Abstract
Notions of land cover relating to physical landscape characters are readily captured by satellite imagery. Land use on the other hand relates more to the societal aspects of a landscape. We argue that much of the spatial configuration of landscape characters is related to land use and that satellite data can be used to represent and investigate interpretations of land use. We propose and demonstrate the joint use of a novel SRPC procedure for satellite imagery together with an explicit representation of category semantics. We use these two mechanisms to identify a collection of conceptual spaces related to land use on Swedish historic summer farms. We also outline a framework for analysis of the relations between two separate ways of knowing: the machine-based knowledge and the human, mental knowledge. An evaluation demonstrates that satellite images can be used to identify land use processes as a mixture of land cover objects occurring in particular spatial contextual relationships closely tied to the land use category semantics. This opens up an unexplored possibility for research on vague spatial ontologies and questions on how to formally articulate different interpretations of space, land use, and other branches of spatial social science.
Introduction
Models that describe the dynamics of water, energy, and carbon in the geo-, bio-, and atmospheres are becoming increasingly sophisticated as public agencies and private organizations are making sustained efforts to monitor changes in the environment. To support these efforts, it is necessary to have detailed and accurate information on land cover, land use, and their changes across geographic scales (DeFries and Belward Citation2000). Developments in remote sensing technology have enabled ever more sophisticated methods to provide information on the physical characteristics (Aplin Citation2004). Questions on how to make use of satellite images for land use studies has also kept researchers occupied ever since the first satellite data became available, and tools are being developed to add social aspects for integrated analysis of land cover and land use dynamics (Treitz and Rogan Citation2004; Verburg and Veldkamp Citation2005). Despite these developments there is a growing concern about the slow progress on the scientific dimension of land use (Fisher, Comber, and Wadsworth Citation2005). Satellite imagery, contrary to general belief, does not automatically provide an impartial tool for objective assessment of a landscape (Hoeschele Citation2000; Robbins Citation2001). As an example, information on land use is not readily observed from a remote vantage point, but requires insights into how the society structures space, how land is used, and sometimes even its history (Cihlar and Jansen Citation2001). Still, certain types of land use often leave a very particular and characteristic spatial pattern in the landscape (Webster Citation1996), which in turn provides an opportunity for investigations into the relationship between patterns of land cover and current and past land use practices (Herold, Scepan, and Clarke Citation2002; Barr, Barnsley, and Steel Citation2004).
Although relations between remote sensing imagery and geographic information systems have been investigated (Gahegan and Flack Citation1999; Fonseca, Egenhofer, Agouris, and Camara Citation2002), these technologies generally have limited potential when concerning incorporation of knowledge about land use and land use history dimensions often encountered in social science interpretations of landscapes. Through this paper we aim to build on this previous work and bring together methods that semi-automatically identify expressions of compound land use objects in satellite images, with methods that enable a formal semantic description of complex objects typically used to signify such specific forms of land use. Our proposal therefore incorporates approaches for investigations into the conceptualization of land use and land use dynamics in geography and landscape analysis. In doing so we demonstrate how we can narrow the gap between land use practice and quantitative spatial data, such as satellite images. This not only has the potential to improve the performance of automated methods that attempt to identify objects in the landscape, but can also provide quantitative aspects to complement the conceptual understanding of land use.
Our approach rests on openness for the complexity found both in the human understanding of a landscape as well as in the used quantitative data. Our case study illustrates how automated processes may generate a result, which corresponds to compound land use concepts. While we do not provide any direct answers to questions of complexity in geography (cf. Schuurman Citation2006), the suggested approach may take us closer to developing methods useful in investigating and seeking an understanding of the complexity of land use dynamics.
This paper is organized as follows: in the first section we provide perspectives on the notions of land cover, land use, and landscape dynamics, and how they relate roughly to the societal and physical aspects of a landscape. The two subsequent sections introduce the main methodological components used in our work; the uncertain conceptual spaces approach to represent semantics; and the spatial relational post classification (SRPC) process for semi-automated derivation of land use expressions in satellite data. These sections are followed by a presentation and evaluation of an empirical case study. Finally, our proposed synthesis of these strands is presented followed by some concluding comments.
Remote sensing of land cover and land use
Although information about landscape can be produced in several ways, we will focus our discussion on categorical land cover and land use data derived from satellite imagery. Land cover can be defined as the observed (bio) physical cover on the earth's surface, and land use as the intentional activities undertaken by individuals in a certain landscape type to produce, change, or maintain it (Di Gregorio Citation2004). Data on land cover and land use is mostly provided as geo-referenced areal units with nominal labels developed from shared notions of landscape character. Remote sensing data sources have several advantages, but a key benefit is the comparatively large area that can be covered at a relatively low cost. In its raw form a satellite image only comprises spectral information based on reflectance values of electromagnetic radiation registered in a predefined grid. To produce a classified image, each image pixel is analytically evaluated and assigned to a spectrally distinct class, and then associated with a concept that corresponds to an identifiable real-world concept. For further details on classification of remote sensing imagery, we refer to introductory texts on remote sensing such as Lillesand, Kiefer, and Chipman (Citation2004) or Campbell (Citation2007).
Land cover
As land cover acquisition progressed many stakeholders recognized the need for standardized nomenclatures in order to create homogeneous information sources, which could be aggregated into comprehensive national or global data sets comparable over time. Some examples of data sets that use standardized nomenclatures are the CORINE Land Cover (CEC Citation1995), AFRICOVER (Kalensky Citation1998), and Global Land Cover 2000 (Bartholomé and Belward Citation2005). Despite availability of standardized classification systems, there remained problematic issues related to spatial heterogeneity (e.g., Crapper Citation1984; Foody and Boyd Citation1999) and category semantics (Robbins Citation2001; e.g., Fisher and Pathirana Citation1990; Gopal and Woodcock Citation1994).
Due to the fact that land cover information is inherently subject to individual variation between producer's and user's understanding of the landscape, Comber, Fisher, and Wadsworth (Citation2005) argued that there are profound uncertainties involved in a naïve use of land cover data without considering class origins and meaning. No one classification system can accurately reflect the social or the natural world (Bowker and Star Citation1999). Notions of land cover are also relativist in the manner it is politically and socially constructed (Crampton Citation2001; Perkins Citation2003). Harvey and Chrisman (Citation1998) effectively illustrated this by highlighting Shapiro's (Citation1995) study of the large (60–90%) disagreement between wetland maps produced by different actors. Another set of examples is given by Wainwright and Bryan (Citation2009) where they describe counter-mapping activities where differing legal arguments about indigenous land claims were articulated through the use of maps. Comber et al. (Citation2005) therefore argued for the importance of ontology (Gruber Citation1993) in land cover nomenclatures as a means to specify and avoid some of the most severe semantic mismatches. One notable effort to support dynamic land cover terminologies is the UN Food and Agriculture Organization Land Cover Classification System (Di Gregorio and Jansen Citation2000), which seeks to establish a formal parametric approach to describe land cover in a manner that allows multiple interpretations of categories across conceptualizations (Jansen and Gregorio Citation2002). This work currently provides the basis for an ISO Technical Committee 211 proposed standard for classification system structure and land cover classification systems (ISO/DIS 19144-1 and ISO/CD 19144-2). Another related effort is the IGBP and IHDP Global Land Project in which development of standard land cover classification systems is a required implementation consideration (GLP Citation2005).
Despite some progress through these initiatives, a common impediment of many standardized land cover terminologies is that they mix terms related to land cover, for example, the CORINE term ‘Broadleaved forest’, with terms related to land use, for example, the CORINE term ‘Pasture’. Some terms also include both land use and land cover notions in their definition, for example, the Global Land Cover 2000 term ‘Mosaic of Cropland/Tree cover/Other Natural Vegetation’. This unfortunate mix of physical properties and human activities has only lately been discussed critically (Bibby and Shepherd Citation2000; Robbins Citation2001; Comber et al. Citation2005) and investigated for the intricate relationships between land use and land cover (Turner et al. Citation1995; Barnsley and Barr Citation1996; Cihlar and Jansen Citation2001; Brown and Duh Citation2004).
Land use
Land use is the intentional activities and inputs that people undertake in a certain landscape type to produce, change, or maintain it (Di Gregorio Citation2004). Defined in this manner, land use embraces concepts that refer to the praxis of farmers, landowners, and other actors. There is a complex interaction between the intentions, actions, and responses of those agents to agriculture policies, external forces, and to the physical landscape that cumulatively result in specific land use effects.
Accordingly, land use must primarily be perceived as socially and economically driven (cf. Bibby and Shepherd Citation2000), and as being created at the interface between society structures; intentions; and concrete practice, technical and physical limitations (Brandt, Primdahl, and Reenberg Citation1999; Erb et al. Citation2007). Furthermore, there is an important feedback from land use to land cover in terms of the impact land use has on physical characteristics such as vegetation, ground cover, structures and so on, that may be readily described using land cover concepts. Consequently, and as several authors have pointed out, land use concepts is often intimately related to a fairly specific but complex and hard to define combination of land cover components appearing in characteristic relations to each other (Gong and Howarth Citation1992; Cihlar and Jansen Citation2001; Lillesand et al. Citation2004) similar to the notion of a relationship between urban form and function (Batty and Longley Citation1994).
In the early developments of classification procedures, land use and land cover were treated in a similar manner; each pixel was seen as the object to classify and this object was assumed to contain all components representative of a particular land use or land cover concept. In a seminal paper, Gurney and Townshend (Citation1983) argued that classes other than the original spectral classes could be separated based on the spatial context of classified pixels, and that the necessary rules to do this could be developed from very simple spatial context evaluations. Examples of spatial context information are distances or directions between different types of pixels and topological relations such as connectivity or containment. So far, examples of contextual image classification methods depend on physical features that are relatively distinct and unproblematic from a land cover classification accuracy standpoint, such as houses and roads in urban settings (Barnsley and Barr Citation1996; Herold et al. Citation2002; Barr et al. Citation2004), or pre-determined vegetation polygons in natural settings (Wicks, Smith, and Curran Citation2002). Other efforts limit the terminology to operate within a land cover hierarchy (Ju, Gopal, and Kolaczyk Citation2005; Weis, Müller, Liedtke, and Pahl Citation2005) assuming that derived classes aggregate naturally in an unproblematic manner. Additionally, spatial relations between objects are typically treated using very limited spatial extents (Bruzzone and Carlin Citation2006) despite the potential to include much wider spatial contexts, up to the range of an entire image scene.
Land use/land cover dynamics
In the emerging land use science (Rindfuss et al. Citation2007) a key challenge is to link the natural and human systems, and approach it as a coupled system because ‘… it is the interactions between the human and natural subsystems that produce land use and land cover change’ (Rindfuss, Walsh, Turner, Fox, and Mishra Citation2004, p. 13976). A main problem with this ambition relates to a large difference in disciplinary approaches, which makes it difficult to integrate social science knowledge with natural science data such as localized land cover data derived from remote-sensed images (Yarnal, Harrington, Comrie, Polsky, and Ahlqvist Citation2009). Furthermore, the traditional approach to land cover and land use is based on a static representation of something that is inherently dynamic and multidimensional, both in terms of physical characters and social activities over time.
Based on this we acknowledge two interlinked and related processes to consider in the study on land changes in remote-sensed data; dynamics of land use coming from changing intentions and practice; and dynamic vegetation change processes. Both are related through feedback loops and often with a delayed response in one process to a change in another, for example, vegetation response to changes in land use practice from grazing to mowing.
In this study we build on previous work by authors who showed that it is possible to establish a relation between a specific type of land use and remote-sensed data by making use of spatial contextual information inherent in the remote-sensed data itself (Wästfelt Citation2009). A subsequent study identified the possibility of using that same technique to identify different farming systems in the Sodo district in Southern Ethiopia (Wästfelt, Tegenu, Nielsen, and Malmberg forthcoming). Taken together these studies show that the method not only seems capable of detecting socioeconomic dimensions in remote-sensed data, but also that this can be done in dramatically different socioeconomic environments. In the current study we seek to go one step further and study a compound land use type, which to our knowledge is undergoing a transition into permanent forest. We also outline a formal framework for how to link the described quantitative process with human qualitative notions of land use.
Compound land use objects – the case of summer farms
Compound land use objects are recognized here as a mixture of land cover objects occurring in particular spatial contextual relationships. There are many examples of compound land use of interest to landscape researchers, for example tracking the changing size and configuration of land management units (Kuemmerlea, Hostertb, St-Louisa, and Radeloffa Citation2009), identification of neighborhoods (Webster Citation1996), and studies of urban sprawl (Irwin, Hyun Jin Cho, and Bockstael Citation2007). In our following case study we will look at summer farms to frame our discussion and evaluate our proposed approach. Summer farms are a type of settlements that traditionally were used for transhumance and common in many mountain regions especially in Europe (Cleary Citation1987; Larsson Citation2003). Transhumance can be defined as a system of livestock farming, which rests on the use of pastoral resources in complementary zones, which, by themselves, can only support livestock for part of the year. This meant that traditional summer farms were always located some distance away from the home farm but still maintained a functional relation with it. The summer farm itself typically consisted of small fields of arable land at its centre, surrounded by meadows, grazing areas, and surrounding forest, which also was used as pastureland historically (Larsson Citation2003). Consequently, there was a local spatial logic for how land use was organized and this logic is still expressed spatially in the landscape (, cf. Haggett Citation1979; Macmillan and Huang Citation2008). An additional characteristic is also the operative scale: fields and meadows are always relatively small as it has never been possible to manage these farms with machinery.
Figure 1. The spatial logic of a summer farm and the example of the Bastberget summer farm in an orthophoto and from the ground.
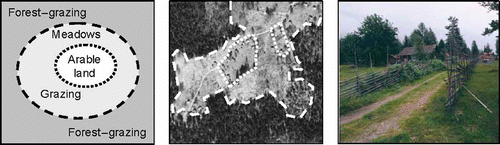
The summer farm praxis is mostly abandoned in northern Europe: a few summer farms are still in use but converted to summer cottages or museums. Thus, the intentions driving traditional land use have been replaced by new incentives. As a result the contemporary spatial expression of summer farms is complicated by remnants of former land use expressions mixed with new forms of land tenure. The differences between summer farms are also significant because of local variation and differing histories, but the base elements are still mixed in a characteristic manner, which we can associate with summer farms. As such we treat a summer farm as an assemblage concept, which can be used as an example of a compound land use concept, with transient function and incentives, but at the same time maintaining a distinctive form of spatial contextual expressions of different land covers. Furthermore, summer farms exhibit a particular spatial pattern that is hard to capture by traditional per-pixel remote sensing approaches (Burnett and Blaschke Citation2003). This provides a good case study for our demonstration and evaluation of a suggested framework for semiautomatic identification of proxies for land use.
We propose that a simple model, such as the graphic in the left panel in , can help and guide our understanding, serve as input to the formalization of a concept, and, more importantly, be enriched by detailed information from a satellite image that can further inform our understanding with the necessary local context. Operationally, and to support an automated remote sensing procedure, we need to identify what it is about a certain land use that contributes to a characteristic spatial expression, and then allow computer algorithms to identify and ‘learn’ how this can be extracted from available empirical image data.
Formalizing land use conceptualizations
If we try to identify summer farms in an aerial photograph, we would use our mental understanding and look for relatively small areas with several small houses that are surrounded by arable fields, pasture, and enclosed by forest. We would also expect these to appear within a reachable distance from existing permanent settlements. Hence, the occurrence of a certain set of objects in a particular spatial context allows us to infer that we are looking at a summer farm (, left).
Figure 2. Two different knowledge processes: the human, mental interpretation of a landscape scene on the left and machine-based numerical clustering and segmentation on the right.
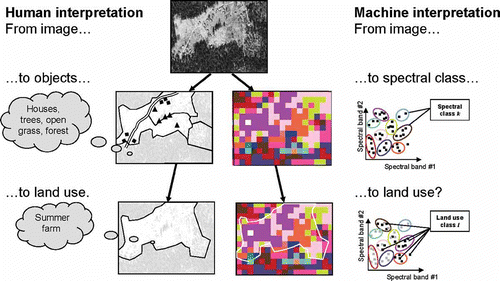
On the other hand, a formal process based on satellite imagery starts with the spectral energies reflected by the land cover. Certain types of ground cover have a characteristic reflection, which sometimes enables standard classification algorithms to establish a probabilistic relationship between a pixel with that spectral signature to a particular type of land cover, for example, coniferous trees, grass, or open water (cf. Lillesand et al. Citation2004). Again, the main problem in our case, and with many other spatially complex land use expressions, is that the land use is tied to a combination of many different types of land cover (, right).
These two processes exemplify two separate ways of identifying and reasoning about things in the landscape: one in which a formal, numerical search for patterns and clusters in a multivariate data (spectral) space forms the basic knowledge elements as classified pixels, and one in which a human observer interprets and identifies a complex of objects in an image of a landscape. They differ not so much in terms of the raw data since both operate on an image with spatially distributed spectral energy from which the basic elements are discerned. But the ensuing processes and outcomes are very different. The human cognitive process is highly complex and its formal specification is very much an active research field (cf. Lakoff Citation1987; Markman Citation1999; Muphy Citation2004). Somehow the human observer can use the image to elicit and combine mentally constructed objects such as houses, trees, and different types of grass areas, and associate these to general concepts of settlement types and infer specific forms of land use based on existing knowledge. However, much of this process is hard to define precisely, let alone formally. The machine-based process on the other hand is far more specific in how it transforms the image into spectrally distinct pixel objects based on some form of clustering process. We are naturally curious about the details of our mental reasoning and how we identify a particular land use, for example, what types of houses and fields; in what configuration; at what distance from other open land, roads, and forests. But all those details have limited value for guiding the formal process if the image does not contain those objects. The following two sections will help to exemplify this by breaking down the Human (H) and Machine (M)-based interpretation processes into tractable steps as outlined in .
Figure 3. An outline of the suggested absolute/relational classification and conceptual space representation. The human (H1–2) and machine knowledge elements (M1–7) are combined to produce a resulting proxy image for land use.
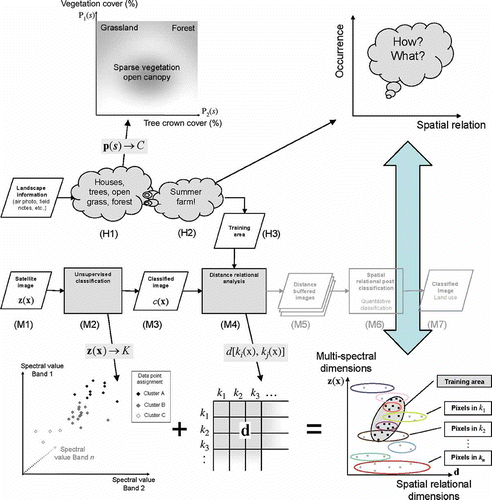
Formalization of human knowledge
The geographic information sciences have acknowledged and worked on documenting and exploring the semantics of geographic information and how to integrate category semantics into spatio-temporal analysis (Cai Citation2002; MacEachren, Gahegan, and Pike Citation2004; Arpinar et al. Citation2006). In terms of representing semantic knowledge several approaches are possible (Schwering Citation2008), and in this work we will illustrate how the above process can be cast in terms of the cognitive theory of conceptual spaces suggested by Gärdenfors (Citation2000). One of the core ideas behind this theory is that mental concepts can be described as geometrical and topological structures in a conceptual space.
Several efforts are underway regarding the application of conceptual spaces to the semantic modeling of geographic phenomena (Song and Bruza Citation2003; Ahlqvist Citation2004; Raubal Citation2004; Schwering and Raubal Citation2005). In this work we will use the ‘Uncertain Conceptual Spaces’ (Ahlqvist Citation2004) approach to define and reason with concepts in a multidimensional semantic space that uses fuzzy set (Zadeh Citation1965) and rough set (Pawlak Citation1992)-based constructs. Similar to the spectral classes, we can formally define concepts, such as land cover categories, in an n-dimensional conceptual space S defined by a collection of properties p identified as salient to the definition of the category of interest. p(
s
) represents the particular values of those properties: for example intervals of certain vegetation cover and tree crown cover percentage values associated with a particular concept, for example, ‘forest’. The mapping thus defines a function that links property values to one of the C concepts of interest ((H1) in ). Because of the vagueness and ambiguity inherent in so many geographic concepts (Fisher Citation2000) the values of p(
s
) can be given as rough fuzzy set definitions (Ahlqvist, Keukelaar and Oukbir Citation2003; Ahlqvist Citation2004) allowing graded or indeterminate boundaries between concepts. Furthermore, the importance of each property to the definition of a particular concept, c ∈ C, can be adjusted by assigning salience weights
w
, which can either use 0 or 1 for exclusion or inclusion, or any value in between for graded importance to the concept definition:
. Further details on the cognitive foundations for this concept representation approach can be found in reference Gärdenfors (Citation2000).
Ahlqvist (Citation2005) demonstrated the use of uncertain conceptual spaces for representing commonly used land use/land cover categories, for translating between different ontologies using semantic similarity metrics, and for detection of graded land cover changes (Ahlqvist Citation2008), using already classified data sets. This representational approach departs significantly from the classical view of categories (Medin Citation1989) with lists of necessary and sufficient features, and the first-order logic reasoning common in many formalizations of knowledge structures.
In our following case study we seek to better understand the human concept of summer farms ((H2) in ). Its formal definition is less tractable than (H1) as it is mostly qualitative, but from its description above it can be schematically conceptualized as two primary information dimensions: one that involves information about the existence of different landscape objects from (H1) and one that involves the spatial configuration of those objects. However, the exact definition of which objects and what relations therefore constitutes a typical summer farm is not known.
Formalization of machine knowledge
In a recent study Wästfelt (Citation2009) demonstrated the possibility of linking land use to complex spatial configurations from satellite data by making use of spatial contextual information supported by qualitative knowledge about land use in the area. His proposal, the Spatial Relational Post Classification (SRPC) method, makes it possible to separate two different land uses within a village despite the fact that they included mixtures of similar land cover types. Schematically the SRPC method incorporates both spectral and space-context criteria in a remote sensing image classification process.
Initially an unsupervised image classification (M2 in ) generates a large number of spectrally distinct classes. A main departure from most approaches is that these computer-generated landscape elements (M3) are accepted as information bearers, even though they do not necessarily mean anything to humans at this point. The computer is then allowed to learn what a target phenomena looks like by providing a training area over, in our case, a typical summer farm, identified based on our own understanding of the concept (H2). This training area is then used to statistically summarize simple spatial relationships between pixels of different spectral classes from (M3). The spatial arrangement is described as the ‘largest nearest neighbor’ relation for all land cover classes appearing in the training area. This relation combines an evaluation of the closest distance between any two patches of different classes and the largest distance from one of those patches to the farthest point in the other. This information is retained and stored for all pairs of classes (see reference Wästfelt Citation2009 for a detailed description). Using the largest–nearest neighbor distance helps to characterize the arrangement of the landscape in and around the training area since it will capture information not only on the relative proximity of different types of land cover classes, but also on the relative patch size. The spatial relation information (M4) is used to re-classify the original image (M3) into class-specific, distance-buffered images (M5) and combine them in a final overlay operation (M6) that selects landscape segments (M7) with similar spectral and spatial context as the training area. Using these simple relational criteria makes it possible to separate pixels from the same class into two or more target classes, that is, two pixels from the same land cover class can be separated into two different land use categories depending on their spatial context. Consequently, SRPC has a strong kinship to intelligence- or knowledge-based image classification as it incorporates external knowledge formalized into a given set of rules in order to mimic human interpretation (Gao Citation2009). SRPC can also be said to be a part of the emergence of non-per-pixel-based methods as exemplified by the Object Based Image Analysis research field and available software such as eCognition® that have followed as consequence of the increase in the supply of high-resolution satellite imagery (Gao Citation2009; Blaschke Citation2010; Schöpfer, Lang, and Strobl Citation2010). However, SRPC differs from these techniques since the identified locations are indicators of a specific combination of both spectral and spatial relationship properties. The selected areas are not only a result of the local character, but also dependent on patterns of surrounding areas.
Following the representational framework proposed by Goodchild, Yuan, and Cova (Citation2007) we can formally specify the machine-based knowledge components. The satellite image is conceived of as a geo-field with a piecewise constant, regular grid of square spatial cells over which spectral reflectance is measured. The discretization imposed by this spatial grid can be formalized as an equivalence relation creating an approximation space (X, θ) where the granularity imposed on the underlying space–time universe X is defined by an equivalence relation θ (Worboys Citation1998; Ahlqvist et al. Citation2003). A finite element of X, in our case a square pixel, is specified by x that defines a specific location in space–time. The full geo-field representation then becomes a collection of measurements of some property Z over (X, θ) such that it defines a function z = z(x) With multi-spectral satellite data we have multiple properties forming an m-dimensional hyperspace formally describing (M1) in .
Similar to the concept formalization above, the unsupervised classification process can be specified through a mapping where the combinations of spectral values at x are linked to one of K spectrally distinct classes. This mapping represents the knowledge component (M2) in , and the resulting image k(x) representing (M3) will be an instance of a geo-field as defined above but with a discrete scale based on the K classes.
To represent the distance relational knowledge component (M4) we generate a distance matrix d with elements by taking values from the evaluation of largest–nearest neighbor distances for pairwise combinations of the K spectrally distinct classes.
While the following knowledge components (M5–7) can be specified in a similar manner, we are mostly interested in these first four components as we are seeking to understand their mapping to their human knowledge counterparts (H1–3). In the following section we outline our proposed view on how the two formalized knowledge domains described above can be bridged.
Linking human and machine-based ways of knowing
Our final objective is to further identify and formalize the links between the formal representations of qualitative human knowledge about land use and quantitative measures of land cover from satellite images. Again, while steps M1–7 in are well-defined and formally described, the human components H1–2 are harder to specify. There are some results from many of the process steps above that help describe and possibly tie together the different knowledge components. We could for example calculate a statistical relation between the end result and identified ground truth data, but that still does not connect the empirical information with mental concept representations. Our ambition is to come closer to a linking of the socially constructed and conceptualized concepts associated with land use with the data-rich empirical information provided by satellite imagery. Through this we believe that it is possible to explore the complexity of land use through detailed accounts of the separate knowledge components and their potential relations.
The geometric interpretation of concept semantics and current practice in quantitative spatial analysis both have immediate relevance to the complex land use phenomena we have discussed above. First, we have demonstrated that the conceptual space formed by (H1) in is structurally similar to the empirical spectral space formed by (M2). But the equivalent of the spectral space in the human domain would be found at a sub-conceptual level as visual perception and this is hard to define. Therefore we find no potential direct link between these two knowledge components. However, there is a closer connection between the human conceptual space H2 and the machine-based (M2 and 4) representations. M4 provides one quantitative summary of distance relations in the classified image in M3. Gärdenfors (Citation2000, p. 93) pointed out that ‘… a relation between two objects can be seen as a simple case of a pattern of the location of the objects along a particular quality dimension …’ for example, dimensions that relate to the shape of objects. In the current context we mean that measures on the spatial relations, such as those described previously between land cover objects, represents such a qualitative dimension in the image, and that it also provides a crucial quantitative proxy for land use, which we can only qualitatively indicate in (H2). In other words, an in-depth spatial contextual analysis of the image could be seen as a formal semantic description of a qualitative dimension that mirrors the land use expressions in a landscape. In this manner the information generated by M1–4 supports a bottom-up description of spectral and spatial structures in empirical data that can provide insights as to how land uses relate to a specific spatial expression in the landscape. This bridge between the human and machine domains is established through the training area (H3) that serve as a boundary object (Star Citation1989; Harvey Citation1999) between the different conceptual worlds expressed in (M1–4) and (H1–2). In line with Gärdenfors' (Citation2000) arguments the conceptual spaces in act as a bridge between connectionist and symbolic levels by finding a common boundary object in the training area.
The case study
To illustrate and evaluate the outlined proposal we have conducted a case study based on summer farms west of the Swedish Town Borlänge. Our study area is approximately 32 × 20 km and a total number of 74 summer farms are known to have existed at some point in time. However, only 10 summer farms are still in use whereas 64 are partly or completely abandoned and to varying degrees overgrown, which illustrates a complex land use/landscape change process. Characteristics for the summer farms still in use are small abandoned fields, an open grazing land, and a surrounding old-growth forest. These elements can be spotted manually in aerial photographs as a distinct open area in a wide forest as in above. Abandoned and overgrown summer farms are harder to identify, but some traces can still be found in the patterns of secondary overgrowth when the time of abandonment is not too long ago.
Data and methods
For the case study a summer farm called Bastberget, one of the summer farms still in use, was selected as a training area (H3 in ). Bastberget was chosen because of its maintained focus on traditional land use management. As primary data (M1 in ) a Landsat satellite image (Landsat 7 scene 194/18, from 28 July 2000) was used for the unsupervised classification step in the SRPC routine, and resulted in 40 spectral classes (M2–3). The subsequent step extracted the distance matrix (M4) holding the spatial relational distances between all classes in the training area. The final SRPC step produced a binary classification with pixels coded 1 where the combined spectral and spatial contextual characteristics matched that of the training area. All other pixels were coded 0.
The spatial representation of summer farms is a challenging issue and it generates some problems for the assessment to follow. As described above, the location of a summer farm is determined by a series of factors including the spatial context. A point representation could represent some central location of the farm, but would not accurately represent the area of influence, whereas an area representation would have to determine where the border of this influence area is. Although the core area of houses and open fields is sometimes well-defined, the actual summer farm land use influence extends well beyond those boundaries. Consequently, individual pixels in the classification result represent a summary of local context characteristics. Similarly, the reference data, representing summer farm locations as points, also represent an area larger than that projected by the point location. Therefore, current principles and practices for classification accuracy assessment (cf. Congalton and Green Citation1999) cannot be applied to the data at hand without some modification. The reason for this is that a simple pixel-by-pixel comparison would introduce both omission and commission errors in an unforeseeable manner.
Point locations of summer farms known to have existed in the study area (The County Administrative Board Dalarna) serve as our reference and ground truth data set. The reference summer farms were separated into three types based on our own field work and interpretations of aerial photographs: Type-1, representing currently used summer farms; Type-2, abandoned but not completely overgrown; and Type-3, overgrown with remnants in vegetation. The rationale for this separation is that although Type-2 and 3 locations represent former summer farms, they now represent a very different land use compared with the target Type-1 as it is exemplified by the training area, a fairly large, currently used summer farm. The ground truth data thus represent a temporal gradient from distinct and currently used summer farms, through objects in various stages of overgrowth, to summer farms that have been abandoned for a long time and are now hard to find even when searching for traces in the field. Since our training area represents Type-1 summer farms, we expect to identify this type with the highest accuracy and Types-2 and 3 with less accuracy since these are various stages of overgrowth and less representative of the training data.
To indicate some amount of areal extent beyond the point locations, we add a 400-m buffer around the reference points. The choice of a 400-m limit is based on the training area and its match with the corresponding reference point. Using these 400-m buffer areas, any intersection with output pixels from the classification process was recorded as a successful match. All other known summer farms that were not within 400 m from an output pixel were counted as not indicated by the process.
Following the previous argument that the training area serves as a link between the automatic processing of image data through the distance matrix and the qualitative human conceptual spaces, we performed two evaluations of the proposed framework. One is an overall accuracy assessment of the derived image as a proxy for summer farm land use, evaluated as a ratio of correctly identified reference areas over the total number of reference areas in each respective category (cf. Congalton and Green Citation1999). The second evaluation is statistical in nature and evaluates the null hypothesis that the outcome is just one of many possible outcomes of completely random guesses. Through randomization we compare the derived outcome with many other possible outcomes and use the generated empirical distribution to evaluate the probability that the actual outcome is significantly different from that of guessing locations at random. The null hypothesis may seem like an overly pessimistic assumption, but it gives us at least a firm estimate of the process performance
For overall accuracy we simply count the number of actual reference areas that coincide with at least one pixel classified as summer farm by the SRPC process. Although this overall accuracy can give an idea of whether the method works well or not, it is hard to say something affirmative about the results: it could be possible to hit some reference areas by mere chance. To address this we conducted the randomization test by performing a random placement of 20,000 400-m buffers in the study area, calculating the probability that one randomly placed buffer would overlap with a pixel in the SRPC result. With this information it is possible to calculate the probability for the different types of summer farm categories result being a product of chance with the help of a binomial probability distribution.
Results
The known summer farm locations are presented as colored circles alongside the result from the SRPC routine in .
Figure 4. A map showing the location of the three different types of summer farms in the study area, and the result from the SRPC process overlaid on a b/w orthophoto, with the yellow color tone on developed areas and the green tone on other land. Type-1: In use; Type-2: Recently abandoned; Type-3: Overgrown.
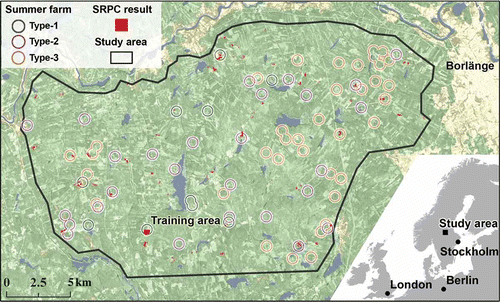
The number of reference areas and the number of classification matches with such areas are summarized in . The first column in shows the absolute number of summer farms in Type-1 (3 + 6), excluding the training area, and the number of summer farms indicated by SRPC (6) in the output classification. The second and third columns show the same numbers for reference summer farms of Type-2 (21 + 6) and 3 (31 + 6) as well as the number of summer farms identified by SRPC.
Figure 5. A summary assessment of the SRPC classification illustrating the number of correct identifications of the known locations of summer farms Types 1–3 and the number of non-identified locations.
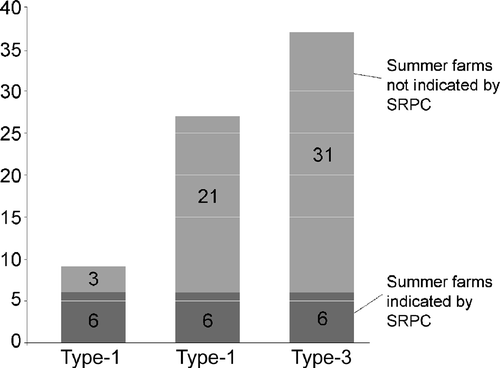
In we see that 6 of 9 known Type-1 summer farms coincide with the classification output, that is, an overall accuracy of 66%. For Types-2 and 3 the quantity is 6 of 27 and 6 of 37, or 22% and 16% overall accuracy, respectively. Thus, the method picks out the target summer farm, Type-1, with a higher ratio of correspondence than for Types-2 and 3. This was expected as Types-2 and 3 are abandoned and the vegetation has made these former summer farms more or less overgrown. It also indicates that we are capturing an additional dimension: the landscape dynamics in the form of the relative abandonment and overgrowing process of summer farms. In this case this is expressed by the decrease in co-occurrence for Types-2 and 3. In addition, the result also identifies a number of places that do not correspond with known summer farms. These places hold the same spectral–spatial configuration of land covers, which can indicate unknown summer farms or other types of land use producing the same spectral–spatial configurations.
In the statistical test 2460 of the 20,000 randomly placed 400-m buffer areas overlapped with at least one pixel in the SRPC output. In other words, there is a 12.3% chance that a randomly placed buffer overlaps with the SRPC result. This baseline probability, π = 0.123, was used to derive four binomial distributions for the statistical evaluation. In the binominal distribution for Type-1 (n = 9, π = 0.123) summer farms are presented, where n is the number of summer farms in the category and the function gives the cumulative probability for any number of overlaps less than x. Similarly, binominal distributions are displayed for n = 27, 37, and 73, corresponding to the reference data for the two other summer farm types, 2 and 3, and all types together.
Figure 6. Cumulative binominal distributions for n = 9, 27,37, and 73 randomly placed buffer overlaps corresponding to the number of reference farms of type 1, 2, 3, and all types respectively.
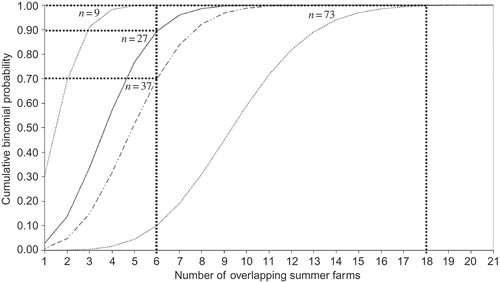
For the analysis, the actual number of summer farms identified by the method was compared to the cumulative probability of any x equal to or larger than that outcome (see vertical lines in ). This is particularly important for large n values when a specific p(x) can become very small and not representative of the probability we look for.
As evidenced by and the Type-1 results cannot be attributed to chance as the probability for that is as low as 0.021%. Type-2 and Type-3 on the other hand are not significantly different from a chance result at 10% and 30% likelihood, respectively. All types' results, on the other hand, are a combination of the previous three and not likely to happen by chance (0.27% chance).
Table 1. Results of binominal distributions
Concluding discussion
We have presented a framework for satellite data interpretation, which considers and incorporates land use semantics in the classification process and into the result. Through a series of classification and post-classification processes we are able to use the identified contextual relations to reclassify different ‘standard’ land cover classes into proxies for land use categories. A detailed specification of each step also enables the creation of quantitative and qualitative dimensions, which help to semantically describe the used and derived categories in formally defined conceptual spaces.
We have outlined an approach that closes in on how to match the technical descriptions of the machine-based process with a semantic specification of compound land use concepts. By focusing attention to the semantic dimensions, the conceptualization of entities in a landscape and its influence on descriptions of land use, we have shown how it may become possible to give more detailed accounts of how to model land use in a geographical landscape. The parallel formal descriptions of geographical concepts based on uncertain conceptual spaces (Ahlqvist Citation2004) together with the SRPC technique (Wästfelt Citation2009) suggest a framework for analysis of the relations between those two ways of knowing. Our empirical results show that such relations can be established through the training area boundary object that acts as a mediator for compatible but epistemologically different concept descriptions. The progressive decline in classification matches from summer farm Types-1–3 indicates that there is a historical transition aspect to the landscape expression that can be recognized in this study. By using the presented technique iteratively through using other training areas it would be possible to map this historical dimension in one and the same remote-sensed image. As such we do not view our results as just a new type of classification routine. Our approach enriches the satellite data with a qualitative perspective built on knowledge and concepts associated with traditional land use, opening opportunities to formally explore the ontological complexity. Despite the specific nature of this example, it generalizes to other contemporary phenomena of interest to landscape change researchers. For example, the idea of urban sprawl can be regarded as a compound land use object where there is currently no consensus on how to define and measure changes (Siedentop and Fina Citation2010; Zhang, Guindon, and Sun Citation2010), and we hope to provide useful insights in this area in our future research. Consequently we argue that our presentation demonstrates that it is possible for remote sensing technology to come closer to identifying other such land use processes if we deepen the knowledge about how to semantically guide the machine-based procedure.
The scope of this paper has not allowed us to go further into the constitutive, telic, and agentive complexity that is internalized by land use concepts (Bibby and Shepherd Citation2000). However, we believe that further studies of the interrelationships between the multiple uncertain concept spaces may shed some light on that complexity. For example, the focus on land use provides the potential for a deeper insight into current landscape changes at an intentional level, and also makes it possible to recognize and understand differences in actual ongoing land use and temporal changes in terms of relative time connections with former land use. This indicates to us that the presented approach provides a potential to analyze landscapes in new ways, especially by enabling a focus on the qualitative aspects of the land and environment using satellite data.
References
- Ahlqvist , O. 2004 . A Parameterized Representation of Uncertain Conceptual Spaces . Transactions in GIS , 8 : 493 – 514 .
- Ahlqvist , O. 2005 . Using Uncertain Conceptual Spaces to Translate Between Land Cover Categories . International Journal of Geographical Information Science , 19 : 831 – 857 .
- Ahlqvist , O. 2008 . Extending Post Classification Change Detection Using Semantic Similarity Metrics to Overcome Class Heterogeneity: A Study of 1992 and 2001 National Land Cover Database Changes . Remote Sensing of Environment , 112 : 1226 – 1241 .
- Ahlqvist , O. , Keukelaar , J. and Oukbir , K. 2003 . Rough and Fuzzy Geographical Data Integration . International Journal of Geographical Information Science , 17 : 223 – 234 .
- Aplin , P. 2004 . Remote Sensing: Land Cover . Progress in Physical Geography , 28 : 283 – 293 .
- Arpinar , B. , Sheth , A. , Ramakrishnan , C. , Usery , Azami , M. and Kwan , M.-P. 2006 . Geospatial Ontology Development and Semantic Analytics . Transactions in GIS , 10 : 551 – 575 .
- Barnsley , M.J. and Barr , S.L. 1996 . Inferring Urban Land Use From Satellite Sensor Images Using Kernel-Based Spatial Reclassification . Photogrammetric Engineering and Remote Sensing , 62 : 949 – 958 .
- Barr , S.L. , Barnsley , M.J. and Steel , A. 2004 . On the Separability of Urban Land-Use Categories in Fine Spatial Scale Land-Cover Data Using Structural Pattern Recognition . Environment and Planning B-Planning & Design , 31 : 397 – 418 .
- Bartholome , E. and Belward , A.S. 2005 . GLC2000: A New Approach to Global Land Cover Mapping From Earth Observation Data . International Journal of Remote Sensing , 26 : 1959 – 1977 .
- Batty , M. and Longley , P.A. 1994 . Fractal Cities: A Geometry of Form and Function , London : Academic Press .
- Bibby , P. and Shepherd , J. 2000 . GIS, Land Use, and Representation . Environment and Planning B-Planning & Design , 27 : 583 – 598 .
- Blaschke , T. 2010 . Object Based Image Analysis for Remote Sensing . ISPRS Journal of Photogrammetry and Remote Sensing , 65 : 2 – 16 .
- Bowker , G.C. and Star , S.L. 1999 . Sorting Things Out: Classification and Its Consequences , Inside Technology Series Cambridge , MA : MIT Press .
- Brandt , J. , Primdahl , J. and Reenberg , A. 1999 . “ Rural Land-Use and Landscape Dynamics–Analysis of ‘Driving Forces’ in Space and Time ” . In Land-Use Changes and Their Environmental Impact in Rural Areas in Europe , Edited by: Krönert , R. , Baudry , J. , Bowler , I.R. and Reenberg , A. 81 – 102 . Paris : UNESCO and the Parthenon Publication Group .
- Brown , D.G. and Duh , J.D. 2004 . Spatial Simulation for Translating From Land Use to Land Cover . International Journal of Geographical Information Science , 18 : 35 – 60 .
- Bruzzone , L. and Carlin , L. 2006 . A Multilevel Context-Based System for Classification of Very High Spatial Resolution Images . IEEE Transactions on Geoscience and Remote Sensing , 44 : 2587 – 2600 .
- Burnett , C. and Blaschke , T. 2003 . A Multi-scale Segmentation/Object Relationship Modelling Methodology for Landscape Analysis . Ecological Modelling , 168 : 233 – 249 .
- Cai , G.R. GeoVIBE: A Visual Interface for Geographic Digital Libraries . paper presented at the 2001 ACM-IEEE JCDL Workshop on Visual Interfaces .
- Campbell , J.B. 2007 . Introduction to Remote Sensing , New York : The Guilford Press .
- CEC . 1995 . “CORINE Land Cover,” , Luxembourg : Commission of the European Communities .
- Cihlar , J. and Jansen , L.J.M. 2001 . From Land Cover to Land Use: A Methodology for Efficient Land Use Mapping Over Large Areas . Professional Geographer , 53 : 275 – 289 .
- Cleary , M.C. 1987 . Contemporary Transhumance in Languedoc and Provence . Geografiska Annaler Series B-Human Geography , 69 : 107 – 113 .
- Comber , A. , Fisher , P. and Wadsworth , R. 2005 . What is Land Cover? . Environment and Planning B-Planning & Design , 32 : 199 – 209 .
- Congalton , R.G. and Green , K. 1999 . Assessing the Accuracy of Remotely Sensed Data: Principles and Practices , Boca Raton , FL : Lewis Publications .
- Crampton , J.W. 2001 . Maps as Social Constructions: Power, Communication and Visualization . Progress in Human Geography , 25 : 235 – 252 .
- Crapper , P.F. 1984 . An Estimate of the Number of Boundary Cells in a Mapped Landscape Coded to Grid Cells . Photogrammetric Engineering and Remote Sensing , 50 : 1497 – 1503 .
- Defries , R.S. and Belward , A.S. 2000 . Global and Regional Land Cover Characterization From Satellite Data: an Introduction to the Special Issue . International Journal of Remote Sensing , 21 : 1083 – 1092 .
- Di Gregorio , A. 2004 . Land Cover Classification System (LCCS), Version 2: Classification Concepts and User Manual , Rome : Food and Agriculture Organization of the United Nations .
- Di Gregorio , A. and Jansen , L.J.M. 2000 . Land Cover Classification System: LCCS: Classification Concepts and User Manual , Rome : Food and Agriculture Organization of the United Nations .
- Erb , K.-H. , Gaube , V. , Krausmann , F. , Plutzar , C. , Bondeau , A. and Haberl , H. 2007 . A Comprehensive Global 5 min Resolution Land-Use Data Set for the Year 2000 Consistent With National Census Data . Journal of Land Use Science , 2 : 191 – 224 .
- Fisher , P. 2000 . Sorites Paradox and Vague Geographies . Fuzzy Sets and Systems , 113 : 7 – 18 .
- Fisher , P. , Comber , A. and Wadsworth , R. 2005 . “ Land Use and Land Cover: Contradiction or Complement ” . In Re-presenting GIS , Edited by: Fisher , P. and Unwin , D. 85 – 98 . Chichester : Wiley .
- Fisher , P.F. and Pathirana , S. 1990 . The Evaluation of Fuzzy Membership of Land Cover Classes in the Suburban Zone . Remote Sensing of Environment , 34 : 121 – 132 .
- Fonseca , F. , Egenhofer , M. , Agouris , P. and Camara , G. 2002 . Using Ontologies for Integrated Geographic Information Systems . Transactions in GIS , 6 : 231 – 257 .
- Foody , G.M. and Boyd , D.S. 1999 . Detection of Partial Land Cover Change Associated With the Migration of Inner-Class Transitional Zones . International Journal of Remote Sensing , 20 : 2723 – 2740 .
- Gahegan , M. and Flack , J. 1999 . The Integration of Scene Understanding Within a Geographic Information System: A Prototype Approach for Agricultural Applications . Transactions in GIS , 3 : 31 – 49 .
- Gao , J. 2009 . Digital Analysis of Remotely Sensed Imagery , New York : McGraw Hill .
- Gärdenfors , P. 2000 . Conceptual Spaces: The Geometry of Thought , Cambridge , MA : MIT Press .
- GLP . Science Plan and Implementation Strategy . IGBP Report No. 53 . 2005 . Stockholm, Sweden: IGBP Secretariat
- Gong , P. and Howarth , P.J. 1992 . Land-Use Classification of Spot HRV Data Using a Cover-Frequency Method . International Journal of Remote Sensing , 13 : 1459 – 1471 .
- Goodchild , M.F. , Yuan , M. and Cova , T.J. 2007 . Towards a General Theory of Geographic Representation in GIS . International Journal of Geographical Information Science , 21 : 239 – 260 .
- Gopal , S. and Woodcock , C. 1994 . Theory and Methods for Accuracy Assessment of Thematic Maps Using Fuzzy-Sets . Photogrammetric Engineering and Remote Sensing , 60 : 181 – 188 .
- Gruber , T.R. 1993 . A Translation Approach to Portable Ontology Specifications . Knowledge Acquisition , 5 : 199 – 220 .
- Gurney , M.C. and Townshend , J.R.G. 1983 . The Use of Contextual Information in the Classification of Remote Sensed Data . Photogrammetric Engineering and Remote Sensing , 49 : 55 – 64 .
- Haggett , P. 1979 . Geography: A Modern Synthesis , New York : Harper International .
- Harvey , F. 1999 . “ Designing for Interoperability: Overcoming Semantic Differences ” . In Interoperation Geographic Information Systems , Edited by: Goodchild , M.F. , Egenhofer , M. , Fegeas , R. and Kottman , C. 85 – 97 . Norwell , MA : Kluwer Academic Publishers .
- Harvey , F. and Chrisman , N. 1998 . Boundary Objects and the Social Construction of GIS Technology . Environment and Planning A , 30 : 1683 – 1694 .
- Herold , M. , Scepan , J. and Clarke , K.C. 2002 . The Use of Remote Sensing and Landscape Metrics to Describe Structures and Changes in Urban Land Uses . Environment and Planning A , 34 : 1443 – 1458 .
- Hoeschele , W. 2000 . Geographic Information Engineering and Social Ground Truth in Attappadi, Kerala State, India . Annals of the Association of American Geographers , 90 : 293 – 321 .
- Irwin , E.G. , Hyun Jin Cho and Bockstael , N.E. 2007 . Measuring the Amount and Pattern of Land Development in Nonurban Areas . Review of Agricultural Economics , 29 ( 3 ) : 494 – 501 .
- Jansen , L.J.M. and Di Gregorio , A. 2002 . Parametric Land Cover and Land-Use Classifications as Tools for Environmental Change Detection . Agriculture Ecosystems & Environment , 91 : 89 – 100 .
- Ju , J.C. , Gopal , S. and Kolaczyk , E.D. 2005 . On the Choice of Spatial and Categorical Scale in Remote Sensing Land Cover Classification . Remote Sensing of Environment , 96 : 62 – 77 .
- Kalensky , Z.D. 1998 . AFRICOVER: Land Cover Database and Map of Africa . Canadian Journal of Remote Sensing , 24 : 292 – 297 .
- Kuemmerlea , T. , Hostertb , P. , St-Louisa , V. and Radeloffa , V.C. 2009 . Using Image Texture to Map Farmland Field Size: A Case Study in Eastern Europe . Journal of Land Use Science , 4 ( 1 ) : 85 – 107 .
- Lakoff , G. 1987 . Women, Fire, and Dangerous Things: What Categories Reveal About the Mind , Chicago , IL : University of Chicago Press .
- Larsson , J. 2003 . Vad är en fäbod? Ett försök att definiera ett begrepp . Bebyggelsehistorisk Tidskrift , 45 : 131 – 142 .
- Lillesand , T.M. , Kiefer , R.W. and Chipman , J.W. 2004 . Remote Sensing and Image Interpretation , New York : Wiley .
- MacEachren , A.M. , Gahegan , M. and Pike , W. 2004 . Visualization for Constructing and Sharing Geo-scientific Concepts . National Academy of Sciences , 101 : 5279 – 5286 .
- Macmillan , W. and Huang , H.Q. 2008 . An Agent-Based Simulation Model of a Primitive Agricultural Society . Geoforum , 39 : 643 – 658 .
- Markman , A.B. 1999 . Knowledge Representation , Mahwah , NJ : Lawrence Erlbaum Associates .
- Medin , D.L. 1989 . Concepts and Conceptual Structure . American Psychologist , 44 : 1469 – 1481 .
- Muphy , G.L. 2004 . The Big Book of Concepts , Cambridge , MA : MIT Press .
- Pawlak , Z. 1992 . Rough Sets: Theoretical Aspects of Reasoning About Data , Norwell , MA : Kluwer Academic Publishers .
- Perkins , C. 2003 . Cartography: Mapping Theory . Progress in Human Geography , 27 : 341 – 351 .
- Raubal , M. 2004 . “ Formalizing Conceptual Spaces ” . In Formal Ontology in Information Systems, Proceedings of the Third International Conference (FOIS 2004) , Edited by: Varzi , A.C. and Vieu , L. 153 – 164 . Amsterdam : IOS Press .
- Rindfuss , R.R. , Entwisle , B. , Walsh , S.J. , Mena , C.F. , Erlien , C.M. and Gray , C.L. 2007 . Frontier Land Use Change: Synthesis, Challenges, and Next Steps . Annals of the Association of American Geographers , 97 : 739 – 754 .
- Rindfuss , R.R. , Walsh , S.J. , Turner , B.L. , Fox , J. and Mishra , V. 2004 . Developing a Science of Land Change: Challenges and Methodological Issues . Proceedings of the National Academy of Sciences of the United States of America , 101 : 13976 – 13981 .
- Robbins , P. 2001 . Fixed Categories in a Portable Landscape: The Causes and Consequences of Land-Cover Categorization . Environment and Planning A , 33 : 161 – 179 .
- Schöpfer , E. , Lang , S. and Strobl , J. 2010 . “ Segmentation and Object-Based Image Analysis ” . In Remote Sensing of Urban and Suburban Areas , Edited by: Rashed , T. and ürgens , C. J . 181 – 192 . Heidelberg, Berlin: Springer .
- Schuurman , N. 2006 . Formalization Matters: Critical GIS and Ontology Research . Annals of the Association of American Geographers , 96 : 726 – 739 .
- Schwering , A. 2008 . Approaches to Semantic Similarity Measurement for Geo-spatial Data: A Survey . Transactions in GIS , 12 : 5 – 29 .
- Schwering , A. and Raubal , M. 2005 . Measuring Semantic Similarity Between Geospatial Conceptual Regions . Lecture Notes in Computer Science , 3799 : 90 – 106 .
- Shapiro , C. Coordination and Integration of Wetland Data for Status and Trend and Inventory . Technical Report No. TR2, Federal Geographic Data Committee, Wetlands Subcommittee . 1995 .
- Siedentop , S. and Fina , S. 2010 . Monitoring Urban Sprawl in Germany: Towards a GIS-Based Measurement and Assessment Approach . Journal of Land Use Science , 5 ( 2 ) : 73 – 104 .
- Song , D. and Bruza , P.D. 2003 . Towards Context Sensitive Information Inference . Journal of the American Society for Information Science and Technology , 54 : 321 – 334 .
- Star , S.L. 1989 . “ The Structure of Ill-Structured Solutions: Boundary Objects and Heterogeneous Distributed Problem Solving ” . In Distributed Artificial Intelligence , Edited by: Glasser , L. and Hahns , M.N. 37 – 54 . San Francisco , CA : Morgan Kaufmann Publishers Inc .
- The County Administrative Board Dalarna . Geographical Register of Known Sites for Former Summer Farms . Falun Sweden (in Swedish) ,
- Treitz , P. and Rogan , J. 2004 . Remote Sensing for Mapping and Monitoring Land-Cover and Land-Use Change . Progress in Planning , 61 : 267 – 279 .
- Turner , B.L. , Skole , D. , Sanderson , S. , Fischer , G. , Fresco , L. and Leemans , R. 1995 . Land-Use and Land-Cover Change: Science/Research Plan , Stockholm , Sweden : IGBP Secretariat .
- Verburg , P.H. and Veldkamp , A. 2005 . Introduction to the Special Issue on Spatial Modeling to Explore Land Use Dynamics . International Journal of Geographical Information Science , 19 : 99 – 102 .
- Wainwright , J. and Bryan , J. 2009 . Cartography, Territory, Property: Postcolonial Reflections on Indigenous Counter-Mapping in Nicaragua and Belize . Cultural Geographies , 16 : 153 – 178 .
- Wästfelt , A. 2009 . Land Use Qualities Identified in Remotely-Sensed Images . International Journal of Remote Sensing , 30 : 2411 – 2427 .
- Wästfelt , A. , Tegenu , T. , Nielsen , M. and Malmberg , B. Mapping Enset-Based Farming Systems in the Sodo District of Ethiopia using Satellite Data and Spatial Relational Post-Classification , forthcoming
- Webster , C.J. 1996 . Urban Morphological Fingerprints . Environment and Planning B-Planning & Design , 23 : 279 – 297 .
- Weis , M. , Müller , S. , Liedtke , C.E. and Pahl , M. 2005 . A Framework for GIS and Imagery Data Fusion in Support of Cartographic Updating . Information Fusion , 6 : 311 – 317 .
- Wicks , T.E. , Smith , G.M. and Curran , P.J. 2002 . Polygon-Based Aggregation of Remotely Sensed Data for Regional Ecological Analyses . International Journal of Applied Earth Observation and Geoinformation , 4 : 161 – 173 .
- Worboys , M. 1998 . Imprecision in Finite Resolution Spatial Data . GeoInformatica , 2 : 257 – 279 .
- Yarnal , B. , Harrington , J. , Comrie , A. , Polsky , C. and Ahlqvist , O. 2009 . “ Lessons Learned from the HERO Project ” . In Sustainable Communities on a Sustainable Planet: The Human-Environment Regional Observatory Project , Edited by: Yarnal , B. , Polsky , C. and ’Brien , J. O . 317 – 338 . Cambridge : Cambridge University Press .
- Zadeh , L.A. 1965 . Fuzzy Sets . Information and Control , 8 : 338 – 353 .
- Zhang , Y. , Guindon , B. and Sun , K. 2010 . Measuring Canadian Urban Expansion and Impacts on Work-Related Travel Distance: 1966–2001 . Journal of Land Use Science , 5 : 217 – 235 .