Abstract
In the Brazilian Amazonia, extensive and durable deforestation is led by ranching profits and increased demand for meat in large, globalized markets. A closer look at the arc of deforestation in southern Amazonia shows deforestation moving northward from ranching hotspots into surrounding frontiers as roads are opened. In the case of the Alta Floresta frontier, however, the road network has become dense enough that topographic features (land flatness and land away from river flooding plains) are now the significant risk factors of forest loss. Conversely, riparian corridors seem to be avoided by deforestation, as water and shade are vital for cattle weight gain or the corridors are waterlogged. Local interactions, expressed as spatial autocorrelation in multivariate models or neighbors in cellular automata forecasts, are also significant. In the short run, these local interactions produce a pattern of irreversible edge deforestation around pastures and fragmentation. But in the long run, spillovers outside of the study area can be expected as a result of economic momentum and the exhaustion of cattle-suitable areas in Alta Floresta.
1. Introduction
Tropical deforestation in the wake of cattle ranching expansion is a phenomenon almost exclusive to tropical America (Lambin and Geist Citation2003). Landscape effects result from a trial-and-error search for agriculturally suitable land using climate, terrain, or soil cues and leading to non-random land conversion (Carpenter, Mayorga, Quintero, and Schroeder Citation2001; Lomolino and Perault Citation2004). Predictions have become a standard way to understand currently significant attractive features of the landscape, as well as future landscape consequences. Here, the ranching expansion in Brazilian Amazonia is remotely sensed in the Alta Floresta frontier, a spatial regression is fitted, and cellular automata are used to forecast future land patterns. The interpretation of results is in terms of land use decisions based both on perceptible cues and emergent properties (such as fragmentation, edge deforestation, and spillovers).
1.1. Problem statement and research questions
This study focuses on identifying the spatial features of deforestation related to ranching expansion. It is hypothesized that some features are cues for land use choices that diminish economic costs, such as proximity to roads which lower transport cost between ranches and meat markets, or proximity to rivers and gentle slopes associated with vegetation growth and water drainage, and hence quality of pastures. Proximity to already deforested areas and dry forest edges would also diminish the effort of forest conversion into pasture. These land use choices make for non-random deforestation, which can be measured as spatial autocorrelation (SA). But due to this simple economic rule of proximity, emergent properties such as edge effects or fragmentation are likely to become apparent on the landscape. If this deforestation logic is taken further in time, additional properties, such as spatial spillovers, may occur beyond the study area. In the following sections, the features of deforestation expansion are examined, starting with roads and topography, continuing with fragmentation and edge effects and then spatial spillovers. This would then allow for the following research questions to be answered: Which are the significant features of ranching-related deforestation expansion? How can the spatial features of deforestation be economically interpreted? Can models with fairly well-known characteristics render all those features and help gauge their relative weight? What are the ultimate consequences of continuing deforestation? Are there any drivers that could curb the trend of deforestation?
1.2. Expansion
The expansion of cattle ranching in Brazil in recent decades has obeyed external markets driven by the nutrition transition that has increased demand for meat (Popkin Citation1993; Kaimowitz, Mertens, Wunder, and Pacheco Citation2004). The historical core of ranching in Brazil lies in the south and southwest regions. But the expansion has reached the Amazonian arc of deforestation, an irregular polygon of disjoint crop and cattle hotspots (). In the immediacies of these hotspots, ranching frontiers have developed with lower cattle densities but deeper penetration in Amazonia.
Figure 1. Study area. The ranching expansion occurs at different scales. It exhibits a core–periphery pattern in Brazil as shown by cattle density (FAO Citation2003). In the arc of deforestation, hotspots of high cattle density are surrounded by frontiers that are in contact with primary forest. In the Alta Floresta frontier under study here, a centrifugal deforestation has taken place centered on the municipal central points. Edge effects and new deforestation spots may now be dominant patterns. The former intensify land use by keeping forest regrowth at bay. The latter extend deforestation. Together they are an expression of the land use transition that posits gradually expanding and intensifying human land encroachment (Foley et al. Citation2005). This responds to land attractiveness (higher primary productivity in Amazonia and low land prices). The ecotone between the Cerrado savannah and the Amazonian moist forest is seemingly more attractive providing pastures with moisture but also making fire management easier. The arc of deforestation is located in this ecotone. Alta Floresta is also a contact region or transition area between forest and savannah (WWF 2006), and edge deforestation replicates this balance of dryness and moisture. The arc of deforestation was outlined based on Advanced Very High Resolution Radiometer (AVHRR) and Moderate Resolution Imaging Spectroradiometer (MODIS) data (DeFries et al. Citation2000; Hansen et al. Citation2003). The arc is composed of tree cover losses ≥ 50% in 1992–2001. The outline was consistent with two independent 1992 and 2001 data sets (Eva et al. Citation2002; Walker and Qi 2009). This outline coincided to a great degree with the risk associated with cattle density (de las Heras and Lake Citation2008).
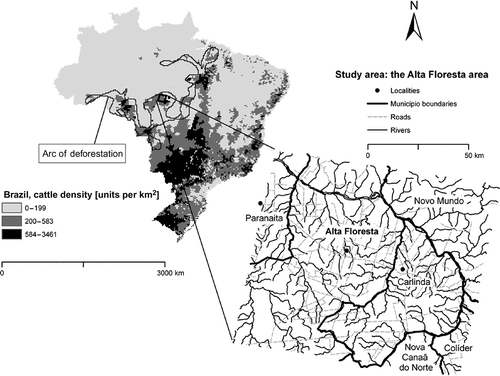
The reasons for the greater impact of cattle ranching in comparison with other land uses are low beef productivity and increasing meat demand leading to large-scale grazing and suppression of fallow, as well as speculation on pastures prompted by state subsidies (Hecht Citation1985; Smith et al. Citation1999; Mertens, Poccard-Chapuis, Piketty, Lacques, and Venturieri Citation2002; Fearnside Citation2005). This can explain why cattle ranches seem larger than crop farms: settlers, in two Brazilian Amazon colonies, clear 2–3 ha per year per average family (Fujisaka, Bell, Thomas, Hurtado, and Crawford Citation1996), while small ranchers are granted 50–100 ha by the State, or 76 ha on average in a typical 6-person ranching household, and large ranchers have in excess of 1000 ha (Walker, Moran, and Anselin Citation2000; Vosti, Braz, Carpentier, D'Oliveira, and Witcover Citation2003). In addition, small and medium-sized agricultural landholdings practice a 2–3 years fallow while pastures are burnt on a regular basis to hinder secondary growth (Cochrane Citation2003) during 6–15 years depending on location, soil quality, and management (Eden Citation1998; Seidl, Silva, and Moraes Citation2001; Vosti et al. Citation2003). Large (>1000 ha) and medium (250–1000 ha) ranches occupy 80–95% of their land as pasture as against an official limit of 50% in Brazilian Amazonia (Pedlowski, Dale, Matricardi, and da Silva Filho Citation1997), a limit which is now 20%. When restrictions to expansion appear, the tendency is either to work more intensively or to buy in more remote areas (Mertens et al. Citation2002).
Some evidence also points to ranching frontiers being more ‘expansive’ than other deforestation fronts. Firstly, pastures seem to be an end point for several land use transition pathways: forest is either converted directly to pastures or to profit-driven cropland and then pastures (Fujisaka et al. Citation1996; Mertens et al. Citation2002; Cardille and Foley Citation2003; Vosti et al. Citation2003), leading to cattle-specialized areas (Cochrane Citation2001; Seidl et al. Citation2001) and estimates that cattle ranching accounts regionally for up to 70% of deforestation (Walker et al. Citation2000; Cerri et al. Citation2004). More recently, soya bean has been cultivated for feed and so is tied to ranching (Donald Citation2004).
Secondly, there is a trend of increasing land speculation tending to land concentration despite which medium and large ranchers do not compete but constitute production chains to supply a growing demand; this mutual reliance makes conversion of forestland into pastures profitable even in remote areas (Mertens et al. Citation2002). Land concentration attracts in-migrants seeking employment whose land has been purchased (sometimes forcibly) and pushes out-migrants farther away in the midst of often violent conflict (Walker et al. Citation2000; Lambin et al. Citation2001).
Finally, as regards overseas markets, Europe has become a large market for bovine spongiform encephalopathy-free meat, and China has become an increasingly affluent market for imported meat (Nepstad, Stickler, and Almeida Citation2005).
1.3. Drivers and recent forecasts
Recent deforestation forecasts show considerable progress but still face a series of challenges. These include accounting for the regionally variable role of road access, the role of irreversibility (i.e., longer secondary growth inhibition in cattle ranches than in shifting cultivation), and trying to grasp the linkages between perceptible land suitability variables (e.g., topography, distance to roads and rivers) and emergent patterns of deforestation (e.g., edge effects, fragmentation, and spillovers).
1.3.1. The role of roads and topography
Forecasts have hitherto relied on roads to map future deforestation in Amazonia (Laurance et al. Citation2001; Soares-Filho et al. Citation2006) because roads have adequately represented deforestation triggers. Indeed, roads or trails seem to be a necessary condition to access forestland and cattle markets. This is why in spite of lower federal investments in roads, deforestation rates have accelerated, highlighting the importance of non-federal or unpaved roads (Câmara et al. Citation2005), which are more difficult to predict. The impact of other roads is also variable depending on distance to markets, the presence of State-promoted settlements (Câmara et al. Citation2005; Laurance, Fearnside, Albernaz, Vasconcelos, and Ferreira Citation2005), or road type (Laurance et al. Citation2002; Nelson, De Pinto, Harris, and Stone Citation2004).
After roads give access to new land, settlers must make land use decisions, which are visible in deforestation patterns. In relation to terrain features, deforestation seems to follow a sequence of decreasing preference in the neotropics: (1) lowland is initially converted, then (2) gentle slopes, (3) steep slopes (Cavelier, Aide, Santos, Eusse, and Dupuy Citation1998; Kinnaird, Sanderson, O'Brien, Wibisono, and Woolmer Citation2003), and (4) deforestation occurs far away from cities and roads (Southworth and Tucker Citation2001; Nagendra, Southworth, and Tucker Citation2003; Lomolino and Perault Citation2004). Exceptions to this order occur where biophysical factors, such as soils and hydrology, limit agriculture (Gallegos, March, Garcia, and Acosta Citation2001), in particular in inaccessible, waterlogged or floodable lowlands (Mas and Puig Citation2001; Seidl et al. Citation2001; Mas, Puig, Palacio, and Sosa-Lopez Citation2004).
In addition to allowing transport between deforestation frontiers and agricultural markets, roads cut out 100 or 1000 km long tracts of forest, causing fragmentation (Millington, Velez-Liendo, and Bradley Citation2003), dryer edges, accumulation of flammable debris, and fast erosion (Laurance, Vasconcelos, and Lovejoy Citation2000; Gerwing Citation2002; Boltz, Holmes, and Carter Citation2003). The impacts are higher in drier areas and periods when roads are precursors of fire (Nepstad et al. Citation1999; Mertens et al. Citation2002).
1.3.2. Fragmentation and edge effects
Edges increase dryness and buildup of flammable wood and therefore the propensity to repeated fires and wildfires (Laurance et al. Citation2000, 2001; Nepstad et al. Citation2001). If a forest burns once, it loses its resistance to successive fires (Gerwing Citation2002). As to fragmentation, it decreases the predator populations of tree herbivores (Rao, Terborgh, and Nunez Citation2001) and causes crises in pollination and seed dispersal (Aguilar, Ashworth, Galetto, and Aizen Citation2006; Laurance et al. Citation2006; Terborgh and Nunez-Iturri Citation2006; Cramer, Mesquita, and Williamson Citation2007). Fragmentation affects sensitive species (Henle, Davies, Kleyer, Margules, and Settele Citation2004). Fragmentation also implies increased edge lengths. Edge effects are probably more important in secondary forests where disturbance effects are prolonged (Laurance et al. Citation2001). These effects are more acute when regrowth is recent (Clark Citation1996; Borges Citation2007).
Fragmentation and edge effects are emergent properties and not intended effects of land use. The foregoing sections point to anthropogenic explanations of these properties, but in the following, it will be important to elucidate the role of SA in regressions and cellular automata to ascertain that these do not produce edge or fragmentation artifacts.
1.3.3. SA as a landscape and process feature
SA is a measure describing the similarity of neighboring locations. If ranches are grouped together on the land, SA will be positive. SA stems from local interactions that produce a pattern departing from randomness. The importance of SA and its effects on other landscape indexes are well acknowledged (Legendre et al. Citation2002). Similarly, in explanatory models, SA is an essential variable (Anselin Citation1988; Fotheringham, Brunsdon, and Charlton Citation2002). In forecasts, SA is embedded in cellular automata models, because the cellular automata are local neighborhoods that help render the spatial character of a landscape (Eastman Citation2006; Soares-Filho et al. Citation2006).
1.3.4. Identifying drivers: spatial regression
In regression models intended to identify drivers of forest loss, SA is a measure of the similarity, or correlation, of things that are close by. This correlation contradicts the assumption of independent cases in regression analysis and may thus lead to biased estimates (Odland Citation1988; Wrigley, Holt, Steel, and Tranmer Citation1996; Anselin Citation2002; Legendre et al. Citation2002). In previous studies, SA has been dealt with using samples to separate out potentially correlated neighboring pixels (Laurance et al. Citation2002; Mertens et al. Citation2002; Stolle, Chomitz, Lambin, and Tomich Citation2003; Verburg, Overmars, and Witte Citation2004). Some have refined the sampling to exclude points in an autocorrelation zone, or via stratification in more homogeneous areas. A handful of studies have used neighborhood covariates (Geoghegan et al. Citation2001), spatially autoregressive models (Bucini and Lambin Citation2002), moving windows, or kernels (Schneider and Pontius Citation2001; Munroe, Southworth, and Tucker Citation2004).
In a regression, SA needs to be controlled for. One method is to use neighborhood covariates, also called lags, in spatial autoregressive models (Anselin Citation1988; Lesch, Strauss, and Rhoades Citation1995). However, neighborhoods might alter the significance tests of deforestation predictors as many neighboring cells will be counted multiple times. Consequently, the test statistics may be correlated, and a multiple comparison problem incurred whereby significance is too easily found (type I error). One solution is a Bonferroni correction but this seems too conservative (Perneger Citation1998; Fotheringham et al. Citation2002; Moran Citation2003; Anselin Citation2007) and it becomes difficult to show significance (type II error). Sampling may limit multiple comparison issues but is best avoided when the probability of deforestation is relatively low. One option is to use a case–controls (CC) approach, as CC logistic regressions have been used successfully (Scott and Wild Citation1986; 1991; Breslow Citation1996). CC are similar to stratified sampling, in that a probability of 1 can be used to draw cases and a smaller probability for controls. In CC as against sampling, the lack of procedures to handle complex samples in regressions is not an issue, as logistic regressions on CC can ignore the sampling scheme (Scott and Wild Citation1986). Some authors suggest an equal number of cases and controls to gain efficiency (Breslow and Chatterjee Citation1999; Wakefield Citation2004). Robustness can be gained by weighting cases using the inverse of the sampling probability (Scott and Wild Citation1991; Breslow Citation1996).
1.3.5. Forecasting: cellular automata
Recent deforestation forecasts have relied on cellular automata (Soares-Filho et al. Citation2004; Verburg, Schot, Dijst, and Veldkamp Citation2004; Pontius and Malanson Citation2005; Eastman Citation2006). A cellular automaton (CA) is a neighborhood with cell values that account for proximity to the central cell in the neighborhood. In other words, the CA is a filter downweighting the influence of faraway cells (Eastman Citation2006), rendering a spatial character similar to the one produced by positive SA. Contiguous cells interact, so despite the uncoordinated cellular behavior, landscape patterns emerge (Wu and Webster Citation2000). Therefore, CAs are a mix of landscape and neighborhood processes; they also bring together the heterogeneous, but often static, accessibility and attractiveness characteristics of land, and the dynamic, self-organizing local interactions (Wu Citation1998).
Some concepts and approaches have become standard in cellular automata, such as splitting the spatial prediction problem into quantity and location of change, using transition probabilities and suitability maps, respectively (Verburg and Veldkamp Citation2004; Eastman Citation2006). In addition, SA is usually accounted for (Eastman Citation2006; Soares-Filho et al. Citation2006). Calibration and validation procedures have been clarified and accuracy statistics are standardized (Lesschen, Verburg, and Staal Citation2005).
The most popular method to ascertain the location of change has been fitting a logistic regression on the drivers of deforestation (Schneider and Pontius Citation2001; Pontius and Batchu Citation2003; Aspinall Citation2004; Verburg, de Nijs, van Eck, Visser, and de Jong Citation2004). The models have also tried to be dynamic, either by iteratively updating suitability maps (Wu Citation2002; Verburg and Veldkamp Citation2004; Eastman Citation2006) or by predicting the location of the drivers and their associated pattern of deforestation (Laurance et al. Citation2001; Soares-Filho et al. Citation2006). The strength of impact varies between drivers and consequently, they have been weighted with a variety of methods such as hierarchical multicriteria evaluations, the ideal point method, participatory techniques, and Bayesian a priori weights (Wu Citation1998; Eastman Citation2006; Soares-Filho et al. Citation2006). As to spatially allocating the quantity of change, it is perhaps the least standardized step, and so only the Multi-Objective Land Allocation (MOLA) algorithm will be described, as it is used in the Markov Chain cellular automaton (MCA; see Section 3). The MOLA algorithm is relevant to the aim of this study relating to using deforestation drivers in forecasts.
1.3.6. Spillovers
In the context of deforestation, spillovers can be defined as forest loss outside the study area as a result of continuing demand for timber or land. There is substantial economic literature on spillovers as beneficial mutual effects between economic sectors, but the notion that these interactions are also spatial is less widespread (for instance, Anselin, Varga, and Acs Citation1997; Murdoch and Sandler Citation2002). In deforestation studies, perhaps the only example is related to the impact of road investments on adjacent municipalities (Pfaff et al. Citation2007).
When referred to ranching, spillovers can be interpreted as attempts to innovate or escape competition from neighbors. Spillovers take place farther in space and later in time than edge effects and fragmentation, and would instead cause new tracts of forest to be felled. Spillovers are thus important features in a forecast, and point to the fallacy of a closed system, often incurred when using a satellite image tile as study area. The cellular automata used in this study mapped spillovers, albeit with some limitations such as spillovers occurring only after forest exhaustion in the study area and random location of spillovers.
1.4. Study area
The Alta Floresta area (56°W, 10°S) is located in the central part of the Brazilian arc of deforestation (), 100 km from the nearest highway connecting the ranching core in southern Brazil and the Amazon basin. Access to external beef markets is dependent on the unpaved BR-163 highway, with a hub in the Colíder ranching hotspot (). Paving BR-163 poses an imminent threat (Soares-Filho et al. Citation2006; Fearnside Citation2007). Alta Floresta is located in the state of Mato Grosso, where the highest deforestation rates in Amazonia have been observed, between Cuiabá (capital of Mato Grosso) to the South and Santarém on the Amazon River to the North. Alta Floresta is therefore a stepping stone in the northward expansion of cattle, and a particularly clear example of cattle encroachment, with low human population density, separated from a highway but connected with distant markets.
The study area comprises land on both banks of the Teles Pires River (or Rio São Manuel), covering partially or totally six municipalities, and centered on the Alta Floresta municipio, which had less than 2000 inhabitants in 1970. Colonization started in the early 1980s due to the Amazonian gold rush (Alta Floresta becoming a major gold commercialization center) and some state incentives (Margulis Citation2004; Otchere, Veiga, Hinton, Farias, and Hamaguchi Citation2004). Its population grew 20-fold and then as gold became scarce (Veiga and Hinton Citation2002; Lacerda, de Souza, and Ribeiro Citation2004) its population growth decreased, just as in most of northern Mato Grosso (Pinto da Cunha Citation2006). As of 2002, most deforestation was driven by cattle ranching, with practically no agricultural crops beyond Sinop, more than 200 km south of Alta Floresta (Margulis Citation2004).
No indigenous protected areas exist in the area. In the north of the study area, therefore not impinging on this study, a Mato Grosso state conservation unit was created in 2000 and extended in 2001 (FEMA-MT Citation2002). In 2006 members of the state parliament intended to reduce the size of this park but were impeded by a court ruling (ICV 2007). In 2005–2006, this conservation unit was the most deforested one in the state (Souza, Veríssimo, Micol, and Guimarães 2006).
2. Data
The land cover data were four Landsat composites (tile 227-067 for 2000, 2002, 2004, and 2006). Bands 7, 4, and 2 were chosen as such composites are sensitive to moisture, vegetation, water, and rock characteristics and discriminate well vegetation formations (Campbell Citation2002; Tucker, Grant, and Dykstra, Citation2004; Josse et al. Citation2007). The images were taken between May and July, coinciding with the second half of the dry season, so cloud cover was negligible, and no seasonal differences affected their comparison.
Road and river data were from the Brazilian Geographical and Statistical Institute obtained via the Sistema de Proteção da Amazônia (SIPAM) system of the Brazilian government. Three categories of roads (State, vicinal, and unnamed) and rivers (Teles Pires River, other named rivers, and unnamed rivers) were considered as they might be linked in various degrees to deforestation.
Altitude data were from the Space Shuttle altimetry at 90 m resolution. The accuracy of elevation was 16 m or better for absolute height and 10 m or better for relative height (Rodriguez et al. Citation2005; Farr et al. Citation2007). Tree height showed a different altitude from adjacent pasture; this was relevant to the correct location of river beds and was solved during the preprocessing.
2.1. Preprocessing
An unsupervised classification of the Landsat composites was performed with Leica Image Analysis for ArcGIS 9.1 and the Isodata algorithm (Leica Geosystems AG, Glattbrugg, Zurich, Switzerland). Nine land classes were obtained which showed differences in moisture or successional age; they were then reduced to forest, non-forest and water using ancillary data (Neave 2007). Some misclassifications were noted between moist vegetation, water, and shadows linked to near-infrared and mid-infrared wavelengths not totally absorbed by water. This was likely due to water turbidity already noted in the Alta Floresta area in the 1990s (Musa Filho Citation1993, cited in MMA Citation2006). A possible source of difference in classification might be the sun–land–satellite geometry differences between years. To help correct these problems, the sequence of land cover from 2000 through 2006 was examined, and improbable trajectories were corrected, as they involved too little time for some changes to take place, such as water temporarily replaced by forest or reforestation within 2 or 4 years ().
Figure 2. Lexis diagram of sojourn time in a land cover class. Sojourn time is the time that a cell remains in a land class. In this example, a forest pixel appears in 2004 sojourning in this state between 2 and 4 years. This short time for forest secondary growth was corrected and the pixel was considered as non-forest throughout the observations. During the classification process several probable misclassifications were noted, especially in 2000 and 2006. These appeared to be due to water not absorbing totally the near-infrared and mid-infrared wavelengths. This led to confusion locally between moist vegetation and water on the one hand, and shadows and water on the other hand. To solve this problem, the sequence of land cover over time was examined to alter cells leading to improbable changes. Improbable trajectories involved too little time for some changes to take place, such as water temporarily replaced by forest or reforestation within 2 or 4 years, especially as Alta Floresta is known for its managed pastures (Margulis Citation2004). By analogy with smoothing spatial filters, this correction can be called a temporal filter. Systematic identification of improbable trajectories was done with the Lexis diagram of calendar year versus sojourn time in a land cover class. For a use of sojourn times, see Soares-Filho et al. (Citation2004); for a use of a correction in high temporal resolution images, see de Barros Ferraz et al. (Citation2005).
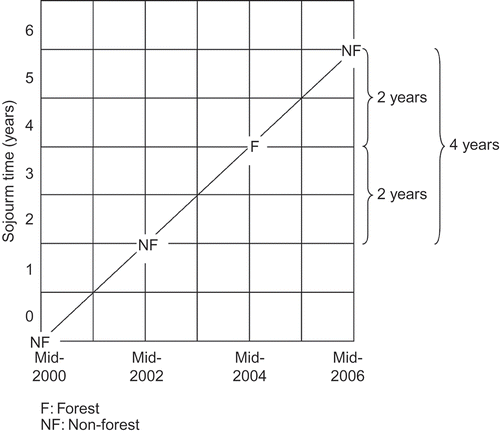
The forest cover maps were validated using 149 GPS ground truthing points located in small remnant patches of forest or near the edges, that is, forest more sensitive to change. Relative errors of 6–20% depending on the year were compatible with the 15% usually expected in classifications (Eastman Citation2006). The higher error in 2006 was probably due to deforestation as the ground points were in the locations most likely to be deforested by then.
Changes in cell land cover over time were then measured by image difference, or post-classification comparison, a method repeatedly found suitable (Mas Citation1999; Coppin, Jonckheere, Nackaerts, Muys, and Lambin Citation2004; Lu, Mausel, Batistella, and Moran Citation2004, 2005). Deforestation was defined for each pixel as changing from forest to non-forest. Uncertainty in the land cover classification was reduced by applying a temporal filter, in pixels where confusion was most likely, due in particular to mixtures of forest and water inside the pixel.
Geometric rectification was done using a 2001 Landsat orthorectified image (Tucker et al. Citation2004; GLCF 2006). Rectification is a time-consuming process, so rectification with ESRI (Redlands, CA, USA) ArcMap 9.1 of the classified images used a linear transformation based on nine link points or three times the minimum number of points needed (n = (p + 1)(p + 2)/2 = 3, with p = polynomial order = 1) for a flat area. Root mean squared errors were 189.2–110.1 m, values larger than the Landsat resolution of 30 m. Therefore to diminish the root mean squared error:resolution ratio, the resolution was aggregated to 1000 m, producing a relative error of 18.9% or lesser, much smaller than the size of an aggregated grid cell. The aggregated data were a fair match of the original land class distribution in all years. The deforestation trend was conserved, and non-forest was still the modal class in the end. All the explanatory variables were aggregated to this new resolution. As a result, space resolution was the same as Soares-Filho et al. (Citation2006) and probably better than Laurance et al. (Citation2001). Hence, the results from this study might be scaled up to larger areas such as Amazonia. The choice of bands 7, 4, and 2 is also appropriate for very large areas and continents (Josse et al. Citation2007).
The images were then referenced to the South American datum UTM Zone 21 South. The rectified Landsat images and Space Shuttle digital elevation model showed precise coincidence but the vector roads had to be edited in ArcMap to match the linear features with high values of infrared on the Landsat images. For rivers, a first editing pass used the digital elevation model to ensure that the rivers occupied the lowest portions of terrain. Occasionally, the apparent altitude difference between forest and pasture provoked rivers to be erroneously located on pastures. So a second editing pass moved erroneously located river segments to their location on the Landsat composite.
3. Methods
Processing the forecast model involved three steps: calibration, validation, and extrapolation (). Calibration identified the significant drivers and their weights and transformed weights into deforestation probability maps. Validation then compared a forecasted and an observed landscape to further refine the forecasting parameters. Finally, the extrapolation carried the procedure forward in time. Following this process, the forecast landscape was assessed in terms of edge deforestation, fragmentation, and SA. The models were discussed socioeconomically and ecologically.
Figure 3. Application of the Idrisi CA_Markov methodology to forest-cover forecasts in Alta Floresta, Amozonia.
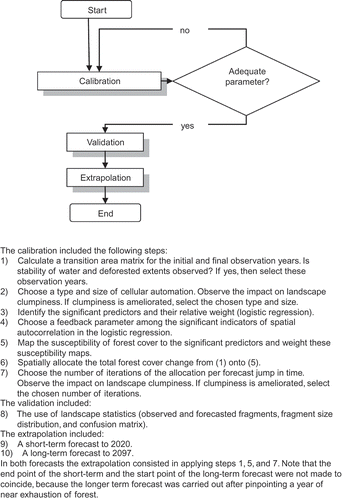
3.1. Identifying and weighting deforestation drivers
The significant drivers of deforestation were identified based on logistic regressions. The potential deforestation drivers were biophysical (elevation, slope, distance to forest edge, remaining forest), landscape-related (median forest cover in an 8-cell neighborhood around each cell and the percentage of forest cells per km2), and accessibility (in terms of distance to different categories of roads and rivers and to central urban points).
The regressions were fitted using SPSS v. 15 (IBM Corporations, Somers, NY, USA). Two goodness-of-fit measures were used: firstly, the Hosmer-Lemeshow C test of lack of fitness where large chi-square values denote lack of fit (Hosmer, Hosmer, leCessie, and Lemeshow Citation1997). The second measure is the receiver operating characteristic (ROC) where differences in the true positive rate are shown according to cut-off points of false positives (Brooker, Hay, and Bundy Citation2002; Pontius and Batchu Citation2003). The ROC and Hosmer-Lemeshow approaches use intuitive groupings with meaningful interpretation, but the ROC does not have to choose cut-off points, as it displays all the values of the modeled probabilities (Liu and Wu Citation2003).
Logistic regression adequacy and assumptions are fulfilled when variation of the residuals is small (i.e., the means of covariates are linked with the predicted variable via a logit transformation), without tendency (all variables, transformations, and interactions are tested), and variance of the predictors is as postulated; Bernoulli in this case (Hosmer et al. Citation1997). Linearity of the relationship with the odds ratio (OR) was checked entering squared variables (Harrell, Lee, and Mark Citation1996). For influential cases, Cook's statistic was used (O'Hara Hines and Hines Citation1995).
The logistic regressions were fitted using three different variants: population of grid cells, CC, and weighted CC (weighting each control cell by the probability of selection). The results were then compared to assess the robustness of the estimates.
3.2. Forecast
In this study we use a MCA implemented in the Idrisi Andes GIS software (Clark Labs, Worcester, MA, Eastman Citation2006). The MCA approach was chosen for two reasons: it is practically an off-the-shelf solution within a well-known GIS software. In addition, profuse literature is available on Markov chains and cellular automata from many disciplines. MCA comprises three steps which were adapted to this study: firstly, a calculation of the amounts of cells changing from forest to non-forest per period. Initially, a Markov chain assumption was that only the immediate previous state compounded all past history. This linear change was used until the date of forest exhaustion was pinpointed. Then a sigmoid curve was fitted between observed remaining forest and total forest exhaustion introducing a slightly more realistic kink around 2075 (Figure 5). Secondly, the probabilities of change, estimated with the spatially explicit regressions for each deforestation predictor, were mapped. Finally, forest and non-forest cells were allocated to the cells with highest probability. MCA uses MOLA. Multi-objective means that there are several predictors of change. MOLA gives each cell the land class in which it has the highest rank. When the ideal situation of a high rank in one class and low ranks in the other classes is not met in a cell, the land class in the neighborhood is taken into account, as proximity to non-forest cells makes neighboring cells more prone to deforestation. Allocation is carried out until the forest and non-forest amounts of cells for the period are achieved. Usually, if the land class changes were calculated for a period of n years, the forecast is performed for the following n years. In this study, n = 4 years. The number of iterations per allocation run, with possible values between 1 and 4, helps calibrate the model. In this application, water was constrained, so that change would only occur between forest and non-forest.
3.2.1. Extrapolation parameters and their landscape interpretation
The extrapolation parameters and their landscape interpretation were as per . The most important one was the transition matrix, as it controlled the number of cells changing from one land class to another. The matrix also controlled the (ir)reversibility of deforestation. In the logistic regression we only modeled deforestation, not secondary growth. Hence, irreversibility was a useful simplification which mimicked the cycle of forest regrowth checked by pasture management. It was also noticed that irreversibility improved the landscape patchiness otherwise blurred by positive SA. Even though an individual pasture is not irreversibly deforested, at the scale of a ranch, a ranching frontier, and even more so a consolidated agricultural region, forest conversion is, for practical forecasting purposes, irreversible. This is especially true if the purpose is to try and foresee the ultimate, long-term exhaustion of forest. On the contrary, fluctuating states between deforestation and secondary growth are much more difficult to predict, since both deforestation and secondary growth need to be forecasted several times. The pace of deforestation was held constant, consistent with previous findings (Michalski, Peres, and Lake 2008).
Table 1. Forecast parameters and their interpretation.
The cellular automata controlled the intensity of SA (the most important driver in the logistic regression, also interpretable as proximity interactions, and deforestation along edges). The effect of the cellular automata was to increase the clumpiness, that is, reduce the patchiness of the landscape. Several sizes and shapes of the cellular automata were tried but this did not seem to substantially modify the forecast outcome.
Despite their importance, feedback effects such as soil depletion or pasture age are difficult to enter in a forecast for lack of information. However, the importance of SA also provided an opportunity to include a feedback effect in the forecast. To do so, the SA covariate map was updated after every 4-year forecast. It was expected that this would modify the edge effect. However, the feedback effect was overridden by the number of cells to be deforested given by the transition matrix.
4. Results
4.1. Observed forest cover change
Over the observation period the trend was a growth of non-forest at a constantly diminishing pace: 4.6 percentage points between 2000 and 2002, 3.1 percentage points in 2002–2004, and 2.0 percentage points in 2004–2006. Forest diminished from 53.5 to 44.2% in 2000–2006, while non-forest augmented from 42.6 to 52.3%. Water represented an average of 3.2% of the study area.
The results of the 1 km resolution logistic regressions explaining observed forest cover change are shown in . The best fit was obtained for 2000–2004. The models showed adequate goodness-of-fit and behavior of residuals. In the three models, protective factors (exp(b) = OR of deforestation < 1.000, where OR = P(deforestation)/P(remains forested)) were consistently the same.
Table 2. Logistic regressions of deforestation according to sampling schemes, significant predictors, Alta Floresta 2000–2004.
4.1.1. The role of topography
Higher altitudes were a protective factor at the north and south borders of the study area. Higher and flatter places seemed to have already been subjected to deforestation in the past, and so less deforestation took place there. This was confirmed by a chi-square test showing that the higher the amount of deforestation prior to 2004, the stronger the altitude–slope interaction (χ2 = 109.5, p < 0.001). As altitude increased, the interaction became stronger as shown by several tests with different altitude. The rest of the significant variables had a lesser effect on deforestation.
Central municipal points in the study area were not significant, suggesting that land around them had already been deforested in the period under study. Similarly, roads and rivers did not seem to have large effects in 2000–2004, except distance to Mato Grosso state roads, which increased slightly the risk of deforestation. This means that in 2000–2004, deforestation happened away from the main roads, where land was still available. So contrary to other deforestation forecasts, local roads were not shown to have an important role in current deforestation in Alta Floresta.
4.1.2. The role of edge effects and fragmentation
The three most significant predictors were the SA covariate, altitude, and the interaction between altitude and land flatness in decreasing order of importance. The SA covariate, with values 0 = non-forest and 1 = forest, meant that when the majority of cells surrounding each 1 km area were forest, the probability of deforestation largely decreased. In other words, deforestation took place in locations adjacent to already deforested places, that is, along forest edges with neighboring non-forest pixels or in small forest fragments surrounded by non-forest.
Conversely, fragmentation meant that a continuous tract of forest was replaced by smaller separated chunks, increasing patchiness. This related to local negative SA and two possible situations: non-forest cells appeared within forest neighborhoods or forest remnants survived although surrounded by non-forest. Positive SA (which entailed clumpiness) was significant in the regressions and therefore was induced in the forecasted maps. By comparing the forecasted and observed 2006 landscapes, clumpiness and fragmentation could be assessed. The forecast underestimated both the number and size of fragments (), and thus highlighted the role of negative SA in the patchiness of the landscape.
Figure 4. Fragments in observed and predicted maps, 2006. A 2006 cellular automata forecast was run and compared with the observed 2006 forest cover. The accuracy of the 2006 forecast was 85.8%; 14.2% were confusions between forest and non-forest. There are more non-forest and especially more forest fragments in the observed map. The fragments are therefore smaller than in the predicted map. Most of the small patch sizes are underestimated in the forecast, especially the 1 and 2 km2 patches. The number of fragments per size class decreases rapidly. The distribution then becomes uniform after 8 km2; at this point, the forecast more closely matches the observed distribution of fragment size, despite small numbers of fragments per size class, and relatively small differences in fragment size between classes. Per land class, the discrepancy in number of fragments affects principally forest patches (245 observed and 112 forecasted patches) as against non-forest (89 observed, 68 forecasted). Although dramatic in appearance, the forecast accuracy figure suggests that fragmentation is an important but second-order feature of pasture expansion. Fragments might in fact have a role in cattle management in preserving shade and moisture.
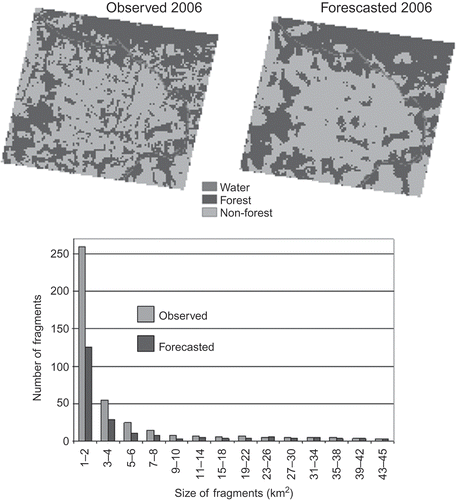
Deforestation along pasture–forest edges and topographic features explained observed landscape change. They were also likely to matter in the near future. A typical forecast quandary was, however, to decide how long the ongoing process would last. An obvious approach was to prolong the trend and characterize the patterns that emerged from it. To do so, the regression estimates (OR) for the three most important variables were transformed into deforestation probability (P = OR/(1 + OR)) at each cell. The probability was then rescaled to the 0–255 range, with a value of 255 for the predictor with higher weight. Less important predictors were constrained to a proportionally narrower range. This value at each cell produced deforestation susceptibility maps for the cellular automata forecast.
4.2. Future forest landscape
Based on the 2000–2004 quantity of deforestation and strength of local interactions among neighboring locations, a forecast to 2006 was carried out and validated. To look at the patterns beyond 2006 an extrapolation was conducted from the 2004 baseline in successive 4-year steps and then in longer steps till near exhaustion. To this effect, change was considered linear (same amount of deforestation between observations), consistent with most of the hitherto observed history of deforestation, and then sigmoid like many depletion processes. Near exhaustion was justified to highlight the last phase of deforestation and was identified to occur around 2097. Three dates were chosen to illustrate long-term land cover change between 2020 and 2097.
4.2.1. Spatial autocorrelation
The patterns that emerged from the forecast were primarily driven by SA or local interactions, as per the multivariate and cellular automata approaches, both of which incorporate pixel neighborhoods. The interpretation of local interactions is that edge effects and edge deforestation dominate landscape transformation in Alta Floresta. But validation also showed the importance of fragmentation in rendering patchiness. In addition, in the calibration step, spillovers outside the study area, suggesting distant interaction, could be spotted as a relevant feature.
The cellular automata forecast entailed an S-shaped SA behavior with a steep increase and then some leveling out (). The persistent high values of SA (Global Moran's I in the 0.917–0.950 range) suggested elevated clumpiness throughout the deforestation history of the study area. This narrow range of SA was maintained during nearly a century of forecasted change, with basically linear forest attrition. No estimate of SA was available prior to 2000.
Figure 5. Spatial autocorrelation (SA) during the putative deforestation history of Alta Floresta. Moran's I can be seen as the ratio of covariation between neighbors divided by the total variation. It is a global index for the whole study area which varies from –1 (dissimilar neighboring values) to 1 (spatially clustered values) and equals 0 in absence of SA (random pattern). Moran's I thus measures the strength of local interactions between neighbors. In Alta Floresta, during the observation period (2000–2004) clumpiness diminished as a product of new deforestation spots. In the forecast period, 2006–2097, clumpiness augmented as cellular automata emphasized the importance of local interactions. The response of Moran's I is nonlinear to a deforestation process that was piecewise linear.
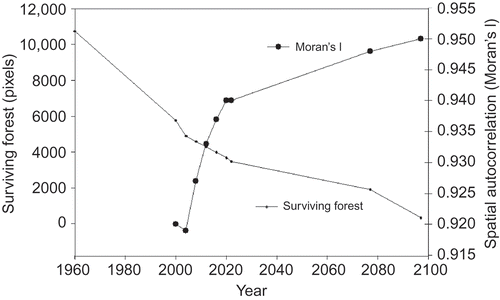
4.2.2. Edge effects versus fragmentation
During 2000–2004 predominantly forested areas were associated with lower deforestation probabilities. Visually, deforestation seemed to take place near already deforested patches (). However, observed 2006 fragmentation suggested less edge deforestation and more fragments than predicted (). In 2008–2020, edge effects slimmed down larger forest patches, made small patches disappear in the center of the image, filled gaps between deforested patches and smoothed out edges. No new patches of deforestation appeared. Interestingly, the distance to rivers was non-significant in the regression, but riparian corridors were well captured by the cellular automata on the banks of the Teles Pires and other main rivers. To humans and their cattle this may mean that the floodplains are unattractive land, valuable for shade and water, or that the riverbanks are too steep. In addition, the riparian corridors were continuous tracts, and thus probably had higher SA, which made them less likely to be deforested than isolated forest patches. By 2020 patchiness was much diminished and most deforested patches were connected.
Figure 6. Observed and forecasted forest cover and edge pattern of deforestation, 2000–2097, Alta Floresta, Mato Grosso. Similar edge effects are observed and forecasted. In the 2000–2004 period, deforestation occurred on the edges and filled small gaps (south of the Teles Pires) and large gaps (north of the river). As a result, clumpiness increased largely, and the non-forest was almost completely connected. Islands of deforestation with no connection to even small previous deforestation patches were very few. Therefore, when the forecasting starts, gaps are not available to be filled, and only edge effects take place. The number of forest fragments decreases, until it rises again, as fragments become small and sparse.
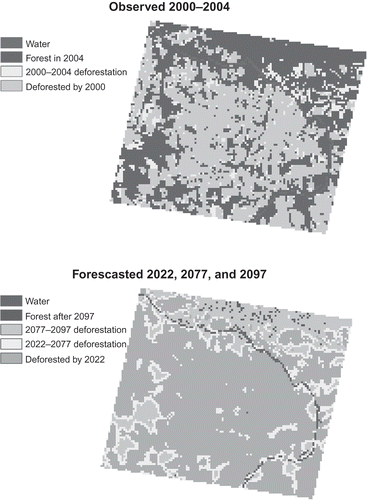
In 2022, remaining forest lay in two large riparian corridors (in the southwest or adjoining the Teles Pires), as well as a large swathe on the north bank of the Teles Pires. A few isolated forest patches persisted in the center and south of the image. Non-forest was by 2022 a very large continuous area in the south and several mid-sized areas in the northeast. Small isolated deforestation islands were found to the north of the Teles Pires River. During 2022–2077, the amount of loss (1570 km2) appeared in the form of edge effects and gap filling. However in the following period, 2077–2097, the same absolute loss, leading to near exhaustion, was due to the total suppression of large forest patches. From thin but widespread, deforestation in its terminal phase engulfed very large forest swathes. This last phase includes an extremely acute fragmentation of the northern bank of the Teles Pires River.
Throughout the putative 140 years of deforestation history in Alta Floresta, the long-term forecasts did not show troubling artifacts stemming from the method. The main limitation of the forecasts was the difficulty in locating small, isolated forest fragments in the midst of existing deforested areas.
4.2.3. Spillovers
Another predictable deforestation pattern are spillovers happening outside the study area () as a result of ongoing cattle expansion and increasingly limited local resources. Spillovers were randomly located in the study area in this exercise and occurred only after forest exhaustion, around the year 2120. For spillovers to occur, local attractiveness and accessibility need to be overall less favorable than in the new locations. Although spillovers can be foreseen, they are difficult to quantify and locate. They also call for a regional more than a local focus.
Figure 7. Spillovers outside the study area, 2120. The study area is the inclined, solid gray rectangle. It was forecasted as totally deforested by 2120. Spillovers, the gray scattered pixels on the background, were deforestation overflowing from the study area as a result of momentum gathered by ranching, and areas susceptible to deforestation are already deforested. That is, because of continuing deforestation, the forecast had to allocate more non-forest pixels than available inside the study area. Spillovers are shown with a random pattern. However, the northward expansion of humans into Amazonia and the non-random deforestation in Alta Floresta both suggest that randomness is inadequate in forecasting spillovers. To overcome this difficulty, the same prediction and forecast as in Alta Floresta need to be carried out in a larger study area comprising both Alta Floresta and additional satellite tiles to the north. At this wider scale, predictors of deforestation may need to include ecotone areas which have both moist and dry characteristics and so favor vegetation growth while allowing for fire controlled pastures. These ecotone characteristics are precisely one of the attractive features of the arc of deforestation for agriculture. It is important to note that spillovers are likely to occur before forest exhaustion in Alta Floresta.
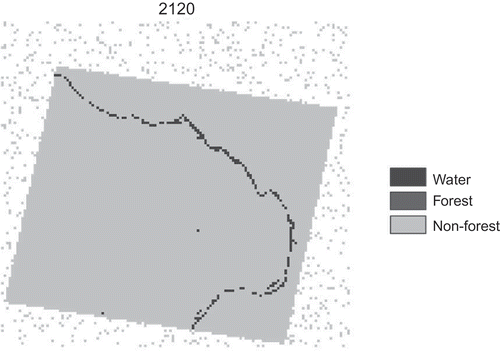
5. Discussion
5.1. The changing roles of roads and topography
In recent Amazonia-wide spatially explicit forecasts (Laurance et al. Citation2001; Soares-Filho et al. Citation2006), a key role is devolved to roads. Here road impacts may have depended on the stage of the deforestation process: In Alta Floresta, two decades after initiating colonization, access tracks seemed to be providing access to pastures. The models suggested that roads cannot be the only factors driving future deforestation at the landscape level. Instead, it seemed that roads trigger future deforestation in a region but eventually their local role might be superseded by other factors. In the observation period, the dirt road density may have become such that access to potential cattle locations was uniform, and other features became more relevant to land use decisions in ranches; these features may have spurred decisions to open up dirt roads.
Roads losing importance in Alta Floresta for land use decisions are consistent with a stage of deforestation occurring far away from cities and roads, elsewhere in the neotropics (Southworth and Tucker Citation2001; Nagendra et al. Citation2003; Lomolino and Perault Citation2004). Hydrological limits to agriculture or to the effectiveness of fire, in particular in inaccessible or floodable lowlands (Gallegos et al. Citation2001; Mas and Puig Citation2001; Seidl et al. Citation2001; Puig, Palacio, Sosa-Lopez 2004), also seem to be the case in Alta Floresta. These lowlands might in fact contain the last forest remnants in the future ().
5.2. Fragmentation and edge effects
Forest loss models in this study showed that edge deforestation and fragmentation were noticeable emergent properties. They resulted, respectively, from positively spatially autocorrelated neighborhoods and from negative autocorrelation and longer distances between forest remnants. Edge effects augmented clumpiness, while fragmentation would increase landscape patchiness. The strength of observed and forecasted local interactions led to important edge deforestation and played down fragmentation to a second-order effect.
The significance of edge deforestation and fragmentation can be assessed by comparing the forecast in the study area with large-scale and long-term studies. A large-scale study of fragmentation and edge effects in the arc of deforestation around the period observed here notes that deforestation occurred mostly close to already deforested areas, and 90% of forest fragments were smaller than 4 km2, although most of the forest was in large continuous patches (Broadbent et al. Citation2008). This gives credence to the forecasted edge deforestation and fragment size distribution. A long-term study stresses the fact that forest fragments are surrounded by pastures frequently set on fire by ranchers to keep secondary growth at bay (Laurance et al. Citation2007). This lends some support to the irreversible pasture simplification. In the same long-term plots, edge effects have dominated fragment dynamics (Laurance et al. Citation2010), an observation somewhat consistent with forecasts dominated by edge deforestation. More generally, deforestation for pasture leads to fragmentation and edge effects (Nepstad, Stickler, Soares-Filho, and Merry Citation2008) supporting the emergence of these properties in the models.
Conservation insights from recent literature also grant a major importance to edges and fragments. These insights include conserving forest edges and the adjacent matrix, especially from fires, erosion, and overgrazing. This allows for fragments to start recovering within one or two decades into the neighboring matrix. Reconnection and enlargement of fragments are also chief considerations (Laurance et al. Citation2007, 2010). This resilience of fragments and edges has been documented even in fragments isolated and disturbed for about a century and also in pastures which are often assumed a low resilience. Moreover, fragments contain propagules to repopulate the neighboring matrix and cattle can be used to browse forest edge grass to assist seedling establishment and disperse seeds (Higuchi et al. Citation2008; Chazdon et al. Citation2009). The resilience of fragments echoes the higher than expected survival of fragments in the Alta Floresta landscape.
New deforestation spots such as occur with fragmentation may respond to innovative, competition-avoidance behavior or to limitations in the supply of suitable land along edges. Alternatively, fragmentation could be due to cadastral history, that is, the location of unoccupied plots, dirt road openings, or slow degradation of forest as timber and firewood are extracted in unoccupied areas. Forecasting new deforestation spots is comparable to forecasting innovation: susceptible areas can be identified but the location, size, and onset of new pastures are difficult to predict, especially as roads do not seem to be vital, and spillovers away from the study area are likely to occur.
5.3. Spillovers or the unintended consequences of economic momentum
The forecast showed that deforestation spillovers outside the study area could derive from unabated deforestation and economic momentum as in ranching hotspots, where investments, vested interests, and well-developed meat production chains (Mertens et al. Citation2002) point to whole regional economies depending on ranches. But spillovers mean innovative attitudes too, higher transaction costs (information costs), emerging opportunities elsewhere, expected profits with shrinking local resources, incentives (such as buoying and subsidized markets, see Hecht Citation1993), or pressure (emerging markets competing for land).
Forest conversion is headed in a north or northwest direction (Dean Citation1995; Brannstrom Citation2000) into the Amazon forest, and spillovers would respond nonlinearly to road access: deforestation rises within 100 km of road investments. Possibly reduced deforestation happens 100–300 km from road investments. Beyond 300 km, again deforestation may rise (Pfaff et al. Citation2007).
Land competition with soya bean and sugarcane will also imprint this direction. The soya bean centroid headed in 2000–2006 toward the pasture centroid and the latter toward the deforestation centroid pushing pastures farther north in Mato Grosso. Indeed, the areas of largest changes in total and soya bean harvested areas were reaching Alta Floresta. Elsewhere most deforestation remains driven by pasture expansion (Barona, Ramankutty, Hyman, and Coomes Citation2010). Even the dense core of ranching in Central Brazil is under pressure from the sugarcane expansion and its economic benefits, resulting in a decrease of pastures and cattle heads. This expansion created in 2000–2006 new sugarcane frontiers in Amazonia competing with food crops (Sparovek, Barretto, Berndes, Martins, and Maule Citation2008).
In addition to local and regional spillovers, there are global market signals with spillover effects (Andam, Ferraro, Pfaff, Sanchez-Azofeifa, and Robalino Citation2008). Brazil is now a world leader in sugar production (like meat, a by-product of the nutrition transition) and sugarcane ethanol (Fischer, Teixeira, Hizsnyik, and van Velthuizen Citation2009). The Amazon forest might be sensitive to both spillovers and land competition between agricultural products.
The onset of spillovers may occur before forest exhaustion. Mounting restrictions, enforcement, and compliance might also have unintended spillover effects which need to be understood. Protected areas have been associated with better compliance, and simultaneously, to deforestation displacements, that is, spillovers (Andam et al. Citation2008). The clamp down on deforestation in the Amazon biome may be pushing deforestation into the Cerrado biome to the south and east of Amazonia (Brannstrom et al. Citation2008; G. Câmara, personal communication, 2010). Even the upper limit to pastures in Amazonian ranches might prove to be a motive for expansion farther away from established ranches. So, the unintended effects of legal restrictions on land must be acknowledged lest they themselves contribute to the expansion of ranching in Amazonia.
5.4. Legal and husbandry limits to deforestation
As deforestation progresses in an already consolidated frontier, limits to available land begin to appear. This may mean that exhaustion may never occur in the future of Alta Floresta. Foremost among federal regulations the Forestry Code of 1965 states that the legal reserve is a manageable area that is not to be clear cut and that in the permanent preservation areas (PPA) any economic use is proscribed. Recently the percentage of the property to be devoted to legal reserve in Amazonia rose to 80% and topographic definitions for the PPA have been set (Provisional Measure 2166/67 of 1998 and Resolution 303/2002 of the National Commission on Environment). Bill 1364/2003 is also under study in the Congress and would force all landholders to delineate and restore their PPA with native species within 5 years (Ribeiro, Meitner, and da Veiga Citation2006). Resolution 369/2006 set a deadline in 2007 for PPA restoration guidelines to be proposed. Unfortunately there seems to be confusion stemming from PPA resolutions due to imprecise definitions, lack of monitoring and enforcement, and generally lack of official awareness as to the importance of riparian corridors (Cota, and Mourão Moura 2009; Pires Luiz, Gomes Leal, Silva Santos, Moreira Tosatti, and Maillard Citation2009).
The aforementioned topographic rules stress the importance of riparian corridors. As shown above, rivers visually had a noticeable protective role. This highlighted the fact that riparian forest corridors are located on lower ground, with gullies or floodable plains likely to be unattractive for cattle. In parallel, riparian forest may be conserved by ranchers to protect their water sources, and to provide cattle with shade, and thus avoid cattle weight loss in the sun. Accordingly, legal requirements and husbandry considerations might soon concur to diminish the pace of deforestation locally and raise the floor level eventually reached by forest in Alta Floresta.
6. Conclusions
This study was concerned with the implications of tropical deforestation in the context of ranching frontiers. It showed that some unexpressed land use choices made by cattle ranchers could be inferred from remotely sensed patterns of deforestation and that these choices could be used to forecast future deforestation. These patterns were locations and their associated risk factors identified using spatial logistic regressions and carried forward in time using CA allocation. A diminished role of roads was shown in the observation period. Instead, topography features (land flatness, land away from river flooding plains, and riparian corridors) were consistently significant or visible in the forecast. Some emergent properties or unanticipated effects of land use decisions could also be measured or identified using these techniques. These effects probably do not emerge from conscious choice or awareness, but rather stem from spatial interactions. Insights were gained into edge deforestation, fragmentation, and spillovers, which arise from the techniques used here but also crop up as key features of ranching expansion and deforestation ecology in the recent literature. The behavioral interpretation of the emergent properties can be that competition for land and pasture management both cancel out forest regrowth along forest edges (edge effects); competition avoidance or ‘innovative’ behavior in new and isolated spots causes fragmentation in the study area; and gathered economic momentum and willingness not to forego profits bring about spatial spillovers outside the study area.
A quantitative appraisal of the results is as follows. Classification accuracy was 80%, deforestation classification was 80% correct in the logistic regression (ROC area under the curve = 80%), and locational accuracy of the forecast was 85.5% in this study. The SA values indicating landscape clumpiness in the forecast were maintained within 0.03 points of the observed values. The number and size of fragments, though underestimated by the forecast, showed that negative SA was also an important factor. The forecast model is adapted to scenarios of quantity, but fragmentation and spillovers are more difficult to render.
Method-wise, it was shown that a fine scale forecast of deforestation (at a Landsat image scale) is feasible, and this exercise can be scaled up using a choice of Landsat bands at a 1 km resolution. Non-supervised classification reduces the amount of effort. Some corrections were necessary for possible sun–land–satellite position differences from year to year and moisture noise in the image classification. Multivariate models and cellular automata can be coupled and they show patterns from aggregated land use choices and edge/fragmentation deforestation processes. Although errors can be minimized in all steps of the procedure, there is no published benchmark for the process as a whole. The main limitation of the forecasts was the difficulty in locating remnant forest patches in the midst of existing deforested areas.
The relevance of identifying choices and their unforeseen consequences lies in the possibility of engaging different types of stakeholders in participatory efforts to reduce tropical deforestation. Toward this end, it was important to try to improve the interpretation of both the visual outputs of forecasts and the tools’ underlying concepts in economic or behavioral terms and in terms of forest landscape impacts. The parameters of the forecast were meaningful both ecologically and economically and were assumed to remain the same (stationary) over space and time in the study area. But this business-as-usual scenario could be refined with the participation of stakeholders.
6.1. Future research
Additional work is needed to understand local interactions, which explained a substantial part of the landscape changes under study. This requires estimates of local SA and locally varying drivers to better handle spatial non-stationarity. Similarly, identifying past triggers and pathways of deforestation in other ranching frontiers, hotspots, and core zones may help understand time non-stationarity and formulate relevant scenarios. Comparative research should help understand how general the drivers, emergent properties, and unintended effects are in other ranching frontiers. Expansion-wise the history of ranching hotspots giving birth to frontiers may shed light on oncoming spillovers.
Deforestation forecasts will have to render both clumpiness and fragmentation, which are two somewhat opposite metrics. As regards quantity of future deforestation, domestic and foreign demand and prices, as well as competition in beef markets with Argentina and the United States, probably will impose upper limits to Amazonian ranching. On the supply side, constant rates (as in a logistic trend) instead of constant absolute change seem to be more consistent with the gradual onset of limits on land available for deforestation. Another challenge is to detect departures from trends either as nonlinearities or as emerging threats.
As to policy recommendations, they warrant much more work and possibly a multi-agent-based approach to grasp expressed preferences as opposed to observable patterns (or revealed preferences) in this work. But the maps forecasted here along with their interpretation could be used as visualization devices toward agent-based deforestation models.
Acknowledgments
We are grateful for the comments from two anonymous reviewers.
Reference
- Aguilar , R. , Ashworth , L. , Galetto , L. and Aizen , M.A. 2006 . Plant Reproductive Susceptibility to Habitat Fragmentation: Review and Synthesis Through a Meta-Analysis . Ecology Letters , 9 : 968 – 980 .
- Andam , K.S. , Ferraro , P.J. , Pfaff , A. , Sanchez-Azofeifa , G.A. and Robalino , J.A. 2008 . Measuring the Effectiveness of Protected Area Networks in Reducing Deforestation . Proceedings of the National Academy of Sciences , 105 : 16089 – 16094 .
- Anselin , L. 1988 . Spatial Econometrics: Methods and Models , Dordrecht : Kluwer .
- Anselin , L. 2002 . Under the Hood: Issues in the Specification and Interpretation of Spatial Regression Models . Agricultural Economics , 27 : 247 – 267 .
- Anselin , L. 2007 . Foundations and Frontiers of Spatial Regression , Urbana , IL : University of Illinois at Urbana-Champaign .
- Anselin , L. , Varga , A. and Acs , Z. 1997 . Local Geographic Spillovers Between University Research and High Technology Innovations . Journal of Urban Economics , 42 : 422 – 448 .
- Aspinall , R. 2004 . Modelling Land Use Change with Generalized Linear Models – a Multi-Model Analysis of Change Between 1860 and 2000 in Gallatin Valley, Montana . Journal of Environmental Management , 72 : 91 – 103 .
- Barona , E. , Ramankutty , N. , Hyman , G. and Coomes , O.T. 2010 . The Role of Pasture and Soyabean in Deforestation of the Brazilian Amazon . Environmental Research Letters , 5 : 024002 doi: 10.1088.1748-9326/5/2/024402
- Boltz , F. , Holmes , T.P. and Carter , D.R. 2003 . Economic and Environmental Impacts of Conventional and Reduced-Impact Logging in Tropical South America: A Comparative Review . Forest Policy and Economics , 5 : 69 – 81 .
- Borges , S.H. 2007 . Bird Assemblages in Secondary Forests Developing After Slash-and-Burn Agriculture in the Brazilian Amazon . Journal of Tropical Ecology , 23 : 469 – 477 .
- Brannstrom , C. 2000 . Coffee Labor Regimes and Deforestation on a Brazilian Frontier, 1915–1965 . Economic Geography , 76 : 326 – 346 .
- Brannstrom , C. , Jepson , W. , Filippi , A.M. , Redo , D. , Xu , Z. and Ganesh , S. 2008 . Land Change in the Brazilian Savanna (Cerrado), 1986–2002: Comparative Analysis and Implications for Land-Use Policy . Land Use Policy , 25 : 579 – 595 .
- Breslow , N.E. 1996 . Statistics in Epidemiology: The Case-Control Study . Journal of the American Statistical Association , 91 : 14 – 28 .
- Breslow , N.E. and Chatterjee , N. 1999 . Design and Analysis of Two-Phase Studies with Binary Outcome Applied to Wilms Tumour Prognosis . Journal of the Royal Statistical Society Series C -Applied Statistics , 48 : 457 – 468 .
- Broadbent , E.N. , Asner , G.P. , Keller , M. , Knappa , D.E. , Oliveira , P.J.C. and Silva , J.N. 2008 . Forest Fragmentation and Edge Effects From Deforestation and Selective Logging in the Brazilian Amazon . Biological Conservation , 141 : 1745 – 1757 .
- Brooker , S. , Hay , S.I. and Bundy , D.A.P. 2002 . Tools From Ecology: Useful for Evaluating Infection Risk Models?” . Trends in Parasitology , 18 : 70 – 74 .
- Bucini , G. and Lambin , E.F. 2002 . Fire Impacts on Vegetation in Central Africa: A Remote-Sensing-Based Statistical Analysis . Applied Geography , 22 : 27 – 48 .
- Câmara , G. , Aguiar , P.D.A. , Escada , M. I. , Amaral , S. , Carneiro , T. , Vieira Monteiro , M.A. , Araújo , R. , Vieira , I. and Becker , B. 2005 . Amazonian Deforestation Models . Science , 307 : 1043c – 1044 .
- Campbell , J.B. 2002 . Introduction to Remote Sensing , 3rd , London : Taylor & Francis .
- Cardille , J.A. and Foley , J.A. 2003 . Agricultural Land-Use Change in Brazilian Amazônia Between 1980 and 1995: Evidence From Integrated Satellite and Census Data . Remote Sensing of Environment , 87 : 551 – 562 .
- Carpenter , F.L. , Mayorga , S.P. , Quintero , E.G. and Schroeder , M. 2001 . Land-Use and Erosion of a Costa Rican Ultisol Affect Soil Chemistry, Mycorrhizal Fungi and Early Regeneration . Forest Ecology and Management , 144 : 1 – 17 .
- Cavelier , J. , Aide , T. , Santos , C. , Eusse , A. and Dupuy , J. 1998 . The Savannization of Moist Forests in the Sierra Nevada De Santa Marta, Colombia . Journal of Biogeography , 25 : 901 – 912 .
- Cerri , C.E.P. , Paustian , K. , Bernoux , M. , Victoria , R.L. , Melillo , J.M. and Cerri , C.C. 2004 . Modeling Changes in Soil Organic Matter in Amazon Forest to Pasture Conversion with the Century Model . Global Change Biology , 10 : 815 – 832 .
- Chazdon , R.L. , Harvey , C.A. , Komar , O. , Griffith , D.M. , Ferguson , B.G. , Martinez-Ramos , M. , Morales , H. , Nigh , R. , Soto-Pinto , L. , van Breugel , M. and Philpott , S.M. 2009 . Beyond Reserves: A Research Agenda for Conserving Biodiversity in Human-Modified Tropical Landscapes . Biotropica , 41 : 142 – 153 .
- Clark , D.B. 1996 . Abolishing Virginity . Journal of Tropical Ecology , 12 : 735 – 739 .
- Cochrane , M.A. 2001 . Synergistic Interactions Between Habitat Fragmentation and Fire in Evergreen Tropical Forests . Conservation Biology , 15 : 1515 – 1521 .
- Cochrane , M.A. 2003 . Fire Science for Rainforests . Nature , 421 : 913 – 919 .
- Coppin , P. , Jonckheere , I. , Nackaerts , K. , Muys , B. and Lambin , E. 2004 . Digital Change Detection Methods in Ecosystem Monitoring: A Review . International Journal of Remote Sensing , 25 : 1565 – 1596 .
- Cota , M.A. and Mourão Moura , A.C. Áreas de Preservação Permanente (APP) – Estudo de Caso sobre o Parámetro Declividade e as Divergências nos Resultados de Mapeamento em Função das Bases Cartográficas e Escalas e/ou Softwares Utilizados . Anais XIV Simpósio Brasileiro de Sensoriamento Remoto . April 25–30 2009 , Seoul , South Korea. pp. 3697 – 3704 . Natal, Brasil : INPE .
- Cramer , J.M. , Mesquita , R.C.G. and Williamson , G.B. 2007 . Forest Fragmentation Differentially Affects Seed Dispersal of Large and Small-Seeded Tropical Trees . Biological Conservation , 137 : 415 – 423 .
- Dean , W. 1995 . With Broadax and Firebrand: Destruction of Brazilian Atlantic , Berkeley , CA : University of California Press .
- de Barros Ferraz , S.F. , Vettorazzi , C.A. , Theobald , D.M. and Ballester , M.V.R. 2005 . Landscape Dynamics of Amazonian Deforestation Between 1984 and 2002 in Central Rondonia, Brazil: Assessment and Future Scenarios . Forest Ecology and Management , 204 : 69 – 85 .
- DeFries , R. , Hansen , M. , Townshend , J. , Janetos , A. and Loveland , T. 2000 . A New Global 1-Km Dataset of Percentage Tree Cover Derived From Remote Sensing . Global Change Biology , 6 : 247 – 254 .
- de las Heras , A. and Lake , I.R. 2008 . “ Regions and Patterns of Forest-Change in Brazil: A Geographically Weighted Regression ” . In in Representing, Modeling, and Visualizing the Natural Environment (Innovations in GIS) , Edited by: Mount , N. , Harvey , G. , Aplin , P. and Priestnall , G. Boca Raton , FL : CRC Press .
- Donald , P.F. 2004 . Biodiversity Impacts of Some Agricultural Commodity Production Systems . Conservation Biology , 18 : 17 – 37 .
- Eastman , J.R. 2006 . Idrisi Andes. Guide to GIS and Image Processing , Worcester , MA : Clark Labs-Clark University .
- Eden , M. 1998 . “ Forest and Environmental Degradation ” . In in Tropical Rain Forest. A Wider Perspective , Edited by: Goldsmith , F.B. London : Chapman & Hall .
- Eva , H.D. , de Miranda , E.E. , Di Bella , C.M. , Gond , V. , Huber , O. , Sgrenzaroli , M. , Jones , S. , Coutinho , A. , Dorado , A. , Guimarães , M. , Elvidge , C. , Achard , F. , Belward , S.A. , Bartholomé , E. , Baraldi , A. , De Grandi , G. , Vogt , P. , Fritz , S. and Hartley , A. 2002 . A Vegetation Map of South America. EUR 20159 EN , Luxembourg : European Commission Joint Research Centre .
- FAO . 2003 . Cattle Density for Latin America , 1st ed , Rome : United Nations Food and Agriculture Organization's Animal Production and Health Division (FAO-AGA) .
- Farr , T.G. , Rosen , P.A. , Caro , E. , Crippen , R. , Duren , R. , Hensley , S. , Kobrick , M. , Paller , M. , Rodriguez , E. , Roth , L. , Seal , D. , Shaffer , S. , Shimada , J. , Umland , J. , Werner , M. , Oskin , M. , Burbank , D. and Alsdorf , D. 2007 . The Shuttle Radar Topography Mission . Reviews of Geophysics , 45 doi: 10.1029/2005RG000183
- Fearnside , P.M. 2005 . Deforestation in Brazilian Amazonia: History, Rates, and Consequences . Conservation Biology , 19 : 680 – 688 .
- Fearnside , P.M. 2007 . Brazil's Cuiaba-Santarem (BR-163) Highway: The Environmental Cost of Paving a Soyabean Corridor Through the Amazon . Environmental Management , 39 : 601 – 614 .
- FEMA-MT . 2002 . Parque Estadual Cristalino: Um Lugar Para Se Conservar , Cuiabá , Mato Grosso : Author .
- Fischer , G. , Teixeira , E. , Hizsnyik , E.T. and van Velthuizen , H. 2009 . Land Use Dynamics and Sugarcane Production , Laxenburg , Austria : International Institute for Applied Systems Analysis .
- Foley , J. , DeFries , R. , Asner , G.P. , Barford , C. , Bonan , G. , Carpenter , S.R. , Chapin , F.S. , Coe , M.T. , Daily , G.C. , Gibbs , H.K. , Helkowski , J.H. , Holloway , T. , Howard , E.A. , Kucharik , C.J. , Monfreda , C. , Patz , J.A. , Prentice , I.C. , Ramankutty , N. and Snyder , P.K. 2005 . Global Consequences of Land Use . Science , 309 : 570 – 574 .
- Fotheringham , A.S. , Brunsdon , C. and Charlton , M. 2002 . Geographically Weighted Regression: The Analysis of Spatially Varying Relationships , Chichester : John Wiley & Sons .
- Fujisaka , S. , Bell , W. , Thomas , N. , Hurtado , L. and Crawford , E. 1996 . Slash-and-Burn Agriculture, Conversion to Pasture, and Deforestation in Two Brazilian Amazon Colonies . Agriculture, Ecosystems & Environment , 59 : 115 – 130 .
- Gallegos , J. , March , I. , Garcia , G. and Acosta , O. 2001 . Uso del suelo y Transformación de Selvas en un Ejido de la Reserva de la Biosfera Calakmul, Campeche, México . Investigaciones Geograficas , 44 : 39 – 53 .
- Geoghegan , J. , Villar , S.C. , Klepeis , P. , Mendoza , P.M. , Ogneva-Himmelberger , Y. , Chowdhury , R.R. , Turner , B.L. and Vance , C. 2001 . Modeling Tropical Deforestation in the Southern Yucatan Peninsular Region: Comparing Survey and Satellite Data . Agriculture Ecosystems & Environment , 85 : 25 – 46 .
- Gerwing , J.J. 2002 . Degradation of Forests Through Logging and Fire in the Eastern Brazilian Amazon . Forest Ecology and Management , 157 : 131 – 141 .
- GLCF. (2006), GeoCover Technical Guide, Global Land Cover Facility. University of Maryland Institute for Advanced Computer Studies http://glcf.umd.edu/library/guide/techguide_geocover.pdf (http://http://glcf.umd.edu/library/guide/techguide_geocover.pdf) (Accessed: 1 September 2007 ).
- Hansen , M. , DeFries , R. , Townshend , J. , Carroll , M. , Dimiceli , C. and Sohlberg , R. 2003 . Global Percent Tree Cover at a Spatial Resolution of 500 Meters: First Results of the MODIS Vegetation Continuous Field Algorithm . Earth Interactions , 7 : 2 – 15 .
- Harrell , F.E.J. , Lee , K.L. and Mark , D.B. 1996 . Tutorial in Biostatistics Multivariable Prognostic Models: Issues in Developing Models, Evaluating Assumptions and Adequacy, and Measuring and Reducing Errors . Statistics in Medicine , 15 : 361 – 387 .
- Hecht , S.B. 1985 . Environment, Development and Politics – Capital Accumulation and the Livestock Sector in Eastern Amazonia . World Development , 13 : 663 – 684 .
- Hecht , S.B. 1993 . The Logic of Livestock and Deforestation in Amazonia . BioScience , 43 : 687 – 695 .
- Henle , K. , Davies , K.F. , Kleyer , M. , Margules , C. and Settele , J. 2004 . Predictors of Species Sensitivity to Fragmentation . Biodiversity and Conservation , 13 : 207 – 251 .
- Higuchi , P. , Oliveira-Filho , A.T. , Bebber , D.P. , Brown , N.D. , Silva , A.C. and Machado , E.L. 2008 . Spatio-Temporal Patterns of Tree Community Dynamics in a Tropical Forest Fragment in South-East Brazil . Plant Ecology , 199 : 125 – 135 .
- Hosmer , D.W. , Hosmer , T. , leCessie , S. and Lemeshow , S. 1997 . A Comparison of Goodness-of-Fit Tests for the Logistic Regression Model . Statistics in Medicine , 16 : 965 – 980 .
- ICV (2007), “Juiz Suspende Lei que Diminui Área do Parque Cristalino,” 24 horas news – 26/01/2007. Estação Vida http://www.icv.org.br/publique/ (http://http://www.icv.org.br/publique/) (Accessed: 15 September 2007 ).
- Josse , C. , Navarro , G. , Encarnación , F. , Tovar , A. , Comer , P. , Ferreira , W. , Rodríguez , F. , Saito , J. , Sanjurjo , J. , Dyson , J. , de Celis , E. , Zárate , R. , Chang , J. , Ahuite , M. , Vargas , C. , Paredes , F. , Castro , W. , Maço , J. and Reátegui , F. 2007 . Ecological Systems of the Amazon Basin of Peru and Bolivia. Classification and Mapping , Arlington , VA : NatureServe .
- Kaimowitz , D. , Mertens , B. , Wunder , S. and Pacheco , P. 2004 . Hamburger Connection Fuels Amazon Destruction. Cattle Ranching and Deforestation in Brazil's Amazon , Bogor , Indonesia : Center for International Forestry Research .
- Kinnaird , M.F. , Sanderson , E.W. , O'Brien , T.G. , Wibisono , H.T. and Woolmer , G. 2003 . Deforestation Trends in a Tropical Landscape and Implications for Endangered Large Mammals . Conservation Biology , 17 : 245 – 257 .
- Lacerda , L.D. , de Souza , M. and Ribeiro , M.G. 2004 . The Effects of Land Use Change on Mercury Distribution in Soils of Alta Floresta, Southern Amazon . Environmental Pollution , 129 : 247 – 255 .
- Lambin , E.F. and Geist , H.J. 2003 . Regional Differences in Tropical Deforestation . Environment , 45 : 22 – 36 .
- Lambin , E.F. , Turner , B.L. , Geist , H.J. , Agbola , S.B. , Angelsen , A. , Bruce , J.W. , Coomes , O.T. , Dirzo , R. , Fischer , G. , Folke , C. , George , P.S. , Homewood , K. , Imbernon , J. , Leemans , R. , Li , B. , Moran , E.F. , Mortimore , M. , Ramakrishnan , P.S. , Richards , J.F. , Skanes , H. , Steffen , W. , Stone , G.D. , Svedin , U. , Veldkamp , T.A. , Vogel , C. and Xu , J.C. 2001 . The Causes of Land-Use and Land-Cover Change: Moving Beyond the Myths . Global Environmental Change-Human and Policy Dimensions , 11 : 261 – 269 .
- Laurance , W.F. , Albernaz , A.K.M. , Schroth , G. , Fearnside , P.M. , Bergen , S. , Venticinque , E.M. and da Costa , C. 2002 . Predictors of Deforestation in the Brazilian Amazon . Journal of Biogeography , 29 : 737 – 748 .
- Laurance , W.F. , Camargo , J.L.C. , Luizão , R.C.C. , Laurance , S.G. , Pimm , S.L. , Bruna , E.M. , Stouffer , P.C. , Williamson , G.B. , Benítez-Malvido , J. , Vasconcelos , H.L. , Van Houtan , K.S. , Zartman , C.E. , Boyle , S.A. , Didham , R.K. , Andrade , A. and Lovejoy , T.E. 2010 . The Fate of Amazonian Forest Fragments: A 32-Year Investigation . Biological Conservation , doi: 10.1016/j.biocon. 010.09.021
- Laurance , W.F. , Cochrane , M.A. , Bergen , S. , Fearnside , P.M. , Delamonica , P. , Barber , C. , D'Angelo , S. and Fernandes , T. 2001 . Environment – the Future of the Brazilian Amazon . Science , 291 : 438 – 439 .
- Laurance , W.F. , Fearnside , P.M. , Albernaz , A.K.M. , Vasconcelos , H.L. and Ferreira , L.V. 2005 . Response . Science , 307 : 1044
- Laurance , W.F. , Nascimento , H.E.M. , Laurance , S.G. , Andrade , A. , Ewers , R.M. , Harás , K.E. , Luizão , R.C.C. and Ribeiro , J.E. 2007 . Habitat Fragmentation, Variable Edge Effects, and the Landscape-Divergence Hypothesis . PLoS ONE , 2 : e1017
- Laurance , W.F. , Nascimento , H.E.M. , Laurance , S.G. , Andrade , A. , Ribeiro , J.E.L.S. , Giraldo , J.P. , Lovejoy , T.E. , Condit , R. , Chave , J. , Harmos , K.E. and D'Angelo , S. 2006 . Rapid Decay of Tree-Community Composition in Amazonian Forest Fragments . Proceedings of the National Academy of Sciences , 103 : 19010 – 19014 .
- Laurance , W.F. , Vasconcelos , H.L. and Lovejoy , T.E. 2000 . Forest Loss and Fragmentation in the Amazon: Implications for Wildlife Conservation . Oryx , 34 : 39 – 45 .
- Legendre , P. , Dale , M.R.T. , Fortin , M.J. , Gurevitch , J. , Hohn , M. and Myers , D. 2002 . The Consequences of Spatial Structure for the Design and Analysis of Ecological Field Surveys . Ecography , 25 : 601 – 615 .
- Lesch , S.M. , Strauss , D.J. and Rhoades , J.D. 1995 . Spatial Prediction of Soil Salinity Using Electromagnetic Induction Techniques 1. Statistical Prediction Models: A Comparison of Multiple Linear Regression and Cokriging . Water Resources Research , 31 : 373 – 386 .
- Lesschen , J.P. , Verburg , P.H. and Staal , S.J. 2005 . Statistical Methods for Analysing the Spatial Dimension of Changes in Land Use and Farming Systems , Nairobi : The International Livestock Research Institute . Wageningen: LUCC Focus 3 Office
- Liu, H., and Wu, T. (2003), “Estimating the Area Under a Receiver Operating Characteristic (ROC) Curve for Repeated Measures Design,” Journal of Statistical Software 8. http://www.jstatsoft.org/i08/il2/ (http://www.jstatsoft.org/i08/il2/) (Accessed: 20 September 2007 ).
- Lomolino , M.V. and Perault , D.R. 2004 . Geographic Gradients of Deforestation and Mammalian Communities in a Fragmented, Temperate Rain Forest Landscape . Global Ecology and Biogeography , 13 : 55 – 64 .
- Lu , D. , Mausel , P. , Batistella , M. and Moran , E. 2005 . Land-Cover Binary Change Detection Methods for Use in the Moist Tropical Region of the Amazon: A Comparative Study . International Journal of Remote Sensing , 26 : 101 – 114 .
- Lu , D. , Mausel , P. , Brondizio , E. and Moran , E. 2004 . Relationships Between Forest Stand Parameters and Landsat TM Spectral Responses in the Brazilian Amazon Basin . Forest Ecology and Management , 198 : 149 – 167 .
- Margulis , S. 2004 . “Causes of Deforestation of the Brazilian Amazon,” World Bank Working Paper No. 22, The International Bank for Reconstruction and Development , Washington , DC : The World Bank .
- Mas , J.F. 1999 . Monitoring Land-Cover Changes: A Comparison of Change Detection Techniques . International Journal of Remote Sensing , 20 : 139 – 152 .
- Mas , J.F. and Puig , H. 2001 . Modalités De La Déforestation Dans Le Sud-Ouest De L’état Du Campeche, Mexique Deforestation in the State of Campeche (Southeastern Mexico) . Canadian Journal of Forest Research , 31 : 1280 – 1288 .
- Mas , J.F. , Puig , H. , Palacio , J.L. and Sosa-Lopez , A. 2004 . Modelling Deforestation Using GIS and Artificial Neural Networks . Environmental Modelling & Software , 19 : 461 – 471 .
- Mertens , B. , Poccard-Chapuis , R. , Piketty , M.G. , Lacques , A.E. and Venturieri , A. 2002 . Crossing Spatial Analyses and Livestock Economics to Understand Deforestation Processes in the Brazilian Amazon: The Case of Sao Felix Do Xingu in South Para . Agricultural Economics , 27 : 269 – 294 .
- Michalski , F. , Peres , C.A. and Lake , I.R. 2008 . Deforestation Dynamics in a Fragmented Region of Southern Amazonia: Evaluation and Future Scenarios . Environmental Conservation , 35 : 93 – 103 .
- Millington , A.C. , Velez-Liendo , X.M. and Bradley , A.V. 2003 . Scale Dependence in Multitemporal Mapping of Forest Fragmentation in Bolivia: Implications for Explaining Temporal Trends in Landscape Ecology and Applications to Biodiversity Conservation . ISPRS Journal of Photogrammetry and Remote Sensing , 57 : 289 – 299 .
- MMA . 2006 . Projeto Macrozonamiento Da Amazônia Legal. Mapas Temáticos Seleccionados (Texto Explicativo) , Brasília : Author .
- Moran , M.D. 2003 . Arguments for Rejecting the Sequential Bonferroni in Ecological Studies . Oikos , 100 : 403 – 405 .
- Munroe , D.K. , Southworth , J. and Tucker , C.M. 2004 . Modeling Spatially and Temporally Complex Land-Cover Change: The Case of Western Honduras . The Professional Geographer , 56 : 544 – 559 .
- Murdoch , J.C. and Sandler , T. 2002 . Economic Growth, Civil Wars, and Spatial Spillovers . Journal of Conflict Resolution , 46 : 91 – 110 .
- Musa Filho , M. 1993 . Os Grandes Projetos De Desenvolvimento E Seus Impactos Ambientais in a Amazônia Brasileira Em Foco . Campanha Nacional em Defesa do Desenvolvimento da Amâzonia , 19 : 33 – 40 .
- Nagendra , H. , Southworth , J. and Tucker , C. 2003 . Accessibility as a Determinant of Landscape Transformation in Western Honduras: Linking Pattern and Process . Landscape Ecology , 18 : 141 – 158 .
- Neave, P. (2007), Flash Earth. http://www.flashearth.com/ (http://http://www.flashearth.com/) (Accessed: 10 December 2006 ).
- Nelson , G. , De Pinto , A. , Harris , V. and Stone , S. 2004 . Land Use and Road Improvements: A Spatial Perspective . International Regional Science Review , 27 : 297 – 325 .
- Nepstad , D. , Carvalho , G. , Barros , C.A. , Alencar , A. , Capobianco , P.J. , Bishop , J. , Moutinho , P. , Lefebvre , P. , Lopes Silva , U. and Prins , E. 2001 . Road Paving, Fire Regime Feedbacks, and the Future of Amazon Forests . Forest Ecology and Management , 154 : 395 – 407 .
- Nepstad , D.C. , Stickler , C.M. and Almeida , O.T. 2005 . Globalization of the Amazon Soy and Beef Industries: Opportunities for Conservation . Conservation Biology , 20 : 1595 – 1603 .
- Nepstad , D.C. , Stickler , C.M. , Soares-Filho , B. and Merry , F. 2008 . Interactions Among Amazon Land Use, Forests and Climate: Prospects for a Near-Term Forest Tipping Point . Philosophical Transactions of the Royal Society B , 363 : 1737 – 1746 .
- Nepstad , D.C. , Verissimo , A. , Alencar , A. , Nobre , C. , Lima , E. , Lefebvre , P. , Schlesinger , P. , Potter , C. , Moutinho , P. , Mendoza , E. , Cochrane , M. and Brooks , V. 1999 . Large-Scale Impoverishment of Amazonian Forests by Logging and Fire . Nature , 398 : 505 – 508 .
- Odland , J. 1988 . Spatial Autocorrelation , Newbury Park , CA : Sage .
- O'Hara Hines , R.J.O. and Hines , W.G.S. 1995 . Exploring Cook's Statistic Graphically . American Statistician , 49 : 389 – 394 .
- Otchere , F.A. , Veiga , M.M. , Hinton , J.J. , Farias , R.A. and Hamaguchi , R. 2004 . Transforming Open Mining Pits Into Fish Farms: Moving Towards Sustainability . Natural Resources Forum , 28 : 216 – 223 .
- Pedlowski , M.A. , Dale , V.H. , Matricardi , E.A.T. and da Silva Filho , E.P. 1997 . Patterns and Impacts of Deforestation in Rondonia, Brazil . Landscape and Urban Planning , 38 : 149 – 157 .
- Perneger , T.V. 1998 . What's Wrong with Bonferroni Adjustments . British Medical Journal , 316 : 1236 – 1238 .
- Pfaff , A. , Robalino , J. , Walker , R. , Aldrich , S. , Caldas , M. , Reis , E. , Perz , S. , Bohrer , C. , Arima , E. , Laurance , W. and Kirby , K. 2007 . Road Investments, Spatial Spillovers, and Deforestation in the Brazilian Amazon . Journal of Regional Science , 47 : 109 – 123 .
- Pinto da Cunha , J.M. 2006 . Dinâmica Migratónia e o Processo de Occupa¸c˜o do Centero-Oeste Brasileiro: o Caso de Mato Grasso . XXVth IUSSP International Population Conference , July 2005, Tours – France, Session No. 409
- Pires Luiz , C.H. , Gomes Leal , L.F. , Silva Santos , M.F. , Moreira Tosatti , S. and Maillard , P. 2009 . A Utilização de Imagens do Satélite CBERS-2 para o Controle da Mata Ciliar . in Anais XIV Simpósio Brasileiro de Sensoriamento Remoto , : 2809 – 2816 . 25–30 April 2009, INPE, Natal, Brasil
- Pontius , G.R. and Malanson , J. 2005 . Comparison of the Structure and Accuracy of Two Land Change Models . International Journal of Geographical Information Science , 19 : 243 – 265 .
- Pontius , R.G.J. and Batchu , K. 2003 . Using the Relative Operating Characteristic to Quantify Certainty in Prediction of Location of Land Cover Change in India . Transactions in GIS , 7 : 467 – 484 .
- Popkin , B.M. 1993 . Nutritional Patterns and Transitions . Population and Development Review , 19 : 138 – 157 .
- Rao , M. , Terborgh , J. and Nunez , P. 2001 . Increased Herbivory in Forest Isolates: Implications for Plant Community Structure and Composition . Conservation Biology , 15 : 624 – 633 .
- Ribeiro, C., Meitner, M., da Veiga, M. (2006), “Environmental Protection in Brazil: Where the Truth Lies,” in 26th ESRI International User Conference, San Diego, CA. http://Proceeding.esri.com/library/userconf/proc06/papers/papers/pap_1680.pdf (http://Proceeding.esri.com/library/userconf/proc06/papers/papers/pap_1680.pdf) (Accessed: 23 August 2007 ).
- Rodriguez , E. , Morris , C. , Belz , J. , Chapin , E. , Martin , J. , Daffer , W. and Hensley , S. 2005 . “An Assessment of the SRTM Topographic Products,” , Pasadena , CA : Technical Report JPL D-31639, Jet Propulsion Laboratory .
- Schneider , L.C. and Pontius , R.G.J. 2001 . Modeling Land-Use Change in the Ipswich Watershed, Massachusetts, USA . Agriculture, Ecosystems and Environment , 85 : 83 – 94 .
- Scott , A.J. and Wild , C.J. 1986 . Fitting Logistic-Models Under Case-Control or Choice Based Sampling . Journal of the Royal Statistical Society Series B-Methodological , 48 : 170 – 182 .
- Scott , A.J. and Wild , C.J. 1991 . Fitting Logistic-Regression Models in Stratified Case-Control Studies . Biometrics , 47 : 497 – 510 .
- Seidl , A.F. , Silva , J. and Moraes , A.S. 2001 . Cattle Ranching and Deforestation in the Brazilian Pantanal . Ecological Economics , 36 : 413 – 425 .
- Smith , J. , van de Kop , P. , Reategui , K. , Lombardi , I. , Sabogal , C. and Diaz , A. 1999 . Dynamics of Secondary Forests in Slash-and-Burn Farming: Interactions Among Land Use Types in the Peruvian Amazon . Agriculture, Ecosystems & Environment , 76 : 85 – 98 .
- Soares-Filho , B. , Alencar , A. , Nepstad , D. , Cerqueira , G. , Vera Diaz , M.C. , Rivero , S. , Solorzano , L. and Voll , E. 2004 . Simulating the Response of Land-Cover Changes to Road Paving and Governance Along a Major Amazon Highway: The Santarem-Cuiaba Corridor . Global Change Biology , 10 : 745 – 764 .
- Soares-Filho , B.S. , Nepstad , D.C. , Curran , L.M. , Cerqueira , G.C. , Garcia , R.A. , Ramos , A. , Voll , E. , McDonald , A. , Lefebvre , P. and Schlesinger , P. 2006 . Modelling Conservation in the Amazon Basin . Nature , 440 : 520 – 523 .
- Southworth , J. and Tucker , C. 2001 . The Influence of Accessibility, Local Institutions, and Socioeconomic Factors on Forest Cover Change in the Mountains of Western Honduras . Mountain Research and Development , 21 : 276 – 283 .
- Souza, C.J., Veríssimo, A., Micol, L., Guimarães, S. (2006) Boletim Transparência Florestal. Estado de Mato Grosso. http://www.icv.org.br/w/library/btf07-jul06.pdf (http://www.icv.org.br/w/library/btf07-jul06.pdf) (Accessed: 19 February 2007 ).
- Sparovek , G. , Barretto , A. , Berndes , G. , Martins , S. and Maule , R. 2008 . Environmental, Land-Use and Economic Implications of Brazilian Sugarcane Expansion 1996–2006 . Mitigation and Adaptation Strategies for Global Change , doi: doi 10.1007/s11027-008-9164–3
- Stolle , F. , Chomitz , K. , Lambin , E. and Tomich , T. 2003 . Land Use and Vegetation Fires in Jambi Province, Sumatra, Indonesia . Forest Ecology and Management , 179 : 277 – 292 .
- Terborgh , J. and Nunez-Iturri , G. 2006 . “ Disperser-Free Tropical Forests Await an Unhappy Fate ” . In in Emerging Threats to Tropical Forests , Edited by: Laurance , W. and Peres , C. Chicago : University of Chicago Press .
- Tucker , C.J. , Grant , D.M. and Dykstra , J.D. 2004 . NASA's Global Orthorectified Landsat Data Set . Photogrammetric Engineering and Remote Sensing , 70 : 313 – 322 .
- Veiga , M.M. and Hinton , J.J. 2002 . Abandoned Artisanal Gold Mines in the Brazilian Amazon: A Legacy of Mercury Pollution . Natural Resources Forum , 26 : 15 – 26 .
- Verburg , P.H. , de Nijs , T.C. , van Eck , J.R. , Visser , H. and de Jong , K. 2004 . A Method to Analyse Neighbourhood Characteristics of Land Use Patterns . Computers, Environment and Urban Systems , 28 : 667 – 690 .
- Verburg , P.H. , Overmars , K.P. and Witte , N. 2004 . Accessibility and Land-Use Patterns at the Forest Fringe in the Northeastern Part of the Philippines . The Geographical Journal , 170 : 238 – 255 .
- Verburg , P.H. , Schot , P.P. , Dijst , M. and Veldkamp , A. 2004 . Land Use Change Modelling: Current Practice and Research Priorities . GeoJournal , 61 : 309 – 324 .
- Verburg , P.H. and Veldkamp , A. 2004 . Projecting Land Use Transitions at Forest Fringes in the Philippines at Two Spatial Scales . Landscape Ecology , 19 : 77 – 98 .
- Vosti , S.A. , Braz , E.M. , Carpentier , C.L. , D'Oliveira , M.V.N. and Witcover , J. 2003 . Rights to Forest Products, Deforestation and Smallholder Income: Evidence From the Western Brazilian Amazon . World Development , 31 : 1889 – 1901 .
- Wakefield , J. 2004 . A Critique of Statistical Aspects of Ecological Studies in Spatial Epidemiology . Environmental and Ecological Statistics , 11 : 31 – 54 .
- Walker , R. , Moran , E. and Anselin , L. 2000 . Deforestation and Cattle Ranching in the Brazilian Amazon: External Capital and Household Processes . World Development , 28 : 683 – 699 .
- Walker, R.T., and Qi, J. (2009), LBA-ECO LC-24 MODIS 1km Forest Cover Map of South America: 2001. Data set. Oak Ridge, TN: Oak Ridge National Laboratory Distributed Active Archive Center. Retrieved 10 May 2010 http://www.daac.ornl.gov (http://http://www.daac.ornl.gov)
- Wrigley , N. , Holt , D. , Steel , D. and Tranmer , M. 1996 . “ Spatial Modelling and the Ecological Fallacy ” . In in Spatial Analysis: Modelling in a GIS Environment , Edited by: Longley , P.A.B. and Batty , M. Cambridge : GeoInformation International .
- Wu , F. 1998 . SimLAND: A Prototype to Simulate Land Conversion Through the Integrated GIS and CA with AHP-Derived Transition Rules . International Journal of Geographical Information Science , 12 : 63 – 82 .
- Wu , F. 2002 . Calibration of Stochastic Cellular Automata: The Application to Rural-Urban Land Conversions . International Journal of Geographical Information Science , 16 : 795 – 818 .
- Wu , F.L. and Webster , C.J. 2000 . Simulating Artificial Cities in a GIS Environment: Urban Growth Under Alternative Regulation Regimes . International Journal of Geographical Information Science , 14 : 625 – 648 .
- WWF (2006) Biomas Brasileiros http://www.wwf.org.br/natureza_brasileira/biomas/index.cfm (http://http://www.wwf.org.br/natureza_brasileira/biomas/index.cfm) (Accessed: 26 May 2006 ).