Abstract
Harmonisation of land-cover data relates to spatial data integration and therefore needs to consider the data concepts adopted and the spatial, temporal, semantic and quality aspects of the data. Differences in semantic concepts are often considered the key obstacle to data integration and interoperability. If the problem of harmonisation is limited to the variation in the semantic content of data expressed as differences in categorisation, then various approaches have been developed to address the methodological issues and for computing semantic similarity. Five Nordic class sets were selected for establishing correspondences between their semantic class contents using the parameterised land cover classification system (LCCS) as a reference system. Subsequently, semantic similarities between pair-wise classes were calculated using a module of LCCS. This article first examines the aspects of land-cover harmonisation and the LCCS methodologies for categorisation and semantic similarity. It then discusses the functioning of LCCS as a reference system in which the more or less corresponding class of the original Nordic classes was determined and the semantic similarity indices computed. Suggestions are provided for improvements in the LCCS methodology, both in establishing correspondences and for computing semantic similarity. Recommendations are given for the way forward in land-cover harmonisation and for measures to express the quality of harmonisation of the semantic contents of class sets at class set level and individual class level.
Introduction
Land cover is defined as ‘the observed (bio)physical cover on the Earth's surface’ (Di Gregorio and Jansen Citation2000) and is widely perceived as an important component of environmental and ecological systems, central to understanding global environmental change (Meyer and Turner Citation1994; Turner et al. Citation1995; Walker, Steffen, Canadell and Ingram Citation1997; Lambin, Rounsevell and Geist Citation2000). Spatial variability is a fundamental quality of land-cover data with important implications for environmental and ecological modelling and analysis (Ahlqvist and Shortridge Citation2006). Environmental models become increasingly more sophisticated and with that the importance of accurate, meaningful and current data on land use and land cover to support these models increases (DeFries and Belward Citation2000). Many geographic entities have undertaken surveys of land cover; often these have been made on the basis of a particular categorisation system (often termed classification system) or class set (usually termed classification, nomenclature or legend). A common problem is, however, that as knowledge advances over time, technology develops and policy objectives change, each survey with a class set designed for its purpose, rather than being part of a sequence, creates a new baseline data set. Whereas in the past, survey maps were illustrations that accompanied a descriptive memoir; nowadays, in the era of geoinformatics, maps are understood as primary data sets (Fisher Citation2003). This poses a further problem regarding the associated class labels that are often rather cryptic and unrelated to any categorisation system where the user may learn the concepts and criteria behind the class labels (Comber, Fisher and Wadsworth Citation2005; Wadsworth, Comber and Fisher Citation2006). Differences in the naming of classes, changes in class definition and addition or removal of classes in data sets covering the same area in different periods create difficulties in the separation of actual changes over time from apparent changes in category definitions.
In practice, results from different surveys do need to be harmonised over time and space (e.g. in relation to trans-boundary issues), and reference to existing information is often required to verify new results (e.g. regarding urban sprawl and landscape changes). Data harmonisation, being defined as ‘the intercomparison of data collected or organised using different classifications dealing with the same subject matter’ (McConnell and Moran Citation2001), thus becomes a prerequisite for many data analyses. Harmonisation will allow countries and institutions to continue to use established methods and data sets made with certain financial and intellectual investments (UNEP/FAO Citation1994; Wyatt and Gerard Citation2001).
Development of the general-purpose land cover classification system (LCCS) (Di Gregorio and Jansen Citation2000) has led to the common belief that once such a categorisation system becomes widely adopted for new surveys, the problem of data harmonisation would be overcome because new data sets would be collected using a single standard system allowing direct comparison of new class sets, whereas existing class sets could be ‘translated’ into the adopted system making possible direct class comparison with new class sets. However, this stance that is geared towards data standardisation, defined as ‘the use of a single standard basis for classification of a specific subject’ (McConnell and Moran Citation2001), assumes falsely that the continuous advances in knowledge, technological developments and/or changing policy objectives will not have any impact on a categorisation framework or its application. With each data collection effort, lessons are learnt that leave their imprint on successive efforts [e.g. co-ordination of information on the European environment (CORINE) land cover 1990 vs. 2000 (Büttner et al. Citation2004)]. Data standardisation may thus be an unrealistic expectation and only partly feasible with the need for data harmonisation always present.
With the emphasis shifting from static land-cover mapping towards more dynamic environmental monitoring and modelling (Lambin et al. Citation2000; McConnell and Moran Citation2001; Dolman, Verhagen and Rovers Citation2003), it is necessary to examine how far research has progressed in data harmonisation methodologies. In this article, first the various aspects of land-cover harmonisation are examined with particular emphasis on semantic contents of classes and semantic similarity (Sections 2–4). This is followed by the examination of the methodology of a particular tool, the LCSS (Section 5) and the experiences with this tool by the Nordic landscape monitoring (NordLaM) project of the Nordic Council of Ministers. LCCS was used as a reference system for establishing correspondences between semantic contents of classes from five Nordic class sets and for computing semantic similarity between those classes (Section 6). While the harmonisation results of the Nordic class sets will be of interest to essentially a Nordic audience, the methodological issues being addressed are relevant to the wider context of spatial data integration, interoperability, land-cover harmonisation and standardisation. Suggestions are provided to improve the methodologies implemented in LCCS (Section 7), and recommendations are provided for measures that would quantify correspondence results as well as discussing some general research questions that are still open (Section 8). Taking stock of land-cover harmonisation and semantic similarity methodologies is especially required in the context of spatial data infrastructure (SDI) initiatives that change data access [e.g. the European Commission's infrastructure for spatial information in Europe (INSPIRE) initiative (INSPIRE Environmental Thematic Coordination Group Citation2002)] and in the context of the UN that promotes the use of modern information technologies in developing countries (e.g. UNCED Agenda 21 and the World Summit on Sustainable Development).
The aspects of harmonisation
Land-cover harmonisation touches the issue of spatial data integration when it concerns spatially explicit data. The recognition that spatial data integration is an essential step in environmental change modelling and initiatives (e.g. planning and decision making) that aim to respond to environmental change is broadening (Comber et al. Citation2005). Increasingly, the need is recognised for a deeper understanding of the wider meaning of data stored in geodatabases (Ahlqvist Citation2004). The latter is particularly important in the context of data interoperability, i.e. the exchange of meaningful information between multiple information sources (Vckovski Citation1999).
In the definition of McConnell and Moran (Citation2001) given above, two distinct levels of harmonisation should be identified:
-
the intercomparison of classes belonging to different categorisation systems and
-
the intercomparison of the data collected with the use of these categorisation systems.
The first level deals with how classes are defined and named, whereas the second level deals with how data were collected and represented (e.g. methods, scale, time, coordinates). In land-cover harmonisation, therefore, the following inter-related aspects have been considered without exception ():
-
the adopted data concepts (level 1 and 2);
-
the spatial aspects (level 2);
-
the temporal aspects (level 2);
-
the semantic aspects (level 1) and
-
the quality aspects (level 1 and 2).
These different aspects of land-cover harmonisation are discussed in more detail below.
Adopted data concepts
Comparison of data sets should include comparison of the Weltanschauung, or worldview, embodied in the data (Comber, Fisher and Wadsworth Citation2004). Differences in the way that land cover may be conceptualised are not addressed by the stated objectives of SDIs or by current metadata or data quality reporting paradigms. Only very few of the available categorisation systems explicitly mention the concept for description of classes – for example, the LCCS states that it is based on a structural–physiognomic approach (Jansen and Di Gregorio Citation2002). Statements that any categorisation system will allow a ‘neutral’ description of land cover ignore the fact that human beings always look at the object land cover in a specific way (e.g. an economist will look at it differently compared to an agronomist or an ecologist). Furthermore, data are collected for an intended purpose, and this leads to a particular or prevalent view. Related to the view with which data are collected is the meaning of the data. The latter may be obvious to the data producer, but it is rarely as clear to the data users unless they were part of the data collection process. Access to data through SDI initiatives implies that countless potential users may be reached. However, in almost all cases to date, the metadata do not provoke users to consider the wider meaning of the data (Comber et al. Citation2004). Land-cover data collected in the context of a forest inventory will focus on description of different parameters than land-cover data collected for surveillance and monitoring of habitats although these data may have some parameters in common. In addition, the purpose for which data are collected may relate to a design with higher thematic and spatial accuracies for certain classes than for others. The lower accuracies may be insufficient for some data users but the metadata do not provide such information. Worse still, some data sets have been collected without proper validation [e.g. the FAO Africover data, some country data sets for CORINE (1990)]. Any data collection without proper validation remains an untested hypothesis (Strahler et al. Citation2006).
Spatial aspects
The International Organization for Standards Technical Committee 211 (ISO TC/211) (www.iso.org) and the open geodata interoperability specification consortium (www.opengis.org) are two sources that have developed numerous standards concerning the spatial aspects of data. The spatial or geometric aspects of the data set comprise the description of the form of the entities through geographic primitives or through a structured geometry (e.g. topology). In general, the spatial aspect considers cases of different representations of the same object. For instance, a road network can be represented by polygons of the road surface, as a network of road axes and nodes, or as an information level where the road is represented by the sequence of borders such as walls and façades of buildings (e.g. cadastre). Each way of representing a road network follows a different set of conceptual and technical practices.
The spatial component describes the dimensions of the geometry (i.e. two dimensions for areas, one dimension for lines and no dimension for points) in relation to the scale and projection in a geodetic reference system. Differences in scale can be overcome by geometric generalisation, but this may imply loss of information; generalisation means also reorganisation of the semantic attributes (see Section 2.4). For instance, elaborating the example used above, one could imagine a matching of road maps of two neighbouring countries. In country A, the roads are depicted at scale 1:5000 by polygons projected in a local coordinate system, whereas in country B, the roads are depicted at scale 1:100,000 by lines projected in UTM WGS84. Data harmonisation in such a case should consider three spatial aspects: (1) how to depict the roads (e.g. as polygons or lines), (2) the scale to be adopted and (3) the geodetic reference system to be used. Harmonisation of data sets that are represented by either polygons or grid cells (raster), such as many thematic data sets, do not represent significant problems of geometric harmonisation because usually these can be restructured using topological procedures.
Temporal aspects
It is necessary to consider the temporal aspects of data sets because certain themes undergo more changes with time than others, and data harmonisation between class sets covering the same subject matter but from different periods may not be meaningful. For example, the first CORINE land-cover data set, CLC1990, spans the period 1986–1998 and the second data set, CLC2000, the year 2000 to ±1 year (Büttner et al. Citation2004). Harmonisation of the temporal aspects should provide a baseline period or year. For example, one may well question whether harmonisation of land-cover data from country A from the year 1995 with those of country B from the year 2007 is at all meaningful, because in the period represented, changes are also likely to have occurred in country A and, as mentioned previously, knowledge and technology have advanced and policy objectives will also have changed.
Semantic aspects
As different applications have different worldviews and semantics, interoperability is primarily understood as a semantic modelling problem (Bishr, Pundt, Kuhn and Radwan Citation1999). The variation in the semantic content of data expressed as differences in categorisation has received limited attention until recently (Feng and Flewelling Citation2004). Comber et al. (Citation2004) report that differences in semantic concepts are often the major barrier to data integration. Achieving semantic interoperability to use existing data sets at a satisfactory level has therefore become a key issue.
Describing land cover is to account for its character, and here different concepts may co-exist in a single class or single categorisation system (EC Citation2001):
-
Two-state character, i.e. present/absent, 1/0, positive/negative, etc. [e.g. in LCCS, the dichotomous phase uses the two-state character for primarily vegetated/primarily non-vegetated, terrestrial/aquatic and artificial/(semi-) natural land covers] and
-
Multi-state character that can be subdivided into quantitative and qualitative, or the so called terminological variables. Two different types of quantitative variables can be distinguished: discrete (e.g. in LCCS in the modular-hierarchical phase vegetation can be described using growth form, leaf type and leaf longevity) and continuous variables (e.g. continuous fields that allow a more precise description of vegetation gradients and mixtures).
Harmonisation between classes that represent a mixture of these characters will be difficult, as will harmonisation between classes that have a two-state character but represent a mixture of quantitative and qualitative variables.
Class descriptions contribute to the definition of boundary conditions that should be applied unequivocally and consistently when establishing correspondence between class sets to avoid errors in data interpretation. The level of certainty with which such class correspondence is established is highest when the same parameters have been applied; a difference in the applied parameters, and thus in boundary conditions, results in a lower certainty level.
It may be necessary to ‘translate’ a class set into a third system, a so-called reference system that functions like a bridge between two class sets: each class in the original class sets will find its more or less corresponding class in the reference system. The use of a reference system may be a sensible choice when many class sets are involved as the number of pair-wise class combinations becomes excessive with comparison of n class sets requiring n(n – 1)/2 comparisons to be made. As Wyatt and Gerard (Citation2001) point out, the use of a reference system requires a single ‘translation’ from each original class set into the reference system and obviates the need for pair-wise class comparisons between every class set of interest.
Quality aspects
The quality aspects concern all the above-mentioned aspects to produce quality land-cover harmonisation results. Harmonisation requires the analysis of data quality because correspondence between data sets having very different levels of quality may not be meaningful (Jansen Citation2006). Often the metadata of a land-cover data set provide information concerning the positional and thematic accuracy. However, there are many other measures of quality and uncertainty that should be specified as they may limit interoperability but that currently are excluded from metadata requirements. One such measure is discussed in the next section.
Methodologies for assessing semantic similarity
One step towards achieving semantic interoperability is to measure the degree of semantic similarity between categorisations. Various practical solutions for overcoming semantic differences have been proposed:
-
use of a standard set of parameters to overcome semantic divergences in categorisation systems (Wyatt et al. Citation1994; Wyatt and Gerard Citation2001; Jansen and Di Gregorio Citation2002);
-
Bayesian probabilities based on a variety of metrics of geometric and semantic similarity to identify areas of change (Jones, Ware and Miller Citation1999);
-
use of a similarity function to determine semantic neighbourhoods and distinguishing features (Rodríguez, Egenhofer and Rugg Citation1999; Rodríguez and Egenhofer Citation2003);
-
uncertain conceptual spaces to represent uncertainty between spatially coincident but semantically divergent data (Ahlqvist, Keukelaar and Oukbir Citation2000; Ahlqvist Citation2004, Citation2005a) or between spatially and semantically divergent data using the parameterised LCCS as a reference system to mediate between two class sets (Ahlqvist Citation2005b);
-
the mathematical theory of concept lattices to link semantics from different data ontologiesFootnote 1 and reveal interrelationships between categories (Kavouras and Kokla Citation2002);
-
use of similarity indices to describe the extent to which descriptions of classes match (Jansen, Mahamadou and Sarfatti Citation2003; Feng and Flewelling Citation2004);
-
semantic statistical approaches using expert knowledge to reconcile the uncertainty between different ontologies using expert descriptions of semantic relations (Comber et al. Citation2004, Citation2005; Wadsworth et al. Citation2006);
-
use of a fuzzy logic framework and expert knowledge to reconcile inconsistent land-cover data (Fritz and See Citation2005);
-
semantic variograms based on semantic similarity metrics to measure spatial variability of categorical data (Ahlqvist and Shortridge Citation2006) and
-
application of weighting by the semantic distance calculated from the four most discriminant LCCS parameters to the confusion matrix in a validation scheme (Mayaux et al. Citation2006).
Mainly the computer and information sciences have developed ways of computing semantic similarity that provide a quantitative measure to the user as to which categories are more similar and which categories are more dissimilar (semantically distant). Measuring semantic similarity of categories, either before or after data collection or between existing data sets, is an emerging area of research. The exploration of category relationships within a categorisation system can reveal how well classes are separated or whether there is a risk of confusion between classes, a situation that may be problematic from a data accuracy perspective (Ahlqvist Citation2005a,Citationc). Semantic similarity addresses the issue of accuracy also in another way: if complete correspondence between classes from different class and data sets is not always possible, then how accurate is class correspondence and how accurate are data harmonisation results? To date, research has not led to any widely accepted methodologies for land-cover harmonisation or to an accepted means for quantifying the quality of harmonisation results.
The points discussed above make it clear that land-cover harmonisation is a multi-faceted issue that concerns both geoinformatics, and statistical and subject matter specialists. A solution offered by any of these without involvement of the others will probably fall short in addressing the complexity of the problem. Efforts that are limited to a crosswalk ‘translation’ effort between categorisation systems and/or class sets ignore such complexity. For example, overviews in which FAO shows that country land-cover maps are ‘translated’ into LCCS (e.g. as shown by Herold, Latham, Di Gregorio and Schmullius Citation2006b) offer the wrong impression of data harmonisation as such efforts have been limited to correspondence of original classes (legends) with LCCS rather than having examined the full meaning of the data.
Semantic differences that affect the interoperability of land-cover and land-use data
One specific issue that affects the interoperability of land-cover data sets is that land-cover class sets often contain land-use elements. Thus, harmonisation of land cover may in different cases imply either a need to make harmonisation of land-use categories or the decision to leave the land-use elements out of the established correspondences, in which case part of the data richness is lost. Though land cover and land use are related, they are not the same (Jansen and Di Gregorio Citation2002). Nowadays, it is advocated to separate the two, but in the practice of much survey work, this is frequently not the case for various justifiable reasons often related to the intended purpose for which data are collected.
According to Brown and Duh (Citation2004), land cover and land use have three major semantic differences that affect their interoperability:
-
The category definitions of land cover and land use are different. Land cover describes what you see on the surface of the earth, whereas land use may relate to an intended purpose that is not necessarily directly observable. For example, ‘undeveloped forest’ is a clear-cut area that continues to be used for forestry (Lund Citation1999).
-
Land cover and land use have different geometric expressions; consequently, a classification crosswalk approach to semantic interoperability that defines interrelations between categorisation systems or class sets without redefining spatial objects, as has been applied for alternative vegetation/land-cover class sets by the International Geosphere Biosphere Programme (IGBP) (Loveland et al. Citation2000), may be an inadequate solution for translation between land use and land cover (i.e. the spatial objects might need to change in addition to the class definitions).
-
Land cover and land use have different spatial rules to assign attributes to features because land-use class definitions tend to integrate information about activities taking place within a spatial unit (e.g. cadastral parcel or zone), whereas land-cover class definitions assess the static and in situ conditions. Thus, the entities of a land-cover data set (e.g. polygons) usually show more spatial variation than those of a spatially explicit land-use data set (assuming both data sets are compiled based on sources of the same level of detail).
Cihlar and Jansen (Citation2001) pointed out that the complex relationships between land cover and land use should be considered from a spatial and thematically consistency viewpoint: in one-to-one and one-to-many land-cover/land-use relationships, the relationship is thematically and spatially unique, whereas in many-to-one land-cover/land-use relationships, either the relationship is not thematically unique but spatially consistent throughout the domain of interest or the relationship is not thematically unique and not spatially consistent throughout the domain of interest. In addition, these relationships may change over time in the domain of interest, as well as vary between different domains of interest.
Methodological issues: the case of LCCS
The objective of the parameterised approach of the LCCS, developed by FAO and UNEP, was to have a consistent and pragmatic methodology for land-cover description in several countries representing different types of environments (Di Gregorio and Jansen Citation2000). Subsequently, the methodology and its software application have been endorsed by the land use and cover change (LUCC) core project of the IGBP and international human dimensions programme on environmental change (IHDP) (McConnell and Moran Citation2001). More recently, FAO's attention has shifted from land-cover mapping to land-cover harmonisation. LCCS is, as a basis of a harmonisation strategy, recommended by the global observations of forest cover–global observation of land dynamics (GOFC–GOLD) and the global terrestrial observing system (GTOS) (Herold et al. Citation2006b). As the LCCS categorisation methodology was never critically reviewed in the scientific literature, it now seems a timely moment to do so, taking into account also the more recent methodological developments discussed above. The focus in this article is on (1) methodological issues in the categorisation that might have repercussions on class comparisons when used as a reference system and (2) on the semantic similarity methodology.
Categorisation issues in the LCCS methodology
The main documents available that describe the LCCS (Di Gregorio and Jansen Citation2000 updated in FAO Citation2005) lack a formal definition of the categorisation rules. This represents a problem because, as the software source is not open, there is no possibility to (easily) understand the behaviour of the software application. The underlying logic can only be derived experimentally by using the software intensively and by defining classes step-by-step with the software to know whether they are correct. Furthermore, identical Boolean class codes are used in LCCS for dissimilar parameters (e.g. in each main land-cover category the first parameter is coded ‘A’ followed by a number, thus ‘A1’ occurs eight times) though numerical class codes are unique. This means that researchers cannot refer to a comprehensive model that would allow them to make comparisons with other categorisation systems to evaluate LCCS. It also means that it is not possible to propose modifications as the formal definition of classes is missing, and thus it is impossible to adequately describe LCCS (Di Costanzo and Ongaro Citation2004). All this has far-reaching consequences for the use of LCCS as a reference system with existing class sets because it implicitly requires adoption of a parameterised approach of which, for the user, the underlying rationale is mainly a ‘black box’.
A parameterised approach is used to define classes organised hierarchically in a tree-like structure. The hierarchical order of parameters is justified in terms of the ease with which the orders are observed, but it would be more correct to speak of a hierarchical tree-like structure with more inclusive and abstract concepts at the top and more detailed concepts further down the hierarchy, a structure that can be modelled with set inclusion (‘is a’) relations (Feng and Flewelling Citation2004; Ahlqvist Citation2004, Citation2005b). The ‘is a’ relation captures superordinate–subordinate relations between two categories.
To shed some light on the ‘black box’, one could formulate, using simple terms, categorisation in the LCCS Classification Module (LCCS-CM) of category A as follows:
The first land-cover parameter, or first and second for the (semi-) natural vegetation categories, is an obligatory element to define a class (). But since codes are not exclusively assigned to a specific parameter, one needs to know to which of the set of eight main land-cover categories the summation of parameters, with or without modifiers, belongs. Thus, one needs in addition the establishment of the land-cover category in this set to understand the meaning of the codes:
Figure 2. Overview of the eight major land-cover categories of LCCS with their parameters grouped under the primarily vegetated and primarily non-vegetated area distinction.
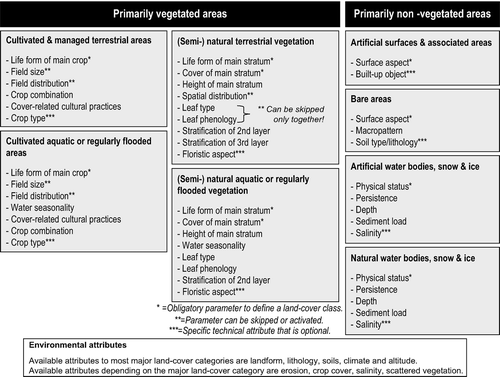
At first sight, LCCS-CM may appear to be a so-called crisp categorisation system with mutually exclusive parameter options, but this is not always true. provides an example for the parameter ‘crown cover’ Footnote 2 used in the primarily vegetated area land-cover categories. The grey areas in the figure indicate threshold values for definition that are formed by a range, and at these percentages, the crown cover can be either sparse or open (10–20%), or open or closed (60–70%). Two-parameter options added in the second version of LCCS-CM introduce inconsistencies in the adopted concept: (1) ‘closed-to-open’ defined as ‘between 100 and 15%’ (FAO Citation2005) does not correspond to the options closed plus open as the range 10–15% is missing and (2) ‘closed-to-open’ defined as ‘between 100 and 40%’ uses the value 40% that only exists as a threshold value for modifier options. Both parameter options ignore the range 60–70% as a threshold value. For the ‘crown cover’ definition and other similar parameter definitions, a fuzzy representation would be more suitable, as suggested by Ahlqvist (Citation2005b).
Figure 3. Overlapping definitions of crown cover parameters and modifiers in LCCS. The definitions of ‘closed-to-open’ have been added in the second version of LCCS using the threshold value of 15% where in other definitions the range 10–20% is given. For consistency's sake the latter has been used.
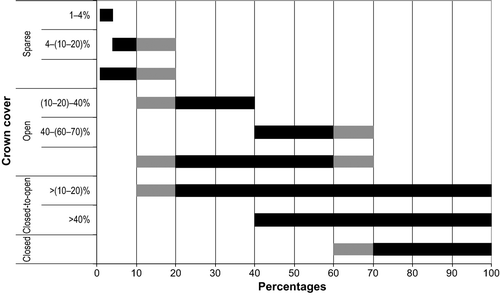
The basic principle adopted in LCCS ‘that a given land-cover class is defined by the combination of a set of independent diagnostic attributes’ (FAO Citation2005, p. 12) may be true in most cases but is clearly not in all, as can be demonstrated by the use of the parameters ‘life form’ (growth form) and ‘height’ as they are interlinked in the definitions (see also Section 5.2). These interlinks determine also which options are valid for the parameter ‘stratification’ (vegetation layering) further down the hierarchy.
Thus, the LCCS-CM methodology is not so clear-cut as appears to be the case when reading the available documentation. There are quite a number of exceptions to the categorisation rules (e.g. to give some examples from the LCCS glossary: herbaceous bamboos can be considered ‘woody’ under (semi-) natural vegetation or a succulent plant such as pineapple can be considered ‘shrub’ under cultivated and managed areas) and restrictions (e.g. vegetation layering cannot describe more than three layers whereas in tropical areas more vegetation layers may occur) that limit the potential multitude of classes. These exceptions and limitations may be the result of the adopted concepts and common sense, but they make LCCS less easily understandable and less easy in its application despite its software.
Semantic similarity issues in the LCCS methodology
When using LCCS as a reference system, the step-by-step definition of a class, or category, described above is also the first step in the ‘translation’ procedure. The ‘translation’, i.e. to find for each class in the original class set its more or less corresponding class in LCCS-CM, begins actually by defining rather than finding the corresponding class in the LCCS-CM. illustrates the parameterisation procedure using a class of the Danish area information system (AIS) as an example. This step introduces uncertainty as defining the corresponding class may differ from person to person. After definition of a class in LCCS-CM, followed by storage in the LCCS legend module that is not further discussed here, import of all classes into the LCCS translator module (LCCS-TM) can take place. The name translator module is misleading, as one does not actually ‘translate’ in the LCCS-TM but one compares and the term ‘translator’ does not refer to an automated thesaurus or text mining procedure for words used in the category names and definitions. In this module, computation of the semantic similarity between the corresponding classes in LCCS terminology can be executed, this is called ‘similarity assessment’. Similarity provides a quantified measure stating how much of a definition is included in another definition, and this occurs between parameter options or a group of parameter options in the case of a class (e.g. how much of the definition of parameter option ‘graminoids’ is found in ‘herbaceous’ or how much of the definition of a deciduous forest is found in an evergreen forest). Also in this case, the LCCS software application is a ‘black box’ because the methodology is not explained in the manual (both versions).
For the calculation of semantic similarity between classes within LCCS-TM, there are various issues influencing the computation. Each parameter used in the definition of the class has the same weight. Weighting is not implemented as the relative importance of the individual parameters in LCCS-CM is linked to their hierarchical order as explained before. The parameters at the top levels of the classification system are those that define broad classes (e.g. the parameters ‘life form’ and ‘crown cover’ are used to define ‘closed trees’); subsequent parameters further refine the defined class (e.g. ‘broadleaved deciduous closed trees’ or ‘multi-layered closed trees’). The order of the parameters in this way supports class comparisons because comparison will first relate to the broadly defined land-cover type to which the class belongs and then relate to differences within the land-cover type.
In the similarity computation, the values attached to the parameter options are of a two-state character, i.e. either 1 (similar) or 0 (dissimilar). In the various methodologies listed in the section methodologies for assessing semantic similarity, the values often comprise the full range from 1 to 0 to express partial similarity (semantic distance). For example, in cases where properties are imposed on ordinal, interval or ratio scales, similarity can also be expressed as an exponential decay function of semantic distance (e.g. ‘very open (10–20)–40%’ is less distant from ‘sparse’ than from ‘closed’), but such functions are not included in the LCCS-TM. Exceptions to the two-state character of the values in the LCCS-TM however exist. For example, the parameter ‘life form’ has the option ‘woody’ that is further subdivided into ‘trees’ and ‘shrubs’, the option ‘herbaceous’ further subdivided into ‘graminoids’ and ‘forbs’ and the option ‘lichens/mosses’ further subdivided into ‘lichens’ and ‘mosses’. One has to ask ‘to what degree are “woody” and “trees” similar?’ In such cases, the LCCS-TM uses the arbitrary value of 0.5 (half similar or dissimilar) for semantic similarity between either ‘trees’ and ‘woody’ or ‘shrubs’ and ‘woody’ and vice versa (Di Gregorio and Jansen Citation2000).
Wyatt et al. (Citation1994) distinguished three types of matches (): (1) source and target classes match exactly; (2) cases where the source class is a subset of the target class and (3) cases where the target class is a subset of the source class. If one compares ‘trees’ with ‘woody’, the situation would resemble case 2, whereas comparison of ‘woody’ with ‘trees’ resembles case 3. The type of match is different and so too could be the value used for computing semantic similarity in LCCS-TM. The above-mentioned cases are further complicated as the parameter ‘height’ also plays a role in their definitions (see also Section 5.1). Closer examination of the parameters ‘life form’ and ‘height’ reveals overlaps between the lower height limit for trees and the upper limit for shrubs (). Similar overlaps exist also between the minimum height for shrubs and the maximum height for herbaceous life forms. This type of partial overlap is not considered in the similarity computation.
Figure 6. Overlaps in the LCCS parameter and modifier threshold values for the parameter ‘height’ grouped according to the ‘life form’ to which they apply. The definition of ‘dwarf shrubs’ in LCCS is given as smaller than 0.5 m, which can mean either 0–0.5 m or 0.3–0.5 m. Given that the lower threshold value for ‘shrubs’ is set at 0.3 m, 0.3–0.5 m has been used for consistency's sake.
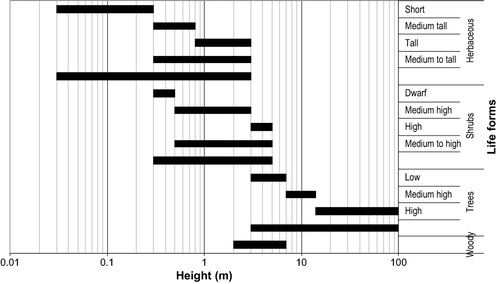
During the computation in LCCS-TM, the software application analyses similarity in two steps: first it will look for the source class parameter in the target class; second if the source class parameter is present in the target class, comparison will take place between source and target class. For example, the first step will analyse whether the parameter ‘life form’ from the source class is present in the target class, if this is so, then the options will be compared, and this could result in ‘trees’ being compared to ‘graminoids’ that are obviously dissimilar and the value 0 will be assigned to the parameter. This process based on commonalities between two classes can be represented in mathematical form by
Though the information richness of classes that include consideration of parameters other than those of land cover or environment can mostly be preserved in LCCS using user-defined parameters, the latter are excluded when computing semantic similarity, and thus for such classes, the index does not represent all the defining elements.
In general, the semantic similarity is highest in a group of classes describing the same land-cover category as they share the same set of parameters. Indices between classes belonging to different land-cover categories are in general small with the exception of cultivated areas and (semi-) natural vegetation because these land-cover categories contain a number of identical parameters to describe plants and their vertical and horizontal arrangements. As a consequence, a similarity can be found between, for instance, graminoid crops with a herbaceous type of (semi-) natural vegetation. Other land-cover categories, such as bare areas and built-up areas, are dissimilar to any other land cover. Artificial water bodies, snow and ice, can be compared to natural water bodies, snow and ice (see ).
Experiences of the NordLaM project with LCCS
The Nordic landscape monitoring (NordLaM) project of the Nordic Council of Ministers decided in 2002 to examine LCCS in the context of land-cover harmonisation at the semantic level using five different Nordic class sets from Denmark, Estonia, Norway (two class sets) and Sweden that are used in landscape monitoring (Groom Citation2004). LCCS was selected to act as mediator, or bridge, between the different ontologies of the class sets. These class sets with a total of 152 classes are from countries with similar types of landscapes but represent different approaches to land cover. The previous findings (Jansen Citation2004a) are critically re-assessed from the methodology viewpoint in this article to underline the importance of (variation in) the semantic content of classes in harmonisation efforts.
The five Nordic class sets include both specific-purpose and general-purpose class sets. What follows is a short description of each class set to give a general idea to the reader:
-
The ‘Area Information System's Land-Cover Map’ (AIS-LCM) of Denmark comprises a general-purpose description of various land-cover types (11 classes) used in land-related research and administrative applications (Groom and Stjernholm Citation2001).
-
The ‘Land-Cover Classification Scheme’ (EELC) of Estonia follows CORINE land cover until the third level with a fourth level comprising detailed vegetation descriptions for wetland land-cover types such as mires (transitional), bogs and fens (Meiner Citation1999). Only a subset of the first 21 classes that refer to the coastal zone and wetlands was analysed.
-
The ‘Monitoring Agricultural Landscapes’ (3Q) of Norway is a class set (57 classes) developed to monitor agricultural land-use patterns, biodiversity and cultural heritage; it contains a mixture of land-cover and land-use characteristics recording also events like trees blown over by strong wind, damage by hailstorms or area burnt by fire (Fjellstad, Mathiesen and Stokland Citation2001).
-
The ‘Digital Field Basis Map’ (DMK) of Norway covers 55% of the country excluding the area above the forest limit. The system focuses on land as a resource for agriculture and forestry (e.g. productivity, degree of cultivation, ploughing depth), and it is thus more geared to land use than land cover (8 classes) (www.skogoglandskap.no/filearchive/Dokument_02_03_nynorsk.pdf).
-
The ‘Land-Cover Data’ (SMD) of Sweden is a general-purpose class set (55 classes) based on CORINE land cover until the third level with a country-specific fourth level including mires, the age and/or height of forest stands and land-use parameters for description of urban areas (Ahlcrona, Olsson and Rosengren Citation2001).
Detailed definitions of the CORINE land-cover classes to the third level, as are included in the Estonian and Swedish class sets, are provided by Bossard, Feranec and Otahel (Citation2000).
Categorisation issues using LCCS as a reference system
Harmonisation of the semantic contents of classes from different class sets using a reference system can be achieved on the condition that the reference system is flexible, can accommodate different classes and allows for acceptable compromises where the correspondence between original class and reference class is less than 100%. To be able to define corresponding classes in the LCCS-CM
-
the main LCCS parameters should coincide with the main parameters used in the original class sets;
-
the hierarchical order of LCCS parameters should not impede defining the corresponding class;
-
the LCCS threshold values in the definition of parameters and parameter options should coincide with those used in the original class sets;
-
information richness of the original classes should be maintained in the corresponding class;
-
there should be fully developed concepts for whichever land-cover type are present and
-
in the original class sets, definitions should be present and unambiguous to establish correspondence.
The main parameters in two LCCS-CM land-cover categories of major interest for landscape-level monitoring were analysed. The relevant classes in the Nordic class sets show that specific-purpose class sets use almost the full range of parameters to describe the cultivated area classes (), whereas in the (semi-) natural vegetated area classes, the parameter use is more dispersed (). Certain parameters or attributes have not been used at all or with only very limited use by these five class sets (e.g. ‘field size’, ‘crop type’ and ‘spatial distribution’). This may be caused by the difficulty to apply such a parameter, or its being not clearly defined or explained, or its being not considered to be of (any) importance. The latter would justify moving such a parameter further down the LCCS-CM parameter hierarchy or ensure that such a parameter can be left out, as is indeed the case already for ‘field size’ and ‘spatial distribution’ while ‘crop type’ is optional.
Table 1. Overview of the use of parameters and two specific attributes in the major land-cover category cultivated terrestrial areas by the different class sets
Table 2. Overview of the use of parameters and one specific attribute in the major land-cover categories (semi-) natural vegetation [terrestrial (A12) and aquatic or regularly flooded (A24)] by the different class sets
Use of the parameters ‘leaf type’ and ‘leaf phenology’ (leaf longevity) is more complicated because one cannot skip ‘leaf type’ to define ‘leaf phenology’ only. As a result, if ‘leaf type’ could not be defined, consequently, one could not add ‘leaf phenology’. More flexibility in LCCS-CM would be required in this case. The almost complete absence of the use of the parameter ‘stratification’ is noteworthy. One explanation of this is that the applications for which the Nordic class sets were created are not interested in the layering of the groups of life forms. Or, it may be that little layering is present in the described vegetation types possibly associated with climate. Or, possibly the use of the ‘stratification’ parameter in LCCS-CM is not seen as straightforward and therefore was passed-over by the translators. Part of the answer may also be that the Nordic class sets are used with remote sensing data applications in which any layering underneath the highest crown cover cannot be identified on the satellite image or aerial photograph. The actual reasons for these patterns in parameter use are not evident.
Threshold values are related to the purpose of a class set, and thus problems were encountered in establishing correspondence as the purposes of the class sets and LCCS-CM differ. The lack of coincidence in threshold values at high levels of the LCCS-CM has a much bigger impact on establishing correspondence than differences at lower hierarchical levels. For instance, the first parameter in the LCCS-CM is to distinguish between primarily vegetated and primarily non-vegetated areas with a threshold value of 4% vegetation cover for at least two months a year. The Norwegian ‘Monitoring of Agricultural Landscapes’ uses 25% crown cover to make the same distinction. If at least 4% of an area is vegetated, LCCS considers the rest of the area to be empty, i.e. there are no other structures or occupied surfaces. If this were not so, one would have to speak of a mixed class in which the vegetated area is subordinate to other land-cover classes (e.g. bare surfaces). Thus, the definition used by ‘Monitoring Agricultural Landscapes’ will either encourage creation in LCCS-CM of mixed classes or else disregard extremely sparse vegetation. In the case of creating mixed classes, correspondence is established as a one-to-many relationship. A second example is the threshold value used for ‘crown cover’, which is the second parameter in the (semi-) natural vegetated areas categories. In the Swedish ‘Land-Cover Data’, 30% is used for distinction between tree-dominated classes (). Thus, this class set comprises a parameter with a definition that came close to the ‘crown cover’ modifier option of 40% in the first version of LCCS-CM. It is important to note that in such a case, the use of a parameter option with a modifier is required. However, in the second version of LCCS, ‘closed-to-open’ with the option ‘more than 40%’ has been added. LCCS contains more options for indication of ‘crown cover’ than most existing categorisation systems, but even so differences of 5–10% in threshold values occur. further shows different thresholds between tree heights used in LCCS-CM and the ‘Swedish Land-Cover Data’: certain tree heights would lead to a different class (e.g. 6 m). Such differences cannot be ignored.
Table 3. Differences in threshold values of various parameters used in LCCS and SMD
In the Norwegian class set, the ‘Digital Field Basis Map’, the thresholds for ‘mixed forest’ are unusual, with 20% crown cover as the lower limit and 50% crown cover as the higher limit for needleleaved trees; thus a forest area with 40% broadleaved trees is classified as needleleaved forest. This is probably due to the larger economic value of needleleaved trees, and therefore this class is of prime interest to the forester. A cultivated area where trees are also present is described as ‘forest’ in this class set, whereas this would be a mixed class in LCCS-CM. In this class set, an area is called ‘forest’ also when it is for the time being without trees as it will be used again for forestry purposes, thus substantiating that category definitions of land cover and land use are different. However, this ‘forest’ situation is analogous to the description of cultivated areas without crops in LCCS-CM, whereas (semi-) natural areas are described by their static and in situ land cover. Thus, the spatial rules for land-cover description in LCCS-CM are distinct for different land-cover categories.
Establishing correspondence between class definitions may lead to the case in which the original Nordic class found correspondence in several LCCS classes due to differences in threshold values, semantic ambiguity or occurrence of two different objects in a class. Thus, the result of the correspondence is a one-to-many relationship. This was the case when a range was included in the definition, especially for the parameter ‘crown cover’ being closed-to-open. This occurred in several vegetation types of the Danish AIS-LCM and the Swedish ‘Land-Cover Data’ class sets and in the ‘forest’ class of the Norwegian ‘Digital Field Basis Map’ where the vegetation can be either closed or open. In the first version of LCCS-CM, one is being forced to create a mixed class creating a one-to-many relationship. In the second version of LCCS-CM, the option ‘closed-to-open’ has been included so that one-to-one relationships can be established.
In other class names, two clearly distinct types of objects co-occur, such as ‘fruit trees and berry plantations’ in the Swedish ‘Land-Cover Data’ class set and in various classes of the AIS-LCM class set. In such cases, various options are possible, taking the ‘Land-Cover Data’ class as an example:
-
A mixed class of fruit tree plantations with berry plantations is created because due to the mapping scale and/or the arrangement of fields these two types of fields cannot be spatially distinguished (one-to-many correspondence relationship).
-
A single class is created containing the dominant crop fruit trees with the berries as a second crop because they occur in the same field. In this case, it is a single class containing a multiple crop (Note: It may also be possible that a single class exist in which the berries are dominant over the trees) (one-to-one correspondence relationship).
-
A mixed class is created combining the two above-mentioned options (one-to-many correspondence relationship).
The best practice in such cases depends on how the two components are arranged spatially, but this information was unavailable for the above-mentioned class. It may also happen that all options occur in practice but that this is not reflected in the original class set and thus poses problems when establishing correspondence. Here, the problem is how to establish correspondence as several options are available. Furthermore, as two types of correspondence relationships may be established, one may well ask whether these would influence the semantic similarity indices.
Information richness in the original classes should be maintained when defining the corresponding classes in the LCCS-TM. Therefore, the occurrence of land-use terminology in some classes related to the monitoring of environmental change (e.g. development of land-use patterns) calls for compromises in establishing correspondence. The difficulty is that semantic differences affect the interoperability between land cover and land use (Brown and Duh Citation2004). LCCS-CM is not dealing with land-use though some management-related parameters are accommodated for cultivated area and built-up area classes. In the Norwegian class sets, grasslands occur that are managed and thus belong to the cultivated areas category of LCCS-CM and some land-cover-related cultural practices could be described. Grasslands that are abandoned and invaded by natural vegetation belong to the (semi-) natural vegetation category and thus land-cover-related cultural practices could not be described. The other class sets also contain classes for which it was difficult to establish any correspondence such as ‘construction sites’, ‘clear felled areas’ and ‘burned areas’ from the Swedish ‘Land-Cover Data’ class set that are relating to a future cover or an event that has removed and/or affected the cover. Here again, the static and in situ description of land cover is requested when actually this is unknown for the classes concerned. For making correspondence, for ‘construction sites’ a mix was chosen between built-up areas, unconsolidated and consolidated materials, whereas the classes concerning burned and clear felled areas were translated as a closed woody vegetation type with an added LCCS user-defined parameter explaining that it refers to a clear felled or burned area. In the draft document of the translation of the CORINE land-cover class set in LCCS (Herold, Hubald, Sarfatti and Di Gregorio Citation2006a), these classes have been translated in a different manner. ‘Burned areas’ are translated as ‘(semi-) natural terrestrial vegetation’ with a user-defined attribute and ‘construction sites’ as an arbitrary mixed class of ‘built-up areas’ with ‘bare areas’. ‘Clear felled areas’ is a fourth level class that is specific for the Swedish class set. All these solutions are very subjective for two reasons: (1) such a solution depends heavily on who is establishing the correspondence and (2) whether this solution or another one was adopted entails introducing uncertainty in the correspondence. One cannot expect to find a perfect match between an actual and a potential land cover. These type of phenomena, and also damage due to hail storms or wind (e.g. in ‘Monitoring Agricultural Landscapes’), cannot be accommodated by any other means than adding user-defined parameters to preserve the information richness of a class, but user-defined parameters are not standardised.
Fully developed concepts are a prerequisite in a reference system. However, the occurrence of lichen-dominated areas with trees cannot be accommodated in LCCS-CM. The lichens concept that is adopted is extremely limited. It is impossible to link this ‘life form’ with any ‘stratification’. This is a significant drawback for the correct establishment of correspondence of vegetation types that include lichens. LCCS-CM cannot claim to be universally applicable as vegetation types in which lichens with trees occur, as are widespread in Nordic countries, cannot be appropriately described.
In the original class sets, definitions should be present and unambiguous to establish correspondence. A problem occurs when a definition is not given, as occurred for the class ‘sparsely vegetated areas’ in the Swedish ‘Land-Cover Data’ class set. These areas were translated as a mixed class containing unconsolidated materials and herbaceous open vegetation. The definition of ‘sparsely’ as used in the ‘Land-Cover Data’ class set is lacking and depending on it could be argued that ‘sparse herbaceous vegetation’ should have been selected for the class correspondence. The corresponding class gives the impression that there are two elements present: (1) bare areas/bare soils with (2) open vegetation. But the concept of sparse vegetation in LCCS is not the same as a mixed class of bare soil with vegetation. Whichever solution is adopted, it means introducing uncertainty.
As illustrated with the above examples, several problems were identified in establishing correspondences related to both the original class sets and the LCCS-CM resulting in several cases in which a questionable solution for ‘translation’ was adopted and where (further) uncertainty was introduced.
Semantic similarity issues using LCCS as a reference system
Pair-wise calculation of semantic similarity between the corresponding classes of the original Nordic classes was performed to quantify the similarity, and inversely dissimilarity, of their semantic class contents. As an example, the comparison of corresponding classes of the Danish AIS-LCM is shown (). The darker the grey shading, the more similar the classes. The matrix shows clearly that comparison of class A (source) with class B (target) results in a different semantic similarity as the comparison of class B (source) with class A (target); thus the indices in the matrix on both sides of the diagonal are asymmetrical. The matrix is based on correspondence with the first version of LCCS. For a number of classes, one-to-many correspondence relationships were established as the original Danish classes comprised either a range (e.g. open-to-closed in the forest classes) or two different objects (e.g. shrubs and grass in ‘shrub and grass heath land’) or made no distinction where in LCCS a distinction is made (e.g. the kind of water bodies). Similarity indices showing high similarity further from the diagonal axis are mostly linked to the aquatic (semi-) natural vegetation type of class ‘marshland’. This class can be regrouped with the terrestrial vegetation classes under the category of (semi-) natural vegetation independent of the environment (e.g. aquatic or terrestrial) in which the vegetation type occurs (grey line in ). The other, lower (but non-zero) off-diagonal similarity indices are caused by the occurrence of similar ‘life forms’ between classes. illustrates that the similarity within a category is in general higher than between categories. One should note that in case of mixed classes being present, only one element of a mixed class could be selected as source class in the computation. When selecting the source class in LCCS, one can set which element of the mixed class will be the source class (e.g. see division of mixed classes 6, 7, 8, 10 and 11 where the and refer to the first and second element of the mixed class). This source class can be compared only to the first element of a mixed target class (comparison of 6.1 with mixed classes 7, 8, 10 and 11 refers to comparison of 6.1 with 7.1, 8.1, 10.1 and 11.1, respectively). Thus, in a case of mixed classes, the semantic similarity calculated addresses only part of the classes present in both source and target class. The question raised earlier when there were various options for correspondence of the class ‘fruit trees and berry plantations’ in the ‘Land-Cover Data’ class set can now be answered: in LCCS-TM, the option selected for correspondence has repercussions for the semantic similarity.
Table 4. Semantic similarity of corresponding classes of the Danish AIS-LCM class set showing one-to-many correspondence relationships used as source class but absent from the target class (based upon LCCS version 1 with similarity in percentages)
Of more interest than the full correspondence matrices is, in the context of this article, to better understand the computation of semantic similarities within LCCS-TM. A series of examples will illustrate how the choices made in the implemented algorithm influence the computed indices. show how the similarity is calculated and illustrate at the same time that the source class greatly determines the type and number of parameters in the computation. One should note that two types of null values occur: (1) null value to indicate that although the parameter or modifier is shared the options are dissimilar and (2) the parameter or modifier of the source class is not present in the target class. The treatments of these different null values in the similarity calculation are identical. Examples 1 and 3 in show the influence of the number of parameters of the source class in the computation. In both examples, only one parameter is common. A parameter that is present in the target class but not in the source class is not considered. Examples 2 and 4 in are selected to show the influence of the decision in LCCS that whether ‘graminoids’ is compared to ‘herbaceous’ or ‘herbaceous’, compared to ‘graminoids’ the value is always 0.5. Example 2 shows the case that the source parameter is a subset of the target parameter, whereas Example 4 shows the case that the target parameter is a subset of the source parameter. Furthermore, comparing Examples 1 with 2 and Examples 3 with 4, one can see the influence of the arbitrary value of 0.5 in the resulting semantic similarity indices.
Table 5. Semantic similarity between cultivated area classes of the 3Q and (semi-) natural vegetated area classes of the SMD class sets
Table 6. Semantic similarity of (semi-) natural terrestrial vegetation and (semi-) natural aquatic or regularly flooded vegetation classes from the 3Q class set with (semi-) natural terrestrial vegetation classes of the SMD class set
Table 7. Semantic similarity between two (semi-) natural terrestrial vegetation classes from the Swedish SMD class set
illustrates how modifiers that define a parameter option in more detail influence the computation, because when used they have the same weight in the computation as a parameter option. In fact, one could argue whether it is correct to count a single element twice when a parameter option is present with its modifier. shows another incongruity in that the weights of all parameters in the computation in LCCS are equal: if the first and most important parameter ‘life form’ is dissimilar and only the ‘crown cover’ is similar, a very high semantic similarity is calculated between two distinct vegetation types. Here the issue of assigning weights to parameters is important (see Section 5.2). When calculating semantic similarity according to Feng and Flewelling (Citation2004) (
EquationEquation 55), weights can be assigned in two different manners: the α or the weights that are assigned to each pair of A∩B, A/B and B/A in EquationEquation (5)
5. For estimating α of categories within a single categorisation system, Rodríguez et al. (Citation1999) suggested that the number of links from both categories to the immediate category that includes both categories can be used. But when using different categorisation systems, this is impossible and a value of 0.5 can be assigned to α (thus 1 – α is also 0.5). Weights assigned to each pair of A∩B, A/B and B/A can be related to the depth and density of the categories in the hierarchical categorisation system. Depth of two categories can affect semantic similarity measures because categories at lower hierarchical levels are more refined than those at higher levels. This implies that two categories at lower hierarchical levels are more similar in semantics than those at higher levels. The density of the categorisation system can also affect semantic similarity measures. This is because categories in a denser portion of the categorisation system (e.g. in LCCS in the primarily vegetated area land-cover categories) are closer in meaning than those in a less dense portion. If the denser portion of the categorisation system has many more categories than the less dense portion, the semantic similarity measures between categories in these two portions of the categorisation system may be skewed compared with measures that are made on two categories from the same portion of the taxonomy. To account for these factors, it has been suggested that the number of links coming out of a category can be used as an estimate for density and the number of levels down in the categorisation system can be used as an estimate for depth. A weight can then be calculated based on the combination of these two estimates (Feng and Flewelling Citation2004). illustrate bias present in the algorithm implemented in LCCS. It is important that the current algorithm be changed to one that takes better into account the importance of parameters used in the definition of classes, the position of the class in the categorisation hierarchy, the type of match and especially one that includes a semantic distance function for partial overlap.
Discussion
Differences in semantic concepts are often the key obstacle to data integration. One should realise that inconsistencies that hamper establishment of correspondence occur both in the Nordic class sets and in the LCCS-CM and that with the use of LCCS-CM as reference system, a further level of uncertainty is introduced compared to direct comparison of the Nordic class sets. Correspondence can be either complete, partial or approximate at best, and in all cases, it would be extremely useful being able to quantify the level of correspondence as this would give an idea not only of how much information was lost but also of uncertainty. It is unrealistic to expect that no information losses will occur, but it is important that such losses are within acceptable, preferably quantified limits.
Establishment of correspondence has also implications for the class set structure because the corresponding class is not necessarily of a similar hierarchical level than the original class. More complicated is the situation in which one-to-many relationships are needed to establish correspondence. In such cases, the corresponding classes may be of different hierarchical levels. Such changes in class set structure lead inevitably to changes in the data structure that were not examined in this article.
In the assessment of LCCS as a reference system, the impression prevailed in the NordLaM project that instead of defining the correspondence between a Nordic class and LCCS, one was establishing how much of LCCS was in the original class. A prerequisite for a reference system would be an approach in which classes can be accommodated that may call for compromises in the adopted concepts and structure of the reference system to conserve information richness, but this type of flexibility is lacking in LCCS. The only way at present to store information richness that does not correspond or coincide to LCCS parameters is the application of user-defined parameters. However, these are not considered in the semantic similarity computation. In such cases, it would be more meaningful to compute semantic similarity directly between the original Nordic classes.
To support the use of the LCCS categorisation methodology as a reference system, there should be clear convincing advantages to counterbalance its semblance to a ‘black box’, as a formal definition of the categorisation rules is lacking. In the NordLaM project, the use as a reference categorisation system alongside the existing system in the country allowed the user to fall back on the well-known existing system, and because both systems are used at the same time, the learning curve of understanding the LCCS categorisation methodology may be less steep. When introduced as a new categorisation system, the user has no fallback option and thus has either to come to terms with the steep learning curve of a ‘black box’ or depend on FAO for support. In the latter situation, it would be important to reflect on the implications of such dependence. For example, what should a user do who wants to apply LCCS in environmental monitoring and modelling using software packages without making a direct link to the LCCS software or a user who basically needs to integrate spatial data? As shown by Ahlqvist (Citation2005b) and Mayaux et al. (Citation2006), one can take from the full LCCS methodology those elements that are useful in a specific application.
Though it seems that quite a number of class sets meanwhile have been translated (according to Herold et al. Citation2006b), the discussion of encountered problems and adopted solutions in these crosswalk ‘translations’ have not been made available to the scientific public apart from the example of 26 classes provided in McConnell and Moran (Citation2001) and the draft document of Herold et al. (Citation2006a) that was made available to the authors. The encountered problems and solutions, however, are a basis for further discussion to reach consensus. Feedback from the user and scientific communities will be indispensable in order to assess and enhance the current methodology.
The current semantic similarity algorithm in LCCS is too simplistic to deal with the complexity of semantic similarity. It seems that many recent developments in the field of semantic similarity metrics have been overlooked in LCCS. The parameterised concept definitions of LCCS could be used to bridge between concepts in different categorisation systems and class sets. However, as Ahlqvist (Citation2004) rightly points out, LCCS-CM uses standard set theoretic representations without recognising a semantic space underlying the concept representation, thus limiting the possibilities to measure in the LCCS-TM semantic similarity based on concept distance. Examination of the semantic similarity metrics in literature makes it evident that a thorough revision of the implemented LCCS-TM methodology is necessary and until that has happened its use is not recommended. Moreover, implementation of various methodologies for semantic similarity should be considered as each methodology has its own merits.
The NordLaM project selected LCCS as a reference system to establish semantic correspondence between Nordic class sets used in landscape monitoring with quantification of semantic similarity as the ultimate goal. However, in establishing correspondence with LCCS-CM, uncertainties were introduced that could not be quantified, whereas the semantic similarity indices resulted in startling findings. Introduction of unknown quantities of uncertainty hamper the proper distinction between real changes from changes in categorisation. As a result, there were no apparent convincing methodological advantages in using LCCS as a reference system, other than that the use of a reference system reduces the number of pair-wise class comparisons to be made.
Currently, there is an urgent need to make the formal definition of the full methodology implemented in LCCS available to the user and scientific communities. The suggestion at the expert consultation in Artimino, Italy, in 2002 to set up a technical panel (FAO Citation2002, p.16; Jansen Citation2004b) that would receive feedback from the user and scientific communities and that would propose in a participatory way improvements of LCCS has, as far as we know, not been realised but such a panel could act as a forum to channel methodological improvements of the system. Such processes are important as FAO's intention is that the LCCS categorisation methodology should become an ISO standard (personal communication, FAO). The critical examination in this article, however, shows that there is not only room for improvement of LCCS but there is a real need, as there are various methodological issues raised in this article that seem significant. If the LCCS is recommended as a basis for a land-cover harmonisation strategy, one should be aware that the implemented methodology has a series of problems and shortcomings.
Recommendations and open research questions
The way forward to land-cover harmonisation is probably adoption of a parameterised approach such as that implemented in LCCS. The advantages of such an approach are that the parameters with which classes are defined become explicit and class comparisons can be made in a systematic manner. However, correspondence needs to be accompanied by a mathematical theory that addresses uncertainty. As the NordLaM project experiences show, there are quite a number of methodological issues for which in each individual harmonisation attempt the so-called best practices are developed but a wider consensus of such practices is lacking. Therefore, each ‘translated’ land-cover class or data set risks to be a result that cannot be replicated by others in exactly the same way, no matter how many official organisation endorse such a ‘translation’. This is a scientifically and practically unsound situation. More research is needed to improve existing and further develop methodologies.
It seems unrealistic to expect that land-cover standardisation will lead to worldwide adoption of a single categorisation system. Each class set has its own worldview and this is also true for LCCS as a categorisation system. Land-cover standardisation would lead to adoption of a single worldview, whereas land-cover harmonisation allows different worldviews to co-exist. The latter seems not only a much more flexible approach but also one that makes the world richer.
Semantic uncertainty is an inseparable companion of almost any information and that is certainly the case for harmonisation efforts in which different types of uncertainty accompany each other. At present, there is no accepted way to derive an overall score of the semantic similarity between two class sets and no measure to establish the success of correspondence at class level. Such indices would be particularly important when translating existing class sets into a reference system's terminology as they could indicate if the correspondence is close to the original class set and how well the fit between original class and corresponding class is. Such a quality assessment of the correspondence per class as well as per class set is suggested as analogous to the thematic accuracy assessment and has been suggested by Ahlqvist et al. (Citation2000) and by Jansen (Citation2006) for land use. Such quality statements are important if correspondence results are to be linked to semantic similarity indices as discussed here, and in the case that they need to be linked to land-cover change dynamics and the boundary conditions verified in the data validation effort are involved. If one wants to monitor gradual changes at the landscape level, then one has the necessity to be able to distinguish between real changes and changes in categorisation definitions. Quantitative semantic similarity metrics may help to better assess such differences, whereas at present, there is often no explicit recognition of semantic differences in cases where two different class sets or categorisation systems are involved.
Methodologies for semantic similarity metrics should be evaluated using a single class set so as to assess the merits of the different methodologies. The cited examples from the literature describe each of their own methodology applied in a particular area, and it is difficult to compare the advantages and disadvantages of these methodologies. Furthermore, it would be interesting to see how semantic similarity indices of the different methodologies vary when applied to the same class set.
The possibility that metadata accompanying land-cover data sets should be extended to comprise more information on data and class accuracy, including the various levels of class (set) correspondence, should be further assessed and discussed. Especially in computer and information sciences, a number of useful suggestions have been made but none seems to have become part of a metadata standard. This is, however, important in the context of SDI as it would inform data users much better as to what one can and cannot do with data.
The distinct aspects of spatial data integration that this article has discussed briefly, i.e. adopted concept, spatial, temporal and quality aspects, should be considered in parallel to the semantic aspects. Harmonisation of land-cover data that deals solely with the semantic contents of the classes is a misrepresentation of the complexity of land-cover harmonisation. If the two different class and data sets to be harmonised are seen as two different ‘objects’, the harmonisation is the establishment of relationships between the two objects. The relationship between any two objects encompasses the assumptions that each makes about the other, including what operations can be performed and what behaviour results (Booch Citation1994).
Acknowledgements
The authors thank Kiira Aaviksoo of the Estonian Environmental Information Centre, Wendy Fjellstad and Arnt Kristian Gjertsen of the Norwegian Institute of Land Inventory and Eva Ahlcrona of Swedish Metria Miljöanalys for making the correspondences of the Nordic land-cover class sets with LCCS, their contributions and Michael Ledwith's (Metria Miljöanalys, Sweden) to the workshops and discussion forum of the Nordic Landscape Monitoring project of the Nordic Council of Ministers. Chris Steenmans of the European Environment Agency is thanked for making available the draft document with the translation of the CORINE land-cover class set into LCCS. Finally, the authors thank A.J. Comber, University of Leicester, for his constructive comments on the manuscript.
Notes
†Land/Natural Resources Consultant, via Girolamo Dandini 21, 00154 Rome, Italy
1 An ontology is an explicit specification of a conceptualisation (Gruber Citation1993). In both computer and information sciences, an ontology is a data model that represents a set of concepts within a domain and the relationships between those concepts. It is used to reason about the objects within that domain. In the context of this article, one can consider ontology to be synonymous with categorisation system.
2 In LCCS, this parameter is called ‘cover’, but in this text the term ‘crown cover’ has been preferred.
References
- Ahlcrona , E. , Olsson , B. and Rosengren , M. 2001 . “ Swedish CORINE Land Cover ” . In Strategic Landscape Monitoring for the Nordic Countries , TemaNord 523 Edited by: Groom , G. and Reed , T. 95 – 99 . Copenhagen : Nordic Council of Ministers .
- Ahlqvist , O. 2004 . A Parameterized Representation of Uncertain Conceptual Spaces . Transactions in GIS , 8 : 493 – 514 .
- Ahlqvist , O. 2005a . “ Using Semantic Similarity Metrics to Uncover Category and Land-Cover Change ” . In Geospatial Semantics (GeoS) First International Congress , Edited by: Rodríguez , M. A. , Cruz , I. F. , Egenhofer , M. J. and Levashkin , S. 107 – 119 . Mexico City, , Mexico : Springer Publishers, Berlin-Heidelberg . 29–30 November 2005
- Ahlqvist , O. 2005b . Using Uncertain Conceptual Spaces to Translate Between Land-Cover Categories . International Journal of Geographical Information Science , 19 : 831 – 885 .
- Ahlqvist , O. 2005c . Probing the Relationship Between Classification Error and Class Similarity . Photogrammetric Engineering & Remote Sensing , 71 : 1365 – 1373 .
- Ahlqvist , O. , Keukelaar , J. and Oukbir , K. 2000 . Rough Classification and Accuracy Assessment . International Journal of Geographic Information Science , 14 : 475 – 496 .
- Ahlqvist , O. and Shortridge , A. 2006 . “ Characterising Land-Cover Structure With Semantic Variograms ” . In Progress in Spatial Data Handling: 12th International Symposium on Spatial Data Handling , Edited by: Riedl , A. , Kainz , W. and Elmes , G. 401 – 415 . Berlin-Heidelberg : Springer Publishers .
- Bishr , Y. A. , Pundt , H. , Kuhn , W. and Radwan , M. 1999 . “ Probing the Concept of Information Communities – a First Step Toward Semantic Interoperability ” . In Interoperating Geographic Information Systems , Edited by: Goodchild , M. , Egenhofer , M. , Fegeas , R. and Kottman , C. 55 – 69 . Dordrecht : Kluwer Academic Publishers .
- Booch , G. 1994 . Object-Oriented Analysis and Design with Applications , 2nd , Santa Clara, CA : Benjamin/Cummings Publishing Company Inc .
- Bossard , M. , Feranec , J. and Otahel , J. 2000 . CORINE Land Cover Technical Guide – Addendum 2000 , Technical Report 40 105 Copenhagen : European Environment Agency .
- Brown , D. G. and Duh , J. -D. 2004 . Spatial Simulation for Translating from Land Use to Land Cover . International Journal of Geographic Information Science , 18 : 35 – 60 .
- Büttner , G. , Feranec , J. , Jaffrain , G. , Mari , L. , Maucha , G. and Soukup , T. 2004 . The European CORINE Land Cover 2000 Project . XX Congress of the International Society for Photogrammetry and Remote Sensing , 1 : 12 2–23 July 2004, Istanbul, Turkey
- Cihlar , J. and Jansen , L. J.M. 2001 . From Land Cover to Land Use: A Methodology for Efficient Land-Use Mapping Over Large Areas . The Professional Geographer , 53 : 275 – 289 .
- Comber , A. J. , Fisher , P. F. and Wadsworth , R. A. 2004 . Assessment of a Semantic Statistical Approach to Detecting Land-Cover Change Using Inconsistent Data Sets . Photogrammetric Engineering & Remote Sensing , 70 : 931 – 938 .
- Comber , A. , Fisher , P. and Wadsworth , R. 2005 . Comparing Statistical and Semantic Approaches for Identifying Change From Land-Cover Data Sets . Journal of Environmental Management , 77 : 47 – 55 .
- Defries , R. S. and Belward , A. S. 2000 . Global and Regional Land-Cover Characterisation From Satellite Data: An Introduction to the Special Issue . International Journal of Remote Sensing , 21 : 1083 – 1092 .
- Di Costanzo , M. and Ongaro , L. 2004 . The Land Cover Classification System (LCCS) as a Formal Language: A Proposal . Journal of Agriculture & Environment for International Development , 98 : 117 – 164 .
- Di Gregorio , A. and Jansen , L. J.M. 2000 . Land Cover Classification System (LCCS): Classification Concepts and User Manual , FAO/UNEP, 177 : Rome .
- Dolman , A. J. , Verhagen , A. and Rovers , C. A. , eds. 2003 . Global Environmental Change and Land Use , Dordrecht : Kluwer Academic Publishers .
- European Commission (EC) . 2001 . Manual of Concepts on Land-Cover and Land-Use Information Systems , 106 Luxembourg : European Commission . EC-EUROSTAT Edition 2000 – Theme 5
- FAO . . Proceedings of the FAO/UNEP Expert Consultation on Strategies for Global Land-Cover Mapping and Monitoring . 6–8 May 2002 , Artimino, Florence, Italy. pp. 38 Rome : FAO/UNEP .
- FAO . 2005 . Land Cover Classification System – Classification Concepts and User Manual 190 Software version 2, Environment and Natural Resources Series 8,
- Feng , C-C. and Flewelling , D. M. 2004 . Assessment of Semantic Similarity Between Land-Use/Land-Cover Classification Systems . Computers, Environment & Urban Systems , 28 : 229 – 246 .
- Fisher , P. F. 2003 . Multimedia Reporting of the Results of Natural Resource Surveys . Transactions in GIS , 7 : 309 – 324 .
- Fjellstad , W. , Mathiesen , H. and Stokland , J. 2001 . “ Monitoring Norwegian Agricultural Landscapes – The 3Q Programme ” . In Strategic Landscape Monitoring for the Nordic Countries , TemaNord 523 Edited by: Groom , G. and Reed , T. 19 – 28 . Copenhagen : Nordic Council of Ministers .
- Fritz , S. and See , L. 2005 . Comparison of Land-Cover Maps Using Fuzzy Agreement . International Journal of Geographical Information Science , 19 : 787 – 807 .
- Groom , G. , ed. 2004 . Developments in Strategic Landscape Monitoring for the Nordic Countries , ANP 705 75 – 118 . Copenhagen : Nordic Council of Ministers .
- Groom , G. and Stjernholm , M. 2001 . “ The Area Information System – A Danish National Spatial Environmental Database ” . In Strategic landscape monitoring for the Nordic countries , TemaNord 523 Edited by: Groom , G. and Reed , T. 81 – 88 . Copenhagen : Nordic Council of Ministers .
- Gruber , T. R. 1993 . Towards Principles for the Design of Ontologies Used for Knowledge Sharing . International Journal Human-Computer Studies , 43 : 907 – 928 .
- Herold , M. , Hubald , R. , Sarfatti , P. and Di Gregorio , A. 2006a . Translating and evaluating the CORINE legend using the UN Land Cover Classification System (LCCS) , Draft version 70 20 September 2006,
- Herold , M. , Latham , J. S. , Di Gregorio , A. and Schmullius , C. C. 2006b . Evolving Standards in Land-Cover Characterisation . Journal of Land Use Science , 1 : 157 – 168 .
- INSPIRE Environmental Thematic Coordination Group (2002), Environmental Thematic User Needs – Position Paper (version 2). Copenhagen: European Environmental Agency www.inspire.jrc.it
- Jansen , L. J.M. 2004a . “ Thematic Harmonisation and Analyses of Nordic Data Sets into Land Cover Classification System (LCCS) Terminology ” . In Developments in Strategic Landscape Monitoring for the Nordic Countries , ANP 705 Edited by: Groom , G. 91 – 118 . Copenhagen : Nordic Council of Ministers .
- Jansen , L. J.M. 2004b . “ Global Land-Cover Harmonisation: Report of the UNEP/FAO Expert Consultation on Strategies for Land-Cover Mapping and Monitoring ” . In Developments in Strategic Landscape Monitoring for the Nordic Countries , ANP 705 Edited by: Groom , G. 75 – 89 . Copenhagen : Nordic Council of Ministers . 6–8 May 2002, Artimino, Italy.
- INSPIRE , Environmental Thematic Coordination Group . 2006 . Harmonisation of Land-Use Class Sets to Facilitate Compatibility and Comparability of Data Across Space and Time . Journal of Land Use Science , 1 : 127 – 156 .
- Jansen , L. J.M. and Di Gregorio , A. 2002 . Parametric Land-Cover and Land-Use Classifications as Tools for Environmental Change Detection . Agriculture, Ecosystems & Environment , 91 : 89 – 100 .
- Jansen , L. J.M. , Mahamadou , H. and Sarfatti , P. 2003 . Land-Cover Change Analysis Using LCCS . Journal of Agriculture and Environment for International Development , 97 : 47 – 68 .
- Jones , C. B. , Ware , J. M. and Miller , D. R. 1999 . “ A Probabilistic Approach to Environmental Change Detection With Area-Class Map Data ” . In Integrated Spatial Databases, Digital Images and GIS , Lecture Notes in Computer Science Vol. 1737 Edited by: Agouris , P. and Stefanidis , A. 122 – 136 . Berlin-Heidelberg, Springer Publishers .
- Kavouras , M. and Kokla , M. 2002 . A Method for the Formalisation and Integration of Geographical Categorizations . International Journal of Geographical Information Science , 16 : 439 – 453 .
- Lambin , E. F. , Rounsevell , M. D.A. and Geist , H. J. 2000 . Are Agricultural Land-Use Models Able to Predict Changes in Land-Use Intensity? . Agriculture, Ecosystems and Environment , 82 : 321 – 331 .
- Loveland , T. R. , Reed , B. C. , Brown , J. F. , Ohlen , D. O. , Zhu , Z. , Yang , L. and Merchant , J. W. 2000 . Development of a Global Land-Cover Characteristics Database and IGBP DISCover from 1 km AVHRR data . International Journal of Remote Sensing , 21 : 1303 – 1330 .
- Lund , H. G. 1999 . A ‘Forest’ by any Other Name… . Environmental Science & Policy , 2 : 125 – 133 .
- Mayaux , P. , Eva , H. , Gallego , J. , Strahler , A. H. , Herold , M. , Agrawal , S. , Naumov , S. , De Miranda , E. E. , Di Bella , C. M. , Ordoyne , C. , Kopin , Y. and Roy , P. S. 2006 . Validation of the Global Land-Cover 2000 Map . IEEE Transactions on Geoscience and Remote Sensing , 44 : 1728 – 1739 .
- Mcconnell , W. J. and Moran , E. F. , eds. 2001 . “ Meeting in the Middle: The Challenge of Meso-Level Integration ” . In An international workshop on the harmonisation of land-use and land-cover classification , LUCC Report Series No. 5 Louvain-la-Neuve : Anthropological Center for Training and Research on Global Environmental Change – Indiana University and LUCC International Project Office .
- Meiner , A. , ed. 1999 . “ Land Cover of ESTONIA ” . In Implementation of CORINE Land Cover Project in Estonia , 133 Tallinn, , Estonia : EEIC .
- Meyer , W. B. and Turner , B. L. , eds. 1994 . Changes in Land Use and Land Cover: A Global Perspective , Cambridge, UK : Cambridge University Press .
- Rodríguez , M. A. and Egenhofer , M. J. 2003 . Determining Semantic Similarity Among Entity Classes From Different Ontologies . IEEE Transactions on Knowledge and Data Engineering , 15 : 442 – 456 .
- Rodríguez , M. A. , Egenhofer , M. J. and Rugg , R. D. 1999 . “ Assessing Semantic Similarities Among Geospatial Feature Class Definitions ” . In Interoperating Geographic Information Systems , InterOp’99 Edited by: Vckofski , A. , Brassel , K. and Schek , H-J. 189 – 202 . Zurich, , Switzerland : Springer Publishers, Berlin-Heidelberg . (Lecture Notes in Computer Science Vol. 1580)
- Strahler , A. H. , Boschetti , L. , Foody , G. M. , Friedl , M. A. , Hansen , M. C. , Herold , M. , Mayaux , P. , Morisette , J. T. , Stehman , S. V. and Woodcock , C. E. 2006 . Global Land-Cover Validation: Recommendations for Evaluation and Accuracy Assessment of Global Land-Cover Maps , 48 European Commission, Directorate-General Joint Research Centre, Institute for Environment and Sustainability .
- Turner , B. L. , Skole , D. , Sanderson , S. , Fischer , G. , Fresco , L. O. and Leemans , R. 1995 . Land-Use and Land-Cover Change Science Research Plan,” IGBP Global Change , Report No.35/IHDP Report No. 7 Stockholm/Geneva : IGBP/IHDP .
- UNEP/FAO . 1994 . “ Report on the UNEP/FAO Expert Meeting on Harmonizing Land-Cover and Land-Use Classifications ” . GEMS Report Series No.25 Nairobi
- Vckovski , A. 1999 . “ Interoperability and Spatial Information Theory ” . In Geographical Information Systems – Principles and Technical Issues , Edited by: Longley , P. A. , Goodchild , M. F. , Maguire , D. J. and Rhind , D. W. 31 – 37 . New York : Wiley .
- Wadsworth , R. A. , Comber , A. J. and Fisher , P. F. 2006 . “ Expert Knowledge and Embedded Knowledge: Or Why Long Rambling Class Descriptions are Useful ” . In Progress in Spatial Data Handling: 12th International Symposium on Spatial Data Handling , Edited by: Riedl , A. , Kainz , W. and Elmes , G. 197 – 213 . Berlin Heidelberg : Springer Publishers .
- Walker , B. , Steffen , W. , Canadell , J. and Ingram , J. , eds. 1997 . The Terrestrial Biosphere and Global Change: Implications for Natural and Managed Ecosystems , IGBP Book Series 4 (synthesis volume) , Cambridge : Cambridge University Press .
- Wyatt , B. K. and Gerard , F. F. 2001 . “ What's In a Name? Approaches to the Inter-Comparison of Land-Use and Land-Cover Classifications ” . In Strategic Landscape Monitoring for the Nordic Countries , TemaNord 523 Edited by: Groom , G. and Reed , T. 113 – 121 . Copenhagen : Nordic Council of Ministers .
- Wyatt , B. K. , Greatorex-Davies , J. N. , Hill , M. O. , Parr , T. W. , Bunce , R. H.G. and Fuller , R. M. 1994 . Countryside Survey 1990: Comparison of Land-Cover Definitions , London : Department of the Environment .