Abstract
Improving the understanding of land use and land cover is a major research challenge for the human-environmental sciences and is essential for many aspects of global environmental research. Considering seasonal vegetation dynamics or phenological dynamics in multi-year series leads to a broader view of land use and land cover. This study is based on the hypothesis that a pixel representing a complex but consistent land use has a typical, distinct and repeated temporal pattern of vegetation index inter-annually, which can be used as characteristic signatures for land use classification. Considering the seasonal events and climatic variability in Indonesia, we characterized the temporal vegetation dynamics of long-term land use by using multi-temporal Moderate Resolution Imaging Spectroradiometer (MODIS) enhanced vegetation index 16-day composite data from 2001 to 2007, and then generated a land use map using those characteristics. Accuracy assessment of the results showed the need to evaluate such methods for land use types that do not have a consistent yearly pattern. On the other hand, the identification of the intensive agriculture lands, such as paddy rice and upland, was satisfactory. Although the mixed pixel issue is quite problematic when using MODIS data, the results indicate that MODIS data offer great promise for characterizing seasonal as well as multi-year variation at large scales. Indeed, the methodology proposed in this research distinguished among many specific land use classes based on temporal land cover information properties. Characterization of temporal vegetation dynamics patterns would provide sufficient, significant and useful information regarding the patterns of land use; consequently it should be possible to consider the actual, subtle nature of inter-annual land use change as well as overall land use.
Introduction
Information on global land use and land cover (LULC) is essential for many aspects of global environmental research (Foley et al. Citation2005; Turner, Lambin, and Reenberg Citation2007). Earth observation from satellites provides spatial measurements of biophysical surface attributes, such as vegetation cover, biomass and landscape heterogeneity (Lambin, Geist, and Rindfuss Citation2006). Analysis of multi-year time series of those attributes and their seasonal evolutions has led to a broader view of LULC (Ramankutty et al. Citation2006; Philippon, Jarlan, Martiny, Camberlin, and Mougin Citation2007; Erasmi, Propastin, Kappas, and Panferov Citation2009).
Commonly, land surface is characterized by distinguishing among different land cover types (Jansen and Gregorio Citation2002). Land cover addresses the layer of soils and biomass, including natural vegetation, crops and human structures that cover the land surface. Land cover is thus directly observable, both in the field as well as from remote sensing images. Land use, in contrast, refers to the purposes for which humans exploit the land cover (Lambin et al. Citation2006) and includes the land management practices. In many cases, land cover may drastically change without any change in land use. The mixed use of land cover and land use in maps and models leads to inconsistencies in land change assessments (Verburg, Van De Steeg, Veldkamp, and Willemen Citation2009).
The monitoring of land surface continuously in space and time, at both seasonal and inter-annual scales, allows characterization of the temporal vegetation dynamics of land use (Lambin, Geist, and Lepers Citation2003; Verburg et al. Citation2009), in other words, distinguishing the actual type of land use based on land cover dynamics. In tropical regions, for example Java Island, Indonesia, such discrimination is an important issue due to the sequential land use patterns that exist in the field. An area classified as a paddy field might undergo a sequence of covers through the year, such as (1) paddy–bareland–secondary crops–bareland, (2) paddy–bareland–inundated–paddy–bareland–secondary crops and (3) paddy–bareland–secondary crops–bareland–inundated–paddy, where the sequence is repeated year after year following the seasons. These temporal covers are characteristic of the cropping systems in some regions of the tropics. In this example, attributes of the land surface are: vegetation (paddy), soil (bareland) and water (inundated), which is defined as land cover. On the other hand, the land use type, which describes the purpose of land, is the paddy rice field. Similar distinctions are important when considering plantation crops, forest and other types, even if there is a difference in time scale of the trajectory cycle (Verburg, Veldcamp, Willemen, Overmars, and Castella Citation2004).
Vegetation dynamics have attracted attention as a means for better understanding land characterization (Verburg et al. Citation2009). Understanding vegetation dynamics requires the characterization of vegetation changes at different temporal scales (Lunetta, Knight, Ediriwickrema, Lyon, and Worthy Citation2006; Galford et al. Citation2008; Martinez and Gilabert Citation2009). The appropriate temporal changes are characteristic of biologically complex ecosystems and are known as phenological dynamics of terrestrial ecosystems, reflecting the response of the earth's biosphere to annual dynamics of the earth's climate and hydrologic cycle (Zhang et al. Citation2003). Moreover, vegetation phenology, as used and studied by remote sensing, refers to the relationship between climate and periodic development of photosynthetic biomass (Li and Kafatos Citation2000; Jakubauskas, Legates, and Kastens Citation2002; Kang et al. Citation2003).
Characterization of vegetation dynamics has often been made by using vegetation index values (Zhang et al. Citation2003; Knight, Lunetta, Ediriwickrema, and Khorram Citation2006; Lunetta et al. 2006). The temporal dynamics of those index values are useful for distinguishing land surface conditions by differentiating among vegetation types and their distributions (DeFries, Hansen, and Townshend Citation1995; Hansen, DeFries, Townshend, and Sohlberg Citation2000; Lloyd Citation2000). The vegetation indices relate to the amount of green leaf biomass. They are computed by algebraic combination of two or more spectral bands and are designed to enhance the contribution of vegetation properties (Huete, Liu, Batchily, and Van Leeuwen Citation1997). The normalized difference vegetation index (NDVI) and the enhanced vegetation index (EVI) are commonly used to measure reliable spatial and temporal inter-comparisons of terrestrial photosynthetic activity and canopy structural variations (Huete et al. Citation2002). Whereas the NDVI is chlorophyll sensitive, the EVI is more responsive to canopy structural variations, including leaf area index, canopy type, plant physiognomy and canopy architecture (Gao, Huete, Ni, and Miura Citation2000). The EVI was developed to optimize the vegetation signal with improved sensitivity in high biomass regions and improved vegetation monitoring through a decoupling of the canopy background signal and a reduction in atmosphere influences (Huete et al. Citation2002).
Some previous studies (Goward, Tucker, and Dye Citation1985; Justice, Townshend, Holben, and Tucker Citation1985; Loveland, Merchant, Ohlen, and Brown Citation1991; Schwartz Citation1999) emphasize that time-series images are needed to recognize the vegetation phenology. In addition, DeFries and Townshend (Citation1994) also pointed out that multi-year data sets would improve the capability to (1) characterize portions of the study area, such as some sites with high inter-annual variability in vegetative activity and (2) ensure that a classification result and land cover change analysis were not the product of short-term fluctuations in vegetative activity. The uses of multi-year data sets are necessary to develop methodologies that utilize information on inter-annual variations to increase the accuracy of the land surface characterization. Initially this work relied on the use of coarse spatial resolution (>1.0 km2) Advanced Very High Resolution Radiometer data but, with the availability of the Moderate Resolution Imaging Spectroradiometer (MODIS) sensor on board Terra and Aqua Satellites after launch in December 1999 and May 2002, respectively, the time-series data analysis can be applied to 250 m pixels (Privette et al. Citation2002; Lobell and Asner Citation2004; Xiao, Liang, Wang, Song, and Wu Citation2009).
Extending the themes mentioned above, this study is based on the hypothesis that a pixel representing a complex but consistent land use has a typical, distinct and repeated temporal pattern of vegetation index inter-annually, which can be used as a characteristic signature for land use classification Consequently, a land use map could be drawn from the temporal pattern of the time-series data. The approach in this article is different from the previous approach used to produce the available land use/cover maps of Indonesia, since we consider a variability of temporal vegetation dynamics in land use classes. The consideration of temporal vegetation dynamics of land use could also be used to support the land use change analysis at a regional scale. This article will investigate temporal vegetation dynamics of long-term land use considering seasonal events and climatic variability in a tropical region. Then, we will generate a land use map using those temporal patterns.
Methods
Study area
This study encompassed all of the island of Java which is located on the southern rim of the Indonesian archipelago (upper left corner: 5°52′S 105°04′E; lower right corner: 8°47′S 114°36′E) and has an area of 132,792 km2 (). The island is administratively divided into six provinces, namely Banten, Jakarta (special capital region), West Java, Central Java, Yogyakarta (special region) and East Java.
The climate of Java reflects the region's location in the tropics, with temperature and humidity remaining constant throughout the year, and rainfall varying between the seasons. Most areas receive 2000–4000 mm of rain annually. The driest areas, with less than 1500 mm, are along the north coast reaching a minimum of less than 1000 mm around Asembagus in the northeast. There are dry intermontane basins with less than 2000 mm around Bandung and Garut in West Java; around Surakarta and Yogyakarta in the centre; and around Madiun, Kediri and the Malang basin in East Java. The wettest areas of Java are mostly inland. Over 4000 mm fall on the mountains bordering the Sunda Strait and on or close to the upper slopes of most peaks in the Central Volcanic Mountains. These include the northern slopes of Ragajembangan, which is the wettest place in Java with more than 6000 mm (Land Resources Department/Bina Program Citation1990). In general, from November until March, the zone of heavy rainfall covers most of Java, while from May to September; the zone of rainfall is limited to the southeast, leaving the eastern parts of the island dry.
According to the BPS-Statistics of Indonesia (Citation2003), about 70.62% of Java is considered to be agricultural, with land use as follows: paddy fields, mixed gardens, uplands/drylands, fishponds and plantations, with as much as 5.43% of the area covered by settlements. Furthermore, Ministry of Public Works (Citation2002) stated that paddy rice accounts for about 2,807,770 ha or 21.14% of Java's total area, while uplands/drylands and non-irrigated areas cover about 6,352,850 ha or 47.84% of the total area.
Forests in Java cover 22.98% of Java's area, consisting 1,246,728 ha of production forest (9.39%), 550,849 ha of protection forest (4.15%) and 529,702 ha of conservation forest (3.99%) (Department of Forestry Citation2002). According to the Act of the Republic of Indonesia No. 41 of 1999 on forestry, a conservation forest is a forest area with specific characteristics, having the main function of preserving plant and animal diversity and its ecosystem. Meanwhile, a protection forest is a forest area having the main function of protecting life-supporting systems for hydrology, preventing floods, controlling erosion, preventing sea water intrusion and maintaining soil fertility (State Gazette of the Republic of Indonesia Citation1999).
Data
Satellite image
The MODIS product used in this study is the Vegetation Indices (VI) Composite 16-day Global 250 m SIN Grid V005 or MOD13Q1 product, which provided the needed vegetation phenology data. In addition, the product had already been systematically corrected for the effects of gaseous and aerosol scattering. The MODIS EVI is embedded in the MOD13Q1 product. The MODIS Land Discipline Group (MODLAND Citation2010) developed the EVI for use with MODIS data following this equation:
The EVI data were developed in the above form (EquationEquation (1)(1)) in order to optimize the vegetation signal with improved sensitivity in high biomass regions. The EVI also minimizes atmospheric influences with the ‘aerosol resistance’ term which uses the blue band to correct aerosols influence in band red (Huete et al.
Citation1997). In this study we used the MODIS EVI data sets which were acquired from January 2001 to December 2007 and captured 161 time series with the interval time 16 days. The data were obtained at no cost from USGS Land Processes Distributed Active Archive Center (USGS LP DAAC Citation2009a). The study area is covered by two MODIS tiles: h28v09 and h29v09.
MODIS EVI data were extracted from the MODIS VI product (MOD13Q1) using the MODIS Reprojection Tool (USGS LP DAAC Citation2009b) and the selected output format was GeoTIFF and coordinate system was geographic coordinate systems on datum World Geodetic System of 1984. The tiled MODIS EVI data were then mosaicked and clipped to cover Java for each composite period and then sequentially stacked to produce the time-series data set.
To examine the details of the temporal pattern of the MODIS EVI, we used a finer spatial resolution Landsat 7 Enhanced Thematic Mapper Plus (ETM+) as well as reference data derived from the ground survey points. The study area is covered by 18 scenes of Landsat images, collected from 2001 to 2007.
Constraints of the MODIS EVI product
Although MODIS data have some advantages in providing basic information related to vegetation phenology, time series of these data inevitably contain disturbances caused by atmospheric variability (Huete and Liu Citation1994) and aerosol scattering (Xiao, Braswell, Zhang, Boles, and Frolking Citation2003). However, improving aerosol correction at the surface reflectance level (MOD09) and a new filtering scheme in the VI algorithm implemented in collection 5.0 have positively impacted the MODIS VI (Didan and Huete Citation2006).
Moreover, to get a greater percentage of clear-sky data, the maximum value composited method is applied to the MODIS VI and is combined with the MODIS BRDF (bidirectional reflectance distribution function) or MOD43 product to generate the 16-day composite MODIS VI (MOD13Q1 product) (Huete, Justice, and Van Leeuwen Citation1999). Nevertheless, if the composite period is too long, the land surface does not remain static; and if it is too short, the atmospheric disturbance cannot be removed effectively (Lu, Liu, and Liang Citation2007); consequently, there are some residual errors. Such noise degrades the data quality and introduces considerable uncertainty in temporal sequences, confusing the analysis of temporal images sequences by introducing significant variations in the EVI time-series data. Therefore, noise reduction or fitting a model to observed data is necessary before phenological stages can be determined (Sakamoto et al. Citation2005; Lu et al. Citation2007).
Various strategies for image preprocessing including smoothers have been applied, such as polynomial and median filters (Van Dijk, Callis, Sakamoto, and Decker Citation1987), a moving window to select the local maximum VI (Viovy, Arino, and Belward Citation1992), temporal window operation (Park, Tateishi, and Matsuoka Citation1999), logistic curve fitting (Zhang et al. Citation2003), the asymmetric Gaussian function fitting approach (Johnson and Kasischke Citation1998), principal component analysis (Li and Kafatos Citation2000) and the Savitzky–Golay filter approach (Chen et al. Citation2004). Several spectral-frequency techniques have also been used, including Fourier-based fitting methods for separating the high-frequency components of noise and the low-frequency components of seasonal changes of VI (Sellers et al. Citation1994; Azzali and Menenti Citation2000; Lunetta et al. 2006) and, most recently, wavelet decomposition, which has been used to denoise and to build a vegetation signatures through feature extraction of MODIS multi-temporal (Bruce, Mathur, and Byrd Citation2006), to characterize crop phenology (Sakamoto et al. Citation2005), to detect temporal changes of annual flooding (Sakamoto et al. Citation2007), to determine changes in the expansion and intensification of crops (Galford et al. Citation2008) as well as to investigate vegetation dynamics in terrestrial ecosystem (Martinez and Gilabert Citation2009).
Image processing
DeFries and Townshend (Citation1994) explained that supervised classification based on temporal change differences faces several issues. First, data sets of a sufficient quality must be available in order to carry out the analysis. Second, in order to carry out a classification each land cover/use type to be included, each category must exhibit a distinct temporal signature based on the temporal pattern change. Third, there must be a systematic procedure for validation of the result. The image processing was designed to addresses these issues and illustrates the process of image data handling and analysis. We used wavelet transforms to reduce the residual noise and reconstruct the temporal pattern of image data. The denoised data were then clustered into several significant temporal patterns, and then the supervised classification was performed based on those temporal patterns.
Figure 2. Image preprocessing and analysis flow. LU, land use; ETM+, Enhanced Thematic Mapper Plus; MODIS, Moderate Resolution Imaging Spectroradiometer; EVI, enhanced vegetation index.
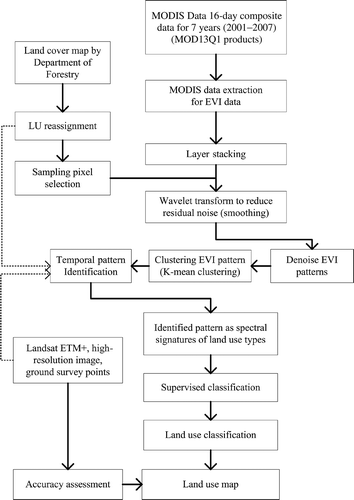
Image transformation using wavelet function
The wavelet transform can decompose signals in time-frequency space in order to identify and reduce the overall noise as well as to maintain useful information in the time-series data. Furthermore, Sakamoto et al. (Citation2005) concluded that the wavelet transform gave the best time profile of EVI for the purpose of detecting phenology stages, and they proposed the Wavelet-based Filter for determining Crop Phenology to remove high-frequency noise in crop phenology data. Galford et al. (Citation2008) has applied the Wavelet-based Filter for determining Crop Phenology in areas influenced by the tropical rainy season in southwestern Brazilian Amazon. They have demonstrated the stability of the wavelet approach to filter out noises in EVI time-series data. In this study, the wavelet transform is applied to a time series of MODIS EVI data in order to generate a land use map based on the annual pattern of land use types on Java Island, Indonesia.
The wavelet transform decomposes a signal into different scales by successively translating and convolving the elements of a high-pass and low-pass scaling filter associated with the mother wavelet. These filters retain the large- and small-scale components of the signals also known as the approximation (A) and detail (D) series, respectively. For most practical applications, the discrete wavelet transform, which analyses signals over a discrete set of scales, follows a hierarchical algorithm for such decomposition, known as the pyramid algorithm (Mallat Citation1989).
In the first level of the decomposition f(t) = A 1 + D 1 the signal has a low-pass filtered component, A 1, and a high-pass filtered component, D 1. In a second step, the approximation A 1 is split as A 1 = A 2 + D 2 and so on. The relationship Dj = Aj– 1 – Aj gives us information about the portion of the signal that can be attributed to variations between the scales [j–1, j]. shows an example of such decomposition corresponding to the paddy field in Java Island.
In order to analyse the fluctuation of the EVI value of each land use type, we used the Coiflet mother wavelet because this wavelet shape gives remarkably good results in determining phenological stages as pointed out by Sakamoto et al. (Citation2005). The order in the wavelet function is a measure of the wavelet's smoothness, where a larger order produces a smoother wavelet (Burke Citation1994). In this processing, order 2 of the Coiflet function was used since the trend of that order is similar to the trend of the original data. This analysis was performed using MATLAB through the 1D multisignal wavelet analysis function (MathWorks Citation2009).
The MODIS EVI data preprocessing was conducted to provide a filtered data set to support multi-temporal (phenological) analysis. shows that the wavelet transform application effectively deals with discontinuous/sharp spike data (resulting from some unspecified error, i.e. residual errors).
Land use classification
Corresponding the previous land cover map
A land cover map provided by the Department of Forestry (DoF) (Citation2008) was used as a reference to select the pixels used to develop the time-series EVI. We used the land cover data set recognizing that the actual types of land use are distinguished based on land cover; therefore, each land cover might be attributed to a number of different land use types.
The DoF land cover map was generated based on the interpretation of Landsat 7 ETM+ acquired in 2005/2006 and performed by visual classification. This classification was performed by digitizing polygons based on image interpretation with minimum mapping unit (MMU) defined by means of the smallest polygon identified (Rocchini, Perry, Salerno, Maccherini, and Chiarucci Citation2006). The land cover map was produced at a scale of 1:250,000 with a MMU of 40 ha.
The DoF is concerned primarily with natural vegetation categories. Using the thematic baseline forestry and designation of forestry-aquatic area maps for 30 provinces at the scale of 1:250,000 (Department of Forestry Citation2008), delineated polygons for those classes could be labelled with two codes, representing categories undisturbed by human activities (primary type) and those disturbed (secondary type). Finally, the satellite data were classified into 23 land cover types (). The 23 categories seem sufficient to represent land cover types in Indonesia. This is near the maximum number of categories that could be discriminated effectively; identification of other categories would likely be difficult to achieve.
Table 1. The 23 categories of land cover type by the Department of Forestry
In addition, although the land cover map provided by the DoF was based on the Landsat ETM+ imagery, for which one pixel of image represents approximately 0.1 hectares of the earth surface (spatial resolution of Landsat about 30 m), the product was generalized based on the smallest polygons that could be identified by visual interpretation. As a result, the DoF classification resolution was effectively courser than a spectral classification using the Landsat data. This classification indicated that approximately 63–83% of 250 m pixel grids contained homogeneous cover types.
In the context of temporal pattern of land use, we need to resolve the differences among the classes in the data set in order to provide a consistent definition for pixel-based analysis. The process of reassigning land use categories based on the knowledge of the phenological pattern of each land use is shown in .
Table 2. The reassigning of land use categories
A number of pixels corresponding to each of the land use categories were selected randomly from the parent category (11 categories). More than one typical temporal pattern might be identified from each land use category, which represents a specific class of land use. The number of pixel samples to be taken for each category is given in .
Training sample sets
Previous studies (Tucker, Townshend, and Goff Citation1985; Townshend, Justice, and Kalb Citation1987; Knight et al. Citation2006) have derived land cover maps from NDVI at continental and regional scales based on phenological differences among cover types as reflected in temporal profiles of NDVI. Similar assumptions were used in this study, that is, that locations displaying similar temporal patterns are inferred to have a similar land use type. The land use types are in turn inferred to have relatively homogeneous characteristics such as fractions of vegetation covers and amount of biomass. The use of the long time series (2001–2007) of EVI was based on the hypothesis that the land use can be distinguished based on land cover dynamics because consistent land use has a typical, distinct and repeated temporal pattern of vegetation index inter-annually.
Distinguishing among temporal patterns in order to recognize land use types was accomplished using the k-means clustering method based on Euclidean distance in an EVI-space in which each EVI image provides one dimension of the cluster space, analogous to spectral clustering. Distinct clusters then represent different temporal patterns–temporal signatures as opposed to spectral signatures. The resulting patterns were then used to discriminate among pixels selected from the reassigned land cover map described in . The clustering method subdivides data sets into k clusters through an iterative process in order to optimize a criterion function (Jain, Murty, and Flynn Citation1999). Clustering yields a number of significant patterns which correspond to 11 categories (illustrated by ).
Figure 5. Clustering process to differentiate the variation of the temporal patterns. LU, land use; EVI, enhanced vegetation index.
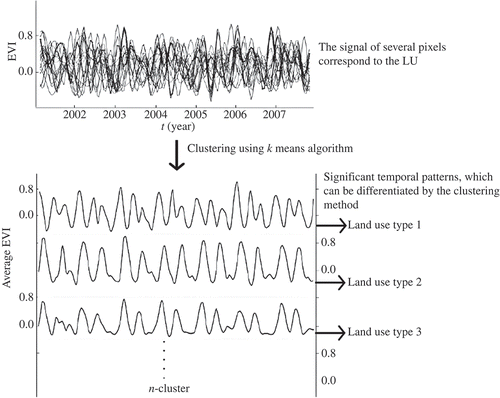
The number of significant patterns, each representing a specific class of land use, was determined based on a statistical measurement of how similar that pattern is to patterns in its own cluster compared to patterns in other clusters, and then combining clusters as needed. Furthermore, the significant pattern was identified based on its temporal profile, a corresponding ground survey data set and other image data such as Landsat or detailed information which was obtained using the ground survey as a reference. illustrates the use of multiple-date Landsat images in conjunction with a 7-year MODIS EVI pattern. Then, based on the information of those images and supported with local knowledge, the type of land use of that EVI pattern is identified. The same method was applied to other EVI patterns in study area. Once land use types were associated with their EVI patterns, we used those identified pixels as a signature sample (training sets) for supervised classification.
Figure 6. (a) The multiple dates of Landsat Enhanced Thematic Mapper Plus (ETM+) in conjunction with 7-year data sets; (b) temporal pattern of Moderate Resolution Imaging Spectroradiometer (MODIS) enhanced vegetation index (EVI) profile. Note that the EVI profile above is assigned to double a cropping system in an agriculture area. The EVI value of the red rectangle of each image on Figure 6a is located by the red point on Figure 6b.
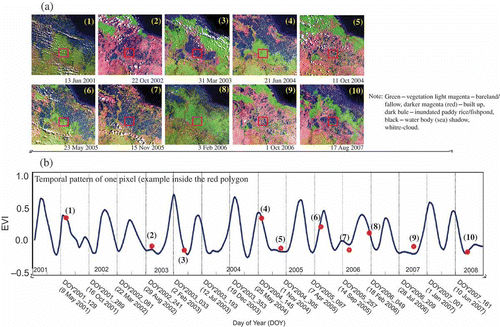
Supervised classification
Several specific land use types that broadly represent the major land use types on Java Island were distinguished according to their EVI temporal patterns. Then, to explore the feasibility of land use classifications for the whole study area (landscape scale), we ran a maximum likelihood supervised classification. The maximum likelihood procedure also classifies each pixel based on its temporal pattern to assign the land use type. The maximum likelihood algorithm (Richards and Jia Citation1999) belongs to the class of parametric classification methods. The mean vector and the variance–covariance matrix for each land use type are estimated using a number of pixels from training sets. Then, using the maximum likelihood rule, the multidimensional space is partitioned into subspaces each uniquely associated with one land use type (Keuchel, Naumann, Heiler, and Siegmund Citation2003).
The whole of the land use type in Java Island was then classified according to the temporal pattern properties of each pixel. Thus, if a pixel falls within the subspace associated with land use type ci , it is labelled as type ci . If the pixel falls within the subspace associated with land use type cj , it is labelled as that land use type, cj .
Accuracy assessment
The creation of a reference data set in order to assess the accuracy of the classification result is one important step in this land use study. A complicating factor in assessing the accuracy of a classification derived from 250 m image data in the Java Island was the disparity between the image pixel size and the average patch size of the landscape. A previous classification of the land cover by Ministry of Environment (MoE) (Citation2008) using Landsat ETM+ had an effective MMU of 2.4 ha. Overlaying a 250 m grid (corresponding to pixel size of MODIS) over this classification revealed that approximately only 20% of the grid contained homogeneous cover types at that MMU. The same issue was mentioned by Atkinson, Cutler, and Lewis (Citation1997), that one problem of moderate spatial resolution imagery is that the intrinsic scale of spatial variation in many land covers is finer than the scale of sampling imposed by the image pixels. The result is that most image pixels contain a mixture of land cover classes, referred to as sub-pixel mixing (Cracknell Citation1998).
The creation of a reference data set to assess the accuracy of a MODIS classification by providing sub-pixel land cover information was described by Knight et al. (Citation2006). They performed an accuracy assessment by sub-pixel reference information in which the USGS Digital Orthophoto Quadrangles were selected as the base data source. The same approach was carried out to our classification result.
To capture the variability, the study area was stratified by landscape, from mountains to coastal, and 2110 locations were selected based on random sampling. Each location was overlaid with a 250 m fishnet corresponding to the pixels of the MODIS EVI data, then 10 by 10 cells were selected as our plot size (a), and then each cell was overlaid with a 100-point dot (b). Each point was then associated with a specific land use based on its location on the Landsat image, a high-resolution image (Google Earth) and the reference data derived from the ground survey points. Whenever possible we provided more detailed land use information (specific classes). In the case of agricultural land, we were supported by a local investigation and other secondary data such as irrigated area, irrigation infrastructure, harvested area, data production and annual rainfall data sets. In situations where detailed information of land use was difficult to ascertain, we provided only a land use category (the parent category) label. This procedure provided the percentage of each land use class within the corresponding MODIS pixels; cells with over 70% of a single land use were taken to be homogeneous and used as a reference for accuracy assessment. The final number of samples in the reference data was 18,505 pixels and 10,000 pixels on the category level and the specific class level, respectively.
Figure 7. (a) A 250 m fishnet (Moderate Resolution Imaging Spectroradiometer (MODIS) pixel size) overlaid with Landsat Enhanced Thematic Mapper Plus (ETM+) and (b) cell on high-resolution image with 100-point dot grid overlay.
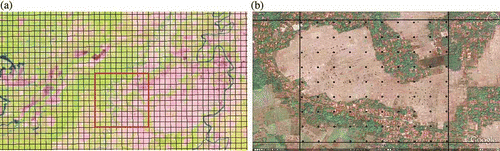
In this study, the accuracy of the classification was assessed using several accuracy parameters: total accuracy, user's accuracy and producer's accuracy. These accuracy parameters involve error matrices describing the number of sample units/points correctly classified, misclassified or not classified which can be characterized as commission error (user's accuracy) and omission error (producer's accuracy), respectively (Congalton and Green Citation1999).
On specific types of land use, for example paddy rice, we performed a statistical comparison with the statistics data at the village level (BPS-Statistics of Indonesia Citation2003) in order to assess the classification result for these specific classes. This was possible due to the availability of data regarding irrigated area, irrigation infrastructure, harvested area, field rate and data production for each village. The statistics’ data of 24,962 villages (1988 sub-districts) in Java Island were used. These data provided more detail about the accuracy, because they were based on a local census. However, this level of detail was only attempted for the land use type of paddy rice.
Results and discussions
Identification of temporal vegetation dynamics
As mentioned earlier, we identified each land use type from a long time series of land surface data sets. The seasonal pattern can be clearly understood from the temporal pattern of EVI. For example, the paddy field area is submerged during the rainy season and then vegetation starts to emerge.
In the upland category, the number of cultivation cycles (seedling to harvesting) that occur yearly depends on the availability of water. The fluctuation of average EVI in the upland area was differentiated into three significant patterns (a). These were identified as mixed garden (LU-1, upland type 1), upland mixed bush (LU-2, upland type 2) and upland with intensive agriculture farming (LU-3, upland type 3), respectively. In fact, intensive farming activity also existed in the upland type 1, but the area was relatively small. Another important feature of upland type 1 was that agriculture made extensive use of mixed cropping and intercropping of annual crops. These practices provide farmers an opportunity for harvesting diverse crops from the same land. On the other hand, the upland type 3 clearly shows the annual vegetation growth cycle, representing intensive cropping with multiple harvests in the upland area.
Figure 8. Identification results of land use type, which can be differentiated from the temporal patterns of enhanced vegetation index (EVI). Group of land use category: (a) upland, (b) paddy field, (c) forest, (d) mangrove, (e) plantation and (f) non-vegetation.
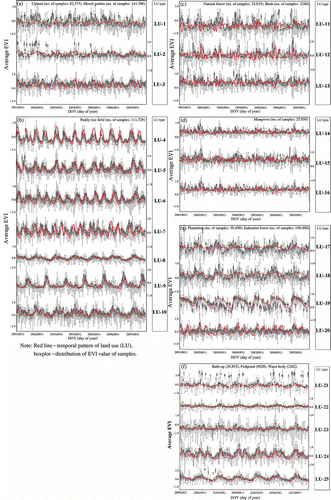
The EVI pattern of a paddy field segmented into seven significant types (b); nevertheless, the successfully classified patterns of a paddy rice cropping system were only represented by paddy type 1 (LU-4), paddy type 2 (LU-5), paddy type 3 (LU-6) and paddy type 4 (LU-7), while paddy type 5 (LU-8) corresponded to the upland which was planted at the end of a rainy season. Paddy type 6 (LU-9) was identified as fish/shrimp pond and became land during the long dry season. Paddy type 7 (LU-10) was classified into upland with intensive cropping.
Based on these patterns, the rice paddies could be sorted into several rice cropping systems. The farmers apply single, double or triple cropping patterns depending on the availability of water, either by irrigation or by rain water. In the irrigated areas, paddy rice can grow during dry season, so that rice crops can be grown 1 to 3 times in this land within a year. For that reason, the crops which are planted in irrigated areas display continuous EVI fluctuation, even in the dry season.
The EVI pattern of forest over 7 years was separable into three types (c). The examination of each pattern indicated that discrimination among them was related to differences in the forest composition. Forest composed of a homogeneous distribution of trees showed a different pattern than that of a heterogeneous mixture of trees. The forest type 1 (LU-11), type 2 (LU-12) and type 3 (LU-13) of the discriminated patterns were identified as forest mixed with bush, bush–shrub mixed grass and dry–land forest, respectively.
Leaf phenology in natural forest has been studied in many tropical regions, such as the Amazon forest (Nepstad et al. Citation1994; Jipp, Nepstad, Cassel, and Carvalho Citation1998; Asner, Townsend, and Braswell Citation2000), South America (Xiao, Hagen, Zhang, Keller, and Moore Citation2006) and Borneo forest (Shimada, Takahashi, and Limin Citation2006). Field measurements in Borneo forests have shown that the canopies respond to a seasonal dry period, with decrease of NDVI of 3–5%. On the other hand, in the tropical forests of South America, the maximum EVI typically occurred between the late dry season and the early rainy season. Moreover, Xiao et al. (2006) pointed out that leaf phenology in the tropical forests is not determined by the seasonality of precipitation, but rather may be driven by the availability of solar radiation. Although the above-referenced studies ascribed different causes for the trends, they successfully demonstrated the leaf phenological phenomena in tropical forest areas.
Similar to the pattern of forest described above, the EVI patterns of mangrove also indicated a stable EVI pattern that did not show any clear periodic fluctuation. Besides the density of vegetation, the variety of their patterns was affected by a number of geophysical factors to which the mangroves have adapted, such as the changing of salinity concentrations, sediment influxes and regular inundation by water (Bird and Ongkosongo Citation1980). Based on the different patterns, the mangrove could be classified into three classes (d). Mangrove type 1 (LU-14) represents a heterogeneous patch of mixed mangrove species, examples of which are distributed around Southern Java. Mangrove type 2 (LU-15) represents mangroves that are significantly influenced by water fluctuation. These areas are typically inundated longer. As with the zonation of mangroves suggested by Watson (Citation1928), mangrove type 2 was dominated by Avicennia spp., Sonneratia spp. and Bruguiera gymnorrhiza, while mangrove type 3 (LU-16) was identified as a mixed garden with bush.
In the case of plantations, different temporal patterns of EVI not only follow the growing season, but also depend on their maintenance, such as weeding and replanting. The plantations are widely distributed in Java: industrial forest plantations, rubber and oil palm plantations. The temporal pattern EVI of plantations was grouped into four types (e). Plantation type 1 (LU-17), type 2 (LU-18) and type 3 (LU-19) were identified as rubber plantations, oil palm plantations and industrial forest plantations, respectively. The industrial forest plantation was related primarily to teak plantations which exhibit leaf falls under water stress during a dry season, that is, between June and October. Meanwhile, plantation type 4 (LU-20) did not fit a plantation pattern, but is more characteristic of an intensive cropping system similar to upland type 3 (LU-3). Thus, plantation type 4 was identified as uplands in the plantation area.
The non-vegetation class is considered to represent water bodies, settlement and open areas. The clustering method differentiated the temporal patterns into five different types (f). Non-vegetation type 1 represented lands which are inundated during the rainy season, but start to be planted in the last days of the rainy season and turn into dry land during the dry season. Thus, we classified that type into rice-paddy in lowland areas (LU-21). This kind of land use is distributed around Eastern Java. Meanwhile, non-vegetation type 2 was classified as a water body, such as lake or river (LU-22). Non-vegetation type 3 was identified as settlement or mining area (open area) (LU-23). Similar to type 1, the fluctuations of EVI in non-vegetation type 4 (LU-24) and type 5 (LU-25) are characteristic of vegetation growth or cropping activities. Therefore, the LU-24 was classified into paddy rice with a double cropping system per year, and LU-25 was classified as a mixed cropping which becomes barren during a dry season.
From the identification of the temporal patterns of EVI, 25 types of land use were distinguished which broadly represent the major land use types of Java Island. These are listed in .
Table 3. The list of land use types as a summary of the analysis of EVI pattern
Land use mapping and accuracy assessment
In order to understand the distribution of each land use type, the 25 type of patterns were used as signature samples to classify the entire study area (). The result of that classification is shown in
Figure 9. The distribution of land use type which was classified by the temporal pattern of enhanced vegetation index (EVI).
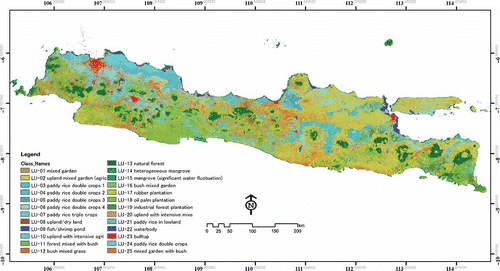
The use of temporal vegetation patterns allowed land use types to be differentiated into many specific land use classes, such as double and triple cropping system type of paddy rice, upland with intensive cropping and industrial forest plantation. Classification of specific classes was evaluated by 10,000 reference pixels, which revealed the overall accuracy and the overall κ statistics to be 71.07% and 64.94%, respectively ().
The greatest error was associated with the mixed garden, mixed bush and forest mixed bush classes. Specifically, 50.76% of mixed garden, 52.71% of bush–shrub mixed grass and 60.79% of forest mixed with bush were assigned to an incorrect category. The lower accuracy in these classes indicated the inability of MODIS to define the mixed type of vegetation and also reflected a difficulty with using temporal vegetation patterns to differentiate classes with no discernible periodical pattern. However, there is high confidence in pixels categorized as paddy rice and upland with several different cropping systems.
The classification results have been regrouped in terms of the 11 land use categories as follows: natural forest, mangrove, industrial forest plantation, plantation, bush, paddy rice field, mixed garden, upland, built-up/settlement, fishpond and water body. The overall accuracy and the overall κ statistics for the 11 land use categories are 80.16% and 70.94%, respectively. In addition, the omission error (producer's accuracy) and commission error (user's accuracy) are presented in .
The bulk of misclassification events contributing to the overall accuracy (19.84%) occurred mostly among the bush, plantation and built-up categories. Interpretation of bush is the most difficult, since this type did not exhibit a consistent temporal pattern, consequently almost a half of bush falls into other categories, such as mix garden and forest. The plantation types such as rubber plantation and oil palm plantation are also difficult to differentiate from forest based on their temporal vegetation profile, so almost 33% of the classified plantation falls into forest category in . In the case of the built-up category, the effective MMU is much smaller than each MODIS pixel, consequently each pixel contains a mixture of built-up and other classes, especially the agriculture lands, for example paddy rice, mixed garden and upland.
In the plantation and mixed garden cases, 52.11% and 46.80% of the error, respectively, is due to omission error (producer's accuracy), meaning that samples in these categories were assigned to other classes. The plantation type is determined by the area covered by a particular plantation crop (rubber and oil palm) which are widely distributed in Java Island. The poor accuracy of plantation and mixed garden types indicated that there is a difficulty with using MODIS data to resolve those areas.
Table 4. Confusion matrix for 25 specific classes of land use
Table 5. Confusion matrix for 11 categories of land use
Comparison of classification result of this study and land cover map derived by DoF
The classification result in terms of the 11 land use categories allows visual comparison with the land cover map provided by the DoF. These data sets were then overlaid to determine locations where the different land use type was present in both data sets.
shows that for certain types of land use, namely paddy rice, natural forest and uplands/drylands, both land use classification results appeared similar. In the case of the built-up class, the result of this study is slightly lower than the area extent indicated in the previous land cover map because of the inability of MODIS data to resolve the built-up areas. Meanwhile, the area extent of bush/shrub and mixed garden in the classification result is slightly greater than in the DoF map, because of many bare lands included in both classes. That probably occurred because the classification procedure used in this study was based on available imagery, which was not necessarily coincident with the presence of bare land.
Figure 10. Comparison of the 11 land cover/use categories derived by Department of Forestry and the classification result using of temporal pattern of Moderate Resolution Imaging Spectroradiometer (MODIS) enhanced vegetation index (EVI).
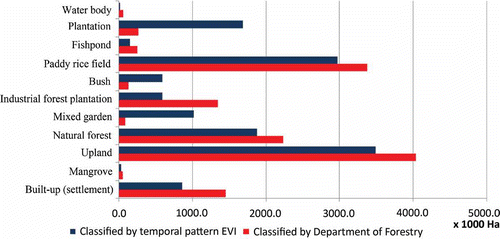
The most significant difference between our results and the previous land cover map is for the plantation and industrial forest plantation categories, as the areas of the categories seem to be reversed. This result indicates a difficulty with distinguishing between plantation and industrial forest plantation based on their temporal vegetation profile. shows that almost 35% and 22% of the classified plantation and industrial forest plantation, respectively, fall into other categories.
The comparison of the land use map derived by classifying the temporal patterns and the existing land cover map is shown in the Significantly, the land use classification assigned by long-term fluctuation in vegetative activity results a simpler land use map than the land cover map based on a single-date image due to short-term fluctuations in vegetative activity.
Assessment of classification result for paddy rice
For the specific class of paddy rice, there is the possibility of comparing the temporal classification results with statistical data collected at the village scale (BPS-Statistics Indonesia 2003) through information about the irrigated paddy area. Paddy rice field can be planted 2 to 3 times per year in irrigated land; however, there are many aspects that impact the actual pattern, such as social capital, farmer welfare, irrigated water, the price of rice and climate.
Figure 12a shows comparison of the irrigated paddy rice area at the province scale between the classification result of MODIS EVI and the tabulations by BPS-Statistics Indonesia (2003). The areas estimated by MODIS EVI classification for western, central and eastern Java were 10% higher, 26% lower and 42% lower, respectively, than the areas determined from the statistical data. Moreover, b indicated that the MODIS EVI procedure overestimates the area represented by large paddy areas and underestimates the number of small paddy fields. Analysis of the distribution revealed that most of the overestimates (points distributed below the best fit straight line) are located in western part of Java; most of the underestimates (points above the correlation line) are located in central and eastern Java. Such results suggest different characteristics of paddy rice on western Java as compared with central and eastern Java.
Figure 12. Comparison of the irrigated paddy rice area between the classification result of temporal pattern of Moderate Resolution Imaging Spectroradiometer (MODIS) enhanced vegetation index (EVI) and BPS-Statistics of Indonesia (Citation2003). (a) comparison at the province scale, (b) scatterplot at sub-district scale.
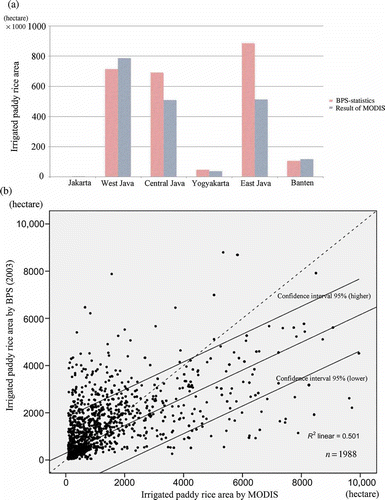
The result above is related to the different size MMU for irrigated paddies in each region. In some areas of central and eastern Java, it is difficult to resolve the minimum unit of paddy area with MODIS EVI; consequently, it is also difficult to characterize the temporal dynamics of paddy rice. Even if the irrigation infrastructure exists locally in these areas, if irrigated water is relatively limited, particularly likely in east Java, double or triple cropping may not be possible in a given year.
Although there is a difficulty caused by the MMU of paddy fields, the characterization of paddy fields in this study is based on available imagery, which was not necessarily coincident with temporarily bare land when employing a single-date image. Temporal vegetation dynamics would provide sufficient information of the use of land; meanwhile the single-date image interpretation is not sufficient to identify the actual land use type because of insufficient documentation of change events.
Conclusion
Monitoring land surfaces continuously in space and time allows characterization of the temporal vegetation dynamics. Considering seasonal vegetation dynamics in a multi-year series leads to a broader view of LULC. In some cases, the land cover may change without any changes in land use type. The mixed use of land cover and land use in maps leads to inconsistencies in land change assessment. This study is based on the hypothesis that land use has a typical, distinct and repeated temporal pattern of the vegetation index inter-annually.
The temporal patterns of land cover, repeated over several years, was sufficient to distinguish a number of significant temporal signatures which were characteristic of different land use types. On Java Island, the temporal pattern of EVI profiles was differentiated into 25 types of specific land use and was then used for land use classification.
Accuracy assessment on 11 types of land use categories (category class level) and 25 types of specific land use classes (specific class level) showed the need to evaluate the effectiveness of the method when applied to land use for which there was no periodic pattern such as bush, mixed garden and forest. However, identification of the intensive agriculture lands (such as paddy rice and upland) in Java Island showed satisfactory results.
Land use classification using the MODIS EVI 250 m data is substantially limited by the moderate spatial data resolution (250 m) (Lobell and Asner Citation2004); land use types smaller than approximately 6.25 ha have a low probability of being identified. As indicated by the accuracy assessment, the resolution limits classification accuracy; smaller features (e.g. bush and built-up) are mixed with other classes. Although the pixel mixing issue is a significant problem with the MODIS data, this study shows that it still offers great promise for characterizing temporal dynamic vegetation at a large scale, since the methodology presented in this research showed successfully the identification of several specific land use classes, such as paddy rice and upland, based on the characteristics of temporal vegetation patterns.
Characterizing of temporal vegetation dynamics patterns would provide sufficient, significant and useful information regarding the patterns of land use; consequently the set of analyses and techniques in this study should be applicable to a large range of applications such as quantifying dynamics of carbon stock, water resource management as well as land use/agricultural planning in regional scale. The land use map developed in this article will be available for potential users by contacting the authors.
Acknowledgements
The authors would like to thank the NASA LP DAAC for making MODIS data available; Brett Lien and Janice Wilson (NASA LP DAAC) for help when downloading the raw data, the GLOVIS USGS and Global Land Cover Facility, University of Maryland for providing us with Landsat image data as supporting image, also the Center for Environmental Research (PPLH IPB) for providing the image and secondary data in this research. We would like to thank the anonymous reviewers for the helpful comments on the manuscript. The field survey in this research was financially supported by JSPS with the Grant-in-Aid for Scientific Research (B) No. 22405033 and the JST Basic Research Programs, Core Research for Evolutional Science and Technology (CREST) in Japan.
References
- Asner , G.P. , Townsend , A.R. and Braswell , B.H. 2000 . Satellite Observation of El-Niño Effects on Amazon Forest Phenology and Productivity . Geophysical Research Letter , 27 : 981 – 984 .
- Atkinson , P.M. , Cutler , M.E.J. and Lewis , H. 1997 . Mapping Sub-Pixel Proportional Land Cover with AVHRR Imagery . International Journal of Remote Sensing , 18 : 917 – 935 .
- Azzali , S. and Menenti , M. 2000 . Mapping Vegetation-Soil-Climate Complexes in Southern Africa Using Temporal Fourier Analysis of NOAA-AVHRR NDVI Data . International Journal of Remote Sensing , 21 : 975 – 996 .
- Bird , E.C.F. and Ongkosongo , O.S.R. 1980 . Environmental Changes on the Coasts of Indonesia , Tokyo : United Nations University .
- BPS-Statistics of Indonesia . 2003 . Statistics of Indonesia 2003 , Indonesia : Badan Pusat Statistik .
- Bruce , L.M. , Mathur , A. and Byrd , J.D. 2006 . Denoising and Wavelet-Based Feature Extraction of MODIS Multi-Temporal Vegetation Signatures . GIScience & Remote Sensing , 43 : 67 – 77 .
- Burke , B. 1994 . The Mathematical Microscope: Waves, Wavelets, and Beyond. A Positron Named Priscilla: Scientific Discovery at the Frontier , Washington , DC : National Academy Press .
- Chen , J. , Jonsson , P. , Tamura , M. , Gu , Z. , Matsushita , B. and Eklundh , L. 2004 . A Simple Method for Reconstructing a High-Quality NDVI Time-Series Dataset Based on the Savitzky-Golay Filter . Remote Sensing of Environment , 91 : 332 – 344 .
- Congalton , R. and Green , K. 1999 . Assessing the Accuracy of Remotely Sensed Data: Principles and Practices , Boca Raton , FL : Lewis .
- Cracknell , A.P. 1998 . Synergy in Remote Sensing-What's in a Pixel? . International Journal of Remote Sensing , 19 : 2025 – 2047 .
- DeFries , R.S. , Hansen , M. and Townshend , J. 1995 . Global Discrimination of Land Cover Types from Metrics Derived from AVHRR Pathfinder Data . Remote Sensing of Environment , 54 : 209 – 222 .
- DeFries , R.S. and Townshend , J.R.G. 1994 . NDVI-Derived Land Cover Classifications at a Global Scale . International Journal of Remote Sensing , 15 : 3567 – 3586 .
- Department of Forestry . 2002 . Recalculation of Production Forest, Protection and Conservation Forest , 2nd , Jakarta , , Republic of Indonesia : The Agency of Forestry Planning, Department of Forestry .
- Department of Forestry . 2008 . Recalculation of Land Cover in Indonesia 2008 , Jakarta , , Republic of Indonesia : The Agency of Forestry Planning, Department of Forestry .
- Didan, K., and Huete, A.R. (2006), MODIS Vegetation Index Product Series. Collection 5 Change Summary. https://lpdaac.usgs.gov/lpdaac/products/modis_products_table/vegetation_indices/16_day_l3_global_250m/v5/terra (http://https://lpdaac.usgs.gov/lpdaac/products/modis_products_table/vegetation_indices/16_day_l3_global_250m/v5/terra) (Accessed: 29 May 2010 ).
- Erasmi , S. , Propastin , P. , Kappas , M. and Panferov , O. 2009 . Spatial Patterns of NDVI Variation over Indonesia and Their Relationship to ENSO Warm Events during the Period 1982–2006 . Journal of Climate , 22 : 6612 – 6623 .
- Foley , J.A. , DeFries , R. , Asner , G.P. , Barford , C. , Bonan , G. , Carpenter , S.R. , Chapin , F.S. , Coe , M.T. , Daily , G.C. , Gibbs , H.K. , Helkowski , J.H. , Holloway , T. , Howard , E.A. , Kucharik , C.J. , Monfreda , C. , Patz , J.A. , Prentice , I.C. , Ramankutty , N. and Snyder , P.K. 2005 . Global Consequences of Land Use . Science , 309 : 570 – 574 .
- Galford , G.L. , Mustard , J.F. , Melillo , J. , Gendrin , A. , Cerri , C.C. and Cerri , C.E. 2008 . Wavelet Analysis of MODIS Time Series to Detect Expansion and Intensification of Row-Crop Agriculture in Brazil . Remote Sensing of Environment , 112 : 576 – 587 .
- Gao , X. , Huete , A.R. , Ni , W. and Miura , T. 2000 . Optical–Biophysical Relationships of Vegetation Spectra Without Background Contamination . Remote Sensing of Environment , 74 : 609 – 620 .
- Goward , S.N. , Tucker , C.J. and Dye , D.G. 1985 . North American Vegetation Patterns Observed with the NOAA-7 Advanced Very High Resolution Radiometer . Plant Ecology , 64 : 3 – 14 .
- Hansen , M. , DeFries , R.S. , Townshend , J. and Sohlberg , R. 2000 . Global Land Cover Classification at 1 km Spatial Resolution Using a Classification Tree Approach . International Journal of Remote Sensing , 21 : 1331 – 1364 .
- Huete , A. , Didan , K. , Miura , T. , Rodriguez , E.P. , Gao , X. and Ferreira , L.G. 2002 . Overview of the Radiometric and Biophysical Performance of the MODIS Vegetation Indices . Remote Sensing of Environment , 83 : 195 – 213 .
- Huete, A.R., Justice, C., and Van Leeuwen, W. (1999), MODIS Vegetation Index (MOD13). Algorithm Theoretical Basis Document (ATBD) version 3. http://modis.gsfc.nasa.gov/data/atbd/land_atbd.php (http://modis.gsfc.nasa.gov/data/atbd/land_atbd.php) (Accessed: 29 May 2010 ).
- Huete , A.R. and Liu , H.Q. 1994 . An Error and Sensitivity Analysis of the Atmospheric- and Soil-Correcting Variants of the NDVI for the MODIS-EOS . IEEE Transactions on Geoscience and Remote Sensing , 32 : 897 – 905 .
- Huete , A.R. , Liu , H.Q. , Batchily , K. and Van Leeuwen , W. 1997 . A Comparison of Vegetation Indices over a Global Set of TM Images for EOS-MODIS . Remote Sensing of Environment , 59 : 440 – 451 .
- Jain , A.K. , Murty , M.N. and Flynn , P.J. 1999 . Data Clustering: A Review . ACM Computing Surveys , 31 : 264 – 323 .
- Jakubauskas , M.E. , Legates , D.R. and Kastens , J.H. 2002 . Crop Identification Using Harmonic Analysis of Time-Series AVHRR NDVI Data . Computers and Electronics in Agriculture , 37 : 127 – 139 .
- Jansen , L.J.M. and Gregorio , A.D. 2002 . Parametric Land Cover and Land-Use Classifications as Tools for Environmental Change Detection . Agriculture, Ecosystems and Environment , 91 : 89 – 100 .
- Jipp , P.H. , Nepstad , D.C. , Cassel , D. and Carvalho , C.R.D. 1998 . Deep Soil Moisture Storage and Transpiration in Forests and Pastures of Seasonally-Dry Amazonia . Climatic Change , 39 : 395 – 412 .
- Johnson , R.D. and Kasischke , E. 1998 . Change Vector Analysis: A Technique for the Multispectral Monitoring of Land Cover and Condition . International Journal of Remote Sensing , 19 : 411 – 426 .
- Justice , C.O. , Townshend , J.R.G. , Holben , B. and Tucker , C.J. 1985 . Analysis of the Phenology of Global Vegetation Using Meteorological Satellite Data . International Journal of Remote Sensing , 8 : 1271 – 1318 .
- Kang , S. , Running , S.W. , Lim , J.H. , Zhao , M. , Park , C.R. and Loehman , R. 2003 . A Regional Phenology Model for Detecting Onset of Greenness in Temperate Mixed Forests, Korea: An Application of MODIS Leaf Area Index . Remote Sensing of Environment , 86 : 232 – 242 .
- Keuchel , J. , Naumann , S. , Heiler , M. and Siegmund , A. 2003 . Automatic Land Cover Analysis for Tenerife by Supervised Classification Using Remotely Sensed Data . Remote Sensing of Environment , 86 : 530 – 541 .
- Knight , J.F. , Lunetta , R.S. , Ediriwickrema , J. and Khorram , S. 2006 . Regional Scale Land Cover Characterization Using MODIS-NDVI 250 m Multi-Temporal Imagery: A Phenology-Based Approach . GIScience & Remote Sensing , 43 : 1 – 23 .
- Lambin , E.F. , Geist , H.J. and Lepers , E. 2003 . Dynamics of Land-Use and Land-Cover Change in Tropical Regions . Annual Review of Environment and Resources , 28 : 205 – 241 .
- Lambin , E.F. , Geist , H.J. and Rindfuss , R.R. 2006 . “ Introduction: Local Processes with Global Impacts ” . In Land-Use and Land-Cover Change: Local Processes and Global Impacts , Edited by: Lambin , E.F. and Geist , H.J. 1 – 8 . Berlin , Heidelberg : IGBP Series. Springer-Verlag .
- Land Resources Department/Bina Program . 1990 . The Land Resources of Indonesia: A National Overview from Regional Physical Planning Programme for Transmigration (RePPProT) , Jakarta , , Indonesia : Land Resources Department, Natural Resources Institute, Overseas Development Administration, London; and Direktorat Bina Program, Direktorat Jenderal Penyiapan Pemukiman, Departemen Transmigrasi .
- Li , Z. and Kafatos , M. 2000 . Interannual Variability of Vegetation in the United States and Its Relation to El-Nino Southern Oscillation . Remote Sensing of Environment , 71 : 239 – 247 .
- Lloyd , D. 2000 . A Phenological Classification of Terrestrial Vegetation Cover Using Shortwave Vegetation Index Imagery . International Journal of Remote Sensing , 11 : 2269 – 2279 .
- Lobell , D.B. and Asner , G.P. 2004 . Cropland Distributions from Temporal Unmixing of MODIS Data . Remote Sensing of Environment , 93 : 412 – 422 .
- Loveland , T.R. , Merchant , J.W. , Ohlen , D.O. and Brown , J.F. 1991 . Development of a Land-Cover Characteristics Database for the Conterminous US . Photogrammetric Engineering and Remote Sensing , 57 : 1453 – 1463 .
- Lu , X. , Liu , R. and Liang , S. 2007 . Removal of Noise by Wavelet Method to Generate High Quality Temporal Data of Terrestrial MODIS Products . Photogrammetric Engineering and Remote Sensing , 73 : 1129 – 1139 .
- Lunetta , R.S. , Knight , J.F. , Ediriwickrema , J. , Lyon , J.G. and Worthy , L.D. 2006 . Land-Cover Change Detection Using Multi-Temporal MODIS NDVI Data . Remote Sensing of Environment , 105 : 142 – 154 .
- Mallat , S.G. 1989 . A Theory for Multiresolution Signal Decomposition: The Wavelet Representation . IEEE Transactions on Pattern Analysis and Machine Intelligence , 11 : 674 – 693 .
- Martinez , B. and Gilabert , M.A. 2009 . Vegetation Dynamics from NDVI Time Series Analysis Using the Wavelet Transform . Remote Sensing of Environment , 113 : 1823 – 1842 .
- Mathworks . 2009 . “Wavelet Toolbox User's Guide,” Matlab R2009b Documentation , The Mathworks, Inc .
- Ministry of Environment . 2008 . Menuju Indonesia Hijau 2008 , Jakarta , , Republic of Indonesia : The Deputy of Controlling for Environmental Damage and Climate Change, Ministry of Environment .
- Ministry of Public Works . 2002 . Research Institute for Water Resources Development , Jakarta , , Republic of Indonesia : Ministry of Public Works .
- MODLAND (2010), Vegetation Indices 16-Day L3 Global 250 m. https://lpdaac.usgs.gov/lpdaac/products/modis_products_table/vegetation_indices/16_day_l3_global250m/mod13q1 (http://https://lpdaac.usgs.gov/lpdaac/products/modis_products_table/vegetation_indices/16_day_l3_global250m/mod13q1) (Accessed: 29 May 2010 ).
- Nepstad , D.C. , Carvalho , C.R.D. , Davidson , E.A. , Jipp , P.H. , Negreiros , P.A.L.G.H. , Silva , T.S. , Trumbore , S.E. and Viera , S. 1994 . The Role of Deep Roots in the Hydrological and Carbon Cycles of Amazonian Forests and Pastures . Nature , 372 : 666 – 669 .
- Park , J. , Tateishi , R. and Matsuoka , M. 1999 . A Proposal of the Temporal Window Operation (TWO) Method to Remove High-Frequency Noises in AVHRR NDVI Time Series Data . Journal of the Japan Society of Photogrammetry and Remote Sensing , 38 : 36 – 47 .
- Philippon , N. , Jarlan , L. , Martiny , N. , Camberlin , P. and Mougin , E. 2007 . Characterization of the Interannual and Intraseasonal Variability of West African Vegetation between 1982 and 2002 by Means of NOAA AVHRR NDVI Data . Journal of Climate , 20 : 1202 – 1218 .
- Privette , J.L. , Myneni , R.B. , Knyazikhin , Y. , Mukelabai , M. , Roberts , G. , Tian , Y. , Wang , Y. and Leblanc , S.G. 2002 . Early Spatial and Temporal Validation of MODIS LAI Product in the Southern Africa Kalahari . Remote Sensing of Environment , 83 : 232 – 243 .
- Ramankutty , N. , Graumlich , L. , Achard , F. , Alves , D. , Chhabra , A. , DeFries , R.S. , Foley , J.A. , Geist , H.J. , Houghton , R.A. , Goldewijk , K.K. , Lambin , E.F. , Millington , A. , Rasmussen , K. , Reid , R.S. and Turner , B.L. II . 2006 . “ Global Land-Cover Change: Recent Progress, Remaining Challenges ” . In Land-Use and Land-Cover Change: Local Processes and Global Impacts , Edited by: Lambin , E.F. and Geist , H.J. 9 – 39 . Berlin , Heidelberg : IGBP Series. Springer-Verlag .
- Richards , J.A. and Jia , X. 1999 . Remote Sensing Digital Image Analysis: An Introduction , 3rd , Berlin : Springer .
- Rocchini , D. , Perry , G.L.W. , Salerno , M. , Maccherini , S. and Chiarucci , A. 2006 . Landscape Change and the Dynamics of Open Formation in a Natural Reserve . Landscape and Urban Planning , 77 : 167 – 177 .
- Sakamoto , T. , Nguyen , N.V. , Kotera , A. , Ohno , H. , Ishitsuka , N. and Yokozawa , M. 2007 . Detecting Temporal Changes in the Extent of Annual Flooding Within the Cambodia and the Vietnamese Mekong Delta from MODIS Time-Series Imagery . Remote Sensing of Environment , 109 : 295 – 313 .
- Sakamoto , T. , Yokozawa , M. , Toritani , H. , Shibayama , M. , Ishitsuka , N. and Ohno , H. 2005 . A Crop Phenology Detection Method Using Time-Series MODIS Data . Remote Sensing of Environment , 96 : 366 – 374 .
- Schwartz , M.D. 1999 . Surface Phenology and Satellite Sensor-Derived Onset of Greenness: An Initial Comparison . International Journal of Remote Sensing , 17 : 3451 – 3457 .
- Sellers , P.J. , Tucker , C.J. , Collatz , G.J. , Los , S.O. , Justice , C.O. and Dazlich , D.A. 1994 . A Global 1 by 1 NDVI Dataset for Climate Studies: Part 2. The Adjustment of the NDVI and Generation of Global Fields of Terrestrial Biophysical Parameters . International Journal of Remote Sensing , 15 : 3519 – 3545 .
- Shimada , S. , Takahashi , H. and Limin , S.H. 2006 . Hydroperiod and Phenology Prediction in a Central Kalimantan Peat Swamp Forest by Using MODIS Data . Tropics , 15 : 435 – 440 .
- State Gazette of the Republic of Indonesia . 1999 . Act of the Republic of Indonesia No. 41 Year 1999 , Jakarta : Author .
- Townshend , J.R.G. , Justice , C.O. and Kalb , V. 1987 . Characterization and Classification of South American Land Cover Types Using Satellite Data . International Journal of Remote Sensing , 8 : 1189 – 1207 .
- Tucker , C.J. , Townshend , J.R.G. and Goff , T.E. 1985 . African Land-Cover Classification Using Satellite Data . Science , 227 : 369 – 375 .
- Turner , B.L. II , Lambin , E.F. and Reenberg , A. 2007 . The Emergence of Land Change Science for Global Environmental Change and Sustainability . Proceedings of the National Academy of Sciences , 104 : 20666 – 20671 .
- USGS LP DAAC (2009a), Data Pool. https://lpdaac.usgs.gov/lpdaac/get_data/data_pool (http://https://lpdaac.usgs.gov/lpdaac/get_data/data_pool) (Accessed: 10 August 2009 ).
- USGS LP DAAC (2009b), MODIS Reprojection Tool, https://lpdaac.usgs.gov/lpdaac/tools/modis_reprojection_tool (http://https://lpdaac.usgs.gov/lpdaac/tools/modis_reprojection_tool) (Accessed: 10 August 2009 ).
- Van Dijk , A. , Callis , S.L. , Sakamoto , C.M. and Decker , W.L. 1987 . Smoothing Vegetation Index Profiles: An Alternative Method for Reducing Radiometric Disturbance in NOAA/AVHRR Data . Photogrammetric Engineering and Remote Sensing , 53 : 1059 – 1067 .
- Verburg , P.H. , Van De Steeg , J. , Veldkamp , A. and Willemen , L. 2009 . From Land Cover Change to Land Function Dynamics: A Major Challenge to Improve Land Characterization . Journal of Environmental Management , 90 : 1327 – 1335 .
- Verburg , P.H. , Veldcamp , A. , Willemen , L. , Overmars , K.O. and Castella , J.C. 2004 . Landscape Level Analysis of the Spatial and Temporal Complexity of Land-Use Change . Geophysical Monograph-American Geophysical Union , 153 : 217 – 230 .
- Viovy , N. , Arino , O. and Belward , A.S. 1992 . The Best Index Slope Extraction (BISE): A Method for Reducing Noise in NDVI Time-Series . International Journal of Remote Sensing , 13 : 1585 – 1590 .
- Watson , J.G. “Mangrove Forests of the Malay Peninsula,” . Malayan Forest Records No. 6 . 1928 . Forest Department, Federated Malay States, Kuala Lumpur
- Xiao , X. , Hagen , S. , Zhang , Q. , Keller , M. and Moore III , B. 2006 . Detecting Leaf Phenology of Seasonally Moist Tropical Forests in South America with Multi-Temporal MODIS Images . Remote Sensing of Environment , 103 : 465 – 473 .
- Xiao , X.M. , Braswell , B. , Zhang , Q.Y. , Boles , S. and Frolking , S. 2003 . Sensitivity of Vegetation Indices to Atmospheric Aerosols: Continental-Scale Observations in Northern Asia . Remote Sensing of Environment , 84 : 385 – 392 .
- Xiao , Z. , Liang , S. , Wang , J. , Song , J. and Wu , X. 2009 . A Temporally Integrated Inversion Method for Estimating Leaf Area Index from MODIS Data . IEEE Transactions on Geoscience and Remote Sensing , 47 : 2536 – 2545 .
- Zhang , X. , Friedl , M.A. , Schaaf , C.B. , Strahler , A.H. , Hodges , J.C.F. , Gao , F. , Reed , B.C. and Huete , A. 2003 . Monitoring Vegetation Phenology Using MODIS . Remote Sensing of Environment , 84 : 471 – 475 .