estimates of the two models, we revisit the issue of spatial autocorrelation and whether our sampling approach, combined with the window metrics we include in Model 2, assuages the concern. Using a modified Moran's I diagnostic developed for limited dependent variable models (Kelijian and Prucha, Citation2001), we report some success. Although we reject the null hypothesis of no spatial autocorrelation for Model 1, at 1.05 on the standard normal distribution, we cannot reject the null for Model 2.
Further evidence for the superiority of Model 2 is given by a likelihood ratio test of the null-restrictions imposed by Model 1 on the effects of the window-based metrics. The chi-square value of the test is 445 with four degrees of freedom, providing clear-cut evidence that the metrics significantly improve the fit of the model.
Turning to the coefficients of the window metrics, all are seen to be highly significant, with the inner ring variable having the strongest positive effect on the conversion hazard. Its magnitude, however, decreases with increases in impervious surface, as evidenced by the negative coefficient of the squared term. Increased water surface also has a positive but somewhat weaker effect, increasing the hazard by 3.9%. The only window metric having a negative effect is that measuring the outer band of impervious surface, pointing to the presence of varying parameters across adjacent bands surrounding the pixel. As noted above, this finding supports research by Irwin and Bockstael (Citation2002), who employ similarly constructed variables derived from parcel-level data in Maryland to test for spatial externalities. The negative coefficient in theirs and the present study suggests that existing development in the vicinity of an undeveloped pixel reduces the hazard of conversion, a likely reflection of preferences for open space. It bears noting that the replication of this result with the pixel level data used here hinges on the inclusion of the inner impervious surface metric. Excluding this variable was found—in an unreported model—to result in a positive and significant effect of outer impervious surface, highlighting the potential for spurious results when spatially lagged effects are unaccounted for.
Beyond improving the fit of the model, the inclusion of the window metrics produces several noteworthy discrepancies with respect to the significance and magnitude of the remaining covariates. The coefficient on elevation is significant but unexpectedly positive in Model 1, a counterintuitive result that is insignificant in Model 2. Likewise, per capita income and the dummy indicating proximity to public lands, both of which are significant and positive in Model 1, are insignificant at the 5% level in Model 2. Another discrepancy is seen with respect to the effect of distance to the nearest city. Model 1 suggests this variable to be a negative and highly significant determinant of the conversion hazard, an effect that fades away with the inclusion of the window metrics in Model 2. This result likely reflects a negative bias imparted on the effect of the distance measure in Model 1 resulting from the combined influence of the positive effect of inner impervious surface on the hazard of conversion together with the negative correlation between this variable and the variable distance to city (equal to –0.42). More plainly stated, our results may suggest that returns to economic activities bear increasingly little relation to the proximity to the urban core, implying, in turn, that a dominant landscape pattern is one characterized by sprawl. Taken together, these results suggest that controlling for the influence landscape pattern can have a substantial bearing on the conclusions drawn with respect to other features of the landscape, many of which have immediate relevance for policy planning.
The remaining statistically significant variables across the two models are largely in agreement: among the stronger effects are the distances to the nearest road and nearest hazardous waste site measures, both of which are significantly greater than zero for both models. The positive sign on the latter is counterintuitive at first glance, but may reflect, among other things, the potential for larger tracts to be at risk of development in less desirable neighborhoods because land is less expensive (Alonso, Citation1964). The variable measuring the return to agricultural land uses has the hypothesized negative effect on the hazard of conversion and is of roughly the same magnitude in both models. Likewise, the forest and wetlands dummy both have the expected negative coefficients. Based on the results from Model 2, forested pixels have roughly 51% of the hazard of non-forest pixels, with the corresponding magnitude for wetlands at 50%. While the coefficients of the 27 county dummies in the model are not shown in the table, using a chi-square test of their joint significance we reject the hypothesis at the 1% level that all of these coefficients are zero in both models. Finally, joint tests of the year dummies are also found to be statistically significant at the 1% level.
7 Validation and simulation
In light of our highly unbalanced sample, we first refer to Goodman and Kruskal's gamma (Goodman and Kruskal, Citation1954, Citation1959, Citation1963) as an indicator of the predictive performance of the two models. This is a non-parametric, symmetric metric based on the difference between concordant (C) and discordant (D) pairs of predicted and actual values of the dependent variable as a percentage of all pairs ignoring ties. Gamma is computed as (C – D)/(C + D), and can be interpreted as the contribution of the independent variables in reducing the errors of predicting the rank of the dependent variable. The value of gamma calculated from the constrained model is 0.85, while that of the unconstrained model is 0.90. The improvement in the predictive ability of the model with the inclusion of the window metrics is thus considerable, reducing the fraction of uncertainty remaining in the constrained model by a third.
Receiver-operating characteristic (ROC) curves are presented in and provide another diagnostic check on the performance of the two models. These are plots of the percentage of converted pixels correctly forecast (on the y-axis) against the percentage of non-converted incorrectly forecast (on the x-axis) for each possible prediction threshold. A key advantage of constructing ROC curves is that selecting an arbitrary threshold for designating whether a predicted probability generated by the model correctly predicts a changed pixel is not necessary. The area under the ROC curve, which ranges from zero to one and is non-parametric, can be interpreted as the proportion of correct forecasts across all possible thresholds. The closer the ROC curve is to the diagonal, the less useful is the model for discriminating between open space and converted pixels. Comparing the two curves, we see that that generated by Model 1 has an area of 0.91, while the area of the curve generated by Model 2 is slightly higher at 0.94. Moreover, a chi-square test that the areas are equal is, at 63.89, clearly rejected, providing further evidence for the superiority of Model 2.
Finally, we developed maps indicating the pattern of development between 1976 and 2001 (i.e. across all four intervals) that is observed and that is predicted by the unconstrained and constrained models. For this visual inspection, we again avoid selecting an arbitrary threshold probability to identify predicted conversions and instead depict a ‘surface’ of estimated conversion probabilities.
The surface is colored according to the relative magnitude of the estimated probability calculated from the parameter estimates of the econometric models in . Dark green indicates where conversion is predicted to be least likely and red where it's most likely. The tighter the correspondence between the high probability colors and the symbols indicating the 609 observed instances of pixel conversion in the sample, the more the estimated model is validated. The performance of the constrained model, seen in , is not impressive, even without the other one for comparison. The areas of high predicted probability are relatively few and many of the observed conversions lie outside them. The unconstrained model's map in depicts a generally superior picture with crisper transitions between areas of very high and very low probability. There is also greater correspondence between the high probability regions and the instances of actual conversion, including the more dispersed ones.
To illustrate the utility of our modeling approach in terms of policy simulation, we make a simple comparison between the unconstrained model and its depiction in —the baseline scenario—and a counterfactual scenario in which all counties are assumed to encourage the conversion process to a degree no greater than that of Durham County (whose effect, ceteris paribus, is relatively low). The simulation approach proceeds interval by interval and is recursive so that estimated probabilities in a given interval are not only influenced by this modification directly but also via its cumulative effect on the window-based metrics. Comparing the resulting map () to the baseline portrayed on , we see that applying Durham County's effect, which ought to reflect in part their particular approach to regional planning, to other counties results in considerably less conversion over the study area as a whole.
8 Conclusion
This paper began with a theoretical model in which the timing of conversion is determined by a comparison of the net discounted returns of the land in developed and commodity-based uses. Based on the theoretical framework, the paper then presented an application of a hazard model as a means of analyzing the effects of static and time-varying socioeconomic and agronomic variables on the conditional risk that land is converted to developed uses. By specifying the complementary log-log derivation of the proportional hazards model, we employed a methodology for modeling a continuous time process using discrete time satellite data.
The empirical analysis confirmed several results uncovered elsewhere in the literature, including significant impacts of proximity to roads, agricultural prices, and population density. As in the parcel-based analyses of Geoghegan et al. (Citation1997) and Irwin and Bockstael (Citation2002), we additionally find support for the hypothesis that spatial effects, as measured here by the landscape pattern metrics derived from the satellite imagery, are important determinants of the land conversion process. Not only did their inclusion significantly improve the fit of the model, but it also produced a tighter correspondence to the actual pattern of change, as evidenced by the gamma statistics, ROC curves, and a cartographic display of the predicted transitions.
These results are significant to a range of issues that have relevance for land use planning, particularly as regards the siting of roads, protected areas, hazardous waste facilities, and other landscape development decisions. Such interventions not only leave a direct ecological footprint, but also impact the future trajectory of change across adjacent land units. Reliably predicting the location, extent, timing and character of these changes is critical to ensuring that the planning process incorporates the human and environmental dimensions of the policy options under consideration. The techniques applied in this study can inform this process by providing both the statistical and spatial confirmation needed for gauging the impacts that are likely to emerge from changes in the variables over which policy makers have leverage.
There are a couple of obvious extensions for using the empirical model estimated in this paper to explore the issue of urbanization. One useful extension involves acquiring data that make finer distinctions among land-use classes and allow for a modeling approach that does so as well. A second stage in the form of a multinomial logit, for example, might be applied to those pixels that convert to examine why they are developed for a particular use (e.g. commercial or low-density residential) as opposed to another.
The simulation exercise we report is merely illustrative, and involved transferring the time invariant factors inherent to one county over to others. Although among these factors may be relative differences in approaches to managing land-use change, the degree to which this is the case cannot be discerned. Thus, a second extension is to enhance the ability to conduct policy simulations and forecasting. Additional satellite imagery would serve to reduce the interval sizes, rendering the model estimated with these data more amenable to policy analysis. With information on when and where land use policies were promulgated and programs implemented, effects could be assessed by adjusting the fixed effects to make room for dummy variables that are switched on over the relevant dates and across the relevant areas. Even without additional imagery, the model's flexibility in incorporating the effects of time on the hazard of conversion could be exploited to facilitate forecasting (Allison, Citation1995). Rather than relying on time dummies, a trend variable measuring the time elapsed since some starting date of interest could be included in the model, such as a change in zoning requirements or the imposition of smart growth programs. Such an approach would enable experimentation with different functional forms of the baseline hazard, including the inclusion of squared and higher-order trend terms to allow for nonlinearities in the hazard rate.
Acknowledgements
The authors wish to thank Manuel Frondel for his constructive comments on an earlier draft.
References
- Alig , R. and Healy , R. 1987 . Urban and built-up area changes in the United States: An empirical investigation of determinants . Land Economics , 63 : 215 – 226 .
- Alig , R. , Kline , J. and Lichtenstein , M. 2004 . Urbanization on the US landscape: looking ahead in the 21st century . Landscape and Urban Planning , 69 : 219 – 234 .
- Allison , P. D. 1995 . Survival Analysis Using the SAS System: A Practical Guide , 211 – 232 . Carey : SAS Institute Inc. .
- Alonso , W. 1964 . Location and Land Use: Toward a general theory of land rent , Cambridge, MA : Harvard University Press .
- Boscolo , M. , Kerr , S. , Pfaff , A. and Sanchez , A. What role for tropical forests in climate change mitigation? The case of Costa Rica. Paper prepared for the HIID/INCAE/BCIE Central America Project . 1998 .
- Brown , M. J. North Carolina's Forests, 1990. USDA Forest Service Technical Report, USFS, Asheville . 1993 .
- Capozza , D. R. and Helsley , R. W. 1989 . The fundamentals of land price and urban growth . Journal of Urban Economics , 26 : 295 – 306 .
- Carrión-Flores , C. and Irwin , E. G. 2004 . Determinants of residential land-use conversion and sprawl at the rural-urban fringe . American Journal of Agricultural Economics , 86 : 889 – 904 .
- Chomitz , K. and Gray , D. 1996 . Roads, land, markets and deforestation: a spatial model of land use in Belize . World Bank Economic Review , 10 : 487 – 512 .
- Cox , D. R. 1972 . Regression models and life tables . Journal of the Royal Statistical Society , B34 : 187 – 220 .
- Cropper , M. , Puri , J. and Griffiths , C. 2001 . Predicting the location of deforestation . Land Economics , 77 : 172 – 186 .
- Environmental Systems Research Institute, Inc. (ESRI) . U.S. National Atlas Federal and Indian Land Areas, ESRI Data & Maps 2000 (CD 2) . 2000a .
- Environmental Systems Research Institute, Inc. (ESRI) . U.S. GDT Park Landmarks, ESRI Data & Maps 2000 (CD 3) . 2000b .
- Environmental Systems Research Institute, Inc. (ESRI), 2000c, Census 2000 TIGER/Line Data. Available online at: http://www.esri.com/data/download/census2000_tigerline/
- Ewing, R., Pendall, R. and Chen, D., 2003, Measuring sprawl and its impact. Available online at: http://www.smartgrowthamerica.org/sprawlindex/MeasuringSprawl.PDF
- Fang , S. , Gertner , G. , Sun , Z. and Anderson , A. 2005 . The impact of interactions in spatial simulation of the dynamics of urban sprawl . Landscape and Urban Planning , 73 : 294 – 306 .
- Fleming , M. 1999 . Growth controls and fragmented suburban development: the effect on land values . Geographic Information Sciences , 5 : 154 – 162 .
- Fulton, W., Pendall, R., Nguyen, M., and Harrison, A., 2001, Who sprawls most? How growth patterns differ across the U.S. Center on Urban & Metropolitan Policy, The Brookings Institution, Washington D.C. Available online at: http://www.brook.edu/es/urban/publications/fulton.pdf
- Geoghegan , J. , Wainger , L. A. and Bockstael , N. E. 1997 . Spatial landscape indices in a hedonic framework: an ecological economics analysis using GIS . Ecological Economics , 23 : 251 – 264 .
- Goodman , L. and Kruskal , W. H. 1954 . Measures for association for cross-classification I . Journal of the American Statistical Association , 49 : 732 – 764 .
- Goodman , L. and Kruskal , W. H. 1959 . Measures for association for cross-classification II . Journal of the American Statistical Association , 54 : 123 – 163 .
- Goodman , L. and Kruskal , W. H. 1963 . Measures for association for cross-classification III . Journal of the American Statistical Association , 58 : 310 – 364 .
- Healy , R. G. 1985 . Population growth in the U.S. South: implications for agricultural and forestry land supply . Rural Development Perspectives , 2 : 27 – 30 .
- Hite , D. , Sohngen , B. and Templeton , J. 2003 . Zoning, development timing, and agricultural land use at the suburban fringe: a competing risks approach . Agricultural and Resource Economics Review , April : 145 – 157 .
- Irwin , E. G. and Bockstael , N. E. 2001 . The problem of identifying land use spillovers: measuring the effects of open space on residential property values . American Journal of Agricultural Economics , 83 : 699 – 705 .
- Irwin , E. G. and Bockstael , N. E. 2002 . Interacting agents, spatial externalities, and the endogenous evolution of residential land use pattern . Journal of Economic Geography , 2 : 31 – 54 .
- Irwin , E. G. , Bell , K. P. and Geoghegan , J. 2003 . Modeling and managing urban growth at the rural-urban fringe: a parcel level model of residential land use change . Agricultural and Resource Economics Review , April : 83 – 102 .
- Jargowsky, P. A. 2001, Sprawl, concentration of poverty, and urban inequality. In Urban Sprawl: Causes, Consequences & Policy Responses , G. Squires (Ed). (Washington D.C.: Urban Institute Press). Available online at http://urbanpolicy.berkeley.edu/pdf/census2000/jargowsky.pdf
- Kelejian , H. H. and Prucha , I. R. 2001 . On the asymptotic distribution of the Moran I test statistic with applications . Journal of Econometrics , 104 : 219 – 257 .
- Kline , J. D. , Moses , A. and Alig , R. J. 2001 . Integrating urbanization into landscape-level ecological assessments . Ecosystems , 4 : 3 – 18 .
- Kunstler , J. H. 1994 . The Geography of Nowhere: The Rise and Decline of America's Man-Made Landscape , New York : Touchstone Books .
- Lilly, J. P. 1998, North Carolina Agricultural History. North Carolina Department of Agricultural and Consumer Services. Available online at: http://www.ncagr.com/stats/history/history.htm
- Nelson , G. and Hellerstein , D. 1997 . Do roads cause deforestation? Using satellite images in econometric analysis of land use . American Journal of Agricultural Economics , 79 : 80 – 88 .
- North Carolina Division of Parks & Recreation, Resource Management Program, 2003, Available online at: http://www.ncsparks.net
- North Carolina Center for Geographic Information and Analysis, 1998, Available online at: http://cgia.cgia.state.nc.us/cgdb/catalog.html
- Pfaff , A. 1999 . What drives deforestation in the Brazilian Amazon? Evidence from satellite and socioeconomic data . Journal of Environmental Economics and Management , 37 : 26 – 43 .
- Powell , D. S. , Taulkner , J. L. , Darr , D. R. , Zhu , Z. and MacCleery , D. Forest Resources of the United States. USDA Forest Service . Gen. Technical Report RM-234 . 1992 .
- Prestemon , J. P. and Abt , R. C. 2002 . “ Timber products supply and demand ” . In The Southern Forest Resource Assessment Edited by: Wear , D. N. and Greis , J. G. 299 – 325 . Asheville USDA Forest Service, General Technical Report SRS-53
- Prestemon, J., Pye, J., Butry, D. and Stratton, D., 2000, Economic Research Unit, USDA Forest Service. ‘Locations of Southern Wood Chip Mills for 2000’. Available online at: http://www.srs.fs.usda.gov/econ/data/mills/chip2000.htm
- Schaberg , R. , Cubbage , F. W. and Richter , D. D. Trends in North Carolina timber product outputs, and the prevalence of wood chip mills. Paper prepared for the study on: Economic and Ecological Impacts Associated with Wood Chip Production in North Carolina . 2000 .
- Sierra Club, 1998, Sierra Club Sprawl Report: 30 Most Sprawl-Threatened Cities. Available online at: http://www.sierraclub.org/sprawl/report98/raleigh.asp
- Srinivasan , S. 2005 . Linking land use and transportation in a rapidly urbanizing context: a study in Delhi, India . Transportation , 32 : 87 – 104 .
- Stear , T. E. Population Distribution, in: North Carolina's Changing Population. University of North Carolina, Carolina Population Center . 1973 .
- Turner , M. G. , Wear , D. N. and Flamm , R. O. 1996 . Land ownership and land-cover change in the Southern Appalachian Highlands and the Olympic Peninsula . Ecological Applications , 6 : 1150 – 1172 .
- U.S. Department of Commerce, 2001, Local Area Personal Income Tables. Available online at: http://www.bea.gov/regional/reis/
- U.S. Department of Agriculture . National Resources Inventory . 1997a .
- U.S. Department of Agriculture . Census of Agriculture, 1997 . 1997b .
- USGS, 1992, National Land Cover Data 1992. Available online at: http://edc.usgs.gov/products/landcover/nlcd.html
- Vance , C. and Iovanna , R. 2005 . Analyzing spatial hierarchies in remotely sensed data: insights from a multilevel model of tropical deforestation . Land Use Policy , 23 : 226 – 236 .
- Wear, D. N. and Greis, J. G., 2002, The Southern Forest Resource Assessment Summary Report. USDA Forest Service Southern Research Station. Available online at: http://www.srs.fs.usda.gov/sustain/report/
Appendix
Elaboration of data sources
The satellite images are taken from the northern half of path 16, row 36 and the southern half of path 16, row 35 of the Landsat satellite orbit. Data for the years 1976 and 1980 were derived from the MSS imaging system, while the TM imaging system was the data source for the years 1986, 1993, and 2001. Because TM and MSS data have different spatial resolutions—58×79 m for MSS and 30×30 m for TM—the data was spatially degraded to a 60×60 m resolution using nearest-neighbor resampling.
The distance to the nearest chipmill was obtained by overlaying a GIS layer of woodchip mill locations and their establishment dates that is available from Prestemon et al. (Citation2000) of the Economic Research Unit of the USDA's Forest Service.
The measure of distance to the nearest road is based primarily on the road network available from ESRI (Citation2000c), which includes interstate, U.S., and state highways, as well as other major streets and thoroughfares. This measure was modified using image interpretation of Landsat data to reflect the conditions existing at the beginning of each interval.
The measures of elevation, slope and the forest dummy were derived directly from the satellite imagery. The wetland category was derived from the 1992 land use and land cover data from the EROS Data Center of the USGS. Data on the location of public lands were derived from shapefiles produced by ESRI (Citation2000a, Citation2000b) and the North Carolina Department of Parks and Recreation (Citation2003). The hazardous waste site data were obtained from the North Carolina Center for Geographic Information and Analysis (Citation1998).
Data on population density, per capita income, and agricultural returns were obtained from the U.S. Department of Commerce (Citation2001) and USDA (Citation1997b). These county-level and time-varying data were linearly interpolated for years in which published data and the satellite imagery did not correspond.
The data set and code used to estimate the models, available either in Stata or SAS format, can be obtained from the authors upon request.