Abstract
Eastern Europe provides unique opportunities to study changes in land use patterns, because much farmland became parcelized in the post-socialist period (i.e. large fields were broken up into smaller ones). Classification-based remote sensing approaches, however, do not capture such land cover modifications and new approaches based on continuous indicators are needed. Our goal is to develop a novel method to map farmland field size based on image texture. We fitted linear regression models to relate field size to Landsat-based image texture for a study area in the border region of Poland, Slovakia and Ukraine. Texture explained up to 93% of the variability in field size. Our field size map revealed marked differences among countries and these differences appear to be related to socialist land-ownership patterns and post-socialist land reform strategies. Image texture has great potential for mapping land use patterns and may contribute to a better understanding of land cover modifications in Eastern Europe and elsewhere.
1. Introduction
Land use change is one of the primary drivers of global environmental change (Foley et al. Citation2005; Turner, Lambin, and Reenberg Citation2007). An improved understanding of how land use decisions are made is urgently needed to better assess the consequences of land use change for ecosystem services and human wellbeing (Rindfuss, Walsh, Turner, Fox, and Mishra Citation2004; GLP Citation2005). Institutions, laws, and political and socio-economic conditions form the background for land use decisions and may increasingly outrank other factors as determinants of land use (Kaimowitz, Thiele, and Pacheco Citation1999; Geist and Lambin Citation2002; Lambin and Geist Citation2006). Linking land use change with its political and socio-economic boundary conditions, however, remains a challenge (GLP Citation2005; Turner et al. Citation2007), partly because it may manifest itself in both conversions (changes from one thematic class to another) and modifications (subtle changes within a thematic class) of land cover (Lambin and Geist Citation2006). However, to date, most studies assessing broad-scale factors of land use change focus on land cover conversions such as deforestation (e.g. Mertens, Sunderlin, Ndoye, and Lambin Citation2000) or urbanization (e.g. Seto and Kaufmann Citation2003). This is problematic because land cover modifications are widespread and possibly more important than land cover conversions (Lambin et al. Citation2001). For example, the area affected by forest degradation in the Amazon (e.g. through selective logging) equals at least the area affected by forest conversions (Asner et al. Citation2005; Foley et al. Citation2007). Agricultural intensification increased the world's food production substantially (Matson, Parton, Power, and Swift Citation1997; Bennett and Balvanera Citation2007), but decreased farmland biodiversity (Donald, Pisano, Rayment, and Pain Citation2002). Despite their importance, land cover modifications have so far been relatively neglected (Lambin and Geist Citation2006) and there is a need to quantify their extent, and to assess their relationship to broad-scale political, institutional and socio-economic conditions.
One prominent case of land cover modifications occurs when the size or configuration of land management units within a land cover class is altered. Such dynamics in land use patterns often take place when changes in politics or socio-economics trigger changes in land use practices, land management policies or land-ownership structures (GLP Citation2005; McConnell and Keys Citation2005). Central and Eastern Europe's farmland provides a good example of such a process (Swinnen and Mathijs Citation1997; Lerman, Csaki, and Feder Citation2004). After World War II, socialist governments across Eastern Europe intensified agriculture and shifted ownership from private citizens to the state (i.e. collectivization, Lerman Citation2001; Van Dijk Citation2003). This transformation was accompanied by a widespread spatial reorganization of land management units. Small pre-socialist farms were dissolved and large, state-controlled agricultural enterprises managed almost all farmland (Lerman Citation2001).
Patterns of farmland changed again drastically after the breakdown of the Soviet Union in 1990, when most Eastern European countries privatized and individualized land management, leading to widespread land-ownership transfers and the downsizing of farms (Lerman et al. Citation2004). Land use patterns changed in many areas, as socialist farmland fields were subdivided (Sabates-Wheeler Citation2002; Van Dijk Citation2003). This physical fragmentation of farmland (hereafter called parcelization) has many economic and ecological consequences. For example, parcelization decreases agricultural efficiency (Sabates-Wheeler Citation2002) and may lead to abandonment of commonly used infrastructure (Penov Citation2004). However, parcelization increases farmland biodiversity (Benton, Vickery, and Wilson Citation2003) and soil stability (Van Rompaey, Krasa, Dostal, and Govers Citation2003). Despite the significance of parcelization for rural Eastern Europe, surprisingly little is known about Eastern Europe's land use patterns and how they changed since 1990.
This lack of information is unfortunate because studying land use patterns offers unique opportunities to better understand the effects of changing institutions, politics and socio-economics on land use decisions. Moreover, field size can be interpreted as an indicator of land ownership and land management, particularly when comparing land use patterns among different countries with similar environmental conditions. However, mapping field size in Eastern Europe is challenging, because cadastral data are largely unavailable or of unknown accuracy. Remote sensing is an alternative that can overcome some of these problems.
Very few studies used remote sensing for automated assessments of field size. This is not surprising, because most conventional image classification and change detection methods stratify images into discrete classes (Southworth, Munroe, and Nagendra Citation2004; Lambin and Geist Citation2006). Using classification-based methods to map field size requires classifying all occurring crop types. Such detailed classifications are only possible for detailed time series of satellite images or where crops have unique spectral properties, for example, in the case of rapeseed (Elliott et al. Citation2004). In most cases, however, acquiring training data for detailed crop type classifications is not feasible, and the spectral similarity of crops inhibits detailed classifications. Field boundaries can also be delineated using image segmentation (Evans, Jones, Svalbe, and Berman Citation2002; Lloyd, Berberoglu, Curran, and Atkinson Citation2004). Yet, this is only possible where fields are much larger than the dimensions of a pixel, because mixed pixels result in poor boundary discrimination (Turner and Congalton Citation1998; Silleos, Perakis, and Petsanis Citation2002; Ozdogan and Woodcock Citation2006). Small fields are common in Eastern Europe because of farmland parcelization (Sabates-Wheeler Citation2002; Van Dijk Citation2003) and this inhibits the use of image segmentation to delineate field boundaries.
An alternative is to characterize land cover using continuous variables, which can detect subtle changes (Southworth et al. Citation2004; Turner Citation2005). A few such methods exist (e.g. change vector analysis, spectral mixture analysis, Coppin, Jonckheere, Nackaerts, Muys, and Lambin Citation2004), but they are based on spectrally homogeneous land cover types (i.e. changes in the signal are related to changes in land cover condition). This is problematic in the case of farmland, where different crops and phenology result in high spectral variability. Moreover, many methods focus on the spectral domain only (Coppin et al. Citation2004; Southworth et al. Citation2004), but the spatial domain also contains important information (Chica-Olmo and Abarca-Hernandez Citation2000; Cihlar Citation2000). Methods based on continuous data that integrate the spectral and spatial domains (Southworth et al. Citation2004; Turner Citation2005) and allow for mapping structural modifications of land cover, such as farmland parcelization, are therefore needed.
Image texture measures tonal variations in the spatial domain by quantifying the variability and spatial distribution of grey level values (Baraldi and Parmiggiani Citation1995; Chica-Olmo and Abarca-Hernandez Citation2000). Because structural information can be important to discriminate between land cover categories, texture measures have widely been used in land cover classifications (Berberoglu, Lloyd, Atkinson, and Curran Citation2000; Presutti, Franklin, Moskal, and Dickson Citation2001). For example, texture measures improve classification of forests (Franklin, Hall, Moskal, Maudie, and Lavigne Citation2000; Coburn and Roberts Citation2004), urban areas (Dekker Citation2003) and agricultural crops (Anys and He Citation1995; Lloyd et al. Citation2004). Texture measures have much less frequently been used to derive continuous, quantitative variables, and the existing studies have mostly assessed vegetation structure in natural ecosystems (Wulder, Ledrew, Franklin, and Lavigne Citation1998; Asner, Keller, Pereira, and Zweede Citation2002; Asner, Bustamante, and Townsend Citation2003). However, to our knowledge no study used texture to map field sizes. This is unfortunate because small fields likely result in high local heterogeneity because of the variability in crop types and phenology, whereas large fields are locally homogeneous. Measures of spatial autocorrelation are sensitive to these field-size-dependent textural characteristics (Lloyd et al. Citation2004; Ozdogan and Woodcock Citation2006). Image texture, therefore, should be able to quantify differences in land use patterns and provide an indicator of field size.
While we are not aware of any image-texture-based study assessing field size in Eastern Europe, two prior studies used different mapping approaches to map field size in Eastern Europe from remote sensing images. Visual interpretation of a 1998 Landsat Thematic Mapper (TM) image provided mean field size for six types of villages in southeast Poland and showed that traditional villages had much smaller fields compared to villages with more intensive land use (Angelstam, Boresjo-Bronge, Mikusinski, Sporrong, and Wastfelt Citation2003). Similar visual interpretation in Albania revealed widespread parcelization between 1988 and 2003 (Müller and Munroe Citation2007). Thus, existing studies were confined to small study regions within single countries. No study mapped field size from remote sensing images for larger areas in Eastern Europe or has compared land use patterns among countries.
Our goal was to map field sizes in 2000 in a study area in Eastern Europe (the border triangle of Poland, Slovakia and Ukraine) using image texture. Our specific objectives were to:
-
build a statistical model that relates field size and image texture;
-
apply this model to our study area to map field size; and
-
compare land use patterns among countries in our study region.
2. Study region
We studied field sizes in the border triangle of Poland, Slovakia and Ukraine (). The boundaries of the study region were based on the extent of one Landsat TM scene (path/row 186/26), landscape features such as rivers and valleys, and administrative boundaries. The 17,800 km2 study region is characterized by a moderately cool and humid climate (average annual temperature of 5.9°C, mean precipitation of 1100–1200 mm, Augustyn Citation2004). Bedrock is largely dominated by Carpathian flysh (sandstone and shale) (Denisiuk and Stoyko Citation2000), but some andesite-basalts occur in the southwest of the study area (Herenchuk Citation1968). Dominating soils are cambisols together with podzols in the mountains, whereas podzoluvisols, greysems, gleysols and fluvisols dominate the plains. The region has mountainous topography (200–1480 m altitude). The three main altitudinal zones of potential natural vegetation are: the foothill zone (<600 m) dominated by broad-leaved forest, mostly beech (Fagus sylvatica) and oak (Quercus robur, Quercus petraea); the montane zone (600–1100 m) characterized by beech, mixed with silver fir (Abies alba) and sycamore (Acer pseudoplatanus); and alpine meadows with dwarfed beech above the timberline (1100–1200 m, Denisiuk and Stoyko Citation2000).
Figure 1. Top: Study area in the border region of Poland, Slovakia and Ukraine in the Carpathians. Bottom: Example of land use pattern in the three countries, namely Poland, Slovakia and Ukraine (Landsat Enhanced Thematic Mapper Plus image from 30 September 2000; band combination: red = band 4, green = band 5, blue = band 3). Available in colour online.
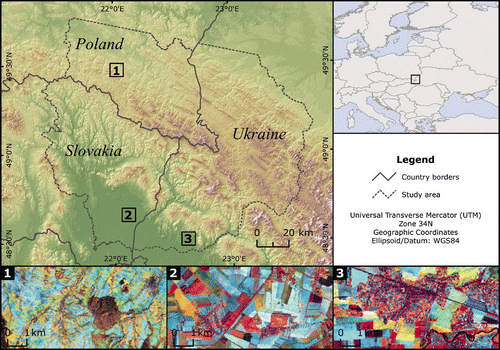
Land use change has substantially altered the study region, particularly in the nineteenth and twentieth centuries. Population growth and agricultural intensification resulted in increasing agricultural area, mainly at the expanse of forests (Turnock Citation2002; Augustyn Citation2004). Today, the densely settled plains and the foothills of the study region are farmed (Kuemmerle, Hostert, Perzanowski, and Radeloff Citation2006). Forests dominate the montane zone (>60%, Kuemmerle et al. Citation2006), but farmland and pastures are widespread in mountain valleys, particularly in Slovakia and Ukraine, where population density is much higher than in Poland (Augustyn Citation2004). Growing season length varies with altitude (from more than 270 days below 500 m to less than 220 days above 800 m) (Zarzycki and Glowacinski Citation1970). Dairy farming and cattle breeding are important agricultural activities. Cereals (e.g. winter wheat, buckwheat), oil crops (e.g. rape, sunflowers), flax, corn and potatoes are cultivated in the plains. Agriculture is an important source of income, but most of the agricultural goods are produced for local markets. Moreover, many land owners depend on subsistence farming, particularly in Ukraine.
Almost all farmland in Slovakia and Ukraine was collectivized during socialism and managed in large, state-controlled agricultural enterprises. In Slovakia, cooperatives prevailed, and land owners retained property rights to their fields. In contrast, in Ukraine all land was owned by the state (Lerman Citation1999; Csaki, Lerman, Nucifora, and Blaas Citation2003), but in Poland, most farmland was never collectivized (Lerman et al. Citation2004). A special case were some parts of the Polish region of the study area that were forcefully depopulated following border changes between the Soviet Union and Poland in 1947, and these lands were transferred into state ownership (Turnock Citation2002; Augustyn Citation2004). After 1990, Slovakia, Poland and Ukraine launched land reforms to privatize farmland and to individualize land use; yet, the countries chose diverse land reform strategies (Lerman et al. Citation2004). As a result, the region has heterogeneous land use patterns and is particularly well suited to study how changes in land ownership and land management manifest themselves in land use pattern, and to explore the relationship between field size and image texture.
3. Data and methods
3.1. Data sets used
We acquired one Landsat TM (21 August 2000) and two Landsat Enhanced Thematic Mapper Plus (ETM + ) images (6 June 2000 and 30 September 2000) from path/row 186/26 to map post-socialist field size patterns in our study area. Precise co-registering is necessary for accurate analysis of multitemporal images (Coppin et al. Citation2004). We orthorectified the images and registered them to the Universal Transverse Mercator coordinate system (World Geodetic System 1984 datum and ellipsoid) (Hill and Mehl Citation2003; Kuemmerle et al. Citation2006). Radiometric correction based on radiometric transfer models was carried out to minimize the effect of differing atmospheric and illumination conditions among images (Hill and Mehl Citation2003; Kuemmerle et al. Citation2006). Thermal bands were not retained because of their lower spatial resolution. Three IKONOS images and 12 Quickbird images available via Google Earth (http://earth.google.com) were used to digitize farm fields as training samples (see Section 3.2). All images had been acquired between 2002 and 2006, and together covered an area of 2890 km2 (16% of the study area). The IKONOS images were georectified by us, whereas the Quickbird images were already orthorectified. All images were pan-sharpened, with a spatial resolution of 1 m for the Ikonos data and 0.67 m for the Quickbird images.
3.2. Sample of fields
To derive ground truth data, we digitized farm fields from IKONOS and Quickbird images for 35 independent sample plots, where each sample plot consisted of many fields. Sample locations were determined at random, maintaining a minimum distance of 1 km between sample plots to reduce potential effects of spatial autocorrelation. This distance was chosen based on the range of positive spatial autocorrelation in semi-variograms of selected texture measures (see Section 3.3). Variograms were based on 1000 random locations, directional variograms were used to account for potential directionality, and Gaussian variogram models were fitted to estimate the range.
Field size differed greatly in the study area (<0.1 to >100 ha) and deciding upon the size of the sample plots carefully was therefore important. We defined a sample plot as a fixed number of fields, rather than a fixed area because no single sample plot size would have been well suited to all conditions. To determine the necessary number of fields, we evaluated how mean field size changed as a function of the number of fields. We did so for two circular test areas, one with very small fields (Poland, 151 ha, n = 331 fields), and one with large fields (Slovakia 1787 ha, n = 173 fields). The locations of these test areas were determined based on field visits and expert knowledge. Fields were consecutively added to the calculation of the mean, based on their centroid's distance to the centre point of the test area. The curves of both test areas suggested that mean field size became stationary after approximately 30 fields were included (data not shown). We therefore digitized the 30 fields from the IKONOS and Quickbird images that were closest to the centre of each of our 35 sample plots. We used less than 30 fields if sample plot size exceeded 200 ha (including the field necessary to reach this limit). The area covered by a single sample plot ranged from 9 to 262 ha. Non-farmland was not digitized and we digitized a total of 770 fields.
3.3. Texture measures
Texture measures quantify heterogeneity in the spatial distribution of grey tone values within a local neighbourhood, either based on the first-order (occurrence) or second-order (co-occurrence) grey level histogram (Haralick, Shanmuga and Dinstein Citation1973; Anys and He Citation1995). Different texture measures capture different aspects of spatial and spectral variability. Their values also partly depend on the size of the moving window used to calculate them (Anys and He Citation1995; Berberoglu and Curran Citation2004). We selected 13 texture measures that are relevant to describe land cover features (Anys and He Citation1995; Berberoglu and Curran Citation2004), including many with low collinearity (Baraldi and Parmiggiani Citation1995; St-Louis, Pidgeon, Radeloff, Hawbaker, and Clayton Citation2006). The set of texture measures that we chose included five occurrence measures (range, mean, variance, entropy and skewness), and eight co-occurrence measures (second mean, sum of squares variance, homogeneity, contrast, dissimilarity, second entropy, angular second moment and correlation) (Haralick et al. Citation1973; Anys and He Citation1995). The grey level co-occurrence matrix was calculated unidirectionally (135°), because field visits and directional variograms did not suggest any particular textural orientation of farmland crops in our study area.
Texture measures were calculated for each of the six multispectral TM/ETM + bands. We calculated texture measures for the June, August and September images to test for phenology effects. We calculated the 13 texture measures for seven window sizes (3, 5, 7, 9, 15, 21 and 51 pixels). In total, we calculated 1638 texture measures (18 bands [three images] × 13 texture measures × seven window sizes). To relate field size to texture measures at the sample plot level, we summarized texture by calculating mean and standard deviation of all pixels within a sample plot for each texture measure. The mean denotes the average texture for each sample plot, whereas the standard deviation texture is a measure of variability of texture per sample plot. All texture measures were calculated using ENVI/IDL image processing software (RSI Citation2003).
3.4. Statistical analysis
We fitted regression models to explore the relationship between field size and image texture. As the dependent variable (Y), we used mean field size per sample plot. Histograms and normal quantile–quantile (QQ) plots suggested a lognormal distribution, and we transformed field size to a normal distribution using the common logarithm (log 10). We used sample plot level mean and standard deviation texture as independent variables (X j). Univariate and multiple regression models were fitted to determine texture measures that were good predictors of field size according to
We derived the best one-, two- and three-dimensional models for each of the groups of variables described above. Several combinations of texture measures were expected to predict field size equally well because of collinearity among some of our input variables. This collinearity is not necessarily disadvantageous when using a best subsets model selection routine, because all possible models with n covariates are compared. Models based on covariates with low collinearity likely explain more total variance than models with collinear covariates. To compare among models, we calculated two measures of goodness of fit: the adjusted coefficient of determination (R 2) and the Bayesian Information Criterion (BIC, Schwarz Citation1978). Both measures account for the number of covariates in the model, thus allowing comparison of models of different dimensionality. Given any two estimated models, the model with the lowest BIC and the highest adjusted R 2 was preferred. We assumed that models performed equally well if their adjusted R 2 values differed by less than 0.02 (equalling a BIC difference of ∼3). We also calculated the p-value for all coefficients to evaluate their significance. To test the robustness of our models, we calculated cross-validation prediction errors using a leave-one-out procedure and a five-fold cross-validation for the best univariate and multiple regression models (Burman Citation1989). We also controlled for the presence of spatial autocorrelation in the residuals in our best multiple regression models based on variograms and found no spatial autocorrelation. All statistical analyses were carried out using R 2.4.1 (R Development Core Team 2006).
3.5. Applying the model to images
Once the best mean texture models (group I) were selected, we applied them to the entire study area to derive a map of field size. Models that used standard deviation texture (group II) performed better. However, we could not apply these models to full images, because the different sizes of the sample plots inhibited the spatially explicit estimation of standard deviation texture. All forests, water bodies and settlements were masked out using previous land cover classifications (Kuemmerle et al. Citation2006; Kuemmerle, Hostert, Radeloff, Perzanowski, and Kruhlov Citation2007). We excluded all areas above 1000 m elevation because farmland does not occur above this altitude in the Carpathians. Clouds in the September 2000 image (0.02% of the study area) were masked out. The best mean texture models (group I) were applied to all unmasked pixels to derive a map of field size for the year 2000. Our pixel-based models do therefore not allow for mapping the size or boundaries of single fields but rather estimate mean field size for the surroundings of a given pixel. Because field size was log-transformed, outliers resulted in unrealistically small or large field sizes. To account for this, we used a 5% cutoff at the extreme ends of the field size distribution.
4. Results
4.1. Statistical models
Texture measures explained the majority (i.e. up to 93%) of the variability in field size. Generally, models based on mean and standard deviation texture (group II models) explained more variability in field size than models based on mean texture only (group I models) ( and ). Multiple regression models using two or three independent variables predicted field size substantially better than univariate models. The increase in adjusted R 2 was strongest from one- to two-dimensional models with an average of 0.17 (range: 0.09–0.29) for models that used mean texture (group I models, ), and 0.12 (range: 0.04–0.24) for models using mean and standard deviation texture (group II models, ). Adjusted R 2 values improved less when adding a third covariate (on average 0.07 for both group I and group II models). All coefficients in the univariate models were highly significant (p < 0.0001). The significance of some coefficients decreased in the two- and three-dimensional models, but all coefficients were significant at p < 0.05 ( and ).
Table 1. Goodness-of-fit measures and regression coefficients p-value of the best models obtained for different combinations of mean texture measures and window sizes (group I models only)
Table 2. Goodness-of-fit measures and regression coefficients p-value of the best models obtained for different combinations of mean and standard deviation texture measures and window sizes (group II models only)
Some texture measures predicted field size better than others. First-order entropy was the best single predictor of field size (), and most of the best univariate models were based on either first or second-order entropy, or angular second moment. The measures used in the best univariate models were highly collinear (e.g. a correlation coefficient of 0.99 between first and second-order entropy). In the multiple regression models, these measures were complemented by first and second-order mean, variance and correlation (). Some texture measures were rarely included in the two- and three-dimensional models (e.g. homogeneity, contrast, and dissimilarity). Texture measures included in one of the best multiple regression models all displayed a low degree of collinearity (e.g. correlation coefficients of <0.10 between mean and entropy).
Table 3. Example of the number of times each texture measure was included in the series of regression models containing one (n = 1), two (n = 2 models) or three (n = 33) covariates that performed equally well (i.e. difference in adjusted R 2 < 0.02) for mean texture of June 2000 (window size 3, total number of variables = 78)
Figure 2. Example of an area characterized by heterogeneous land use pattern in high-resolution Quickbird data (left), June 2000 Landsat ETM + data (first principal component, middle), and image texture derived from the Landsat image (first-order entropy of band 7 calculated at a window size of 3 pixels, right). The subset is centred on the village of Bezovce in Slovakia (21.15E, 48.63N).
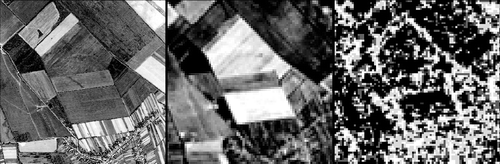
Goodness of fit varied strongly among Landsat bands used to derive the texture measures, but model predictions were similar for collinear Landsat TM/ETM + bands (e.g. bands in the visual domain). Most texture measures included in our best models were based on short wavelength infrared (SWIR) and visible bands (). The SWIR bands were particularly important for the univariate mean texture models (group I); whereas texture measures based on the visible bands were mostly included in the univariate group II and in the two- and three-dimensional models. As expected because of the collinearity among different texture measures and Landsat bands, several models performed equally well (i.e. difference of adjusted R 2 < 0.02). The number of similar models was generally lower for group I models compared to group II models and increased with the number of covariates allowed ( and ).
The goodness of fit of the regression models varied among window sizes and was best at small window sizes ( and ). Our univariate models revealed that most texture measures had a clear peak in goodness of fit at small or intermediate window sizes and R 2 values decreased rapidly for larger window sizes (). Combining texture measures from different window sizes did not substantially improve model predictions (i.e. increase in adjusted R 2 < 0.02), both for group I models (), and for group II models ().
Figure 3. Examples of the relationship between prediction accuracy (R 2 of field size vs. texture measures) and window size used to calculate texture measures. Mean field size was estimated using mean texture (left) and standard deviation of texture (right).
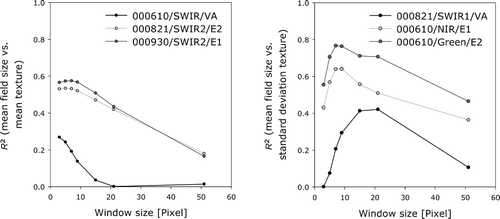
Comparing regression models based on texture measures from different images revealed moderate differences in goodness of fit. For group I models, texture measures from the June and September images yielded higher model predictions than models based on the August image (), but combining texture measures from all three images did not increase goodness of fit substantially. This was different for group II models. Goodness of fit was comparable among the three dates, however, predictions improved when combining texture from different images (). Our best model explained 93% of the variance and used three covariates: mean angular second moment (September 2000 image, TM band 6), standard deviation of first-order entropy (June 2000, band 1) and standard deviation variance (August 2000, band 3); all calculated for a window size of 9 pixels ().
Table 4. Regression coefficients of the variables included in the best models and of the intercept for each subgroup (bold models in and )
Among all the models fitted, we selected the best one-, two- and three-dimensional models for group I and group II based on the adjusted R 2 and BIC statistics, and chose only models where all coefficients were significant (p < 0.1). In cases where several models performed equally well (i.e. difference in adjusted R 2 < 0.02), we selected the model that was derived using a smaller selection of input variables, resulting in six best models (). Cross-validation for these six models (bold models in and ) showed that the robustness of the multiple regression models was relatively high (). Prediction errors of the multiple regression models were substantially lower than those of the univariate models (by a factor of 2–3). Errors ranged from 0.20 to 1.41 (log field size) and were lower for group II compared to group I models. The prediction errors were similar when using a leave-one-out strategy or a five-fold cross-validation approach ().
Table 5. Mean prediction errors of mean field size (log) for the one-, two- and three-dimensional group I (mean texture) and group II (mean and standard deviation texture) models
4.2. Applying the model to images
We used the absolute best two- and three-dimensional mean texture models (group I) based on the adjusted R 2 to map field size in our study area. Because our ultimate goal was to predict field size, we did not have to consider other equally good models. The optimal two-dimensional model used two September 2000 texture measures calculated at a window size of 15 pixels (second-order mean and correlation from band 3) and explained about 74% of the variance in field size at the sample plot level (). The absolute best three-dimensional mean texture model relied on three September 2000 texture measures derived for a window size of 7 pixels (variance of TM band 1, correlation of band 2 and second-order mean of band 3) and had an adjusted R 2 of 0.84 (). Applying these best two- and three-dimensional models to all unmasked pixels of the September 2000 image yielded field sizes between 0.07–142 ha and 0.04–1565 ha, respectively (10th and 90th percentile of all estimated pixels). The field size map revealed diverse spatial patterns of field size across our study region, and maps from the two-dimensional and three-dimensional models were highly similar (). Large fields dominated the plains in the north and south, whereas mountain valleys were dominated by small fields. Field visits and visual comparison with the Quickbird and IKONOS images confirmed these patterns.
Figure 4. Field size map of the border region of Poland, Slovakia and Ukraine for the year 2000. Top: map derived using the best two-dimensional mean texture model. Bottom: map derived using the best three-dimensional mean texture model. The three-dimensional map is shown using the colour scheme of the two-dimensional map for better comparison. Small map insert indicates pixel-level differences in predicted field size between the two- and three-dimensional model (Coordinate System: UTM/Zone 34N; Ellipsoid/Datum: WGS84).
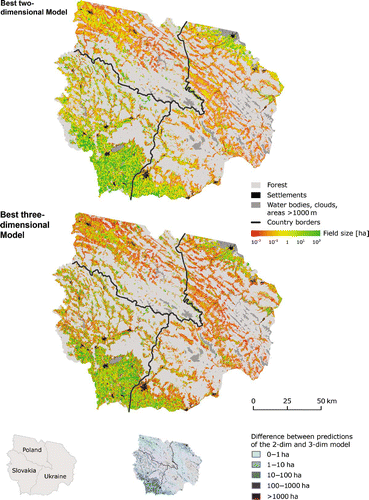
Field size patterns in Poland, Slovakia and Ukraine differed markedly. Poland had small fields in most areas (), but some large fields (>1 ha) occurred in the valleys along the Polish–Slovak border and in the northwest of the study area (). In Slovakia, field sizes were substantially larger than in the other two countries (). In particular, the southern plains were characterized by very large fields, often exceeding 100 ha. Mountain valleys had a mix of large and small fields, with valleys in the north exhibiting a higher percentage of large fields than valleys in the south. Ukraine showed the most heterogeneous patterns of field sizes. Although the overall distribution of field sizes was similar to Poland's distribution (), small and large fields were much more clustered in Ukraine. Mountain valleys were characterized by very small fields (<0.1 ha, ). Large fields were mainly found in the northern and southern plain, but the pattern was more heterogeneous than in Slovakia, and clusters of large and small fields occurred next to each other. Very small fields occurred often in the vicinity of larger settlements ().
Figure 5. Distribution of field sizes for the Polish, Slovak and Ukrainian region of the study area. Whiskers indicate the 90th and 10th percentiles.
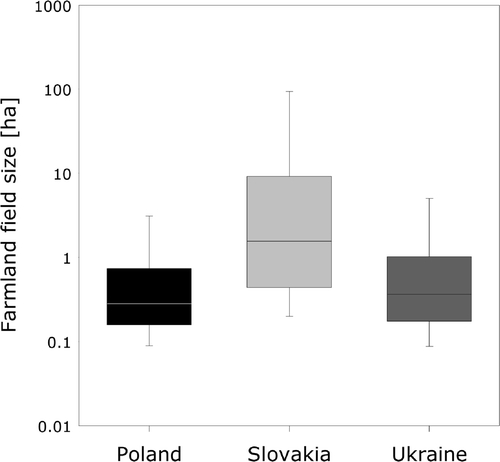
Field size co-varied with altitude in all three countries (). In Poland, fields were smaller at low altitudes and increased with elevation. In Slovakia and Ukraine, field sizes were much larger at lower altitudes compared to intermediate altitudes. At higher altitudes, areas of small and large fields occurring side by side in Slovakia, whereas field size consistently decreased along the altitudinal gradient in Ukraine, and the highest mountain valleys there displayed smallest fields (). Three field visits (summer 2004, spring 2005 and spring 2006) included all three countries and confirmed the plausibility of the land use patterns in our field size maps.
5. Discussion
5.1. Mapping parcelization using texture measures
We found a strong relationship between field size and Landsat TM/ETM + texture measures and we used our models to map field size patterns for our full study region. We therefore suggest that texture measures bear considerable potential to map land use patterns and changes therein. This may be especially important in areas that are undergoing rapid change and where alternative data sources (e.g. cadastral maps) are not readily available or of unknown reliability, such as in Eastern Europe and the former Soviet Union.
Our predictions of field size varied based on the texture measures used, but some clear patterns emerged. As expected, the best predictors of field size were texture measures related to the local heterogeneity of grey level values. However, different texture measures quantify different aspects of this heterogeneity. We found measures that characterize the ‘orderliness’ of an image (i.e. regular distribution of grey values, Hall-Beyer 2007), such as entropy and the second angular moment, to be most sensitive to variations in field size. Entropy measures the degree of disorder of grey level values (first-order entropy) or grey level value pairs (second-order entropy) (Anys and He Citation1995; Baraldi and Parmiggiani Citation1995; Anys, Bannari, He, and Morin Citation1998). Angular second moment (sometimes also referred to as energy) is strongly, but inversely related to entropy, and measures the uniformity of an image (Haralick et al. Citation1973; Gong, Marceau, and Howarth Citation1992; Baraldi and Parmiggiani Citation1995). Farmland fields, patches of similar grey values, are often organized in distinct geometric patterns (e.g. along valleys, or perpendicular to roads to provide easier access to farmers). This likely explains why measures such as entropy and angular second moment predicted field size best.
Variance, correlation, and first- and second-order mean were, in addition to the above measures of orderliness, often included in the best multiple regression models (two or three covariates). Variance describes the variability of grey level values within a given window (Haralick et al. Citation1973). In other words, variance directly relates to our underlying hypothesis that local heterogeneity is highest where small fields dominate. Correlation is a measure of grey level linear dependency in an image (Haralick et al. Citation1973) and uncorrelated to the measures of orderliness. Linear dependencies are characteristic for agricultural land use patterns (i.e. farmland fields are often rectangular), thereby explaining the sensitivity of the correlation feature towards field size. First and second-order mean (the average or expected combination of two co-occurring grey level values within a window) both relate to purely spectral rather than textural characteristics. In univariate models, these measures predicted field size poorly. However, first and second-order mean based on bands from the visible domain were frequently included in our best multiple regression models, likely because they provided additional information for separating soil and vegetation patches (i.e. fields in agricultural areas).
Some texture measures predicted field size poorly and were not included in any of the best models. Particularly, measures that quantify image contrast (e.g. dissimilarity, contrast or homogeneity) yielded lower predictions than those measures that quantify the organization of contrasting features (i.e. image orderliness). The weak relationship between contrast measures and field size was not surprising, because the degree of image contrast is not related to particular land use patterns. Moreover, contrast measures are particularly sensitive to periodic features in an image (Baraldi and Parmiggiani Citation1995). In agricultural landscapes with many different crop types and bare fields, such reoccurring patterns are scarce. Other measures that were poor predictors of field size included statistical parameters that are not related to the spatial organization of grey level values (e.g. histogram skewness).
Selecting an appropriate window size is a crucial step when characterizing image features based on texture (Anys and He Citation1995). Texture measures calculated using intermediate window sizes (e.g. 7, 9 or 15 pixels) yielded the best field size predictions ( and ). At such window sizes, many small fields (e.g. in areas of subsistence farming) are found within a chosen window, and result in high local heterogeneity. Large fields, on the other hand, were still relatively homogeneous at such window sizes. These differences translated into distinct textural characteristics that were useful to map field size (Ozdogan and Woodcock Citation2006). Most texture measures displayed a clear peak in predictions at these intermediate window sizes, and decreased rapidly for larger windows. This also indicated that the range of window sizes tested was sufficient.
Landsat bands in different spectral domains predicted field size differently. The SWIR bands and the bands in the visible domain captured much of the variation in farm fields, making texture measures calculated from these bands highly suited for field size mapping. The SWIR bands are particularly sensitive to variations in moisture content, and are important for mapping agricultural areas, for separating senescent and green vegetation, and to differentiate soils types (Cohen and Goward Citation2004). The visible bands are especially helpful to separate vegetation and bare soil. On the other hand, senescent vegetation and soils are spectrally relatively similar in the near-infrared domain, thus explaining the lower predictions from texture measures based on the NIR band. Predictions from texture measures calculated from the SWIR and visible bands were fairly comparable, suggesting that senescent vegetation/soil discrimination was more important than separating senescent and green vegetation to map field size in our case.
Several combinations of texture measures, Landsat TM/ETM + bands, and window sizes resulted in comparable predictions of field size in both univariate and multiple regression models. This was expected, because some Landsat TM/ETM + bands are highly collinear (Small Citation2004), several of our texture measures are strongly correlated (Baraldi and Parmiggiani Citation1995; St-Louis et al. Citation2006), and texture measures calculated using similarly sized windows did not differ substantially (). Being conservative in the number of covariates included in our models was therefore important. We used a maximum number of three covariates and the best subsets procedure was effective in selecting only variable combinations that displayed a low degree of collinearity (e.g. correlation coefficients among the variables in the best three-dimensional mean texture (group I) model were 0.20, 0.31 and −069). We suggest that the strong collinearity among some of our input variables did not hinder our methodology, but simply led to a higher number of models that predicted field size equally well. Predicting field size likely does not depend on the exact combination of texture measures, window sizes and Landsat bands. This is an advantage for transferring our methodology to other regions, because testing all possible combinations of input parameters is not necessary to find a model with similar goodness of fit than the absolute best model. We also suggest that reducing the dimensionality of the feature space (e.g. principal component transformation) may not be necessary, because the leaps procedure effectively selects variable combinations that explain the total variance best. We tested our models using the first three principal components per image instead of the original six Landsat TM/ETM + bands, but this did not improve model predictions (results not shown).
Model predictions were fairly stable for comparable input variable selections from different images throughout the year, particularly for multiple regression models that used standard deviation texture. For mean texture models, the autumn image (September) yielded higher predictions, likely because of the presence of green vegetation, senescent crops, harvested fields and bare soil. This spectral diversity of crop types resulted in higher local heterogeneity where land use patterns are dominated by small fields, and therefore a possible explanation for better predictions compared to the June and August images, where crop types are spectrally more homogeneous. However, the difference in goodness of fit among models from different images was relatively small (i.e. difference in adjusted R 2 < 0.06). Using combinations of input variables from different images did not improve model predictions substantially. We therefore suggest that a single image suffices to predict field sizes from texture measures.
Applying the multiple regression models fitted at the sample plot level to our full study region was successful (). Both the two- and three-dimensional models yielded comparable patterns of field size for our study region. A disadvantage was the log-transformed nature of the dependent variable, which exponentially amplified outliers in the texture measures (e.g. because of errors of commission in the water and settlement masks), particularly in the map generated from the three-dimensional model. Cutting the extreme ends of the field size distribution partly addressed this problem, but this approach requires expert knowledge regarding the possible range of field sizes. Unrealistically high and low field sizes in the map generated from the three-dimensional model may also indicate over-fitting. However, only a very small fraction of the study region was affected (predictions of <0.01 ha for ∼3% of the study area; >300 ha for ∼2% of the study area) and the cross-validation results did not suggest over-fitting. The robustness of our multiple regression models was also confirmed by the low cross-validation errors.
Our results show that image texture is a useful tool to map field size for areas with a high proportion of mixed pixels as well as for areas with very large fields. The field size maps proved useful to identify land use patterns and to compare these patterns among countries. We therefore suggest that texture has significant potential to monitor agricultural intensification and changes in land use patterns in Eastern Europe and in other regions of the world. Because texture is easily derivable from raw image data, it may represent an important variable (Southworth et al. Citation2004; Turner Citation2005) to assess landscape structure and land dynamics based on the spatial domain, and to assess structural land cover modifications in human-dominated landscapes. Moreover, land use pattern information is important to understand the relationship between land tenure and land use change. Incorporating land use patterns in land use change models has so far largely been based on cadastral maps (Verburg Citation2006). Such data are unavailable in many regions of the world, particularly those that experience rapid land use change, and field size variables based on image texture may be a useful alternative.
5.2. Field size in the border triangle of Poland, Slovakia and Ukraine
We found marked differences in field size among the Polish, Slovak and Ukrainian region of our study area. The study area was part of the Austro-Hungarian Empire for approximately 150 years before 1914. During that time, land management was relatively homogeneous (Turnock Citation2002). Today's differences in field size among countries therefore likely originated in socialist and post-socialist times. In our case, these differences are related to land-ownership patterns and land management in socialist times, combined with different strategies to re-privatize farmland and individualize land use in the post-socialist period.
Poland had the smallest field sizes, particularly in areas below 500 m elevation. The possible reason is that Poland was the only Eastern European country where collectivization failed (Van Dijk Citation2003; Lerman et al. Citation2004), small family farms persisted, and fields were never aggregated (Lerman et al. Citation2004). However, the exceptions were Polish mountain valleys, where border changes between the Soviet Union and Poland after 1947 led to a depopulation and the establishment of large-scale, state-owned farms (Turnock Citation2002; Augustyn Citation2004). After 1990, private farmland changed little, whereas state land was auctioned off, set aside, or converted to forest (Augustyn Citation2004). Our results show the largest fields in the Polish part of our study area at altitudes above 500 m, mostly clustered in the mountain valleys close to the border with Slovakia ().
In Slovakia, all farmland became collectivized in socialist times and small farms were dissolved into large-scale, state-controlled agricultural enterprises (Lerman Citation2001; Csaki et al. Citation2003). Although land owners retained the title to their land, land was managed by the state (Van Dijk Citation2003; Lerman et al. Citation2004). After 1990, Slovakia privatized the agricultural sector and restituted farmland to former owners, but this has not led to widespread parcelization and farming often continues on large fields (Trzeciak-Duval Citation1999; Csaki et al. Citation2003). This is reflected in our results by the high share of large fields, particularly in the southern plains. In such areas, socialistic land use patterns were effectively preserved (Mathijs and Swinnen Citation1998). Likely explanations are the relatively slow pace of reform (Csaki et al. Citation2003) and Slovakia's land owners, who often chose to lease their land to the successor organizations of the former cooperatives (Trzeciak-Duval Citation1999; Lerman Citation2001). In Slovak mountain valleys, household fields occur next to fields managed by large-scale agricultural enterprises. Moreover, farmland abandonment was widespread in Slovak mountain valleys resulting in relatively large, homogeneous areas, thus explaining the occurrence of very large fields in these areas.
In Ukraine, we found heterogeneous patterns of field size, and strong differences between mountain valleys and the plains in the north and south. In socialistic times, all farmlands in Ukraine were state owned and managed in large-scale farming enterprises (Ash and Wegren Citation1998; Lerman Citation2001). After the breakdown of the Soviet Union in 1991, Ukraine chose to distribute farmland among the workers of the state farms and collectives (Lerman et al. Citation2004). Land reform, however, was slow and much of the farmland is still managed by large-scale successor organizations. As a consequence, we found clusters of large fields, particularly in the plains. Parcelization occurred in some areas (Ash and Wegren Citation1998; Lerman et al. Citation2004) and we found evidence of parcelization in the vicinity of larger settlements, where people use farmland for subsistence farming, and land is accessible and potentially more valuable. Mountain valleys were almost exclusively characterized by very small field sizes, because Ukrainian mountain valleys have a high population density, and many people depend on subsistence farming.
6. Conclusions
Field size is an important indicator of land use patterns and changes therein, but there is a lack of methods to map field size from remote sensing images. Our results showed that image texture may have great potential to fill this gap. We found a strong relationship between Landsat-based texture measures and field size, and our models explained up to 93% of the variability in field size. Our best models relied on entropy and the second angular moment calculated at intermediate window sizes (7 × 7 to 15 × 15 pixel windows) on the visible or SWIR bands, but field size predictions were relatively independent from the exact combination of texture measures, window sizes and Landsat bands used. Our method allowed us to map field size for our entire case study area and image texture may thus be a reliable continuous indicator to map structural land cover modifications.
Studying land use patterns in areas that are undergoing political and economic transitions allows the assessment of the effects of changing institutions, land management policies, and land ownership on land use. Land use patterns differed markedly among the Polish, Slovak and Ukrainian regions of our study area. These differences were likely related to land ownership and land management in socialist times as well as dissimilarities in land reform strategies after the fall of the Iron Curtain in 1989. Land use patterns were highly fragmented where land ownership was private during and after socialism (Poland) and where farmland was distributed after the system change (Ukraine), but large fields dominated areas where farmland had been restituted to former owners (Slovakia). Image texture, which can easily be derived from raw remote sensing images, allows the quantification of these differences. Thus, image texture may be an important variable to better understand the relationship between land tenure and land use change and for incorporating land use pattern information in models of land use change. This may be particularly valuable in areas of the world where cadastral maps are currently unavailable. Image texture has large untapped potential to contribute to an improved understanding of the spatial extent, causes and consequences of land cover modifications, such as the post-socialist parcelization of farmland in Eastern Europe.
Acknowledgements
We are grateful to A. Reenberg and L. Jorgensen for editing this special issue. We also thank P.C. Alcántara Concepción, M. Dubinin, A. Janz, N.S. Keuler, D. Müller, A. Prishchepov and S. Schmidt for valuable discussions and helpful comments on prior versions of this manuscript. We express gratitude to A. Damm and A. Janz for programming assistance and to T. Schiller for helping with the data processing. This research would not have been possible without support by NASA's Land Cover and Land Use Change (LCLUC) Program and by the International Office of Humboldt-Universität zu Berlin.
References
- Angelstam , P. , Boresjo-Bronge , L. , Mikusinski , G. , Sporrong , U. and Wastfelt , A. 2003 . Assessing Village Authenticity with Satellite Images: A Method to Identify Intact Cultural Landscapes in Europe . Ambio , 32 ( 8 ) : 594 – 604 .
- Anys , H. , Bannari , A. , He , D. C. and Morin , D. 1998 . Zonal Mapping of Urban Areas Using MEIS-II Airborne Digital Images . International Journal of Remote Sensing , 19 ( 5 ) : 883 – 894 .
- Anys , H. and He , D. C. 1995 . Evaluation of Textural and Multipolarization Radar Features for Crop Classification . IEEE Transactions on Geoscience and Remote Sensing , 33 ( 5 ) : 1170 – 1181 .
- Ash , T. N. and Wegren , S. K. 1998 . “ Land and Agricultural Reform in Ukraine ” . In Land Reform in the Former Soviet Union and Eastern Europe , Edited by: Wegren , S. K. 48 – 67 . London and New York : Routledge .
- Asner , G. P. , Bustamante , M. M.C. and Townsend , A. R. 2003 . Scale Dependence of Biophysical Structure in Deforested Areas Bordering the Tapajo's National Forest, Central Amazon . Remote Sensing of Environment , 87 ( 4 ) : 507 – 520 .
- Asner , G. P. , Keller , M. , Pereira , R. and Zweede , J. C. 2002 . Remote Sensing of Selective Logging in Amazonia: Assessing Limitations Based on Detailed Field Observations, Landsat ETM + , and Textural Analysis . Remote Sensing of Environment , 80 ( 3 ) : 483 – 496 .
- Asner , G. P. , Knapp , D. E. , Broadbent , E. N. , Oliveira , P. J.C. , Keller , M. and Silva , J. N. 2005 . Selective Logging in the Brazilian Amazon . Science , 310 ( 5747 ) : 480 – 482 .
- Augustyn , M. 2004 . Anthropogenic Changes in the Environmental Parameters of the Bieszczady Mountains . Biosphere Conservation , 6 ( 1 ) : 43 – 53 .
- Baraldi , A. and Parmiggiani , F. 1995 . An Investigation of the Textural Characteristics Associated with Gray-Level Cooccurrence Matrix Statistical Parameters . IEEE Transactions on Geoscience and Remote Sensing , 33 ( 2 ) : 293 – 304 .
- Bennett , E. M. and Balvanera , P. 2007 . The Future of Production Systems in a Globalized World . Frontiers in Ecology and the Environment , 5 ( 4 ) : 191 – 198 .
- Benton , T. G. , Vickery , J. A. and Wilson , J. D. 2003 . Farmland Biodiversity: Is Habitat Heterogeneity the Key? . Trends in Ecology & Evolution , 18 ( 4 ) : 182 – 188 .
- Berberoglu , S. and Curran , P. J. 2004 . “ Merging Spectral and Textural Information for Classifying Remotely Sensed Images ” . In Remote Sensing Image Analysis: Including the Spatial Domain , Edited by: Van Der Meer , F. D. and De Jong , S. M. 113 – 136 . Dordrecht : Kluwer Academic Publishers .
- Berberoglu , S. , Lloyd , C. D. , Atkinson , P. M. and Curran , P. J. 2000 . The Integration of Spectral and Textural Information Using Neural Networks for Land Cover Mapping in the Mediterranean . Computers & Geosciences , 26 ( 4 ) : 385 – 396 .
- Burman , P. 1989 . A Comparative-Study of Ordinary Cross-Validation, v-fold Cross-Validation and the Repeated Learning-Testing Methods . Biometrika , 76 ( 3 ) : 503 – 514 .
- Chica-Olmo , M. and Abarca-Hernandez , F. 2000 . Computing Geostatistical Image Texture for Remotely Sensed Data Classification . Computers & Geosciences , 26 ( 4 ) : 373 – 383 .
- Cihlar , J. 2000 . Land Cover Mapping of Large Areas from Satellites: Status and Research Priorities . International Journal of Remote Sensing , 21 ( 6–7 ) : 1093 – 1114 .
- Coburn , C. A. and Roberts , A. C.B. 2004 . A Multiscale Texture Analysis Procedure for Improved Forest Stand Classification . International Journal of Remote Sensing , 25 ( 20 ) : 4287 – 4308 .
- Cohen , W. B. and Goward , S. N. 2004 . Landsat's Role in Ecological Applications of Remote Sensing . Bioscience , 54 ( 6 ) : 535 – 545 .
- Coppin , P. , Jonckheere , I. , Nackaerts , K. , Muys , B. and Lambin , E. 2004 . Digital Change Detection Methods in Ecosystem Monitoring: A Review . International Journal of Remote Sensing , 25 ( 9 ) : 1565 – 1596 .
- Csaki , C. , Lerman , Z. , Nucifora , A. and Blaas , G. 2003 . The Agricultural Sector of Slovakia on the Eve of EU Accession . Eurasian Geography and Economics , 44 ( 4 ) : 305 – 320 .
- Dekker , R. J. 2003 . Texture Analysis and Classification of ERS SAR Images for Map Updating of Urban Areas in the Netherlands . IEEE Transactions on Geoscience and Remote Sensing , 41 ( 9 ) : 1950 – 1958 .
- Denisiuk , Z. and Stoyko , S. M. 2000 . “ The East Carpathian Biosphere Reserve (Poland, Slovakia, Ukraine) ” . In Biosphere Reserves on Borders , Edited by: Breymeyer , A. and Dabrowski , P. 79 – 93 . Warsaw : UNESCO .
- Donald , P. F. , Pisano , G. , Rayment , M. D. and Pain , D. J. 2002 . The Common Agricultural Policy, EU Enlargement and the Conservation of Europe's Farmland Birds . Agriculture Ecosystems & Environment , 89 ( 3 ) : 167 – 182 .
- Elliott , L. J. , Mason , D. C. , Wilkinson , M. J. , Allainguillaume , J. , Norris , C. , Alexander , M. and Welters , R. 2004 . The Role of Satellite Image-Processing for National-Scale Estimates of Gene Flow from Genetically Modified Crops: Rapeseed in the UK as a Model . Journal of Applied Ecology , 41 ( 6 ) : 1174 – 1184 .
- Evans , C. , Jones , R. , Svalbe , I. and Berman , M. 2002 . Segmenting Multispectral Landsat TM Images into Field Units . IEEE Transactions on Geoscience and Remote Sensing , 40 ( 5 ) : 1054 – 1064 .
- Foley , J. A. , Asner , G. P. , Costa , M. H. , Coe , M. T. , Defries , R. , Gibbs , H. K. , Howard , E. A. , Olson , S. , Patz , J. , Ramankutty , N. and Snyder , P. 2007 . Amazonia Revealed: Forest Degradation and Loss of Ecosystem Goods and Services in the Amazon Basin . Frontiers in Ecology and the Environment , 5 ( 1 ) : 25 – 32 .
- Foley , J. A. , Defries , R. , Asner , G. P. , Barford , C. , Bonan , G. , Carpenter , S. R. , Chapin , F. S. , Coe , M. T. , Daily , G. C. , Gibbs , H. K. , Helkowski , J. H. , Holloway , T. , Howard , E. A. , Kucharik , C. J. , Monfreda , C. , Patz , J. A. , Prentice , I. C. , Ramankutty , N. and Snyder , P. K. 2005 . Global Consequences of Land Use . Science , 309 ( 5734 ) : 570 – 574 .
- Franklin , S. E. , Hall , R. J. , Moskal , L. M. , Maudie , A. J. and Lavigne , M. B. 2000 . Incorporating Texture into Classification of Forest Species Composition from Airborne Multispectral Images . International Journal of Remote Sensing , 21 ( 1 ) : 61 – 79 .
- Geist , H. J. and Lambin , E. F. 2002 . Proximate Causes and Underlying Driving Forces of Tropical Deforestation . Bioscience , 52 ( 2 ) : 143 – 150 .
- GLP (Global Land Project) . “Science Plan and Implementation Strategy,” . IGBP Report No. 53/IHDP Report No. 19, 64 . 2005 . IGBP, Stockholm
- Gong , P. , Marceau , D. J. and Howarth , P. J. 1992 . A Comparison of Spatial Feature-Extraction Algorithms for Land-Use Classification with Spot HRV Data . Remote Sensing of Environment , 40 ( 2 ) : 137 – 151 .
- Hall-Beyer, M. (2007), “The Grey-Level Co-occurence Matrix Tutorial.” http://www.fp.ucalgary.ca/mhallbey/tutorial.htm (Accessed: 15 May 2007 ).
- Haralick , R. M. , Shanmuga , K. and Dinstein , I. 1973 . Textural Features for Image Classification . IEEE Transactions on Systems, Man, and Cybernetics , SMC3 ( 6 ) : 610 – 621 .
- Herenchuk , K. I. 1968 . Pryroda Ukrayinskykh Karpat [Nature of the Ukrainian Carpathians] , Lviv : Vydavnytstvo Lvivskoho Universytetu [In Ukrainian] .
- Hill , J. and Mehl , W. 2003 . Geo- and Radiometric Pre-Processing of Multi- and Hyperspectral Data for the Production of Calibrated Multi-Annual Time Series . Photogrammetrie – Fernerkundung – Geoinformation (PFG) , 1 : 7 – 14 . [In German]
- Kaimowitz , D. , Thiele , G. and Pacheco , P. 1999 . The Effects of Structural Adjustment on Deforestation and Forest Degradation in Lowland Bolivia . World Development , 27 ( 3 ) : 505 – 520 .
- Kuemmerle , T. , Hostert , P. , Perzanowski , K. and Radeloff , V. C. 2006 . Cross-Border Comparison of Land Cover and Landscape Pattern in Eastern Europe Using a Hybrid Classification Technique . Remote Sensing of Environment , 103 : 449 – 464 .
- Kuemmerle , T. , Hostert , P. , Radeloff , V. C. , Perzanowski , K. and Kruhlov , I. 2007 . Post-Socialist Forest Disturbance in the Carpathian Border Region of Poland, Slovakia, and Ukraine . Ecological Applications , 17 ( 5 ) : 1279 – 1295 .
- Lambin , E. F. and Geist , H. J. , eds. 2006 . Land Use and Land Cover Change – Local Processes and Global Impacts , Berlin, Heidelberg, New York : Springer .
- Lambin , E. F. , Turner , B. L. , Geist , H. J. , Agbola , S. B. , Angelsen , A. , Bruce , J. W. , Coomes , O. T. , Dirzo , R. , Fischer , G. , Folke , C. , George , P. S. , Homewood , K. , Imbernon , J. , Leemans , R. , Li , X. B. , Moran , E. F. , Mortimore , M. , Ramakrishnan , P. S. , Richards , J. F. , Skanes , H. , Steffen , W. , Stone , G. D. , Svedin , U. , Veldkamp , T. A. , Vogel , C. and Xu , J. C. 2001 . The Causes of Land-Use and Land-Cover Change: Moving Beyond the Myths . Global Environmental Change-Human and Policy Dimensions , 11 ( 4 ) : 261 – 269 .
- Lerman , Z. 1999 . Land Reform and Farm Restructuring in Ukraine . Problems of Post-Communism , 46 ( 3 ) : 42 – 55 .
- Lerman , Z. 2001 . Agriculture in Transition Economies: From Common Heritage to Divergence . Agricultural Economics , 26 ( 2 ) : 95 – 114 .
- Lerman , Z. , Csaki , C. and Feder , G. 2004 . Evolving Farm Structures and Land-Use Patterns in Former Socialist Countries . Quarterly Journal of International Agriculture , 43 ( 4 ) : 309 – 335 .
- Lloyd , C. D. , Berberoglu , S. , Curran , P. J. and Atkinson , P. M. 2004 . A Comparison of Texture Measures for the Per-Field Classification of Mediterranean Land Cover . International Journal of Remote Sensing , 25 ( 19 ) : 3943 – 3965 .
- Mathijs , E. and Swinnen , J. F.M. 1998 . The Economics of Agricultural Decollectivization in East Central Europe and the Former Soviet Union . Economic Development and Cultural Change , 47 ( 1 ) : 1 – 26 .
- Matson , P. A. , Parton , W. J. , Power , A. G. and Swift , M. J. 1997 . Agricultural Intensification and Ecosystem Properties . Science , 277 ( 5325 ) : 504 – 509 .
- Mcconnell , W. J. and Keys , E. 2005 . “ Meta-Analysis of Agricultural Change ” . In Seeing the Forest and the Trees: Human-Environment Interactions in Forest Ecosystems , Edited by: Moran , E. F. and Ostrom , E. 325 – 354 . Cambridge : MIT Press .
- Mertens , B. , Sunderlin , W. D. , Ndoye , O. and Lambin , E. F. 2000 . Impact of Macroeconomic Change on Deforestation in South Cameroon: Integration of Household Survey and Remotely-Sensed Data . World Development , 28 ( 6 ) : 983 – 999 .
- Miller , A. 1990 . Subset Selection in Regression , London : Chapman & Hall .
- Müller , D. and Munroe , D. K. 2008 . Changing Rural Landscapes in Albania: cropland abandonment and forest clearing in the post-socialist transition . Annals of the Association of American Geographers , 98 ( 4 ) : 855 – 876 .
- Ozdogan , M. and Woodcock , C. E. 2006 . Resolution Dependent Errors in Remote Sensing of Cultivated Areas . Remote Sensing of Environment , 103 ( 2 ) : 203 – 217 .
- Penov , I. 2004 . The Use of Irrigation Water in Bulgaria's Plovdiv Region During Transition . Environmental Management , 34 ( 2 ) : 304 – 313 .
- Presutti , M. E. , Franklin , S. E. , Moskal , L. M. and Dickson , E. E. 2001 . Supervised Classification of Multisource Satellite Image Spectral and Texture Data for Agricultural Crop Mapping in Buenos Aires Province, Argentina . Canadian Journal of Remote Sensing , 27 ( 6 ) : 679 – 684 .
- R Development Core Team . 2006 . R: A Language and Environment for Statistical Computing , Vienna : R Foundation for Statistical Computing .
- Rindfuss , R. R. , Walsh , S. J. , Turner , B. L. , Fox , J. and Mishra , V. 2004 . Developing a Science of Land Change: Challenges and Methodological Issues . Proceedings of the National Academy of Sciences of the United States of America , 101 ( 39 ) : 13976 – 13981 .
- RSI . 2003 . IDL Reference Guide, IDL Version 6.0 , Boulder : Research Systems Inc .
- Sabates-Wheeler , R. 2002 . Consolidation Initiatives After Land Reform: Responses to Multiple Dimensions of Land Fragmentation in Eastern European Agriculture . Journal of International Development , 14 : 1005 – 1018 .
- Schwarz , G. 1978 . Estimating the Dimension of a Model . Annals of Statistics , 6 ( 2 ) : 461 – 464 .
- Seto , K. C. and Kaufmann , R. K. 2003 . Modeling the Drivers of Urban Land Use Change in the Pearl River Delta, China: Integrating Remote Sensing with Socioeconomic Data . Land Economics , 79 ( 1 ) : 106 – 121 .
- Silleos , N. , Perakis , K. and Petsanis , G. 2002 . Assessment of Crop Damage Using Space Remote Sensing and GIS . International Journal of Remote Sensing , 23 ( 3 ) : 417 – 427 .
- Small , C. 2004 . The Landsat ETM Plus Spectral Mixing Space . Remote Sensing of Environment , 93 ( 1–2 ) : 1 – 17 .
- Southworth , J. , Munroe , D. and Nagendra , H. 2004 . Land Cover Change and Landscape Fragmentation: Comparing the Utility of Continuous and Discrete Analyses for a Western Honduras Region . Agriculture Ecosystems & Environment , 101 ( 2–3 ) : 185 – 205 .
- St-Louis , V. , Pidgeon , A. M. , Radeloff , V. C. , Hawbaker , T. J. and Clayton , M. K. 2006 . High-Resolution Image Texture as a Predictor of Bird Species Richness . Remote Sensing of Environment , 105 : 299 – 312 .
- Swinnen , J. F.M. and Mathijs , E. 1997 . “ Agricultural Privatisation, Land Reform and Farm Restructuring in Central and Eastern Europe: A Comparative Analysis ” . In Agricultural Privatization, Land Reform and Farm Restructuring in Central and Eastern Europe , Edited by: Swinnen , J. F.M. , Buckwell , A. and Mathijs , E. 333 – 373 . Aldershot, , UK : Ashgate .
- Trzeciak-Duval , A. 1999 . A Decade of Transition in Central and Eastern European Agriculture . European Review of Agricultural Economics , 26 ( 3 ) : 283 – 304 .
- Turner , M. G. 2005 . Landscape Ecology in North America: Past, Present, and Future . Ecology , 86 ( 8 ) : 1967 – 1974 .
- Turner , M. D. and Congalton , R. G. 1998 . Classification of Multi-Temporal SPOT-XS Satellite Data for Mapping Rice Fields on a West African Floodplain . International Journal of Remote Sensing , 19 ( 1 ) : 21 – 41 .
- Turner II , B. L. , Lambin , E. F. and Reenberg , A. 2007 . Land Change Science Special Feature: The Emergence of Land Change Science for Global Environmental Change and Sustainability . Proceedings of the National Academy of Sciences , 104 ( 52 ) : 20666 – 20671 .
- Turnock , D. 2002 . Ecoregion-Based Conservation in the Carpathians and the Land-Use Implications . Land Use Policy , 19 ( 1 ) : 47 – 63 .
- Van Dijk , T. 2003 . Scenarios of Central European Land Fragmentation . Land Use Policy , 20 ( 2 ) : 149 – 158 .
- Van Rompaey , A. , Krasa , J. , Dostal , T. and Govers , G. 2003 . Modelling Sediment Supply to Rivers and Reservoirs in Eastern Europe During and After the Collectivisation Period . Hydrobiologia , 494 ( 1–3 ) : 169 – 176 .
- Verburg , P. H. 2006 . Simulating Feedbacks in Land Use and Land Cover Change Models . Landscape Ecology , 21 ( 8 ) : 1171 – 1183 .
- Wulder , M. A. , Ledrew , E. F. , Franklin , S. E. and Lavigne , M. B. 1998 . Aerial Image Texture Information in the Estimation of Northern Deciduous and Mixed Wood Forest Leaf Area Index (LAI) . Remote Sensing of Environment , 64 ( 1 ) : 64 – 76 .
- Zarzycki , K. and Glowacinski , Z. 1970 . Bieszczady. Przyroda Polska [Nature of Poland: Bieszczady] , Warsaw : Wiedza Powszechna [In Polish] .