Abstract
Worldwide, land change is responsible for one-fifth of anthropogenic carbon emissions. In Brazil, three-quarters of carbon emissions originate from land change. This study represents a municipal-scale study of carbon fluxes from vegetation in Rio Branco, Brazil. Land-cover maps of pasture, forest, and secondary growth from 1993, 1996, 1999, and 2003 were produced using an unsupervised classification method (overall accuracy = 89%). Carbon fluxes from land change over the decade of imagery were estimated from transitions between land-cover categories for each time interval. This article presents new methods for estimating emissions reductions from carbon stored in the vegetation that replaces forests (e.g., pasture) and sequestration by new (>10–15 years) forests, which reduced gross emissions by 16, 15, and 22% for the period of 1993–1996, 1996–1999, and 1999–2003, respectively. The methods used in the analysis are broadly applicable and provide a comprehensive characterization of regional-scale carbon fluxes from land change.
1. Introduction
Accounting for carbon fluxes from the terrestrial biosphere in the Amazon has garnered focused attention from various research communities for at least a decade (e.g., De Moraes, Seyler, Cerri, and Volkoff Citation1995; Fearnside Citation1996; Fearnside and Barbosa Citation1998; Houghton et al. Citation2000; Hirsch, Little, Houghton, Scott, and White Citation2004; Asner et al. Citation2005; Palm et al. Citation2005). Land-cover/land-use change (or land change) influences carbon fluxes both to and from the terrestrial biosphere. Methods to account for sinks and sources include modeling ecological productivity (Potter et al. Citation1993; Ito and Oikawa Citation2002; Hirsch et al. Citation2004; Nightingale, Phinn, and Held Citation2004), inverting atmospheric transport models (Ciais, Peylin, and Bousquet Citation2000; Fujita et al. Citation2003; Rödenbeck, Houweling, Gloor, and Heimann Citation2003), and land change analyses (Houghton et al. Citation2000; Naughton-Treves Citation2003).
Worldwide, land change accounts for approximately 20% of carbon emissions (Watson et al. Citation2001). In Brazil, however, 75% of carbon fluxes to the atmosphere are the result of land change (Houghton et al. Citation2000). Carbon budgets for the Amazon tend to focus on emissions from deforestation (e.g., Fearnside Citation1996; Fearnside and Barbosa Citation1998; Houghton et al. Citation2000; Naughton-Treves Citation2003). Emissions from conversion of forest and secondary growth to pasture or cropland, however, are offset by sequestration by secondary growth (Houghton et al. Citation2000; Naughton-Treves Citation2003), replacement vegetation such as forage species in pasture, and forests recently transitioned from late secondary growth, or ‘new’ secondary forests. These new forests are accumulating carbon, but they cannot be distinguished from surrounding forests using remotely sensed data (Moran, Brondizio, Mausel, and Wu Citation1994; Steininger Citation1996; Helmer, Brown, and Cohen Citation2000).
Land change can be observed and quantified by comparing maps of the same area from two dates. Multitemporal classifications are used to examine land change both qualitatively (exchanges between map categories) and quantitatively (area of landscape that has changed) (Rogan and Chen Citation2004). Combined with biophysical data such as ecosystem productivity or hydrology these classifications can indicate changes in ecological functioning of the landscape. For example, patterns in land change are often used to estimate carbon losses and uptake by the terrestrial biosphere (Flint and Richards Citation1993; Houghton Citation1995; Fearnside and Barbosa Citation1998; Houghton et al. Citation2000; Naughton-Treves Citation2003).
This municipal-scale study of Rio Branco, Brazil, seeks to elaborate a budget of carbon emissions from transitions between forest, pasture, and secondary vegetation. Land-cover maps were produced using three classification methods – classification tree analysis, maximum likelihood, and an unsupervised clustering algorithm. Carbon accumulation and losses from terrestrial biomass are derived from observed changes in land cover.
2. Study area
Rio Branco is the capital city of the state of Acre, Brazil, in the Southwest Brazilian Amazon with an average temperature of 25°C and annual precipitation of about 2000 mm ().
The municipality of Rio Branco measures 8831 km2 about 3% of which is urban land cover, which was excluded from the analysis. Approximately 75% of the municipality of Rio Branco is forested and about 22% is agricultural land cover (ZEE Citation2000). Nearly 80% of agricultural land is large-scale pasture, the rest is smaller pastures, and a marginal area of cropland, used for products such as coffee, soy, and corn as well as subsistence agriculture (ZEE Citation2000). Although international beef markets (Valdes Citation2006) have contributed to land-cover change in Amazonia (Kaimowitz et al. Citation2004), most of the beef produced in Rio Branco is consumed in the region, because of its isolation from the largest Brazilian markets to the south, which are often inaccessible by road. A large highway-paving effort has been undertaken in Peru, however, which will link Rio Branco with markets in western South America and East Asia, which is likely to accelerate deforestation rates in the region because of increased access to forested land and international markets (Brown, Brilhante, Mendoza, and Ribeiro de Oliveira Citation2001).
2.1. Land change
The conversion of forest to pasture is the most prevalent form of land change in the Amazon (Fearnside Citation1996), and Rio Branco is a nexus of such change. The state of Acre forms the westernmost point of the Arc of Deforestation (Fearnside Citation2005) extending from east to west across the southern Amazon. Deforestation rates in Acre have historically been lower than other states in the Brazilian Amazon (INPE Citation2004). For the entire Amazon, deforestation rates have risen steadily for the last 30 years (Laurance et al. Citation2001), with occasional reductions stemming from economic policies that curtailed expansion, such as the Plano Real (Fearnside Citation2005).
The precipitation gradient that stretches across the Amazon results in a shorter dry season in the western Amazon than in central and eastern regions. As a result, forests in Acre are subject to a dry season of roughly 4 months, whereas some parts of the eastern Amazon may experience no pronounced dry season at all (Sombroek Citation2001). Because of cloud cover (usually greater than 30% [Asner Citation2001]), forest growth in the Amazon is limited primarily by the available sunlight (Huete et al. Citation2006). The dominant growing strategy of forests in Acre is to grow upward quickly during the drier months. Therefore, faster-growing species are thinner than their slower-growing counterparts in eastern Amazon, with lower specific gravity (Baker et al. Citation2004).
3. Land-cover classification
3.1. Data
The remotely sensed data used in the analysis are Landsat Thematic Mapper (TM) and Enhanced Thematic Mapper-plus (ETM+) (path 02 row 067). The images were acquired during the dry season, which lasts from June until October (image acquisition dates: 6 June 1993, 1 August 1996, 2 August 1999, and 13 August 2003), when cloud cover is minimal. The 90-m digital elevation model (DEM) used in the topographic correction, as well as the image classification, is a product of the Shuttle Radar Topography Mission (SRTM).
Digital aerial photographs of Rio Branco were made available by the local zoning board, Zoneamento Economico Ambiental Social e Cultural (ZEAS). These photographs were used to locate appropriate sites for training data in the land-cover classification. The photographs were acquired in July 2005, and are composed of blue, green, and red bands. These data have a spatial resolution of 0.3 m and a total spatial extent of 103 km2 (1.2% of the municipality). A continuous distance to roads layer (spatial resolution of 30 m) and a binary map of conservation units in Rio Branco were also available from ZEAS for use in classifying the Landsat imagery.
Additional fine-grain spatial data were obtained from Digital Globe through Google Earth, a web server that provides Quickbird and other fine-grain imagery free of charge. These images were used to validate the land-cover maps. The fine spatial resolution data for Rio Branco covered 205,700 ha, or 29% of the study area. The date of the images ranged from August 2002 to March 2003. The spatial resolution is the same as the aerial photography used in this study (0.3 m) (Toutin and Cheng Citation2002), and the positional accuracy of the imagery (about 30 m) for the analysis (Potere Citation2008).
3.2. Preprocessing
The TM and ETM+ imagery was masked to the limits of the municipality of Rio Branco. Images from 1993, 1996, 1999, and 2003 were processed to remove atmospheric scattering effects using the Cos(t) model, which combines a Dark Object Subtraction model with attenuations for absorption effects using the cosine of the solar zenith angle (Chavez Citation1996). Geometric correction of the Landsat imagery was based on known locations of features such as bridges and road intersections (18 ground control points, RMSE = 13.2 m). The DEM was interpolated to 30 m to match the Landsat data. The DEM was georeferenced to match the Landsat imagery (RMSE = 14.8 m) based on 25 ground control points placed according to recognizable features such as oxbow lakes. Nearest-neighbor resampling was used in all post-geometric correction procedures. Topographic effects were removed from the imagery using a process that incorporates a hillshading model derived from the DEM. A regression was performed for each image band using the hillshading image as the independent variable. The slope and intercept of the linear regression were used to estimate solar illumination in the image, which was then subtracted from each image (Kuzera, Rogan, and Eastman Citation2005) to create a topography-independent spectral reflectance value/image.
Finally red and near-infrared bands of the Landsat imagery (bands 3 and 4, respectively) were employed to create a Normalized Differenced Vegetation Index (Tucker Citation1989). Normalized Differenced Vegetation Index, an indicator of vegetation abundance, and vigor were used as an input in image classification.
4. Methods
4.1. Image classification
4.1.1. Map legend
The map categories used in classifications of the study area were pasture, secondary growth, and forest. Pasture is defined as actively grazed rangeland, often mixed with some shrubs, trees, and leguminous species. Secondary growth includes both arboreous and shrubby successional growth. Trees in secondary vegetation areas were usually short stature and interspersed with shrubby and herbaceous vegetation. Areas of late secondary succession (10–15 years) exist, which were dominated by trees but shorter in stature than surrounding forest. Also included in the secondary vegetation category were some areas with many trees that are not forests such as riparian buffers in pasture. (These buffers are required by law, but are not separated by any barrier from the pasture and are often grazed by cattle.) Forests were defined as closed canopy bamboo or hardwood stands (>15 years). For all classifications, water and urban areas (275 km2) were masked using a supervised method because these classes were not of interest in the analysis.
4.1.2. Classification
Before classification, images were first processed to determine their constituent per-pixel fractions of pasture, secondary growth, and forest to be used in the supervised classifications. A linear spectral unmixing algorithm was employed, wherein the endmembers (pasture, forest, and secondary growth) were derived from homogeneous land cover in the fine-grain imagery. Membership in an endmember class is expressed as a fraction, so that each pixel is assumed to represent a mixture of endmembers (Adams et al. Citation1995). Pixels therefore express the proportion of an endmember class (e.g., pasture) varying from zero to one.
Three digital classification algorithms were compared in this analysis: classification tree analysis, maximum likelihood classification, and an unsupervised clustering algorithm. These three algorithms were chosen because of the apparent challenge of accurately mapping land cover in tropical forest regions (Eastman, Toledano, Crema, Zhu, and Jiang, Citation2005). The maximum likelihood approach is an industry standard and serves as a baseline for comparison among the three different classification algorithms (Rogan, Franklin and Roberts Citation2002). Classification trees are a novel and less-used method that has proven effective in heterogeneous landscapes (Friedl, Brodley and Strahler Citation1999). The unsupervised approach is more conservative from an empirical standpoint but is considered invaluable when faced with heterogeneous land cover and prevalent spectral confusion over space (Wulder, Franklin, White, Cranny, and Dechka, Citation2004).
Classification tree analysis uses training data to determine classification rules that bifurcate data until resulting categories are assigned to the appropriate class (Breiman, Friedman, Olshen, and Stone Citation1984). Maximum likelihood is a parametric Bayesian classifier that assigns pixels to a class based on the posterior probability of their membership in a particular class. Finally, the unsupervised technique used in the analysis is a clustering algorithm that forms clusters based on histogram peaks in bandspace (Eastman Citation2006a). Reflectance values for each band determine a pixel's location in bandspace, and apparent grouping of pixels (peaks) are used to demarcate clusters, to which the closest pixels in bandspace are assigned.
Training sites for the supervised classifications were selected from areas of pure pasture, secondary growth, and forest determined from the fine-grained imagery. Using a flood polygon method (Eastman Citation2006b), a representative pixel was selected by the user, after which the algorithm automatically selected other adjacent pixels according to their spectral similarity. To minimize the Hughes effect of error associated with the number of classes and the amount of training data used (Hughes Citation1968), a minimum of 90 pixels (three training sites with a minimum of 30 pixels) were used as training data for each class. Training sites were selected that were ‘pure’ examples of the land-cover type (i.e., did not contain pixels with mixed land cover according to fine-grain imagery) but were representative of the heterogeneity of a class.
Owing to within-class heterogeneity (such as different age classes grouped as ‘secondary growth’), an unsupervised classification was employed to determine the spectral classes that occur within the images using a clustering algorithm. Images were classified using a clustering mechanism that created classes based on spectral similarities, grouping pixels based on histogram peaks of pixel values in bandspace (Eastman Citation2006a). The unsupervised classification was based on the same reflectance values corrected for atmospheric and topographic effects used in supervised classification. Thirty clusters were produced for each image used in the analysis (1993, 1996, 1999, and 2003), which were grouped into new classes representing forest, pasture, and secondary growth (urban and water were generally not distinct clusters). The clusters were labeled based on visual interpretation of the fine-grain satellite imagery. A total of 90 samples were examined in the unsupervised classification and corresponding aerial photograph. For each cluster, three ground features were located in the fine-grain imagery to determine the corresponding class.
Classifications of the 2003 image run using each method (classification tree analysis, maximum likelihood, and clustering) were validated by selecting 30 samples randomly located within each class. Sample locations for accuracy assessment were identified in the fine-grain satellite imagery. The land cover in the location of the sample point (and the area within a 15-m radius) was compared with the class indicated in the classified image. A score between 1 (lowest) and 5 (highest) was recorded to express the agreement between the classified image and the imagery with higher spatial resolution (Woodcock and Gopal Citation2000). A score of 5 was assigned to sites that clearly matched the classification. A score of 1 was assigned to sites that could not have matched the classification at the time that the image was acquired. A median score of 3 was assigned to sites that could possibly have matched the classification at the time that the image was acquired, but did not match in the fine-grain validation image.
As a result of the high classification accuracy of the 2003 image using an unsupervised method (89%), images from previous years (1993, 1996, and 1999; for which fine-grain imagery is unavailable) were classified using the same approach. In this instance, however, classes were labeled based on visual interpretation of a composite image of bands 3, 4, and 5 (corresponding to the red, near-infrared, and mid-infrared bands) of the Landsat image used in the classification.
4.2. Estimating carbon emissions from land change
The amount of carbon emitted to the atmosphere was calculated as a function of the amount of carbon stored in terrestrial vegetation and the areal extent of each land-use type. Calculations of carbon emissions to the atmosphere from land change usually employ measurements of deforested area to estimate emissions from conversion. To be more comprehensive, some calculations subtract the amount of carbon that is sequestered by secondary vegetation (e.g., Naughton-Treves Citation2003).
Secondary growth is likely to be classified as mature forest after 10–15 years, because of the difficulty of separating secondary forests using spectral information from the TM sensor (Moran et al. Citation1994; Steininger Citation1996; Helmer et al. Citation2000). Most carbon accounting that uses remotely sensed imagery to identify land-cover types considers all secondary forests greater than 10–15 years to be forest, because of the difficulty of identifying secondary forests (Moran et al. Citation1994; Steininger Citation1996; Helmer et al. Citation2000). The danger of such an approach is that secondary forests are accumulating carbon at a much higher rate, resulting in an underestimate of uptake. Storage of carbon in these secondary forests is overestimated, as these forests have less biomass. In this approach, secondary forests were identified from the land change trajectory.
The value used for carbon stored in forests in Rio Branco (150 tC/ha) was taken from observation of carbon stocks from Lewis et al. (Citation2002). It should be noted that this is less than other biomass estimates for the southwest Amazon region from Brown, Nepstad, Pires, Luz, and Alechandre (Citation1992) (213 tC/ha, total forest carbon) and Brown et al. (Citation1995) (163 tC/ha, above ground biomass and coarse woody debris). The value used for carbon stored in pasture and secondary vegetation (2.85 and 6.86 tC/ha, respectively) was from Lewis et al. (Citation2002). Both pasture and secondary growth values were time-averaged, representing a value midway between the minimum and the maximum amount of carbon stored in these areas from the time of conversion. These amounts were significantly lower than an estimate based on a growth rate multiplied by the time elapsed between classification dates, which is likely the more accurate value because accumulation rates do not reflect losses from harvest (Watson et al. Citation2001). Finally, the amount of carbon sequestered by secondary vegetation per year (3.91 tC/ha) was also taken from estimates published for the region (Lewis et al. Citation2002).
Baseline calculations were made of carbon emissions from land-cover change, or carbon that was lost to deforestation and gained in secondary or fallow areas. Before the biomass loss was converted to carbon emissions, two more subtraction procedures were performed. First, the amount of carbon in the biomass that replaced the forested area, pasture or secondary vegetation, was subtracted from the net emissions estimate. Second, carbon sequestered in the transition from secondary vegetation to forest was subtracted from net emissions. Using the multitemporal land-cover maps, the transition between secondary growth and forest was used to identify secondary forests greater than 10–15 years old. Although the area that transitioned from secondary vegetation to forest in the classification does not signify a wholesale change from 6.86 to 150 tC/ha, the carbon stored in these areas increased beyond the secondary growth category. A value of 15 tC/ha (calculated as the fraction of carbon present in vegetation approximately one-tenth the age of a mature stand) was used as an estimate of the amount of carbon likely to be stored in these ‘new’ secondary forests.
First, the amount of carbon lost in the process of deforestation was calculated using the following equation:
The amount of carbon lost in the process of converting secondary or fallow areas to pasture was calculated using the following equation:
The amount of carbon sequestered by secondary vegetation between two observation periods is calculated using the following equation:
The amount of carbon gained by terrestrial vegetation when secondary vegetation ages beyond about 15 years (the age beyond which secondary forest is indistinguishable from other forests) was calculated using the following equation:
Finally, the carbon fluxes to the atmosphere as a result of deforestation and conversion of secondary growth to pasture was a function of the above equations.
5. Results
5.1. Image classification
Although there is no universal standard for accuracy assessment of classified imagery, some suggestions have been made about what is considered sufficient (Anderson, Hardy, Roach, and Witmer Citation1976; Congalton Citation1991; Rogan et al. Citation2003). In this instance, overall accuracy should be 85% or higher (Anderson et al. Citation1976; Rogan et al. Citation2003). The accuracy of classification tree and maximum likelihood classifications were not sufficient to be used in the analysis ().
Table 1. User's accuracy of land-cover classifications using classification tree analysis, maximum likelihood, and unsupervised methods
The overall accuracy of the classification tree analysis was 82%, whereas the accuracy of the maximum likelihood classifier was 76%. The unsupervised classification, with an accuracy of 89%, was sufficient for use in the analysis.
5.2. Results: trends in carbon uptake 1993–2003
Changes in the landscape of Rio Branco were determined by comparing land-cover maps for the period of 1993–1996, 1996–1999, and 1999–2003 ().
The pattern that emerges from land-change analysis reflects an initially moderate amount of deforested area (29,396 ha – about 3% of the municipality) followed by a significant drop in deforested area (20,000 ha) accompanied by high amount of secondary growth, and finally a reversion to a high amount of pasture formation from both secondary vegetation and forested areas (deforestation = 39,873 ha) ().
Figure 3. Transitions between land cover types for the three intervals used in the analysis: 1993–1996, 1996–1999, and 1999–2003.
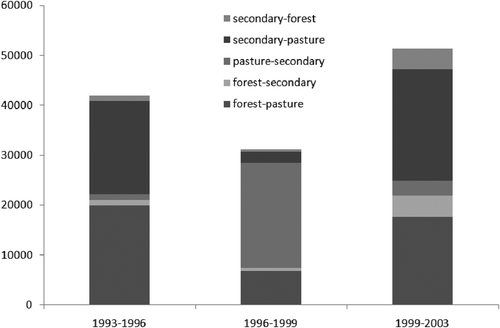
In the first time interval, 1993–1996, 29,296 ha of forest were converted to pasture. Fifty-five percent of new pastures formed were converted from forest, 45% were converted from secondary vegetation. In the second time interval, 1996–1999, deforestation rates slowed considerably (a 32% reduction from the previous time period). During the 1996–1999 interval, a much higher percentage of pasture formation came from deforestation than from re-clearing secondary growth (87%). At the same time, 26% of existing pasture reverted to secondary vegetation.
In the final period of the analysis deforestation rates were increasing again (100% higher than the second time interval and 36% higher than the first interval). The proportion of pasture formation from forest (as opposed to re-clearing areas of secondary growth/fallow) was 44% from 1999 to 2003, much lower than the previous time intervals. The relatively low amount of pasture created from forest in the last time interval reflects a surfeit of secondary vegetation left over from the second time period. Ranchers will preferentially create pasture from secondary growth, Ceteris paribas, because this method is far less labor intensive than clearing forest. Secondary vegetation cover decreased in the final interval, to an amount close to the proportion found in the 1993 landscape. It is likely that, similar to the rest of the state of Acre, secondary growth will continue to decline with increasingly intensive land change (Salimon and Brown Citation2000).
Carbon emissions to the atmosphere from land-cover change in the Amazon are summarized for each time period (1993–1996, 1996–1999, and 1999–2003) in . Emissions declined and sequestration increased during the second period (1996–1999), at which time Rio Branco was a sink for atmospheric carbon. In the final interval, the vegetation of Rio Branco still acted as a net sink, although much weaker than in the second interval because of declines in secondary and increased deforestation.
Table 2. Carbon fluxes (metric tons) per land cover transition for each time period in the analysis
5.2.1. 1993–1996
During the earliest time period in the analysis, the net amount of carbon emitted from the atmosphere as a result of land-cover change was 358,550 t, or 0.36 Mt of carbon (
EquationEquation 5(5)). Emissions from conversion of forest to pasture accounted for 1,190,538 tons (1.19 Mt), and emissions from secondary vegetation to pasture amounted to 99,266 t (0.99 Mt), for a total 1,289,804 t (1.29 Mt) from land-cover conversion to pasture. The effect of subtracting the amount of carbon stored in replacement land-cover types accounted for an average of 6.51%. Subtracting carbon sequestered by new forests reduced gross emissions from land change by 3.40%, and sequestration by secondary growth further reduced the figure by 55.79%.
5.2.2. 1996–1999
In the period between 1996 and 1999, the vegetation of Rio Branco was a sink of atmospheric carbon. The net amount of carbon sequestered from the atmosphere was 570,829 tons, or 0.57 Mt.
The amount of carbon emitted from forest conversion was 810,041 tons (0.81 Mt). Additionally, 11,846 tons (0.01 Mt) of carbon was emitted as a result of pasture formation from secondary vegetation for a total of 821,886 tons (0.82 Mt) of carbon emitted from land-cover change. Accounting for replacement vegetation amounted to an average reduction of 6.70%. New forests reduced emissions by 1.51% and sequestration by secondary growth offset emissions by 154.55%, causing a shift to a carbon sink.
5.2.3. 1999–2003
During the period of 1999–2003, the landscape was a weaker sink than the preceding interval. The amount of carbon sequestered from conversion of forest and secondary vegetation to pasture in the period from 1999 to 2003, is 110,242 tons (0.11 Mt).
The amount of carbon emitted from forest conversion was 1,614,851 tons (1.61 Mt). An additional 117,958 tons (0.12 Mt) of carbon was emitted to the atmosphere as a result of pasture conversion, for a total of 1,732,810 tons (1.73 Mt). Accounting for replacement vegetation resulted in an average reduction of 7.6%. New forests reduced emissions by 6.23% and sequestration by secondary growth caused a further reduction of 84.90%.
6. Discussion
The comparative method of land-cover classification is highly recommended, particularly in the context of highly dynamic regions with a range of potential conditions (and, hence, spectral signatures) for each land-cover type. In circumstances where ample training data are available, supervised classification may be more successful. In this case, an unsupervised method was better suited to the diversity of conditions and lack of ground-based observations of land cover.
Over the assessment period, vegetation in Rio Branco was a source of carbon from 1993 to 1996, a sink from 1996 to 1999, and sink of diminished strength from 1999 to 2003. The shift in the region from a carbon source to a carbon sink is likely related to an economic policy that was enacted and repealed during the time of the study. The Plano Real, a fiscal austerity measure implemented in an attempt to curb inflation, was instated in 1994 (Williamson Citation2002; Fearnside Citation2005). The value of the Brazilian currency was tied to the United States dollar in an effort to stimulate outside investment and curb inflation (Williamson Citation2002). The program was initially successful at curbing inflation, but eventually the cost of agricultural inputs became too high and economic growth (and, hence, pasture formation) stagnated. An unintended result of the policy is that the cost of land, materials, and wages were not commensurate with returns on investment (Williamson Citation2002). Deforestation rates also slowed because falling land values made speculation unattractive (Fearnside Citation2005).
The first time period, during which the region was a net source of carbon, was a time of economic growth because of the early success of the Plano Real. In the second time period (1996–1999) the region became a sink of atmospheric carbon, a change that is likely related to economic stagnation in the region (Fearnside Citation2005). This observation in the Brazilian Amazon is not unique – post-1994 deforestation rates over the entire region slowed in response to decreased economic activity (Fearnside Citation2005). Despite an increase in world beef prices, ranchers in Brazil lacked financial incentives to expand production.
Another difference between the period from 1993 to 1996 and 1996 to 1999 was that far more pasture was created from forest than from secondary growth. A possible explanation for this phenomenon is that the high price of land made unauthorized expansion of clearings more pervasive. Deforesting an area for ranching essentially laid claim to the land, a process likely preferable to buying land outright during this period. This pattern changed again in the final period of the analysis from 1999 to 2003, when more pastures were created from secondary vegetation than forest. The relatively low amount of pasture created from forest in the last time interval likely reflects an excess of secondary vegetation left over from the second time period.
The trends elucidated above, with a change in sign from source to sink of atmospheric carbon, bear further examination. The switch from source to sink is due to dynamic and multidirectional land-cover change influenced by an economic policy directed toward increasing financial stability. In the case of the last time period examined (1999–2003), the economic policy was no longer in effect, the Brazilian currency having been devalued in January of 1999. The immense amount of secondary growth produced in the second interval was not immediately reclaimed as pasture, however. The secondary vegetation that persists continues to affect the sign of carbon emissions by sequestering atmospheric carbon, but this vegetation will not remain indefinitely. Economic policies that cause a reduction in development are not a common occurrence in Brazil or anywhere in the world.
Another factor impeding the continuation of a carbon sink in secondary vegetation in Rio Branco is infrastructural developments and expansion of the built environment (Laurance et al. Citation2001; Fearnside Citation2005). The paving of the highway BR-317, linking Rio Branco with ports in Bolívia and Peru will be completed in the next few years. This highway will connect Rio Branco with new economic markets and increase demand for agricultural output such as beef (Brown et al. Citation2001; De Los Rios Maldonado, Brown, Valeriano, and Duarte Citation2007). Land cover is expected to continue to change over the next few decades, and carbon emissions will likely increase as the direct result of anthropogenic activity.
Solutions aimed at reducing carbon emissions will have to contend with the need for economic growth. Ideally, development will proceed with consideration of unintended consequences such as carbon emissions, loss of wildlife habitat, and disruption of ecosystem functions. The degree to which Rio Branco and other expanding urban areas are able to do this will determine their carbon footprint.
7. Conclusions
The results of the study demonstrate that carbon emissions from land-cover change are not consistent over time in magnitude or extent due to changes in the characteristics of pasture formation related to economic policy and currency valuation. Variation in sign and magnitude of carbon fluxes are a common observation at varying spatial and temporal scales (Fung Citation2000; Baldocchi et al. Citation2001, Schimel et al. Citation2001). Economic policies that slow development reduce carbon emissions by removing incentives for land change, which results in large areas reverting to secondary vegetation and slower forest conversion (e.g., Naughton-Treves Citation2003). Such policies can reduce emissions for years following their repeal. Although the reduction of carbon emissions may appear superficially to be a positive effect of this policy, the overall result is economically untenable. Sequestration measures therefore must be viewed in the broader (economic, ecological, cultural) context of the country in which they take place.
This analysis is unique in that it includes emissions reductions from replacement vegetation and new forests. The inclusion of new carbon pools has not, however, challenged the evidence that carbon fluxes from land-cover change in the region are increasing. Carbon emissions from land-cover change in Rio Branco will most likely continue to rise in the coming years.
Deforestation rates in Acre are increasing with the demand for goods produced from converted land. Links with new economic markets in western South America and East Asia will provide new outlets for products from Acre. Secondary growth is likely to diminish with shorter fallowing periods or continuous cultivation. Sequestration from secondary growth accounted for the majority of emissions offsets in this analysis; therefore carbon fluxes are likely to increase both as a function of increasing deforestation and as decreasing secondary growth.
Acknowledgments
The research described in this article has been funded wholly or in part by the United States Environmental Protection Agency (EPA) under the Science to Achieve Results (STAR) Graduate Fellowship Program. EPA has not officially endorsed this publication and the views expressed herein may not reflect the views of the EPA. The authors thank Judson Valentim, Nadia Pereira, I. Foster Brown, Eufran Amaral and B.L. Turner. Aerial photographs used in the analysis were provided by the Prefeitura Municipal de Rio Branco.
References
- Adams , J. B. , Sabol , D. E. , Kapos , V. , Filho , R. A. , Roberts , D. A. , Smith , M. O. and Gillespie , A. R. 1995 . Classification of Multispectral images based on fractions of endmembers: Application to land-cover change in the Brazilian Amazon . Remote Sensing of the Environment , 52 : 137 – 154 .
- Anderson , J. R. , Hardy , E. E. , Roach , J. T. and Witmer , R. E. 1976 . A Land Use and Land Cover Classification System for Use with Remote Sensor Data , Reston, VA : U.S. Geological Survey . Professional Paper 964
- Asner , G. P. 2001 . Cloud Cover in Landsat Observations of the Brazilian Amazon . International Journal of Remote Sensing , 22 : 3855 – 3862 .
- Asner , G. P. , Knapp , D. E. , Broadbent , E. N. , Oliveira , P. J.C. , Keller , M. and Silva , J. N. 2005 . Selective Logging in the Brazilian Amazon . Science , 310 : 480 – 482 .
- Baker , T. R. , Phillips , O. L. , Malhi , Y. , Almeida , S. , Arroyo , L. , di Fiore , A. , Erwin , T. , Killeen , T. J. , Laurance , S. G. , Laurance , W. F. , Lewis , S. L. , Lloyd , J. , Monteagudo , A. , Neill , D. A. , Patiño , S. , Pitman , N. C.A. , Silva , J. , Natalino , M. and Martínes , R. V. 2004 . Variation in Wood Density Determines Spatial Patterns in Amazonian Forest Biomass . Global Change Biology , 10 : 545 – 562 .
- Baldocchi , D. , Falge , E. , Gu , L. , Olson , R. , Hollinger , D. , Running , S. , Anthoni , P. , Bernhofer , C. , Davis , K. , Evans , R. , Fuentes , J. , Goldstein , A. , Katul , G. , Law , B. , Xuhui , L. , Malhi , Y. , Meyers , T. , Munger , W. , Oechel , W. and Paw U , K. T. 2001 . FLUXNET: A New Tool to Study the Temporal and Spatial Variability of Ecosystem-Scale Carbon Dioxide, Water Vapor, and Energy Flux Densities . Bulletin of the American Meteorological Society , 82 : 2416 – 2434 .
- Breiman , L. , Friedman , J. H. , Olshen , R. A. and Stone , C. J. 1984 . Classification and Regression Trees , Belmont, CA : Wadsworth International Group .
- Brown , I. F. , Brilhante , S. H.C. , Mendoza , E. and Ribeiro de Oliveira , I. 2001 . “ Estrada de Rio Branco, Acre, Brasil aos Portos do Pacifico: Como maximizar os beneficios e minimizar os prejuizos para o desenvolvimento sustentavel da Amazonia Sul-Ocidental ” . In Encuentro Internacional de Integracion Regional – Bolivia, Brasil y Peru , Arquipa, , Peru : Centro Peruviano de Estudios Internacionales .
- Brown , I. F. , Martinelli , L. A. , Thomas , W. W. , Moreira , M. Z. , Ferreira , C. A.C. and Victoria , R. A. 1995 . Uncertainty in the Biomass of Amazonian Forests: An Example from Rondonia, Brazil . Forest Ecology and Management , 74 : 175 – 189 .
- Brown , I. F. , Nepstad , D. C. , Pires , I. O. , Luz , L. M. and Alechandre , A. S. 1992 . Carbon Storage and Land-Use in Extractive Reserves, Acre, Brazil . Environmental Conservation , 19 : 307 – 315 .
- Chavez , P. S. 1996 . Image-Based Atmospheric Corrections Revisited and Improved . International Journal of Remote Sensing , 62 : 1025 – 1036 .
- Ciais , P. , Peylin , P. and Bousquet , P. 2000 . Regional Biospheric Carbon Fluxes as Inferred from Atmospheric CO2 Measurements . Ecological Applications , 10 : 1574 – 1589 .
- Congalton , R. G. 1991 . A Review of Assessing the Accuracy of Classifications of Remotely Sensed Data . Remote Sensing of Environment , 37 : 35 – 46 .
- De Los Rios Maldonado , M. J. , Brown , I. F. , Valeriano , D. and Duarte , V. 2007 . Modificaç[otilde]es No Método Do PRODES Para Estimar a Mudança Da Cobertura Florestal Na Bacia Trinacional Do Rio Acre Na Região De Fronteira Entre Bolívia, Brasil E Peru Na Amazonia Sul-Ocidental. XIII Simpósio Brasileiro De Sensoriamento Remoto , 5903 – 5910 . Florianópolis, , Brazil : INPE .
- De Moraes , J. F.L. , Seyler , F. , Cerri , C. C. and Volkoff , B. 1995 . Land Cover Mapping and Carbon Pools Estimates in Rondonia, Brazil . International Journal of Remote Sensing , 19 : 921 – 934 .
- Eastman , J. R. 2006a . Idrisi Kilimanjaro: CLUSTER Operation , Worcester, MA : Clark Labs .
- Eastman , J. R. 2006b . Idrisi Kilimanjaro: Supervised Classification , Worcester, MA : Clark Labs .
- Eastman , J. R. , Toledano , J. , Crema , S. , Zhu , H. and Jiang , H. 2005 . In-Process Classification Assessment of Remotely Sensed Imagery . GeoCarto International , 20 ( 4 ) : 33 – 44 .
- Fearnside , P. M. 1996 . Amazonian Deforestation and Global Warming: Carbon Stocks in Vegetation Replacing Brazil's Amazon Forest . Forest Ecological Management , 80 : 21 – 34 .
- Fearnside , P. M. 2005 . Deforestation in Brazilian Amazonia: History, Rates, and Consequences . Conservation Biology , 19 : 680 – 688 .
- Fearnside , P. M. and Barbosa , R. I. 1998 . Soil Carbon Changes from Conversion of Forest to Pasture in Brazilian Amazonia . Forest Ecology and Management , 108 : 147 – 166 .
- Flint , E. P. and Richards , J. F. 1993 . “ Trends in Carbon Content of Vegetation in South and Southeast Asia Associated with Changes in Land Use ” . In Effects of Land-Use Change on Atmospheric CO2 Concentrations. South and Southeast Asia as a Case Study , Edited by: Dale , V. New York : Springer-Verlag .
- Friedl , M. A. , Brodley , C. E. and Strahler , A. H. 1999 . Maximising land cover classification accuracies produced by decision trees at continental to global scales . IEEE Transactions on Geoscience and Remote Sensing , 32 : 969 – 977 .
- Fujita , D. , Ishizawa , M. , Maksyutov , S. , Thornton , P. E. , Saeki , T. and Nakazawa , T. 2003 . Inter-Annual Variability of the Atmospheric Carbon Dioxide Concentrations as Simulated with Global Terrestrial Biosphere Models and an Atmospheric Transport Model . Tellus B , 55 : 530 – 546 .
- Fung , I. 2000 . Variable Carbon Sinks . Science , 290 : 1313
- Helmer , E. H. , Brown , S. and Cohen , W. B. 2000 . Mapping Montane Tropical Forest Successional Stage and Land Use with Multi-Date Landsat Imagery . International Journal of Remote Sensing , 21 : 2163 – 2183 .
- Hirsch , A. I. , Little , W. S. , Houghton , R. A. , Scott , N. A. and White , J. D. 2004 . The Net Carbon Flux Due To Deforestation and Forest Re-Growth in the Brazilian Amazon: Analysis Using a Process-Based Model . Global Change Biology , 10 : 908 – 924 .
- Houghton , R. A. 1995 . Land-Use Change and the Carbon Cycle . Global Change Biology , 1 : 275 – 287 .
- Houghton , R. A. , Skole , D. L. , Nobre , C. A. , Hackler , J. L. , Lawrence , K. T. and Chomentowski , W. H. 2000 . Annual Fluxes of Carbon from Deforestation and Regrowth in the Brazilian Amazon . Nature , 403 : 301 – 304 .
- Huete , A. R. , Didan , K. , Shimabukuro , Y. E. , Ratana , P. , Saleska , S. R. , Hutyra , L. R. , Yang , W. , Nemani , R. R. and Myneni , R. 2006 . Amazon Rainforests Green-up with Sunlight in Dry Season . Journal of Geophysical Research Letters , 33 Doi: 10.1029/2005GL025583
- Hughes , G. F. 1968 . On the Mean Accuracy of Statistical Pattern Recognizers . IEEE Transactions on Information Theory , 14 : 55 – 63 .
- INPE . 2004 . Monitoramento Da Floresta Amazônica Brasileira Por Satélite , Brazil : Instituto de Pesquisa Espaciais . Projeto PRODES, São José dos Campos
- Ito , A. and Oikawa , T. 2002 . A Simulation Model of the Carbon Cycle in Land Ecosystems (Sim-CYCLE): A Description Based on Dry-Matter Production Theory and Plot Scale Validation . Ecological Modeling , 151 : 147 – 179 .
- Kaimowitz , D. , Mertens , B. , Wunder , S. and Pacheco , P. . Hamburger connection fuels Amazon destruction: Cattle Ranching and Deforestation in Brazil's Amazon . Center for International Forestry Research . Jakarta, Indonesia.
- Kuzera , K. , Rogan , J. and Eastman , J. R. . Monitoring Vegetation Regeneration and Deforestation Using Change Vector Analysis: Mt. St. Helens Study Area . American Society for Photogrammetry and Remote Sensing Annual Conference . Baltimore, MD.
- Laurance , W. F. , Cochrane , M. A. , Bergen , S. , Fearnside , P. M. , Delamônica , P. , Barber , C. , D'Augelo , S. and Fernandes , T. 2001 . The Future of the Brazillian Amazon . Science , 291 : 438 – 439 .
- Lewis , J. , Vosti , S. , Witcover , J. , Ericksen , P. J. , Guevara , R. and Tomich , T. , eds. 2002 . Alternatives to Slash-and-Burn in Brazil: Summary Report and Synthesis of Phase II , Nairobi : CGIAR .
- Moran , E. F. , Brondizio , E. , Mausel , P. and Wu , Y. 1994 . Integrating Amazonian Vegetation, Land-Use, and Satellite Data . Bioscience , 44 : 329 – 338 .
- Naughton-Treves , L. 2003 . Deforestation and Carbon Emissions at Tropical Frontiers: A Case Study from the Peruvian Amazon . World Development , 32 : 173 – 190 .
- Nightingale , J. M. , Phinn , S. R. and Held , A. A. 2004 . Ecosystem Process Moels at Multiple Scales for Mapping Tropical Forest Productivity . Progress in Physical Geography , 28 : 241 – 281 .
- Palm , C. A. , van Noordwijk , M. , Woomer , P. L. , Alegre , J. C. , Arevalo , L. , Castilla , C. E. , Cordeiro , D. G. , Hairiah , K. , Kotto-Same , J. , Moukam , A. , Parton , W. J. , Ricse , A. , Rodrigues , V. and Sitompul , S. 2005 . “ Carbon Losses and Sequestration Following Land Use Change in the Humid Tropics ” . In Slash and Burn Agriculture: The Search for Alternatives , Edited by: Palm , C. , Vosti , S. A. , Sanchez , P. A. and Erikson , P. J. New York : Columbia University Press .
- Potere , D. 2008 . The Horizontal Positional Accuracy of Google Earth's High Resolution Imagery Archive . Sensors , 8 : 1 – 10 .
- Potter , C. S. , Randerson , J. T. , Field , C. B. , Matson , P. A. , Vitousek , P. M. , Mooney , H. A. and Klooster , S. A. 1993 . Terrestrial Ecosystem Production: A Process Model Based on Global Satellite and Surface Data . Global Biogeochemical Cycles , 7 : 811 – 842 .
- Rödenbeck , C. , Houweling , S. , Gloor , M. and Heimann , M. 2003 . CO2 Flux History 1982–2001 Inferred from Atmospheric Data Using a Global Inversion of Atmospheric Data . Atmospheric Chemistry and Physics Discussions , 3 : 2575 – 2659 .
- Rogan , J. and Chen , D. 2004 . Remote Sensing Technology for Mapping and Monitoring Land-Cover and Land-Use Change . Progess in Planning , 61 ( 4 ) : 301 – 325 .
- Rogan , J. , Franklin , J. and Roberts , D.A . 2002 . A Comparison of methods for monitoring multitemporal vegetation change using Thematic Mapper Imagery . Remote Sensing of the Environment , 80 : 143 – 156 .
- Rogan , J. , Miller , J. , Stow , D. , Franklin , J. , Levin , L. and Fischer , C. 2003 . Land-Cover Change Monitoring with Classification Trees Using Landsat TM and Ancillary Data . Photogrammetric Engineering and Remote Sensing , 69 : 793 – 804 .
- Salimon , C. and Brown , I. F. 2000 . Secondary Forests in Western Amazonia: Significant Sinks for Carbon Released from Deforestation? . Interciencia , 25 : 198 – 202 .
- Schimel , D. S. , House , J. I. , Hibbard , K. A. , Bousquet , P. , Ciais , P. , Peylin , P. , Braswell , B. H. , Apps , M. J. , Baker , D. , Bondeau , A. , Canadell , J. , Churkina , G. , Cramer , W. , Denning , A. S. , Field , C. B. , Friedlingstein , P. , Goodale , C. , Heimann , M. , Houghton , R. A. , Melillo , J. M. , Moore , B. III , Murdiyarso , D. , Noble , I. , Pacala , S. W. , Prentice , I. C. , Raupach , M. R. , Rayner , P. J. , Scholes , R. J. , Steffen , W. L. and Wirth , C. 2001 . Recent Patterns and Mechanisms of Carbon Exchange by Terrestrial Ecosystems . Nature , 414 : 169 – 172 .
- Sombroek , W. 2001 . Spatial and Temporal Patterns of Amazon Rainfall . Ambio , 30 : 388 – 396 .
- Steininger , M. K. 1996 . Tropical Secondary Forest Regrowth in the Amazon: Age, Area and Change Estimation with Thematic Mapper Data . International Journal of Remote Sensing , 17 : 9 – 27 .
- Toutin , T. and Cheng , P. 2002 . QuickBird—a Milestone for High Resolution Mapping . Earth Observation Magazine , 11 ( 4 ) : 14 – 18 .
- Tucker , C. J. 1989 . Red and Photographic Infrared Linear Combinations for Monitoring Vegetation . Remote Sensing of Environment , 8 : 127 – 150 .
- Valdes , C. 2006 . Special Section: An Update on FMD in Brazil and a look at the Pornk Industry and Domestic Demand . Livestock, Dairy & Poultry , Outlook/LDP-M-139/January 19, 2006. Economic Research Service, USDA, pp. 13–17
- Watson , R. T. , Noble , I. R. , Bolin , B. , Ravindrath , N. H. , Varedo , D. J. and Dokken , D. J. 2001 . Land Use, Land-Use Change and Forestry , Cambridge : Cambridge University Press . Special Report of the IPCC (Intergovernmental Panel on Climate Change)
- Williamson , J. 2002 . “ Brazil's Plano Real: A View From the Inside ” . In Development Economics and Structuralist Macroeconomics: Essays in Honor of Lance Taylor , Edited by: Dutt , A. and Ros , J. Cheltenham, UK : Edward Elgar .
- Woodcock , C. E. and Gopal , S. 2000 . Fuzzy Set Theory and Thematic Maps: Accuracy Assessment and Area Estimation . International Journal of Geographical Information Science , 14 : 153 – 172 .
- Wulder , M. , Franklin , S. , White , J. , Cranny , M. and Dechka , J. 2004 . Inclusion of Topographic Attributes in an Unsupervised Classification of Satellite Imagery . Canadian Journal of Remote Sensing , 30 ( 2 ) : 137 – 149 .
- ZEE . 2000 . Zoneamento Ecológico Econômico Do Acre: Aspectos Socioeconômicos a Ocupação Territorial , Rio Branco, Brazil : Governo do Estado do Acre, SECTMA .