Abstract
Given growing concerns about biodiversity loss and carbon emissions stemming from tropical deforestation, it is important to identify the factors associated with land abandonment as they might indicate areas that were deforested but are unsuitable for long-term cultivation. This article utilizes a high-resolution, Landsat-based data-set called TerraClass to relate land abandonment to precipitation, slope, farmgate price of beef, market access, soil quality, and land tenure characteristics in the Brazilian Amazon. The spatially explicit statistical models reveal that rates of land abandonment increase with higher rainfall and slope values, even when controlling for distance to roads, farmgate prices, type of settlement, and soil quality. Moreover, land adjacent to a major road is more likely to be consistently cultivated, but land near large rivers and far from highways is more likely to be abandoned. Land ownership type also influences the likelihood of land abandonment as settlements based on communal property rights have higher rates of land abandonment than settlements with private lots. These results suggest that policies aimed at occupying tropical forests such as the Amazon must consider these biophysical and socioeconomic factors in order to foster human development aligned with sustainability principles.
1. Introduction
Tropical forests play a crucial role in the regulation of global climate through the carbon cycle and energy surface budget (Beer et al., Citation2010). These forests also harbor considerable biodiversity and provide resources for indigenous and traditional communities (Robinson & Redford, Citation1994). The Brazilian Amazon is of particular significance in this regard because of its tremendous size, encompassing 5 million km2 (Perz & Skole, Citation2003), and because the region has been plagued by high levels of deforestation over the past decades, although rates have declined in recent years. In the meantime, the Brazilian Amazon also became a major supplier of soybean and cattle products to both global and domestic markets, adding billions of dollars to the country’s economy (Garrett, Lambin, & Naylor, Citation2013; Walker, Browder, et al., Citation2009). Since the mid-1990s, the total value of Brazil’s agriculture has swelled over threefold (Cremaq, Citation2010) and secured Brazil as one of the world’s largest agriculture exporters. Brazil has risen to become the world’s second largest soybean exporter with annual exports of over 24 million tons (Richards, Myers, Swinton, & Walker, Citation2012). Concurrently, in 2007, Brazil overtook Australia to become the world’s largest beef producer, with roughly three-quarters of this expansion occurring at the expense of native Amazon forest (Bowman et al., Citation2012).
Given the economic and ecological importance of these mutually exclusive land covers (i.e., forest, and crops or pastures), it is important to identify factors indicative of land abandonment, forest regrowth or underutilization because such areas were deforested but were not kept in production, thus generating little economic benefits. Areas similar to these could be the target of policies such as reducing emissions from deforestation and forest degradation (REDD) programs because they have the potential to be converted back to forest without imposing a large opportunity cost to society. Conservation biologists and land change scientists have addressed this issue from the ecological and economic perspectives (Börner & Wunder, Citation2008; Harvey, Dickson, & Kormos, Citation2010; Nepstad et al., Citation2009). The purpose of this article was to add to this literature by considering the biophysical (e.g., slope, climate and soils) and socioeconomic factors behind land abandonment, thus contributing to the debate on how to exploit our natural resources more sustainably by avoiding the unnecessary debasement of natural systems.
Land abandonment and forest regrowth result from the interplay of both socioeconomic and biophysical factors (Perz & Skole, Citation2003; Redo, Grau, Aide, & Clark, Citation2012; Yackulic et al., Citation2011). Socioeconomic factors, such as income, job opportunities in the service sector, agricultural technology utilization, prices of inputs and outputs, and migration, shift the economic rent curves among different land uses thereby hindering or promoting the utilization and occupation of agricultural and forested lands (Pfaff & Walker, Citation2010; Rudel, Perez-Lugo, & Zichal, Citation2000). Biophysical factors are notable in this regard because they influence both the likelihood that native forests will be deforested in the first place and the potential of sustained agricultural production through time (Brannstrom, Citation2000; Koulouri & Giourga, Citation2007; Sluiter & Jong, Citation2007).
Our article quantifies the magnitude that biophysical and certain socioeconomic factors play in land abandonment by employing spatially explicit econometric models. To date, statistical analyses conducted to investigate this issue in the Amazon have relied on coarse units of observation defined by political boundaries, typically either at the municipality or Brazilian census tract level (Chomitz & Thomas, Citation2003; Perz & Skole, Citation2003). Although political boundaries are convenient spatial entities that are easily manipulated within a geographic information system (GIS) and whose attributes can be merged to demographic and socioeconomic variables, the aforementioned level of aggregation results in a loss of information (Goodchild, Citation1993). In addition, these census-based studies depended on reported land uses and land covers rather than remotely sensed, observed data. The difference between reported and remotely sensed data can be quite significant (Geoghegan et al., Citation2001). Although a few studies have utilized data from remotely sensed imagery, they are constrained to a smaller extent covered by roughly 20 Landsat scenes, a small fraction of the 227 scenes necessary to cover the whole Brazilian Amazon (Lucas, Honzak, Amaral, Curran, & Foody, Citation2002; Mello & Alves, Citation2011). This article makes use of more precise data obtained through remote sensing that results in a set of observations that is orders of magnitudes larger than ones used in previous studies (i.e., million compared to hundreds) to provide a basin-wide, high-resolution analysis of the entire Brazilian Amazon.
The article is organized as follows. Following this introduction, we discuss three potential sources of land abandonment, namely choice under uncertainty, forest transition theory, and the use of slash-and-burn agricultural techniques. We then present conceptual models for relating biophysical and socioeconomic factors to land abandonment. Next, we describe the methods and data sources. Lastly, we present the results of the models, followed by a discussion about the implications of our findings.
2. Contextual background
Previous research has linked land abandonment and forest regrowth to three major sources (1) imperfect information regarding biophysical endowments which erroneously instigated deforestation of marginal lands (Barham & Coomes, Citation1996; Brannstrom, Citation2000), (2) forest transition theory (Mather, Citation1992), and (3) slash-and-burn agriculture. We briefly address these three frameworks below.
In a world under perfect competition and complete information, classical economics dictates that agents make optimal decisions in terms of land allocation and use and would only deforest if expected profits are positive. Expectations, however, can be distorted by inaccurate or incomplete information, particularly in frontier settings where, by definition, knowledge regarding physical attributes of the region and appropriateness for agriculture are yet to be fully understood. Informational deficiencies can also manifest in the form of incomplete information regarding past environmental conditions and/or an inability to accurately predict future climatic events (Bacic, Bregt, & Rossiter, Citation2006).
Historically, imperfect information regarding the frontier has encouraged settlement in marginally productive agricultural lands. This lack of understanding, in turn, has triggered numerous instances of devastating social and environmental ramifications. Most notably, the Dust Bowl experience of the United States highlights the repercussions of unrealistic expectations governing the endowments of the land and climate (Cook, Miller, & Seager, Citation2009). Similar examples of failed agricultural endeavors are well documented throughout history, including experiences within post-Soviet Russia (O’Hara, Citation1997; Prishchepov, Müller, Dubinin, Baumann, & Radeloff, Citation2013) and South Africa (Phillips, Citation1999).
In tropical environments, willingness to deforest land for commercial purposes is directly tied to the agents’ perceived notion of how much rent they will earn from cultivation. Yet, agents often decide to deforest primary vegetation with imperfect information about the land’s suitability for agricultural production (Brannstrom, Citation2000).
Once landholders realize that their deforested land is no longer profitable to cultivate, they abandon it. Land is often of poor quality for cultivation when the climate is too wet (Chomitz & Thomas, Citation2003), or too dry (Marangos & Williams, Citation2005), or the soil is nutrient-deficient (Witcover, Vosti, Carpentier, & De Araújo Gomes, Citation2006). These particular biophysical constraints increase the agents’ vulnerability to negative shocks, such as reduction in agricultural prices or bad weather, leading to land abandonment. In Australia, for example, climate change has shifted the reliability and prediction of rainfall, which has caused reductions in agricultural production and increased levels of land abandonment (Marangos & Williams, Citation2005). Just like the United States’ Dust Bowl experience, farmers are witnessing prolonged periods of unsuitable environmental conditions, inconsistent with their initial expectations.
Land abandonment has also been studied through the framework of forest transition theory, a long-term process wherein a region’s forest cover initially decreases in extent to make way for logging, pasture, or agriculture, and then undergoes a sustained expansion due to abandonment of agricultural fields (Grainger, Citation1995; Mather, Citation1992; Mather & Needle, Citation1998; Walker, Citation1993). Studies have shown that socioeconomic drivers such as migration (Rudel et al., Citation2000), domestic trade (Pfaff & Walker, Citation2010), international trade (Walker, Citation1993), crop market changes (Alves, Escada, Pereira, & De Albuquerque Linhares, Citation2003), capital scarcity (Perz & Skole, Citation2003), and increased competition (Angelsen, Citation2007) all play a role in a forest transition. For instance, as a nation undergoes economic development, rural members of society migrate to the urban centers, which subsequently reduces the pressure on agricultural land and encourages forest regrowth. Biophysical factors also play a role in forest transition theory. In the United States, for example, agricultural lands with marginal productivity in the northeast transitioned to forests once the Midwest’s more productive lands were brought into production. Moreover, rising labor costs in turn raised the cost of agriculture such that it become cost-prohibitive to continue cultivation on these less productive, marginal lands (Barbier, Burgess, & Grainger, Citation2010; Pfaff & Walker, Citation2010; Rudel et al., Citation2005).
In nations where rates of deforestation have decreased following industrialization, researchers have identified instances of forest expansion occurring. Thus far, many advanced industrial nations have undergone well-documented forest transitions (Gellrich & Zimmermann, Citation2007; Mather, Citation2004; Pfaff & Walker, Citation2010). Additionally, a number of recent studies have alluded to the prospect of a forest transition occurring within the developing world (Grau & Aide, Citation2008; Meyfroidt, Rudel, & Lambin, Citation2010). As key forest transition indicators, such as urbanization and industrialization, have increased within the Amazon, researchers have even entertained the notion of a forest transition within Brazil (Pfaff & Walker, Citation2010).
Land abandonment can also be temporary as part of a rotation system used to restore soil fertility. In tropical regions, shifting or swidden agriculture is practiced by many small-scale farmers to clear forests for agriculture without intensive use of technology or capital (Fujisaka, Bell, Thomas, Hurtado, & Crawford, Citation1996). This traditional method of farming is dependent on alternating periods of crop production with vegetation fallow. Smallholders first cut down the forest, let the biomass dry for a few months and then burn it to clear the land and to create a temporary injection of nutrients into otherwise poor-quality soils (Tomich, Van Noordwijk, Vosti, & Witcover, Citation1998). This fertility is short-lived, however, because without chemical fertilizers the soil becomes nutrient-deficient for additional cultivation after 1–2 years (Silva-Forsberg & Fearnside, Citation1997). Because smallholders often lack the capital to invest in technology or hire additional labor to combat these hurdles, they abandon the depleted land in favor of a different site with sufficient biomass that can be converted into fertilizing ashes (Metzger, Citation2002). Following a period of fallow in which vegetation recovers and accumulates nutrients, slash-and-burn practitioners revisit a site and the process commences anew (Silva-Forsberg & Fearnside, Citation1997).
The fallow period, or temporary land abandonment, may last from 2 to 30 years and can also be related to agroclimatic characteristics. For instance, heavy rainfall following burns can cause rapid leaching or runoff and may significantly reduce soil fertility, thereby reducing the viability of the cultivation cycle (Silva-Forsberg & Fearnside, Citation1997). Prolonged rainfall seasons with short dry periods can also hinder the proper drying of the cut plant material prior to the burn, which can reduce the biomass ash conversion.
Although the literature provides the sources and theoretical underpinnings of land abandonment, less is known about the relative impact of the various biophysical and socioeconomic factors on land abandonment in the Amazon. A notable exception includes the study by Chomitz and Thomas (Citation2003) who assessed the effect of precipitation as a bioclimatic factor, on the likelihood of deforestation and cattle stocking density in the Amazon. Chomitz and Thomas’ econometric models show that the rate of deforestation and cattle stocking density decline with increasing rainfall levels, holding other attributes constant.
Although pioneering, their research did not address land abandonment and was ultimately limited by coarse, census-level data, where land uses are based on census reporting, not direct observation. On the other hand, other studies based on fine remote sensing data covered only a small extent and therefore did not contain enough biophysical heterogeneity (e.g., variation in rainfall) that is required for inferential analysis. Thus, a data source that accurately measures the amount of forest regrowth for a large extent is critical to properly assess the biophysical factors leading to land abandonment. Within the Amazon biome, ecosystem recovery and vegetation regrowth can take place at a rapid rate. When abandoned and left fallow, former agricultural or pastoral land is recolonized by native vegetation (d’Oliveira, Alvarado, Santos, & Carvalho, Citation2011; Uhl & Jordan, Citation1984). We take this vegetative regrowth as an indicator of abandonment, either temporary or permanent. Thus, for purposes of this article, land abandonment is defined as land previously deforested that is left to its own spontaneous dynamics (Baudry, Citation1991), which eventually leads to observed signs of regrowth and regeneration through the lens of remote sensors.
3. Conceptual model and methods
Our conceptual model aims to explain land abandonment as a function of biophysical characteristics such as rainfall, soil type, and topography and socioeconomic factors such as farmgate beef prices and agricultural settlements. Our hypothesis is that certain biophysical attributes limit agricultural productivity and therefore decrease the likelihood of continued agricultural use of the land, which leads to abandonment. We also hypothesize that higher farmgate beef prices create higher rents, which incentivize more intensive use of the land, thereby decreasing abandonment. A third hypothesis is that abandonment is more prevalent among smallholder farmers, given that many of them adopt slash-and-burn agriculture.
These hypotheses are tested through the implementation of a statistical model where the proportion of abandonment y in a given unit of observation extent i is a linear function of both biophysical factors, socioeconomic variables, and other control variables X. Since the proportion y is left-censored at zero and right-censored at 100%, this leads to a two-sided Tobit model where the observed response variable y is expressed in terms of the underlying latent variable:
The vector contains the coefficients to be estimated and
is the error term, assumed to be normally distributed. The unit of observation for this study is a 1 km2 grid cell (see below). The dependent variable, y, represents the proportion of abandonment occurring within this 1 km2 grid cell that has experienced at least partial deforestation (see below).
It seems plausible that a given observation i may show a proportion of abandonment similar to nearby observations. To take this potential spatial dependency effect in censored observations into account, we also estimated a spatial autoregressive probit model as follows (LeSage & Pace, Citation2009)
To compare against the Tobit regressions, we also report the ordinary least squares (OLS) and generalized linear model (GLM) regression results; the latter has been developed to estimate proportional or fractional response models such as ours (Papke & Wooldridge, Citation1996). To estimate this model, we make use of a probit link function of the binomial family.
3.1. Digital data-sets
The dependent variable was derived from a processed data-set called TerraClass that was produced by Brazil’s Instituto Nacional de Pesquisas Espaciais (INPE) and Empresa Brasileira de Pesquisa Agropecuária (EMBRAPA). The data used in the statistical analyses covers the Legal Amazon; a political denomination that includes nine states (see ). At 30-m spatial resolution, this Landsat TM-based product classifies each pixel into 15 land-use/land-cover (LULC) categories, separated for the years 2008 and 2010. TerraClass shows that 79.4% of the Legal Amazon was still forested in 2008, 17.5% was deforested, and roughly 3% of the area was classified as water. provides descriptive statistics of each LULC in 2008 for the subset used in our analysis. Significant land-cover types for the study area include 35.4% forest, 29.2% pasture, and 5.1% agriculture. A novel aspect of the TerraClass data-set is that pixels are classified into more cover types than the customary forest-deforested binary categories. TerraClass includes three regeneration classes that represent areas of land abandonment. In 2008, they include:
Dirty pasture (Pasto sujo: 5.5% total study area): areas indicating between 50% and 80% of herbaceous vegetation cover and species of grasses. The remaining 20% to 50% is associated with sparse shrub cover.
Regeneration with pasture (Regeneração com pasto: 4.2% total study area): areas that are early in the process of native vegetation regeneration. These areas show dominance of colonizing tree and shrub species.
Secondary vegetation (Vegetação secundária: 13.1% total study area): areas that are in an advanced process of shrubby vegetation regeneration. These areas are characterized by a high diversity of plant species.
Figure 1. Overview of study area and independent variables. Legal Brazilian Amazon (A), average yearly precipitation over a 10-year (1999–2008) period (B), predominant soil types (C), elevation (D), farmgate price of beef (E), and INCRA settlements (F).
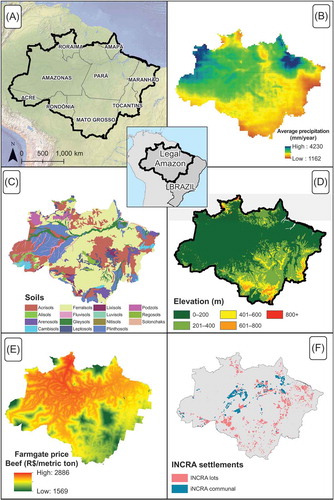
Table 1. Land-use and land-cover categories included in the TerraClass data-set.
The dependent variable, proportion of abandonment (y), is therefore given by: y = (area abandoned)/(all other deforestation land uses + abandonment). The denominator is not always equal to the 1 km2 grid size because water and cells that were not classified, due to cloud cover or shade, were not factored into the calculation (see below). provides an overview of the proportion of abandonment occurring in the study area. In 2008, an area of 261,866 km2 was categorized as abandoned and in 2010 that number rose to 285,669 km2, for an increase of 8.4%. The rise of abandonment in 2010 came from areas that in 2008 were pasture (52.5%), agriculture (34.0%), and recently deforested (8.2%). The remaining area was not categorized in 2008 due to cloud cover.
3.2. GIS processing
We first created a 1 km2 polygon layer covering the extent of our study area, which was used within a GIS to calculate the proportion of abandonment and generate our regression variables. We chose 1 km2 because this resolution is a good compromise between level of detail and computational feasibility as the original 30-m data-set for the whole Amazon contains 5.38 billion cells. Another incentive for aggregating into 1 km2 cells is to make the measurement ‘abandonment’ more consistent and robust. One individual 30-m cell may switch from one year to another based on factors that sometimes may be random or a product of misclassification, creating a lot of noise. But through aggregation, this measurement is more indicative of systematic abandonment over a larger extent.
Within a GIS, the TerraClass data-set, in vector format, was intersected with the 1 km2 layer to produce a standardized data-set of the study area for both 2008 and 2010. Each individual polygon was then assigned the percentage of LULC class within the square (). This method of aggregation has the advantage of computational efficiency while maintaining the fine-scale information contained in the original data-set.
We excluded from the analysis grid cells outside the forest biome, particularly those in the natural savannas or cerrado in the eastern Legal Amazon. Although the cerrado is an ecologically important biome and falls within the Legal Amazon, our intent is to model the Amazon forest biome as illustrated in . Even with the exclusion of the cells in the intensively farmed cerrado, the ‘Arc of Deforestation’ is still clearly visible along the south and eastern front of the Amazon in the states of Rondônia, Mato Grosso, Pará, and Tocantins. Areas along major rivers and primary roads also show significant signs of human impact.
For the statistical analyses, we used solely the 1 km2 grid cells that showed at least some level of deforestation and discarded all the cells that did not present any deforestation or other agricultural use of land. In order for land to undergo abandonment and regrowth, the land must first be deforested. Thus, abandonment is conditional on prior deforestation. To see this, we can write the definition of condition probability of abandonment A given deforestation D as . If we had used the full sample, then we would have to model not only abandonment but also the probability of a given pixel being deforested. By restricting the sample space to include only cells with deforestation, we simplify the statistical analysis by essentially setting P(D) = 1. The resulting population of interest was comprised of 1,141,814 individual 1 km2 grid cells.
By conditioning for prior deforestation, we account for areas that have low levels of land abandonment merely because there is less deforestation to begin with in these regions. We believe that the drivers of abandonment are much less understood than the drivers of deforestation, particularly at the scale of the Amazon. As a supplementary analysis, we ran a multinomial logistic regression to investigate the drivers behind deforested land-cover types, abandoned lands, and forest. In the interest of space and because this model strays from the core of the present article, the results are available in the Appendix.
Ideally, our analysis of land abandonment would include a variable that depicts ‘time since clearing’. However, to our knowledge, Amazon-wide, multidecadal, high-resolution data-sets do not exist for various reasons such as completeness (e.g., lack of images due to cloud cover), computational feasibility, human and financial resource constraints, and other factors. To measure the extent to which biophysical factors are related to land abandonment, we needed heterogeneity for key variables (e.g., precipitation, soils, terrain), which is only possible to obtain by making the extent larger, an opportunity made possible by the release of the TerraClass data-sets. To test that the general pattern of abandonment is consistent between the years 2008 and 2010, we created a new data-set comprising the grid cells that showed what we call persistent abandonment during this time span (). Although the 2-year difference between data-sets is not enough to identify permanent abandonment or even temporary abandonment, e.g., shifting cultivation, the overall spatial pattern of abandonment has remained largely unchanged during this period. At this 1 km2 scale, virtually 100% of the 1 km2 grids showed abandonment in both the 2008 and 2010 data-sets. Almost 47% of grid cells showed increase in abandonment, while 45% showed decrease. The average change in proportion abandoned for the cells with reported increase or decrease was 13% and −11%, respectively.
We used two approaches to assign values of the independent variables discussed below to each 1 km2 observation. For the continuous variables, such as rainfall and slope, we used a weighted average based on the intersection between the 1 km2 grid and the variable in question. For instance, if 70% of the grid received 2000 mm of rain and the other 30% received 1800 mm, then the assigned value was 1940 mm (2000*0.7 + 1800*0.3). For categorical values, we first converted the vector files to a 1-km raster using the nearest neighbor interpolator and then assigned the value directly to the grids. provides the descriptive statistics for each explanatory variable.
Table 2. Descriptive statistics for the explanatory variables.
3.2.1. Precipitation
High levels of precipitation have been shown to be associated with less deforestation and lower cattle ranching productivity in the Amazon, presumably because of higher rates of land abandonment (Chomitz & Thomas, Citation2003). Precipitation measurements were garnered via the Tropical Rainfall Measuring Mission (TRMM) with a 0.25° spatial resolution, product 3B43 version 7. Using raster algebra, we converted the hourly rainfall values to total rainfall per month and produced a 10-year (1999–2008) average rainfall raster, which was then assigned to each 1 km2 cell. This decadal rainfall average is a proxy for climate and a reliable indicator for the climatic conditions leading to abandonment. We also assigned to each cell the variability in annual rainfall. Variations in rainfall rates impact the consistency of soil moisture available for agriculture, consequently creating crop production risks (Fitzjarrald et al., Citation2008; Kurukulasuriya & Rosenthal, Citation2003). To generate this variable, we created a raster of the coefficient of variation, defined as the ratio between the standard deviation and mean of annual precipitation (1998–2011), all multiplied by 100. The Amazon exhibits a distinct increase in precipitation values from south to north and west to east, except for higher rainfall rates along the Atlantic Coast in northern Pará state. shows the amount of precipitation across the entire Amazon. Average precipitation values for the study area range from a relatively dry 1345 mm/year in the southern section of Mato Grosso state to a maximum of 4230 mm/year in the humid northwest corner of Amazonas state.
3.2.2. Slope and elevation
Land with higher slope values will likely have higher abandonment percentages either because the soil is more likely to be eroded by rain or because slopes hamper the use of machinery necessary to restore soil productivity (Uhl & Mattos, Citation1994). Slope values in degrees were calculated in a GIS using the Horn algorithm from a 90-m digital elevation model obtained from the Shuttle Radar Topography Mission (USGS, Citation2009). Slope values for each 1 km2 cell averaged 2.78° over the entire study area, with a high of 43.04° and a standard deviation of 2.59°. Elevation values consistently showed little change over the extent of our study area (95% of the cells showed elevation below 400 m). The relief fluctuates locally, e.g., rolling hills, but at the basin scale, the study area is relatively flat. This local variability is captured in the slope variable.
3.2.3. Soils
Land abandonment is more likely to occur in regions with nutrient-deficient soils because these soils impede agriculture sustainability (Perz & Skole, Citation2003). The cultivation of the Amazon’s tropical soil requires intensive preparation and labor if slash-and-burn technology is adopted or large amounts of capital for fertilizer and machinery in the case of commercial agriculture (Witcover et al., Citation2006). Fifteen soil types were obtained from the Harmonized World Soil Database (FAO/IIASA/ISRIC/ISSCAS/JRC, Citation2009) at 30 arc-second resolution (). Within each 1 km2 cell, ferralsol (sometimes referred to as oxisol) soil groups comprise a majority of the soil at 50.3%. Ferralsols are characterized as strongly weathered red or yellow soils with a chemically poor, but physically stable, subsoil. Acrisols (ultisols) are the second largest representation at 24.0% and are typified as a soil with subsurface accumulation of low activity clays and low base saturation (FAO/IIASA/ISRIC/ISSCAS/JRC, Citation2009). The remaining 13 catalogued soil types averaged less than 10% per 1 km2 cell.
3.2.4. Farmgate prices and distance to roads and rivers
Von Thünen’s bid rent theory specifies that transportation costs impact the price of agricultural goods, and consequently, high transportation costs decrease rent (Von Thünen, Citation1966). Based on these expectations, it is hypothesized that land abandonment is more likely to occur in regions far from primary roads and major rivers where farmgate prices do not meet costs. Since planted and abandoned pastures account for 80% of all cleared lands, we created a variable called ‘farmgate beef price’ within a GIS that is a proxy for land rents. Prices of beef for 2010 (in Brazilian reais per ton) were garnered from interviews with farmers, from meat packing plants, and from published agricultural almanacs (AnualPec Citation2003, Citation2009; Arima et al., Citation2011). The farmgate price was calculated by subtracting transportation costs from the price of beef at known locations (e.g., meat packing plants and slaughterhouses). Transportation costs were calculated using the least cost distance functions in a GIS that took into account the cost of traversing a raster cell, according to the mode of transportation and quality and type of infrastructure. As an alternative to farmgate prices, we also used in the regressions the Euclidean distance to primary roads (Instituto Brasileiro de Geografia e Estatística [IBGE], Citation2003) and major rivers (IBGE, Citation1999). These were calculated using built-in functions within a GIS. By including the variables distance to primary roads and rivers, we also attempt to control for the fact that we observe more agriculture in the drier part of the basin. The average farmgate price for each cell is R$ 2505/metric ton of beef and the average distance to a primary road from a cell is roughly 15 km, while the distance from any one grid to a major river is about 22.8 km.
3.2.5. States
We included state binary variables to control for any state-level factors that might impact land abandonment. For example, certain states might have a more effective agricultural extension service than others that may lead to higher or lower observed abandonment. Although Amazonas is the largest state in the Legal Amazon, due to its low absolute amount of deforestation, it ranked fifth in the number of cells included in our study (n = 108,801). Pará contained the most cells (n = 384,818) and Roraima the fewest (n = 23,932). Cells within Acre had the highest proportion of abandonment with a mean of 75.9% and Mato Grosso the fewest at 35.9%.
3.2.6. INCRA settlements
For many smallholders, abandonment is characteristic of their agricultural system, i.e., slash-and-burn and pousio (fallow) techniques. To capture whether cells containing smallholders are more likely to have abandonment, we used GIS data from Brazil’s colonization agency, the Instituto Nacional de Colonização e Reforma Agrária (INCRA). This data-set contains all settlements managed by INCRA, including colonization projects, settlement projects, and sustainable development projects, among others. We created two categorical variables based on whether land within an INCRA settlement is separated into individual lots and is privately held or whether land within a given settlement is predominately communal and/or has restrictions on deforestation. The first INCRA variable, called ‘INCRA Lots’, contains the following settlement projects: assentamento, assentamento conjunto, assentamento dirigido, casulo, estadual, and integrado de colonização. The second INCRA variable, called INCRA communal, contains the following settlement types: florestal, agroextrativista, quilombola, and desenvolvimento sustentável. For a description of each type of settlement, please refer to Instituto Sócio-Ambiental (ISA) (Citation2014). The omitted category represents cells not within INCRA’s jurisdiction.
3.2.7. Protected areas
The percentage of land under protected status within each grid cell was initially calculated but ultimately excluded from all models. Although protected areas make up a sizeable portion of the Amazon (Walker, Moore, et al., Citation2009), they are not relevant as an explanatory factor of abandonment for two reasons. First, secondary forest regrowth within protected areas was relatively low (1.48%) compared to areas outside their borders (10.06%). This is likely on account of the limitations placed on development within a protected area. Second, the forces dictating forest regrowth within previously cleared plots in a protected area are inherently different from land outside of a protected area’s borders. Land use within a protected area is potentially prone to abandonment due to land tenure complications rather than cultivation potential.
3.3. Partial effects interpretation
The assessment of the impact of each biophysical variable on land abandonment is tantamount to calculating the partial effects of those variables on the dependent variable. Interpreting the partial effects of a Tobit regression is not as straightforward as reading the coefficient values directly from an OLS regression (Wooldridge, Citation2012) because our interest lies in the observed outcome y, not on the latent variable y*. Thus, for the sake of pedagogical clarity, we calculate the estimated value of y for incremental values of each choice variable, one at a time, while holding all the other control variables constant at their mean values, and plot the partial effects in graphs (). For a two-sided Tobit model, the expected value is:
Thus, substituting the population parameters by the regression coefficients in the equation yields the predicted values.
4. Results
The statistical models generally support the hypotheses that biophysical and socioeconomic factors are correlated with the proportion of land abandonment in the Amazon. The objective of this analysis is not to predict the proportion of land abandonment but rather to highlight the significance and magnitude of the factors associated with abandonment. The importance of the model is not necessarily to provide an exact replica of the data-set (i.e., high pseudo-R2), but instead to obtain consistent estimators of the impact of each biophysical and socioeconomic factor on abandonment and thus a consistent assessment of partial effects. Results of the models are outlined in and mapped in and . Graphs showing the partial effect of the explanatory variables on the proportion of abandonment are shown in . Tobit 1 is a static look at the proportion of abandonment for cells in 2008 only, while the rest of the models test cells that were persistently abandoned in both 2008 and 2010.
Table 3. Model results for the proportion of land abandonment.
Figure 5. Spatial Probit 2 estimated probability, taken to be the predicted proportion of land abandonment.
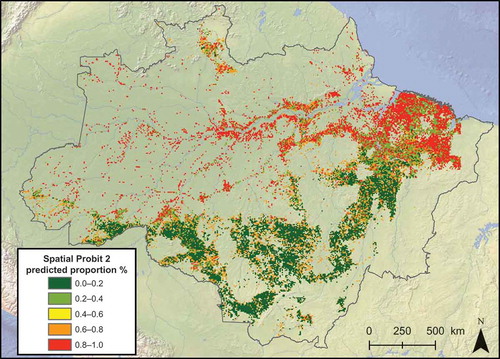
Figure 6. The partial effect of precipitation (A), slope (B), distance to major rivers (C), precipitation variability (D), and distance to primary roads (E) on the proportion of land abandonment.
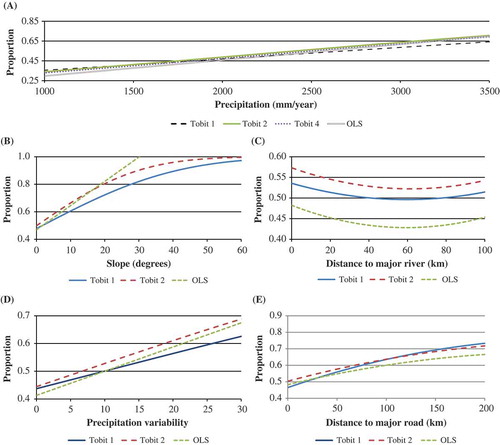
4.1. Determinants of land abandonment
Each of our regression models finds a statistically significant positive correlation between precipitation and the proportion of abandonment. These results imply that land abandonment rises with increased rainfall. The four Tobit models exhibit similar trajectories and predictions. An annual rainfall rate of 1300 mm yields a level of abandonment of 39% (Tobit 1), 38% (Tobit 2), 39% (Tobit 3), and 37% (Tobit 4) of the land in agricultural use within a 1 km2 plot (holding all the other variables constant at mean value – i.e., ceteris paribus). As rainfall increases to a humid 3000 mm/year, the proportion of land that was deforested and abandoned increases to 59% (Tobit 1), 64% (Tobit 2), 63% (Tobit 3), and 63% (Tobit 4). Likewise, the OLS model presents a similar range of 37% to 60%. These findings match aforementioned studies that show lower productivity of agriculture in very high rainfall locations. These moist regions have a high prevalence of pests and diseases which interfere with the well-being of livestock and crops (Sombroek, Citation2001). High levels of rainfall might also favor the rapid recolonization of native vegetation, particularly through the suppression of fire (Uhl & Kauffman, Citation1990). Like annual precipitation rates, our models also indicate that rainfall variability is positively correlated with abandonment. These results suggest that land abandonment increases in areas with higher year-to-year fluctuations of precipitation. Rainfall variability from season to season affects the availability of soil moisture for crops and surface water for cattle and thus poses agriculture production risks for farmers, leading to increases in land abandonment.
The models also reveal a strong positive relationship between proportion of abandonment and terrain slope. According to the models, slope values over 45° yield abandonment rates close to 100%. An advantage of the two-sided Tobit over the OLS model is also clearly demonstrated here. The OLS model predicts that for slope values over 30°, the 1 km2 cell will contain over 100% proportion of abandonment, an unrealistic situation. Most likely, this higher proportion of abandonment on steep slopes is related to the difficulty in reestablishing pasture productivity, which requires mechanized machinery to plow, reseed, and fertilize the soil (Freitas, Hawbaker, & Metzger, Citation2010).
The Tobit 3 and Spatial Probit 2 models show a positive relationship between the proportion of abandonment and farmgate prices, contrary to our original hypothesis. Upon further inspection, we noticed that high farmgate prices are observed along navigable rivers near Manaus (Amazonas state) and Boa Vista (Roraima) because of high beef prices in those two locations and cheap water transportation costs (Arima, Barreto, & Brito, Citation2006).Those two states also contain higher levels of abandonment along rivers thus driving the positive effect for farmgate beef prices. In order to obtain a clearer understanding of this phenomenon, we substituted the distance to road and distance to river variables for farmgate prices in subsequent models.
Although most soil types were significant at the 0.05 significance level, they exhibited little to no partial effect on the proportion of land abandonment, with respect to the omitted variable. In some cases, the existence or absence of a particular soil type only affected the proportion of abandonment by a few hundredths of a percent. This circumstance is likely due to the relatively coarse spatial resolution of the soil data. The relationship between soil quality and land abandonment is widely documented, and with a higher resolution soil data-set, the magnitude of that effect could be better quantified.
The proportion of land abandonment with respect to the distance to major rivers is represented by a downward-shaped curve, with each of the models that include this variable having negative coefficients for the distance to rivers level form and positive for the quadratic variable. The OLS model exhibits an accelerated reduction of abandonment over the Tobit models, but they all exhibit similar trajectories. From 0 km up to 80 km, which comprises 95% of the observed values, the proportion of abandonment decreases as the distance to major rivers increases. This phenomenon indicates that abandonment is more likely to occur in the floodplains of large rivers but is less common on terra firme.
In contrast to the distance to major rivers, the proportion of land abandonment with respect to the distance to primary roads consistently increases as the distance increases, with all of the models showing positive coefficients for the level term and negative for the quadratic form. Within the immediate vicinity of a major road, Tobit 2 predicts the proportion of abandonment at 50% once a cell is deforested, ceteris paribus. At a distance of 100 km from a principal road, the predicted proportion of land abandonment increases to 63%. These results reemphasize the well-established notion that roads increase agricultural rents, thereby favoring continuous agricultural use of land (Pfaff et al., Citation2007). Road construction reduces transportation costs and possibly attracts more capitalized farmers who can afford to sustain land productivity (Walker, Defries, et al., Citation2009). Areas near roads are more likely to be in a perpetual state of deforestation, whereas areas far from roads are more likely to be abandoned after being deforested.
The binary variables for the Brazilian states were statistically significant. With respect to the omitted variable Pará, the coefficients for Mato Grosso, Acre and Rondônia had negative signs, indicating that the models predict the proportion of abandonment to be lower in those states.
With respect to the INCRA settlements, all of the models predict the proportion of abandonment to decrease in cells that are within an INCRA settlement where lots are privately owned, compared to the omitted variable representing cells that are not settlements. This result is surprising and contradicts our initial assumption that abandonment would be more prevalent within smallholders’ settlements. One explanation might be related to the large number of smallholders who either are still not legally settled by INCRA or do not belong to settlements and therefore were included in the omitted category. It is also possible that our initial assumption is incorrect. Smallholders in these private-based settlements are highly integrated to the market economy and therefore likely to hire labor to clear and restore pastures (Walker & Arima, Citation2011).
The opposite effect is observed for cells that are within an INCRA settlement where land is primarily communal and/or has some restrictions on deforestation. This result is expected because subsistence agriculture based on slash-and-burn agriculture is likely to be more predominant in ‘communal’ types of settlements.
As for the spatial probit models, the results show that the coefficients’ signs, or the direction of the effects, are consistent with the other models and are also statistically significant. The spatial autoregressive parameter ρ is also significant indicating spatial dependency even for samples 8 km apart. The spatial model yields better predictive ability than the nonspatial models. For Spatial Probit 2, the correlation between predicted and actual rates of abandonment is 0.48. This better match can be visualized by comparing the shaded maps in and .
In all, the regression results are remarkably consistent in terms of direction of the coefficients’ effect. The magnitudes of the partial effects for the variables of interest were also robust across specifications.
5. Discussion and conclusion
The results provide strong evidence that biophysical factors, settlement-type variables, and river adjacency are significantly related to land abandonment rates. Rainfall and slope in particular showed a strong and positive correlation with the proportion of deforested area abandoned, even when controlling for distance to roads, farmgate prices, time of settlement, and soil quality. These results corroborate previous findings that economic returns to agriculture in marginal lands are lower than in drier, flatter areas of Amazonia. With respect to the distance to transportation networks, the results support the notion that land adjacent to a major road is likely to be under perpetual cultivation. The propensity for land abandonment is lower for INCRA settlements that are under private control than for settlements based on communal arrangements.
It could be argued though that the effect of precipitation on abandonment is largely being driven by the northeastern part of the Legal Amazon, a region that was settled by Europeans in early colonial periods and where precipitation levels are also high. The results could be an artifact of a spurious relationship whereby higher levels of abandonment are in fact caused by exhausted soil fertility due to long settlement, not because of rainfall. To test the robustness of this precipitation–abandonment relationship, we removed this region from the analysis (total of 150,000 km2) and reran the Tobit regression with the remaining observations (, Tobit 4). The results still show a strong effect of precipitation on abandonment and suggest that this effect is not driven by regional forces but is basin-wide.
Overall, the statistical analyses derive three important policy implications for future development plans in the Amazon and tropical regions in general.
First, policies aimed at encouraging agricultural expansion through the construction of roads into forested regions characterized by high levels of rainfall may result in no-win outcomes in terms of both ecological impacts and agricultural production. Apart from the fact that roads deteriorate faster when subject to extreme rainfall, these roads might encourage deforestation only for temporary gains as evidenced by the large proportion of deforested lands showing signs of abandonment in those areas. On the other hand, the results also suggest that the construction of roads in drier areas of the Amazon might favor permanent conversion of forest into agricultural uses with little posterior abandonment, particularly in the case of northern Mato Grosso state (). In these areas, the assessment of trade-offs between economic development and ecosystem services benefits supplied by forests should be a priority for future research, given the suitability of those lands for agriculture and biological importance of the remaining forests (Capobianco et al., Citation2001; Walker, Defries, et al., Citation2009).
Second, recent attempts to decrease protection of forests in Brazil along rivers and steep slopes may not be appropriate for the Amazonian context, particularly if the primary agricultural use is planted pasture or mechanized agriculture. In Brazil, ranchers and farmers are required to maintain a riparian buffer of 30–600 m, depending on the width of the river, and are not allowed to deforest on top of hills and on slopes above 45°, a mandate established by the 1965 Forestry Code. Although the new code approved in 2012 does not change these numbers substantially, political pressure from agricultural interests to reduce those parameters almost succeeded (Tollefson, Citation2012). Our results show that the rate of abandonment along rivers and steep slopes is quite high, confirming the need for enforcement of current environmental legislation.
Finally, these results may be useful to inform market-based initiatives to reduce deforestation and degradation (e.g. REDD), on account of their importance in the overall strategy to mitigate carbon emissions. Our results show that areas located in marginal lands, characterized by high rainfall levels, steep slopes, and nearness to water would be good candidates for such programs given the small economic opportunity costs attached to their agricultural use, relative to their high return of ecosystem services in the form of carbon storage. Obviously, these programs should, in addition, target areas that show positive probabilities of deforestation, which was not modeled in this work.
The statistical results also raise some interesting issues for future research. For instance, the difference in abandonment levels between the two INCRA settlement types requires further investigation. Although we speculate about potential causes for those differences, only in loco field work can pinpoint the actual factors that are driving more or less land abandonment.
On the political and scientific front, we want to emphasize the importance of continued production of time series LULC data-sets during times when governments face budgetary cuts and fiscal adjustments. Only these types of data-sets, released at regular intervals, will allow analysts to tackle the more general and long-term issue(s) of forest transition and agricultural sustainability. We praise the high-quality, high-resolution land-use classification for such a large extent provided by INPE/EMBRAPA and hope that this effort continues for decades to come.
Despite recent reductions in deforestation rates, substantial land change is likely to continue in the Brazilian Amazon for the foreseeable future. Increased investments in hydropower and road paving could lead to a rebound of deforestation in areas unsuitable for sustained agricultural production (Nazareno & Lovejoy, Citation2011). This study provides insights into the biophysical and socioeconomic attributes that lead to land abandonment after deforestation and may facilitate planning and policy to foster sustainable development practices. Thus, it is up to governments at different levels to avoid creating incentives that lead to myopic and short-sighted decisions by individual agents. To support a more sustainable use of resources, governments should encourage settlers to leave the forest in its original condition rather than deforest, abandon the land, and let vegetation regrow.
Acknowledgements
We would like to thank two anonymous referees who provided excellent comments on earlier versions of the manuscript. We remain responsible for any outstanding errors.
Disclosure statement
No potential conflict of interest was reported by the authors.
Additional information
Funding
Notes
1. We opted to use the function sarp_g (spatial probit) instead of sart_g (spatial Tobit) from LeSage’s library because the latter allows for either upper or lower censoring, not both. This model was estimated in the University of Texas at Austin’s Stampede supercomputer.
References
- Alves, D. S., Escada, M. I. S., Pereira, J. L. G., & De Albuquerque Linhares, C. (2003). Land use intensification and abandonment in Rondônia, Brazilian Amazônia. International Journal of Remote Sensing, 24(4), 899–903. doi:10.1080/0143116021000015807
- Angelsen, A. (2007). Forest cover change in space and time: Combining the von Thünen and forest transition theories (Working Paper No. 4117). Retrieved from http://go.worldbank.org/1R6K4W2A61
- AnualPec. (2003). Anuario da Pecuaria Brasileira. São Paulo: Instituto FNP.
- AnualPec. (2009). Anuario da Pecuaria Brasileira. São Paulo: Instituto FNP.
- Arima, E., Barreto, P., & Brito, M. (2006). Cattle ranching in the Amazon: Trends and implications for environmental conservation. Belém: IMAZON.
- Arima, E., Richards, P., Walker, R., & Caldas, M. (2011). Statistical confirmation of indirect land use change in the Brazilian Amazon. Environmental Research Letters, 6(2), 024010. doi:10.1088/1748-9326/6/2/024010
- Bacic, I., Bregt, A., & Rossiter, D. (2006). A participatory approach for integrating risk assessment into rural decision-making: A case study in Santa Catarina, Brazil. Agricultural Systems, 87, 229–244. doi:10.1016/j.agsy.2005.01.008
- Barbier, E. B., Burgess, J. C., & Grainger, A. (2010). The forest transition: Towards a more comprehensive theoretical framework. Land Use Policy, 27(2), 98–107. doi:10.1016/j.landusepol.2009.02.001
- Barham, B. L., & Coomes, O. T. (1996). Prosperity’s promise: The Amazon rubber boom and distorted economic development. Boulder, CO: Westview Press.
- Baudry, J. (1991). Ecological consequences of grazing extensification and land abandonment: Role of interactions between environment, society and techniques. Options Mediterraneennes. Serie A: Seminaires Mediterraneens, 15, 13–19.
- Beer, C., Reichstein, M., Tomelleri, E., Ciais, P., Jung, M., Carvalhais, N., … Papale, D. (2010). Terrestrial gross carbon dioxide uptake: Global distribution and covariation with climate. Science, 329(5993), 834–838. doi:10.1126/science.1184984
- Börner, J., & Wunder, S. (2008). Paying for avoided deforestation in the Brazilian Amazon: From cost assessment to scheme design. International Forestry Review, 10(3), 496–511. doi:10.1505/ifor.10.3.496
- Bowman, M. S., Soares-Filho, B. S., Merry, F. D., Nepstad, D. C., Rodrigues, H., & Almeida, O. T. (2012). Persistence of cattle ranching in the Brazilian Amazon: A spatial analysis of the rationale for beef production. Land Use Policy, 29(3), 558–568. doi:10.1016/j.landusepol.2011.09.009
- Brannstrom, C. (2000). Coffee labor regimes and deforestation on a Brazilian frontier, 1915–1965*. Economic Geography, 76, 326–346. doi:10.2307/144390
- Capobianco, J. P. R., Veríssimo, A., Moreira, A., Sawyer, D., Dos Santos, I., & Pinto, L. P. (2001). Biodiversidade na Amazônia brasileira. São Paulo: Instituto Socioambiental.
- Chomitz, K. M., & Thomas, T. S. (2003). Determinants of land use in Amazonia: A fine-scale spatial analysis. American Journal of Agricultural Economics, 85(4), 1016–1028. doi:10.1111/1467-8276.00504
- Cook, B. I., Miller, R. L., & Seager, R. (2009). Amplification of the North American “Dust Bowl” drought through human-induced land degradation. Proceedings of the National Academy of Sciences of the United States of America, 106(13), 4997–5001. doi:10.1073/pnas.0810200106
- Cremaq, P. (2010). Brazilian agriculture: The miracle of the Cerrado. The Economist, p. 26.
- d’Oliveira, M. V. N., Alvarado, E. C., Santos, J. C., & Carvalho Jr., J. A. (2011). Forest natural regeneration and biomass production after slash and burn in a seasonally dry forest in the Southern Brazilian Amazon. Forest Ecology and Management, 261(9), 1490–1498. doi:10.1016/j.foreco.2011.01.014
- FAO/IIASA/ISRIC/ISSCAS/JRC. (2009). Harmonized world soil database (version 1.1). Rome: FAO.
- Fitzjarrald, D. R., Sakai, R. K., Moraes, O. L., Cosme De Oliveira, R., Acevedo, O. C., Czikowsky, M. J., & Beldini, T. (2008). Spatial and temporal rainfall variability near the Amazon-Tapajós confluence. Journal of Geophysical Research: Biogeosciences (2005–2012), 113(G1), G00B11. doi:10.1029/2007JG000596
- Freitas, S. R., Hawbaker, T. J., & Metzger, J. P. (2010). Effects of roads, topography, and land use on forest cover dynamics in the Brazilian Atlantic Forest. Forest Ecology and Management, 259, 410–417. doi:10.1016/j.foreco.2009.10.036
- Fujisaka, S., Bell, W., Thomas, N., Hurtado, L., & Crawford, E. (1996). Slash-and-burn agriculture, conversion to pasture, and deforestation in two Brazilian Amazon colonies. Agriculture, Ecosystems & Environment, 59(1–2), 115–130. doi:10.1016/0167-8809(96)01015-8
- Garrett, R. D., Lambin, E. F., & Naylor, R. L. (2013). The new economic geography of land use change: Supply chain configurations and land use in the Brazilian Amazon. Land Use Policy, 34, 265–275. doi:10.1016/j.landusepol.2013.03.011
- Gellrich, M., & Zimmermann, N. E. (2007). Investigating the regional-scale pattern of agricultural land abandonment in the Swiss mountains: A spatial statistical modelling approach. Landscape and Urban Planning, 79(1), 65–76. doi:10.1016/j.landurbplan.2006.03.004
- Geoghegan, J., Villar, S. C., Klepeis, P., Mendoza, P. M., Ogneva-Himmelberger, Y., Chowdhury, R. R., & Vance, C. (2001). Modeling tropical deforestation in the southern Yucatan peninsular region: Comparing survey and satellite data. Agriculture, Ecosystems and Environment, 85, 25–46. doi:10.1016/S0167-8809(01)00201-8
- Goodchild, M. F. (1993). Data models and data quality: Problems and prospects. In M. F. Goodchild, B. O. Parks, & L. T. Steyaert (Eds.), Environmental modeling with GIS (pp. 8–15). New York, NY: Oxford University Press.
- Grainger, A. (1995). The forest transition: An alternative approach. Area, 27(3), 242–251. doi:10.2307/20003580
- Grau, H. R., & Aide, M. (2008). Globalization and land-use transitions in Latin America. Ecology and Society, 13, 16. doi:10.1057/9780230603554
- Harvey, C. A., Dickson, B., & Kormos, C. (2010). Opportunities for achieving biodiversity conservation through REDD. Conservation Letters, 3(1), 53–61. doi:10.1111/j.1755-263X.2009.00086.x
- Hausman, J., & McFadden, D. (1984). Specification tests for the multinomial logit model. Econometrica: Journal of the Econometric Society, 52(5), 1219–1240.
- Hosmer Jr., D. W., & Lemeshow, S. (2004). Applied logistic regression. New York, NY: John Wiley & Sons.
- Instituto Brasileiro de Geografia e Estatística (IBGE). (1999). hidrografia_principal. Retrieved from http://downloads.ibge.gov.br/downloads_geociencias.htm
- Instituto Brasileiro de Geografia e Estatística (IBGE). (2003). estradas_principal_ibge. Retrieved from http://downloads.ibge.gov.br/downloads_geociencias.htm
- Instituto Sócioambiental (ISA). (2014). Modalidade dos projetos de assentamentos criados pelo INCRA na atualidade. Retrieved from http://www.socioambiental.org/banco_imagens/pdfs/DefinioTiposdeAssentamentoeCrditos.pdf
- Koulouri, M., & Giourga, C. (2007). Land abandonment and slope gradient as key factors of soil erosion in Mediterranean terraced lands. Catena, 69(3), 274–281. doi:10.1016/j.catena.2006.07.001
- Kurukulasuriya, P., & Rosenthal, S. (2003, June). Climate change and agriculture: A review of impacts and adaptations world bank climate change series paper no. 91. Paper prepared and published for the Rural Development Group and Environment Department of the World Bank, Washington, DC.
- LeSage, J. (1999). Spatial econometrics. Retrieved December 15, 2003, from http://www.spatial-econometrics.com/
- LeSage, J., & Pace, R. K. (2009). Introduction to spatial econometrics. Boca Raton, FL: CRC Press.
- Lucas, R. M., Honzak, M., Amaral, I. D., Curran, P. J., & Foody, G. M. (2002). Forest regeneration on abandoned clearances in central Amazonia. International Journal of Remote Sensing, 23(5), 965–988. doi:10.1080/01431160110069791
- Marangos, J., & Williams, C. (2005). The effect of drought on uncertainty and agricultural investment in Australia. Journal of Post Keynesian Economics, 27(4), 575–594.
- Mather, A. S. (1992). The forest transition. Area, 24(4), 367–379. doi:10.2307/20003181
- Mather, A. S. (2004). Forest transition theory and the reforesting of Scotland. Scottish Geographical Journal, 120, 83–98. doi:10.1080/00369220418737194
- Mather, A. S., & Needle, C. L. (1998). The forest transition: A theoretical basis. Area, 30(2), 117–124. doi:10.1111/j.1475-4762.1998.tb00055.x
- Mello, A. Y. I., & Alves, D. S. (2011). Secondary vegetation dynamics in the Brazilian Amazon based on thematic mapper imagery. Remote Sensing Letters, 2(3), 189–194. doi:10.1080/01431161.2010.506206
- Metzger, J. P. (2002). Landscape dynamics and equilibrium in areas of slash-and-burn agriculture with short and long fallow period (Bragantina region, NE Brazilian Amazon). Landscape Ecology, 17(5), 419–431. doi:10.1023/A:1021250306481
- Meyfroidt, P., Rudel, T. K., & Lambin, E. F. (2010). Forest transitions, trade, and the global displacement of land use. Proceedings of the National Academy of Sciences of the United States of America, 107(49), 20917–20922. doi:10.1073/pnas.1014773107
- Nazareno, A. G., & Lovejoy, T. E. (2011). Energy production: Giant dam threatens Brazilian rainforest. Nature, 478(7367), 37. doi:10.1038/478037b
- Nepstad, D., Soares, B. S., Merry, F., Lima, A., Moutinho, P., Carter, J., … Stella, O. (2009). The end of deforestation in the Brazilian Amazon. Science, 326(5958), 1350–1351. doi:10.1126/science.1182108
- O’Hara, S. L. (1997). Agriculture and land reform in Turkmenistan since independence. Post-Soviet Geography and Economics, 38(7), 430–444. doi:10.1080/10889388.1997.10641056
- Papke, L. E., & Wooldridge, J. M. (1996). Econometric methods for fractional response variables with an application to 401(k) plan participation rates. Journal of Applied Econometrics, 11, 619–632. doi:10.1002/(SICI)1099-1255(199611)11:6<619::AID-JAE418>3.0.CO;2-1
- Perz, S. G., & Skole, D. L. (2003). Secondary forest expansion in the Brazilian Amazon and the refinement of forest transition theory. Society & Natural Resources, 16(4), 277–294. doi:10.1080/08941920390178856
- Pfaff, A., Robalino, J., Walker, R., Aldrich, S., Caldas, M., Reis, E., … Kirby, K. (2007). Road investments, spatial spillovers, and deforestation in the Brazilian Amazon. Journal of Regional Science, 47, 109–123. doi:10.1111/j.1467-9787.2007.00502.x
- Pfaff, A., & Walker, R. (2010). Regional interdependence and forest “transitions”: Substitute deforestation limits the relevance of local reversals. Land Use Policy, 27(2), 119–129. doi:10.1016/j.landusepol.2009.07.010
- Phillips, S. T. (1999). Lessons from the dust bowl: Dryland agriculture and soil erosion in the United States and South Africa, 1900–1950. Environmental History, 4, 245–266. doi:10.2307/3985305
- Prishchepov, A. V., Müller, D., Dubinin, M., Baumann, M., & Radeloff, V. C. (2013). Determinants of agricultural land abandonment in post-Soviet European Russia. Land Use Policy, 30(1), 873–884. doi:10.1016/j.landusepol.2012.06.011
- Redo, D. J., Grau, H. R., Aide, T. M., & Clark, M. L. (2012). Asymmetric forest transition driven by the interaction of socioeconomic development and environmental heterogeneity in Central America. Proceedings of the National Academy of Sciences, 109, 8839–8844. doi:10.1073/pnas.1201664109
- Richards, P. D., Myers, R. J., Swinton, S. M., & Walker, R. T. (2012). Exchange rates, soybean supply response, and deforestation in South America. Global Environmental Change, 22(2), 454–462. doi:10.1016/j.gloenvcha.2012.01.004
- Robinson, J. G., & Redford, K. H. (1994). Measuring the sustainability of hunting in tropical forests. Oryx, 28(4), 249–256. doi:10.1017/S0030605300028647
- Rudel, T. K., Coomes, O. T., Moran, E., Achard, F., Angelsen, A., Xu, J., & Lambin, E. (2005). Forest transitions: Towards a global understanding of land use change. Global Environmental Change, 15(1), 23–31. doi:10.1016/j.gloenvcha.2004.11.001
- Rudel, T. K., Perez-Lugo, M., & Zichal, H. (2000). When fields revert to forest: Development and spontaneous reforestation in post-war Puerto Rico. The Professional Geographer, 52(3), 386–397. doi:10.1111/0033-0124.00233
- Silva-Forsberg, M. C., & Fearnside, P. M. (1997). Brazilian Amazonian caboclo agriculture: Effect of fallow period on maize yield. Forest Ecology and Management, 97(3), 283–291. doi:10.1016/S0378-1127(97)00070-4
- Sluiter, R., & Jong, S. (2007). Spatial patterns of Mediterranean land abandonment and related land cover transitions. Landscape Ecology, 22(4), 559–576. doi:10.1007/s10980-006-9049-3
- Sombroek, W. (2001). Spatial and temporal patterns of Amazon rainfall. Consequences for the planning of agricultural occupation and the protection of primary forests. Ambio, 30, 388–396.
- Tollefson, J. (2012). Brazil set to cut forest protection. Nature, 485(7396), 19. doi:10.1038/485019a
- Tomich, T. P., Van Noordwijk, M., Vosti, S. A., & Witcover, J. (1998). Agricultural development with rainforest conservation: Methods for seeking best bet alternatives to slash-and-burn, with applications to Brazil and Indonesia. Agricultural Economics, 19(1), 159–174. doi:10.1016/S0169-5150(98)00032-2
- Uhl, C., & Jordan, C. F. (1984). Succession and nutrient dynamics following forest cutting and burning in Amazonia. Ecology, 65(5), 1476–1490. doi:10.2307/1939128
- Uhl, C., & Kauffman, J. B. (1990). Deforestation, fire susceptibility, and potential tree responses to fire in the eastern Amazon. Ecology, 71, 437–449. doi:10.2307/1940299
- Uhl, C., & Mattos, M. M. (1994). Economic and ecological perspectives on ranching in the Eastern Amazon. World Development. doi:10.1016/0305-750X(94)90066-3
- USGS. (2009). Shuttle radar topography mission (SRTM) - “finished.” United States Geological Survey. Reston, VA: Author.
- Von Thünen, J. H. (1966). Isolated state: An English edition of Der isolierte Staat. Oxford: Pergamon Press.
- Walker, R. (1993). Deforestation and economic development. Canadian Journal of Regional Science, 16(3), 481–497.
- Walker, R., & Arima, E. Y. (2011). Smallholder timber sales along the Transamazon Highway: A comment. Ecological Economics, 70(9), 1565–1567. doi:10.1016/j.ecolecon.2010.11.018
- Walker, R., Browder, J., Arima, E., Simmons, C., Pereira, R., Caldas, M., … Zen, S. (2009). Ranching and the new global range: Amazônia in the 21st century. Geoforum, 40, 732–745. doi:10.1016/j.geoforum.2008.10.009
- Walker, R., Defries, R., Vera-diaz, M. C., Shimabukuro, Y., Keller, M., Bustamante, M., … Silva Dias, (2009). The expansion of intensive agriculture and ranching in Brazilian Amazonia. In Amazonia and Global Change Geophysical Monograph Series, 186, 61–81. doi:10.1029/2008gm000735
- Walker, R., Moore, N. J., Arima, E., Perz, S., Simmons, C., Caldas, M., … Bohrer, C. (2009). Protecting the Amazon with protected areas. Proceedings of the National Academy of Sciences, 106(26), 10582–10586. doi:10.1073/pnas.0806059106
- Witcover, J., Vosti, S. A., Carpentier, C. L., & De Araújo Gomes, T. C. (2006). Impacts of soil quality differences on deforestation, use of cleared land, and farm income. Environment and Development Economics, 11, 343–370. doi:10.1017/S1355770X0600283X
- Wooldridge, J. M. (2012). Introductory econometrics: A modern approach. Mason, OH: Cengage Learning.
- Yackulic, C. B., Fagan, M., Jain, M., Jina, A., Lim, Y., Marlier, M., … Uriarte, M. (2011). Biophysical and socioeconomic factors associated with forest transitions at multiple spatial and temporal scales. Ecology and Society, 16(3), 15. doi:10.5751/ES-04275-160315
Appendix. Multinomial logit model
Given that deforestation and abandonment are inextricably linked, we perform a multinomial logistic regression that compares areas of abandonment with deforested areas and stable forest cover. Multinomial logistic regression models are used when the dependent variable contains two or more categories (Hosmer & Lemeshow, Citation2004). For our study, deforested land-use categories and land abandonment are compared to a base category, forest cover. The application of a multinomial logistic model relies on the independence of irrelevant alternatives (IIA) assumption to hold true. Under the IIA assumption, we would expect no systematic modification to the model’s coefficients if we exclude one of the outcomes from the model. To test for a violation of the IIA we conducted a Hausman test (Hausman and McFadden 1984).
The dependent variable for the multinomial logistic regression was the categorical land use/land cover from the 2010 TerraClass data-set at 30-m resolution. Here, we adopted a stratified random sampling by drawing two random cells from within each of the 1 km2 grid, for a total of approximately 10 million sample observations. The same independent variables were used as those employed in the other statistical models; however, these variables were taken at their original resolutions, e.g., 90 m for the elevation and slope values. Indigenous territories and conservation units are included because they encompass forested land cover, which is a part of the population of interest for this particular model. Conservation units make up approximately 23% of the cells, and indigenous territories represent 21% of the total cells.
The results of the multinomial regression () reflect the possibilities of three types of land uses in relation to a stable forest as the base layer: agriculture, pasture, or abandonment. Relative to forest cover, instances of deforestation from agriculture and pasture land uses are expected to decrease with an increase in slope, while land abandonment increases with higher slope values. The model also predicts that all classes of deforestation decrease as a function of annual precipitation and precipitation variability. Contrary to our other models, in this model, instances of abandonment decrease with increased annual precipitation rates. This phenomenon occurs because there is less initial deforestation in the most humid regions of the Amazon, a situation that we accounted for in the previous models by conditioning for deforestation.
As demonstrated in the literature, and reflected by the multinomial logistic model, conservation areas and indigenous territories inhibit deforestation as shown by their negative coefficients (Walker, Moore, et al., 2009). Land abandonment is also expected to be lower in these areas. The type of land ownership also has a statistically significant influence on land cover. INCRA settlements based on communal property rights, many of which have restrictions on deforestation, have less occurrences of pasture and land abandonment than settlements with private lots. The model shows that instances of deforestation and land abandonment decrease as the distance to a primary road increases. This negative effect is expected for deforestation as deforestation is uncommon in the remote regions of the Amazon where access to the market is inaccessible. Like the negative relationship between annual precipitation rates and land abandonment, the negative correlation between the distance to roads variable and land abandonment is due to the fact that very little deforestation occurs in the remote areas of the Amazon, and consequently, there are few instances of abandonment.
The results of the multinomial logistic model confirm many of the well-known factors behind deforestation in tropical forests. Notably, protected areas inhibit deforestation, while areas adjacent to major roads experience more deforestation. However, the model’s prediction of land abandonment is less reliable owing to the lack of conditioning for prior deforestation.
Table A1. Model results for the multinomial logistic regression. Forest cover used as base category.